Introduction
The surface morphology of the ice sheets at scales of one to a few ice thicknesses is a result of the interaction of moving ice and a rough or sticky bed. Quantitative mapping of the small-scale surface morphology can therefore be used to evaluate the character of the ice-bed interaction and bed topography, and is an important input parameter for models of ice How (e.g. Reference Fastook, Brecher and HughesFastook and others, 1995). In addition, wind features, such as snow megadunes (Reference SwithinbankSwithinbank, 1988; Reference Bromwich, Parish and ZormanBromwich and others, 1990), can in some areas be a component of the surface morphology at the kilometer scale. However, current knowledge of the topography of the interior of ice sheets, represented by radar-altimetry-based digital elevation models (DEMs) for the most part, is too coarse spatially to represent these features.
Satellite imagery provides a path to greater spatial resolution. Several recent studies using Landsat, Système probatoire pour l'observation de la terre (SPOT) and the advanced very high-resolution radiometer (AVHRR) show that subtle topographic features are easily seen in enhanced satellite images (Reference Bindschadler and VornbergerBindschadler and Vornberger, 1990; Reference Seko, Furukawa, Nishio and WatanabeSeko and others, 1993; Reference Scambos and NeresonScambos and Nereson, 1995). Numerous studies have discussed the small-scale topography, or “undulation field”, visible in satellite imagery as an indicator of bed topography or basal resistance variations (e.g. Reference Dowdeswell and McIntyreDowdeswell and McIntyre, 1987; Reference Seko, Furukawa, Nishio and WatanabeSeko and others, 1993).
Photoclinometry, sometimes referred to as “shape from shading”, exploits the fact that the brightness of a visible or near-infrared image pixel is a function of surface reflectivity and slope orientation with respect to the Sun. This relationship between slope and brightness is called the “photometric function” (Reference McEwenMcEwen, 1991). In the case of the polar ice sheets, several factors combine to make the general problem of photoclinometry simpler. Snow-covered ice sheets have a more uniform albedo than most terrestrial surfaces. The variation in reflectance with respect to illumination and viewing angle is well behaved if the snow is fresh and free of surface frost, and for near-nadir viewing a Lambertian, or perfectly diffuse, reflectance pattern is a good approximation (Reference SteffenSteffen, 1987; see also Reference WarrenWarren, 1982). Since slopes over much of the interior are low relative to summertime solar elevation, no shadowing occurs (crevassed surfaces are an exception, and pose a problem as discussed below).
The AVHRR sensor is well suited to the problem. AVHRR has high radiometric resolution in the visible/near-infrared bands, which in this case translates into a sensitivity to small slope changes. The polar regions are imaged several times per day by AVHRR sensors on the U.S. National Oceanic and Atmospheric Administration (NOAA) polar orbiters, providing image data at several solar azimuths for any region (including the poles themselves, something not possible with Landsat or SPOT). Although the moderate resolution of the AVHRR sensor (1.1 km pixel size at best) is often cited as a drawback for applying il to mapping problems, in the case of the polar ice sheets many of the Emportant features, such as surface undulations, flow structures, ridges, ice-stream margin scars, etc., are at least a few kilometers in scale. Further, AVHRR data for the polar regions are available at no or low cost from several sources.
Polar photoclinometry has been discussed in several publications, most pertinently by Reference Bindschadler and VornbergerBindschadler and Vornberger (1994) and Reference CooperCooper (1994). Cooper's approach is a simplified method that, based on the assumptions of low slope and a Lambertian photometric relationship, takes a single image and converts it into a field of values linearly related to elevation. The result is intended to be used as a guide for mapping true surface elevations by providing information useful for extrapolation of widely spaced elevation data. Bindschadler and Vornberger use Landsat Thematic Mapper data to derive a detailed elevation map within a grid of airborne laser altimetry data. They derive the photofunction from the laser elevation data and spatially filtered image data (although they also discuss the derivation of the relationship by absolute means). The Bindschadler and Vornberger approach is similar to ours, but they use a single image and rely on the altimetry data to provide slope information in the cross-Sun direction.
Photoclinometry
A general discussion of photoclinometric theory as applied to ice sheets is given in Reference Bindschadler and VornbergerBindschadler and Vornberger (1994). With our assumption of a Lambertian reflectance pattern for fresh snow in a near-nadir satellite image, the photoclinometric equation becomes

(equation (1) of Bindschadler and Vornberger) where DN is the raw sensor value, or “data number”, C is a constant of conversion from radiance units to sensor units, I is the incident illumination at the surface, R is the reflectivity, θ is the angle between the surface normal and the solar incidence vector, L 0 is the minimum radiance threshold for the sensor, and T is the radiance at the sensor from all sources other than the surface, such as atmospheric scattering. This equation is equivalent to the simple equation for a linear relationship with respect to cos θ,

(equation (11) of Bindschadler and Vornberger), where A = CIR and B = C(-L 0 + T). If the simplifying assumption of Lambertian reflectance is valid, we should see a linear relationship between DN and cos θ.
Method
In our approach, we consider the ice-sheet topography as a combination of two continuous surfaces: a low-spatial-frequency surface at the resolution of the existing DEM for a region; and a higher-frequency surface (though still smoothed due to pixel sampling) that contains details not represented in the DEM but resolved by the imagery (Fig. 1). In order to resolve slopes in all directions, two images having different solar azimuths must be used; ideally, images with solar azimuths separated by 90°. Slope information in the images is calibrated by comparing low-pass-filtered image data with the slopes represented in the DEM, i.e. by empirically determining the photometric function using image data blurred to the scale of the existing slope knowledge. With two images acquired with near-perpendicular Sun azimuths, two nearly perpendicular slope vectors may be determined for each pixel location using the image photofunctions, from which the absolute slope in an image x, y coordinate system may be derived. However, the images must be accurately co-registered so that the slope vectors for a given pixel in each image correspond to the same patch of surface. Integrating the slopes provides the elevations of the higher-frequency surface not resolved by the DEM. Adding this information to the DEM provides an improved representation of the surface.

Fig. 1. Cartoon illustrating our model of the ice-sheet surface for purposes of photoclinometry.
Having described this overview of the process, let us review the steps in detail. An example photometric relationship covering part of northeast Greenland is given in Figure 2. The y axis of this plot is the sensor value for the NOAA-14 AVHRR channel 1 scene of this area spatially smoothed to 25 km scale. The x axis is solar surface incidence angles derived from a recent DEM covering the area produced by the Danish Cadastral Survey, Kort- og Matrikelstyrelsen (KMS; see Reference EkholmEkholm, 1996) and knowledge of sun position at the time the image was acquired. The incidence angles are determined by taking the gradient of the DEM surface in the solar azimuth direction. They include the variation of solar elevation due to curvature of the earth (more precisely, curvature of the World Geodetic System 1984 (WGS84) ellipsoid).
Although the KMS DEM dataset is reported with a gridcell spacing of approximately 2 km, the actual resolution of the surface defined by the data is considerably coarser over the northern part of the ice sheet (personal communication from Reference EkholmS. Ekholm, 1997). A series of visual comparisons of the blurred image data with surfaces derived from the DEM, and a comparison of the DEM with recent airborne laser altimetry data, were used to set the scale of the image blurring for the empirical photometric determination. We found the best match to be about 25 km. A blurring, or low-pass, spatial filter equal to 25 km ground scale was applied to the image data.
Tests show that channel 1 of the AVHRR (covering the spectral range 0.55-0.9 μm) has the best-correlated relationship with surface incidence angle, better than channel 2 or any combination of the two. The other channels (3, 4 and 5) are dominated by thermal emissions. We assume the problem with channel 2 is due to the greater effect of grain-size variations in the spectral range 0.73—1.1 μm (see Reference WarrenWarren, 1982). Correlation between low-pass-filtered channel 1 images and surface incidence angle cosine varied from 0.99 to 0.94 (r 2 value) relative to a linear best-fit equation through the data. The Lambertian approximation is justified.
With this level of correlation, the precision of a single-pixel surface slope determination is set primarily by the sensor's quantization (for AVHRR, the sensor noise level is below the quantization; see Hastings and Emery, 1992 and references therein). A ± 0.5 DN range equates to a slope precision of +0.7 m km for the photometric equation of Figure 2. Accuracy of the slope determinations is derived from the accuracy of the DEM's representation of a smoothed version of the true surface. If the DEM is accurate, then the photometric function should provide an accurate empirical slope calibration of the satellite image.
Having determined the photometric function for the two images, we then apply them to the images and begin the co-registration process. Initially, the images are geo-registered to the same earth projection, in this case a polar stenographic projection, using the orbit ephemeris information. The ephemeris data are not perfect, nor are small satellite pointing errors accounted for, so two images of the same area are typically misregistered by 1-4 km. To precisely co-register the images, we test the continuity of the surface they define. Only a unique relative position of the images, and the slope fields they represent, will define a smooth, continuous surface, i.e. one that has no non-differentiable. steps or edges (as we assume the ice surface to be). Such a surface has a minimum residual elevation in any closed-loop path through the slope vectors (it would be a zero residual elevation if the images, photometric functions, and registrations were perfect). A program was written to systematically shift the slope fields (images) over one another, calculate the absolute slopes in the image. x, y directions and evaluate the residual elevations in a series of closed loops across the scene. The co-registration vector was taken to be the shift that resulted in the minimum mean residual elevation. This approach gave a very sensitive measure of registration (Fig. 3). To facilitate co-registering to greater precision, the image data were resampled to 500 in equivalent pixel scale. At this scale, our residual error in a closed loop surrounding one pixel (a 4 km closed path through 8 pixels) was typically 0.75 m.
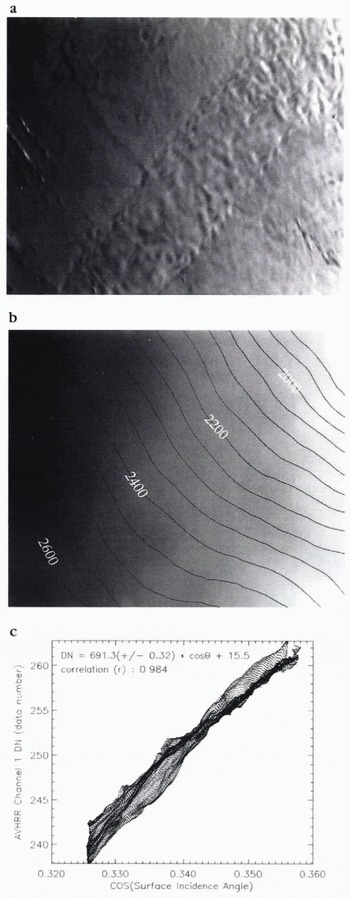
Fig. 2. (a) AVHRR image subscene of northeast Greenland near the ice-stream feature described in Fahnestock and others (1993, 1997). The subscene is centered on 76° 45′ N, 33° 0′ W, and covers an area of 215 km by 175 km. The image was acquired at 0812 h on 18 May 1995 (NSIDC scene ID a_tms_950518_0812). (b) Low-pass filtered version of the same image with contours from KMS DEM overlain. (c) Plot of brightness values (digital numbers, or DN) from channel 1 vs cosine of surface solar incidence angle for the AVHRR sub-scene. The equation refers to ta best-fit line through the data. The outer lines are limits imposed prior to determining the photofunction equation to eliminate clouds, rocks and shadows (none were present in this scene).

Fig. 3. Mean residual elevations (m) for an eight-pixel closed loop, given a range of registration vectors for the two images, x and y axes are in pixel units relative to an initial registration vector determined from the satellite pointing and orbit data.
With the two slope fields co-registered and converted into absolute slope vectors in the image x, y directions, the slopes may be converted into elevations. This is a mathematical problem with a long history (sec Reference HurtHurt, 1991). In general, it is not possible to convert a field of slope values to a field of z values for arbitrarily large grids, nor does there appear to be a kernel-based approach in the literature we have examined. However, in this case, the problem is again simplified by seeking only to add detail to an existing DEM surface. We use a straightforward approach, starling in the middle of the scene and calculating elevation differences between adjacent pixel centers, multiplying mean slope by the spatial separation of the pixels. It should not matter which of the absolute slope components (x or y direction) we use to convert slopes to elevations. The results of slope conversion to elevation for both the x and y components of slope are shown in Figure 4a and b.
This approach to converting slope to elevation allows errors derived from noise in the images to accumulate in a random-walk fashion as the elevation calculation proceeds from the center to the edge of the image, giving the curtain-like appearance to the elevation fields in Figure 4a and b. To mitigate this, we constrain our derived elevation field to be zero-mean at the scale of the DEM, In other words, we use a linear high-pass filter in the direction of integration on the elevation images to reduce the scale over which the random-walk error can accumulate (Fig. 4c and d). The scale of the filtering is the same as the spatial scale of the DEM, 25 km. At spatial scales greater than 25 km, we assume that the DEM is a perfectly accurate representation of the surface; features smaller than that spatial scale are derived from photoclinometry. Random-walk errors accumulate, on average, in proportion to the square root of the number of “steps”. With a filter scale of 25 km, the random-walk error in the elevation-determination step is constrained to about ✓12.5 ? 0.7 m (the elevation error due to a 0.5 DN noise per pixel), or ~2.5 m. This is the approximate scale of the remaining curtain-like elevation errors in Figure 4c and d. We reduce this error still further by taking the average of the two elevation fields (x-direction-derived and y-direction-derived) after the filtering. This average elevation field is added to the original DEM to create the final product.
Throughout the discussion of the method, the technique was illustrated using data from AVHRR images of the northeast Greenland ice stream (Reference Fahnestock, Bindschadler, Kwok and JezekFahnestock and others, 1993). Figure 5 shows a comparison of the results of photoclinometric enhancement of the DEM with the original data. With the additional detail added from imagery in Figure 5b, the path of the ice stream is clearly seen. The surface of the ice stream is shown to be composed of undulations of a few tens of meters relief and a few kilometers across, with margins marked by an intermittent trough on either side. The troughs are approximately 5—8 m lower than the surrounding surfaces, and 5 km across. The markedly greater local relief on the ire stream is clearly shown. These features illustrate the type of information added to the enhanced DEM, and demonstrate the greater value of the enhanced data for ice-dynamics studies.
Validation
The study area covers the track of an airborne laser altimetry profile collected as part of the Arctic Ice Mapping (AIM) program in 1995. The flight path of the profile is shown in Figure 5b. Λ comparison of part of the profile with data from the original DEM and the enhanced DEM is shown in Figure 6. The laser altimetric data were gathered from a P-3 Orion aircraft flying approximately 400 m above the snow surface. Vertical accuracy of the laser profile is about 0.2 m, and the horizontal geolocation accuracy is better than 50 m. Some shilling of the image data was required to get the best match to the laser profile, which is not surprising given the ~2 km geolocation accuracy of AVHRR imagery when not using ground control. Although the two images were co-registered to within 500 m of each other, the absolute geolocation of the images was not refined to this level. The best match to the laser profile was found by shilling the enhanced DEM grid 1.4 km from its calculated coordinates.

Fig. 4. (a) x-direction raw elevations, (b) y-direction raw elevations, (c) filtered elevations, x direction, and (d) filtered elevations, y direction.

Fig. 5. (a) Three-dimensional view of the, KMS DEM in the study area. View direction is to the southwest, (b) Three-dimensional view of same area with enhanced topographic detail from photoclinometry. The black line indicates the location of an airborne laser elevation profile. The spike in the line is due to a spurious elevation in the laser profile from thin clouds.
The general correlation between the undulations mapped by photoclinometry and those shown in the laser profile validates the photoclinometric technique. However, the laser altimetry profile indicates a surface relief somewhat greater than that measured by the photoclinometry. This difference is probably due to the inherent smoothing of the image data by die pixel sampling and processing. The pixel size in the two scenes ranges between 1.1 and 1.4 km, and the georegistration step resampled the images to a 500 m grid using a cubic-spline weighting scheme, further smearing the original sampling of the surface. The function used to sample the gridded elevations of the enhanced DEM along the flight path uses a bilinear interpolation scheme. Thus, elevations in the photoclinometric profile in Figure 6 represent a mean elevation of a swath perhaps 2-3 km wide. The laser altimeter, in contrast, samples at a spatial rate of once every few meters across a swath of about 125 m.

Fig. 6. Comparison of elevations from the original DEM and the photoclinometrically enhanced DEM with the laser altimeter profile over part of its track.
The absence of features in the laser altimeter profile that are completely undetected by the photoclinometry suggests that the surface has few surface structures with wavelengths of 125-1000 m; therefore, AVHRR has sufficient resolution to detect most of the significant ice-sheet undulations of scale greater than the sastrugi. This result is to be expected from ice-dynamical considerations: surface features formed by interaction with the bed have length scales greater than about one or two times the ice thickness, with the exception of crevasses (e.g. Reference PatersonPaterson, 1994). Wind-generated features in some areas may be in the 100-1000 m range (e.g. snow megadunes, katabatic streamline dunes; sec Reference SwithinbankSwithinbank, 1988; Reference Bromwich, Parish and ZormanBromwich and others, 1990), but are not present in the test area.
Discussion
The photoclinometric technique
The demonstrated technique relies on a uniform albedo over a smooth, shadow-free surface. However, ice surfaces are not always smooth or uniform. Several features may complicate the process: clouds, nunataks, dust-covered snow, hoar-frost, wet snow, and blue ice are examples. In general, these problems may be avoided by careful selection of the images (i.e. selecting cloud-free scenes) or by masking areas that are not dry snow. For hoar patches, the best mitigation may be to select early-spring images. Recent studies of special sensor microwave/imager data in central Greenland suggest that hoar events occur in summer and early fall, when warmer, more humid air comes in contact with the cold ice plateau (Reference ShumanShuman and others, 1997). The images used for this study were acquired on 18 May, and no evidence of hoar-related albedo variations across the scenes was noted in these images, or in other spring images of this area. However, a similar search among springtime images of the Siple Coast area of West Antarctica shows that patches occur at least as early in the season as 1 November. It is possible that the lower altitude and frequent marine weather systems penetrating the ice shelf allow hoar formation earlier in the season here.
Using a DEM to calibrate the AVHRR photometric function allows the presented technique to be applied over any ice cap having an accurate DEM. However, the technique depends on the accuracy of the DEM, both for establishing the photometric function and as a base elevation field for photoclinometric enhancement. The fundamental requirement for the DEM is that it be a valid representation of the spatially averaged surface height at some spatial scale. In areas with only widely separated point-elevation data, such as the interior of the Antarctic ice sheet, the derived DEMs would in general not be accurate enough to derive an accurate photometric function. Further, as the spatial scale of “validity” increases (i.e. as the scale over which the true surface must be averaged to match the DEM increases), the residual random-walk error of the elevation determination from the images becomes greater. The several recent DEMs that include satellite radar altimetry, covering Greenland, some of the smaller ice caps in the Northern Hemisphere, and Antarctica north of the ERS-1 and -2 satellite nadir tracks (~81.5° S), are probably accurate enough for our method. Also, grids of airborne laser altimetric data at 5 or 10 km flight spacing, such as are flown as part of the AIM or SOAR (Support Office for Airborne Reconnaissance) surveys, would also yield a suitable DEM for enhancement.
Photoclinometery from satellite images translates radio-metric resolution into slope resolution, and therefore processing techniques that improve radiometry improve the potential accuracy of the elevation determination. Previous studies of Landsat image processing have cited principal components processing as one means of enhancing surface detail by enhancing the radiometric sensitivity (Orheim and Lucchitta, 1987; Reference Scambos, Dutkiewicz, Wilson and BindschadlerScambos and others, 1992). However, this approach, or any other method of combining several channels, will not work well if it includes a channel covering the 0.9 μm snow-absorption feature, since this has a strong correlation with grain-size. Another processing technique currently under development is data cumulation. This process uses several images of the same area and combines them at sub-pixel scale to improve both spatial and radio-metric resolution (Reference Albertz and ZelianeosAlbertz and Zelianeos, 1990; Reference Kvaran, Scambos and FahnestockKvaran and others, 1996). Data cumulation improves the spatial resolution to roughly one-half the original pixel size (more importantly, it eliminates aliasing of sub-pixel-scale features) and the radiometric resolution is improved by the square root of the number of scenes combined (typically six to 15 scenes are combined, so the factor of radiometric improvement is about 2-4).
The near future will see the launching of several satellites with both greater radiometric and greater spatial resolution than AVHRR or the other current imaging sensors such as SPOT or Landsat. In particular, the launching of the moderate-resolution imaging spectrometer (MODIS) on the AM-1 satellite of the NASA Earth Observing System program, planned for late 1998, will provide a sensor with 250 m resolution, 12-bit quantization of the visible-band signal, and a signal-to-noise ratio substantially greater than AVHRR.
The northeast Greenland ice stream
The photoclinometrically enhanced DEM of the ice stream shows several features pertinent to understanding the mechanics of its flow, and quantifies the vertical scale of its surface morphology. The ice stream is shown to have a surface relief of ~10 times that of the surrounding terrain (~50 m vs 3-5 m), very similar to the ice streams of the Siple Coast (see airborne laser surface-elevation profiles in Reference Bindschadler, Vornberger, Blankenship, Scambos and JacobelBindschadler and others, 1996). Assuming that ice thickness is more or less uniform across the study area, the best explanation for the greater relief is dynamic topography due to the greater ice-flow speed within the stream. Reference Kwok and FahnestockKwok and Fahnestock (1996) measured the speed of a small central part of the northeast Greenland ice stream at > 100 m a−1; they also estimated the local surface relief at “< 100 m”, consistent with our mapping. They futher noted that the ratio of ice speed within the stream to ice in the surrounding ice sheet was about 5, and shear strain was concentrated in the marginal zone. In the few places the margins were observed from the air, no noticeable surface crevassing was seen; however, SAR images show dark (low-backseatter) bands coincident with the margin troughs (Reference Fahnestock, Bindschadler, Kwok and JezekFahnestock and others, 1993), probably indicating the presence of buried crevasses. At lower elevations along the ice stream, more concentrated crevassing has been seen both visually and with radar. Such a large velocity contrast implies a rapid variation of basal conditions, i.e. from frozen to thawed. The strong contrast in local relief between ice outside and ice within the ice stream may illustrate the effects of basal sliding (as is observed in the West Antarctic ice streams).
The troughs coincident with the shear margins of the ice stream may also be due to a contrast in bed conditions, or may indicate localized melting in the shear zone near the bed. With a strong reduction in basal shear going from the ice sheet to the ice stream, the marginal ice may be thinned as the ice speed increases due to lower resistive forces (see e.g. Reference Thomas, Stephenson, Bindschadler, Shabtaie and BentleyThomas and others, 1988). However, such a model would create a topographic step across the margin, rather than a trough. Recent results from radar traverses over the former margins of Ice Stream C, West Antarctica, indicate that melting probably occurs beneath ice-stream shear margins, an inescapable result of the driving stress being dissipated there (personal communication from C. F. Raymond and others, 1997). At the northern margin of Ice Stream C, basal melting has removed at least 100 m of ice over 2000 years. If a similar process is going on actively al the margins of the northeast Greenland ice stream, it could explain the margin trough features we observe.
Summary
The photoclinometric method shown here recovers much of the ice-surface topography missed by satellite radar altimetry. Futher, the combination of AVHRR imagery and satellite radar altimetry appears to be capable of recovering most of the surface present at scales larger than crevasses and sastrugi, i.e. most of the topography pertinent to ice-dynamics modelling. In this instance, the technique provides an enhanced DEM with a spatial resolution scale of 2-3 km and an accuracy of a few meters.
Acknowledgements
Support for this study was provided by U.S. National Science Foundation grants OPP 9317007 and OPP 9418723. The image data for this study come from the U.S. National Snow and Ice Data Center's (NSIDC) polar 1 km AVHRR dataset, funded by NASA grant NAS5-32392. S. Ekholm of KMS generously provided the base DEM of the area.