Background
The worldwide older adult population continues to grow steadily each year, and this growth is accompanied by increasing prevalence rates of various chronic conditions (Tkatch et al., Reference Tkatch, Musich, MacLeod, Alsgaard, Hawkins and Yeh2016) and Alzheimer’s disease (AD) and related dementias (ADRD) (Barnes & Yaffe, Reference Barnes and Yaffe2011). There are approximately 50 million individuals currently diagnosed with dementia of various etiologies worldwide, and it is estimated that 139 million will be diagnosed by 2050 due to longer life expectancy and demographic changes (World Health Organization, 2022).
A comprehensive understanding of early risk markers in cognitively normal individuals is critical to accurately detect those at risk for ADRD and implement early interventions prior to symptom manifestation. A plethora of research has found that subjective cognitive decline (SCD), defined as the self-experienced perception of decline in one or more cognitive domains compared to a previous state, may be an early risk marker of AD (Jessen, Reference Jessen2014; Molinuevo et al., Reference Molinuevo, Rabin, Amariglio, Buckley, Dubois, Ellis, M., Hampel and Rami2017), and is related to brain abnormalities consistent with AD pathology (Amariglio et al., Reference Amariglio, Becker, Carmasin, Wadsworth, Lorius, Sullivan, Maye, Gidicsin, Pepin, Johnson and Rentz2012; Jessen et al., Reference Jessen, Amariglio, Van Boxtel, Breteler, Ceccaldi, Chételat, Dubois, Ellis and Van Der Flier2014; Jia et al., Reference Jia, Li, Li and Cao2021; Rabin et al., Reference Rabin, Smart and Amariglio2017; Schwarz et al., Reference Schwarz, Lange, Benson, Horn, Wurdack, Lukas, Buchert and Flöel2021; Zhao et al., Reference Zhao, Luo, Zhao, Mok, Su, Yin, Sun, Lu, Shi and Han2019). Since many individuals may report SCD for reasons other than preclinical AD (e.g., depression and other mood disturbances, poor physical health) (Molinuevo et al., Reference Molinuevo, Rabin, Amariglio, Buckley, Dubois, Ellis, M., Hampel and Rami2017; Rabin et al., Reference Rabin, Smart and Amariglio2017), a deeper understanding of the individual characteristics that strengthen the ability of SCD severity to predict cognition and AD biomarkers is needed.
Several studies have demonstrated that higher SCD reporting is associated with lower objective cognition in various community-based older adult samples (Blom et al., Reference Blom, Koek, Zwartbol, van der Graaf, Kesseler, Biessels, Geerlings, van der Graaf, Grobbee, Rutten, de Borst, Kappelle, Leiner and Doevendans2019; Burmester et al., Reference Burmester, Leathem and Merrick2016; Corlier et al., Reference Corlier, Shaw, Hayes-Larson, Mungas, Farias, Glymour and Mayeda2020; Jessen, Reference Jessen2014; Kielb et al., Reference Kielb, Rogalski, Weintraub and Rademaker2017; Nakhla et al., Reference Nakhla, Cohen, Salmon, Smirnov, Marquine, Moore and Zlatar2021; Rabin et al., Reference Rabin, Wang, Mogle, Lipton, Derby and Katz2020; Studart Neto & Nitrini, Reference Studart Neto and Nitrini2016; Zlatar et al., Reference Zlatar, Tarraf, González, Vásquez, Marquine, Lipton, Khambaty, Zeng and Youngblood2022) and increases the risk of progressing to dementia (Mazzeo et al., Reference Mazzeo, Padiglioni, Bagnoli, Carraro, Piaceri, Bracco, Sorbi and Bessi2020; Mendonça et al., Reference Mendonça, Alves and Bugalho2016; Mitchell et al., Reference Mitchell, Beaumont, Ferguson, Yadegarfar and Stubbs2014). However, other studies have shown that SCD may be related more closely to depression than objective cognition (Molinuevo et al., Reference Molinuevo, Rabin, Amariglio, Buckley, Dubois, Ellis, M., Hampel and Rami2017; Slavin et al., Reference Slavin, Brodaty, Kochan, Crawford, Trollor, Draper and Sachdev2010; Zlatar et al., Reference Zlatar, Moore, Palmer, Thompson and Jeste2014). Given conflicting findings, it is important to study if the severity of SCD reporting is sensitive to cognitive abilities and markers of brain health in non-clinic-based community samples, while accounting for depressive symptoms (Jessen et al., Reference Jessen, Amariglio, Buckley, van der Flier, Han, Molinuevo, Rabin, Rodriguez-Gomez and Saykin2020, Reference Jessen, Amariglio, Van Boxtel, Breteler, Ceccaldi, Chételat, Dubois, Ellis and Van Der Flier2014; Rabin et al., Reference Rabin, Wang, Mogle, Lipton, Derby and Katz2020).
AD pathology is linked to preclinical changes in cerebrovascular function and cardiovascular disease (CVD) risk factors such as hypertension, hyperlipidemia, diabetes, and metabolic syndrome, which increase the risk of developing dementia (Knopman & Roberts, Reference Knopman and Roberts2010). The two-hit vascular hypothesis of AD (Zlokovic, Reference Zlokovic2011) posits that damage to the brain’s microcirculation (hit one) initiates a cascade of vascular-related neuronal dysfunction, mediated by changes in blood-brain barrier function and reductions in cerebral blood flow (CBF). These vascular changes lead to a second hit, which arises from increased amyloid-β accumulation and impaired amyloid-β clearance mechanisms, which exert neurotoxic effects on the brain leading to degeneration and dementia. Given the involvement of cerebrovascular dysfunction in preclinical AD, and more specifically decreased CBF, it is important to study if SCD severity relates to CBF in cognitively normal individuals.
However, research studying the association of SCD with CBF is sparse. One study found that greater CBF did not support memory performance in those with SCD, whereas greater CBF was associated with better memory performance in those without SCD, suggesting neurovascular dysregulation in those with SCD (Hays et al., Reference Hays, Zlatar, Campbell, Meloy and Wierenga2018). Another community-based study also found higher CBF in the left parahippocampal gyrus of individuals with SCD compared to those without SCD (Wenyi Li et al., Reference Li, Jiang, Zou, Zhang, Sun, Jia, Li and Xu2022), suggestive of neurovascular dysregulation (Hays et al., Reference Hays, Zlatar and Wierenga2016; Østergaard et al., Reference Østergaard, Aamand, Gutiérrez-Jiménez, Ho, Blicher, Madsen, Dalby, Drasbek and Møller2013; Wierenga et al., Reference Wierenga, Hays and Zlatar2014). Moreover, a longitudinal study showed that baseline SCD (measured as a continuous variable in a community-based sample) was associated with reduced global CBF in older adults over a 3.8 year follow-up period (Kresge et al., Reference Kresge, Liu, Khan, Pamidimukkala, Cambronero, Moore, Bown, Pechman and Jefferson2020). Given the importance of CBF to maintaining brain health and supporting cognitive function, and its role as a potential early marker of cognitive decline (Hays et al., Reference Hays, Zlatar and Wierenga2016; Rabin et al., Reference Rabin, Smart and Amariglio2017; Wierenga et al., Reference Wierenga, Hays and Zlatar2014), research is needed to better understand if SCD is associated with CBF in older adults.
We examined if SCD severity (with SCD as a continuous variable rather than a diagnostic criterion) is associated with memory performance and with CBF in areas of the middle temporal lobe (MTL) that are implicated in preclinical AD. We hypothesized that greater SCD severity would be associated with worse memory performance and lower CBF in the MTL, adjusting for demographics and mood. To identify individual characteristics that may increase the likelihood that SCD reporting may be due to preclinical AD rather than other mood or health characteristics, we explored if those with greater CVD risk showed a stronger association of SCD severity with memory performance and CBF in the MTL.
Method
Participants
Participants were 52 community-dwelling research volunteers from the community with normal cognition and who were overall healthy, with mostly low CVD risk. They were recruited from ongoing aging studies at the UC San Diego’s (UCSD) Wellness Initiative for Senior Enrichment (WISE) Lab and Veteran Affairs San Diego Healthcare System (VASDHS). Participants were recruited from Research Match, registries, word of mouth, and community outreach. Study protocols were approved by UCSD or VASDHS Institutional Review Boards, and all participants provided written informed consent. Research was completed in accordance with the Helsinki Declaration.
Inclusion criteria for this sample consisted of individuals between the ages of 65 to 85 years, who are English-speaking as needed to complete cognitive testing, have no contraindications for magnetic resonance imaging, and ambulate independently. Exclusionary criteria included a pre-existing diagnosis of mild cognitive impairment (MCI) or dementia, history of vascular events (e.g., myocardial infarction, transient ischemic attacks, stroke), diabetes, chronic psychiatric conditions, major neurologic disorders, history of falls resulting in hospitalization in the past two years, and poorly controlled chronic conditions. Cognitive impairment for exclusion was based on comprehensive neuropsychological testing and followed the recommendation outlined by Jak and colleagues, which was defined by performance greater than one standard deviation below the norm on at least two measures within the same cognitive domain (Jak et al., Reference Jak, Bondi, Delano-Wood, Wierenga, Corey-Bloom, Salmon and Delis2009).
SCD measurement
SCD severity was measured as a continuous variable with the Everyday Cognition Scale (ECog) (Farias et al., Reference Farias, Mungas, Reed, Cahn-Weiner, Jagust, Baynes and DeCarli2008), a questionnaire of perceived cognitive decline compared to ten years inquiring about the loss in cognitively mediated functional abilities (e.g., remembering recent events, communicating thoughts, planning a shopping trip, multitasking). The ECog has been validated in older adults with normal cognition, MCI, and dementia and has the following subscales: Global Function, Memory, Language/Semantic, Visuospatial, and Executive: Planning, Organization, Divided Attention (Farias et al., Reference Farias, Mungas, Reed, Cahn-Weiner, Jagust, Baynes and DeCarli2008). The questionnaire consists of 39 items each scored on a scale of 1–4 (1 = better or no change, 2 = questionable/occasionally worse; 3 = consistently a little worse; 4 = consistently much worse) (Farias et al., Reference Farias, Mungas, Reed, Cahn-Weiner, Jagust, Baynes and DeCarli2008). Total raw scores range from 39 to 156 points, with higher scores reflecting perception of more severe cognitive decline.
Memory assessment
All participants completed a comprehensive neuropsychological battery. A Memory Composite Score was calculated based on a priori tests of immediate and delayed verbal recall by averaging across z-scores derived from each tests’ raw score. The memory tests included in the composite were: Wechsler Memory Scale-Revised (WMS-R) Logical Memory (LM)-I immediate recall (Wechsler, Reference Wechsler1987); WMS-R LM-II delayed recall (Wechsler, Reference Wechsler1987); Rey Auditory Verbal Learning Test (RAVLT) – Trials 1-5 Total (Schmidt, Reference Schmidt1996) [OR] California Verbal Learning Test 2nd Edition (CVLT-II) – Trials 1–5 Total (Delis et al., Reference Delis, Kramer, Kaplan and Ober2000); RAVLT – Short Delay Free Recall (Schmidt, Reference Schmidt1996) [OR] CVLT-II – Short Delay Free Recall (Delis et al., Reference Delis, Kramer, Kaplan and Ober2000); RAVLT – Long Delay Free Recall (Schmidt, Reference Schmidt1996) [OR] CVLT-II – Long Delay Free Recall (Delis et al., Reference Delis, Kramer, Kaplan and Ober2000). Participants from the WISE Lab (n = 39) were administered the RAVLT (Schmidt, Reference Schmidt1996), and participants from the VASDHS Lab (n = 13) were administered the CVLT-II (Delis et al., Reference Delis, Kramer, Kaplan and Ober2000). Given that the CVLT-II and RAVLT differ in word list length, we standardized the raw scores based on the means and standard deviations of each test prior to creating the memory composite. Previous research has supported the comparability between the CVLT-II and RAVLT (Beier et al., Reference Beier, Hughes, Williams and Gromisch2019; Grimes et al., Reference Grimes, Zanjani and Zakzanis2017; Samudra et al., Reference Samudra, Jacobs, Aulino and Abou-Khalil2020; Stallings et al., Reference Stallings, Boake and Sherer1995), particularly for raw scores (Stallings et al., Reference Stallings, Boake and Sherer1995; Wiens et al., Reference Wiens, Tindall and Crossen1994).
Assessment of depression
Depressive symptomatology was assessed with the Geriatric Depression Scale (GDS) – a 30-item, self-report questionnaire, with higher scores reflecting worse depressive symptoms (Yesavage et al., Reference Yesavage, Brink, Rose, Lum, Huang, Adey and Leirer1982). Total scores of 0–4 suggest no depression, 5–8 suggest mild depression, 9-11 suggest moderate depression, and 12–15 suggest severe depression (Greenberg, Reference Greenberg2012). Since the GDS contains cognitive items (Montorio & Izal, Reference Montorio and Izal1996) that may increase the shared variance with the ECog, we created a modified GDS score by removing the following items from our total score: item 14 “Do you feel like you have more problems with memory than most?”; item 26: “Do you have trouble concentrating?”; item 29: “Is it easy for you to make decisions?”. The modified GDS score (m-GDS) was used as a covariate in all analyses.
CVD Risk assessment
The Framingham Stroke Risk Profile (FSRP) (D’Agostino et al., Reference D’Agostino, Wolf, Belanger and Kannel1994) is a scale that evaluates the probability [percentage] of stroke occurrence over a 10-year period in adults ages 55 and older. FSRP scores of less than 10% suggest low stroke risk, 10-19% suggest moderate stroke risk, and 20% or more suggest high stroke risk (Bosomworth, Reference Bosomworth2011; D’Agostino et al., Reference D’Agostino, Wolf, Belanger and Kannel1994). The FSRP is corrected for sex and accounts for several risk factors: age (in years), systolic blood pressure (mm Hg), history of CVD (e.g., myocardial infarction, coronary insufficiency, congestive heart failure), left ventricular hypertrophy, and/or atrial fibrillation, diabetes mellitus, cigarette smoking, and use of antihypertensive medications.
Brain imaging acquisition and processing
Magnetic resonance imaging (MRI) data was acquired on a GE Discovery MR 750 3T whole-body system with a body transmit coil and an 8-channel receive-only head coil at the UCSD’s Center for functional MRI. The structural brain sequence was a high-resolution T1-weighted Fast Spoiled Gradient Recall (3DFSPGR) scan: 172 1mm contiguous sagittal slices, field of view (FOV) = 25 cm, repetition time (TR) = 8 ms, echo time (TE) = 3.1 ms, flip angle = 12, inversion time = 600 ms, 256 × 192 matrix, Bandwidth = 31.25 kHz, frequency direction = S-I, NEX = 1, scan time = 8 min and 13 s. T1-weighted images were processed using FreeSurfer 6.0 software. The images underwent skull stripping, B1 bias field correction, gray matter-white matter segmentation, reconstruction of cortical surface models, and parcellation and labeling of cortical surface regions and subcortical structures (Dale et al., Reference Dale, Fischl and Sereno1999; Fischl et al., Reference Fischl, Salat, Busa, Albert, Dieterich, Haselgrove, Kouwe, Killiany and Klaveness2002).
CBF was assessed with a 2D Pseudo Continuous Arterial Spin Labeling (ASL) MRI (2DPCASL) sequence; TR = 4500 ms, TE = 3.2 ms, FOV = 24 cm, labeling duration = 1800 ms, postlabeling delay = 2000 ms, with a single shot spiral acquisition and a total scan time of 4:30 min plus a 40.5 s calibration scan. The calibration scan was acquired immediately after the ASL scan using a spiral readout with TR = 4.5 s and TE = 3.2 ms with 8 dummy radiofrequency (RF) pulses (amplitude set to zero) to generate a 36 s delay followed by a 90-degree RF pulse in the last repetition interval to generate proton density-weighted contrast. Two field map scans were collected for offline field map correction for signal bunching and dropouts in the frontal/medial temporal lobes.
CBF quantification was conducted using the Cerebral Blood Flow Biomedical Informatics Research Network (CBFBIRN) database and analysis pipeline (Shin et al., Reference Shin, Ozyurt and Liu2013). CBFBIRN quantifies CBF and adjusts for partial volume effects (Shin et al., Reference Shin, Ozyurt and Liu2013) by using a combination of custom MATLAB (MathWorks, Reference MathWorks1996) routines and various Analysis of Functional Neuroimages (AFNI) (Cox, Reference Cox1996) and FMRIB Software Library (FSL) (Smith et al., Reference Smith, Jenkinson, Woolrich, Beckmann, Behrens, Johansen-Berg, De Luca, Drobnjak and Flitney2004) functions (Zlatar et al., Reference Zlatar, Hays, Mestre, Campbell, Meloy, Bangen, Liu and Wierenga2019). We used MATLAB to form a mean ASL image from the average difference of the control and tag images. For CBF calibration, we used a proton density image for conversion of the ASL difference signal into physiological units (ml/100g/min). Slice timing delays were accounted for, making the postlabeling delay slice specific. Skull stripping of the high-resolution T1-weighted image was performed using AFNI’s 3dSkullStrip. We used FSL’s Automated Segmentation Tool (FAST) algorithm to define cerebrospinal fluid, gray matter, and white matter regions. To correct CBF for partial volume effects and ensure that CBF values were not influenced by known decreased perfusion in the white matter or increased volume of cerebrospinal fluid, we used a linear regression method (Asllani et al., Reference Asllani, Borogovac and Brown2008) with a 5 × 5 regression kernel to obtain corrected gray matter CBF measurements. For each participant’s partial volume corrected quantified CBF map (in units of ml/100 g tissue/min), voxels with negative intensities were replaced with zero.
We used FreeSurfer 6.0 software to create anatomical ROIs for the CBF data. For each participant, using AFNI’s @SUMA_AlignToExperiment program, the FreeSurfer formatted T1-weighted brain volume was registered to the ASL CBF-aligned T1-weighted anatomical image which was derived from CBFBIRN. The resulting co-registration matrix was used to align the FreeSurfer aparc + aseg segmentation volume to the ASL CBF-aligned T1-weighted image using AFNI’s 3dAllineate program. The CBF-aligned FreeSurfer volumes were visually inspected to ensure proper alignment and were then downsampled to the resolution of the CBF ASL image using AFNI’s 3dfractionize program. Mean CBF was extracted for each FreeSurfer ROI, for each participant, which were entered as outcome variables in our models. We focused on investigating average CBF in AD-related regions of the MTL (hippocampal, parahippocampal, and entorhinal cortex) to determine their association with ECog total scores.
ASL MRI studies of older adults show similar patterns of regional CBF compared to studies using fluorodeoxyglucose positron emission tomography (FDG-PET) and single photon emission computed tomography (SPECT) (Chen et al., Reference Chen, Beyea, D’Arcy, Zhang and Rockwood2011; Takahashi et al., Reference Takahashi, Ishii, Hosokawa, Hyodo, Kashiwagi, Matsuki and Murakami2014). ASL has advantages over PET and SPECT given its use of a noninvasive, endogenous tracer rather than an intravenously administered contrast agent and also allows quantification of cerebral perfusion (in milliliters per 100 g of tissue per minute). Notably, the use of ASL in older adult populations may give rise to unique challenges given that the signal to noise ratio may be lower due to reduced CBF and potential vascular changes with aging. Age-related partial volume effects due to tissue atrophy and prolonged transport time from the labeling position to the tissue (i.e., arterial transient time) due to factors such as internal carotid stenosis or vessel tortuosity may affect the ASL signal and lead to underestimation or overestimation of CBF (Kilroy et al., Reference Kilroy, Apostolova, Liu, Yan, Ringman and Wang2014). To minimize the effects of these potential issues, we followed the white paper recommendations for the implementation of ASL published by the International Society for Magnetic Resonance in Medicine and the European Consortium for ASL in Dementia including a PCASL labeling approach and a relatively long postlabeling delay (i.e., 2,000 ms in older adults rather than the standard 1,800 ms) (Alsop et al., Reference Alsop, Detre, Golay, Günther, Hendrikse, Hernandez-Garcia, Lu, MacIntosh and Smits2015). Future studies using angiograms to improve tagging efficiency and quantifying arterial transit time delays may improve quantification of CBF among older adults (Alsop et al., Reference Alsop, Detre, Golay, Günther, Hendrikse, Hernandez-Garcia, Lu, MacIntosh and Smits2015).
Statistical analyses
Analyses were conducted using IBM SPSS Version 28.0 (IBM, 2021). Data were screened for normality by inspecting skewness and kurtosis limits (Field, Reference Field2009), and no significant outliers were detected for the memory outcome variable. There was one outlier for the ECog total scores (>4 standard deviations above the mean), which was removed.
We removed one participant that had CBF values (i.e., entorhinal) outside of the expected physiological range (<10 or >150 ml/100g/min) (Bangen et al., Reference Bangen, Nation, Clark, Harmell, Wierenga, Dev, Delano-Wood, Zlatar, Liu and Bondi2014). There were also three statistical outliers in the dataset with mean regional CBF values within the range that our group has considered physiologically plausible (i.e., >10 and <150; Bangen et al., Reference Bangen, Nation, Clark, Harmell, Wierenga, Dev, Delano-Wood, Zlatar, Liu and Bondi2014, Reference Bangen, Werhane, Weigand, Edmonds, Delano-Wood, Thomas, Evangelista, Clark and Liu2018), but were excluded given statistical considerations (>3 standard deviations above the mean). This is not uncommon since several studies have shown that older adults at risk for cognitive decline demonstrate elevated CBF, which may represent a compensatory mechanism and/or neurovascular dysregulation (Bangen et al., Reference Bangen, Kaup, Mirzakhanian, Wierenga, Jeste and Eyler2012; Thomas et al., Reference Thomas, Weigand, Cota, Edmonds, Wierenga, Bondi and Bangen2022). Altogether, a total of 5 outliers (1 Ecog + 4 CBF) were removed, which resulted in a final sample of 52 participants for all analyses.
Pearson correlations between the predictor variables were within acceptable limits (all rs < .6). For all regression models, tolerance levels were >.1 and variance inflation factors <.2, indicating no evidence of multicollinearity (Field, Reference Field2009). Covariates of interest were theoretically selected based on the SCD literature and following best practices (Jessen et al., Reference Jessen, Amariglio, Van Boxtel, Breteler, Ceccaldi, Chételat, Dubois, Ellis and Van Der Flier2014; Molinuevo et al., Reference Molinuevo, Rabin, Amariglio, Buckley, Dubois, Ellis, M., Hampel and Rami2017). As such, we included age, sex, years of education, and m-GDS scores in all regression models.
Relationship between ECog, objective memory, and CBF in the total sample (n = 52)
Linear regression models were conducted to determine the association of continuous ECog scores with (1) memory composite scores, (2) CBF in the hippocampus, (3) CBF in the parahippocampal gyrus, and (4) CBF in the entorhinal cortex. All models were adjusted for age, sex, years of education, and m-GDS scores.
Exploratory analysis: ECog, objective memory, and CBF stratified by CVD risk
Linear regression models stratified by FSRP scores were conducted to determine if the association of ECog with memory and CBF was higher in those with greater FSRP scores. We dichotomized the continuous FSRP% Stroke Risk variable into “lower FSRP scores” (n = 21) and “higher FSRP scores” (n = 31) groups based on a median split (median value = 6%). Only 14 participants in the sample had FSRP scores ≥10, suggestive of medium to high stroke risk (Bosomworth, Reference Bosomworth2011; D’Agostino et al., Reference D’Agostino, Wolf, Belanger and Kannel1994), which was too small to dichotomize groups based on this criteria. Since we are studying a healthy sample of older adults, and our strict inclusion criteria excluded individuals with very high CVD risk, results are interpreted in the context of those with overall low CVD risk. T-tests and chi-square analyses explored differences in demographics and mood, FSRP scores, blood pressure, CBF, and memory performance between lower and higher FSRP score groups. We adjusted for demographic characteristics (age, sex, education) and mood (m-GDS scores).
Results
Participants’ characteristics are displayed in Table 1. They were, on average, 72.90 years old (range = 65–83 years), mostly female (69%), relatively well-educated (mean = 16.98 years, SD = 2.28 years), predominantly Caucasian/White (87%), and with overall low CVD risk status as indicated by FSRP scores (mean = 8.12, SD = 5.34).
Table 1. Descriptive and demographic information (n = 52)
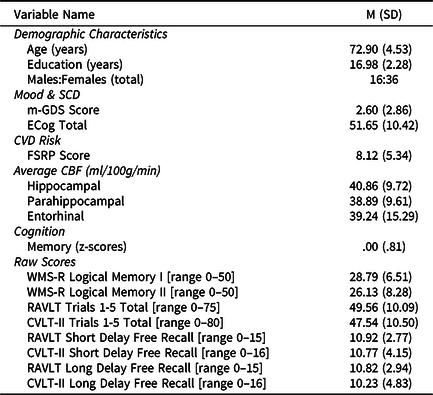
Note. Abbreviations: SCD = subjective cognitive decline; ECog = Everyday Cognition Scale; m-GDS = modified Geriatric Depression Scale; CVD = cardiovascular (risk); CBF = cerebral blood flow; FSRP = Framingham Stroke Risk Profile; WMS-R = Wechsler Memory Scale - Revised; RAVLT = Rey Auditory Verbal Learning Test; CVLT-II = California Verbal Learning Test- 2nd Edition.
All values listed are means (standard deviations) unless otherwise indicated.
Relationship between ECog, objective memory, and CBF in the total sample (n = 52)
Fully adjusted linear regression models (Table 2 & Figures 1 and 2) revealed that higher ECog total scores were significantly correlated with lower memory performance after adjusting for age, sex, education, and m-GDS. Similarly, higher ECog total scores were associated with lower CBF in the entorhinal cortex after adjusting for age, sex, education, and m-GDS. Results did not change when using the total GDS scores instead of the m-GDS scores as a covariate.
Table 2. ECog scores predicting memory and CBF in the total sample (n = 52)

Note. *p < .05, **p < .01, ***p < .001. All models were adjusted for age, sex, years of education, and modified GDS scores. B (SE) denotes unstandardized coefficient and corresponding standard error. β denotes the standardized coefficient.
Abbreviations: ECog = Everyday Cognition Scale; CBF = cerebral blood flow.
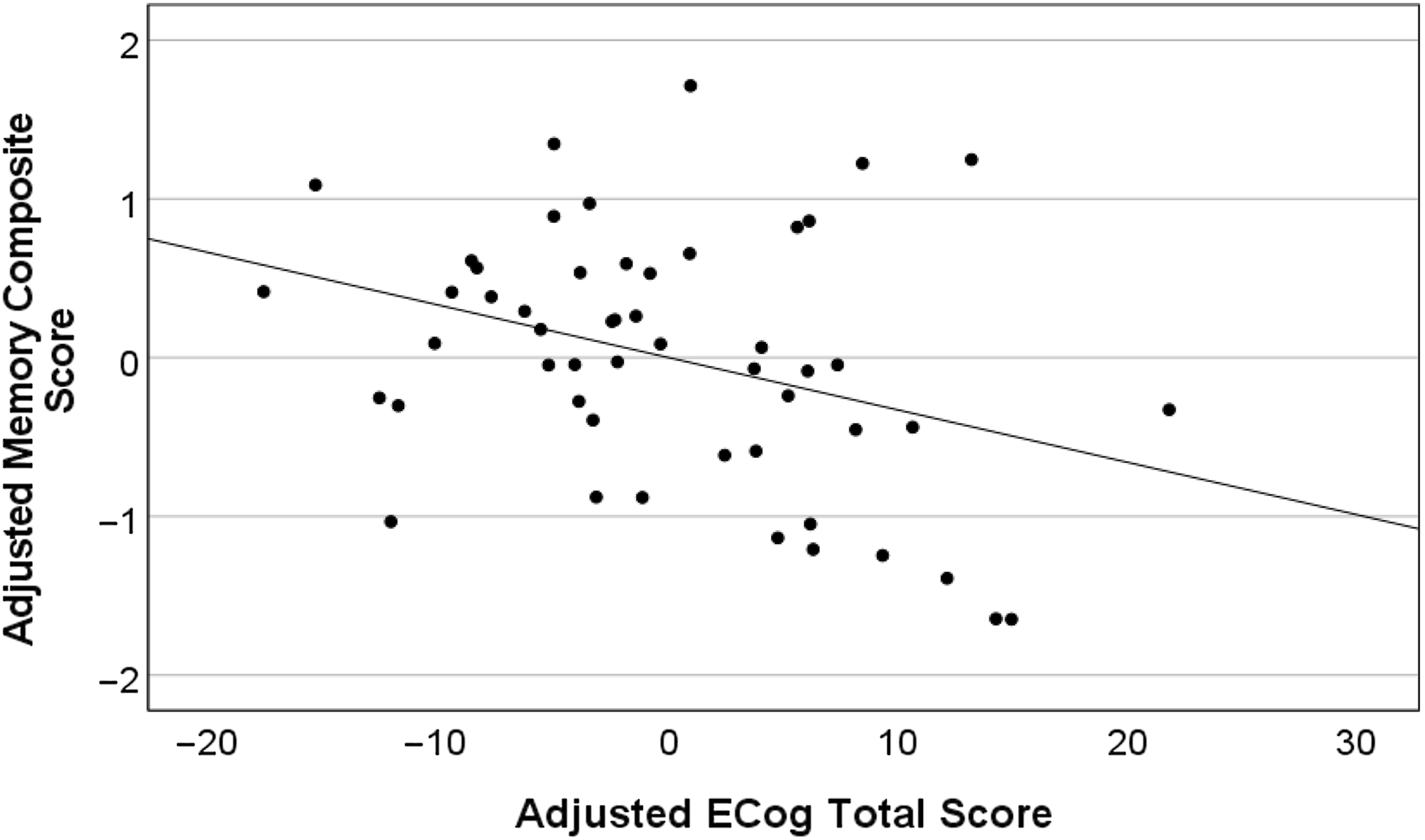
Figure 1. Higher ECog total scores predict lower memory performance in the total sample (n = 52). Partial regression plot depicts residual values adjusted for age, years of education, sex, and modified GDS scores.
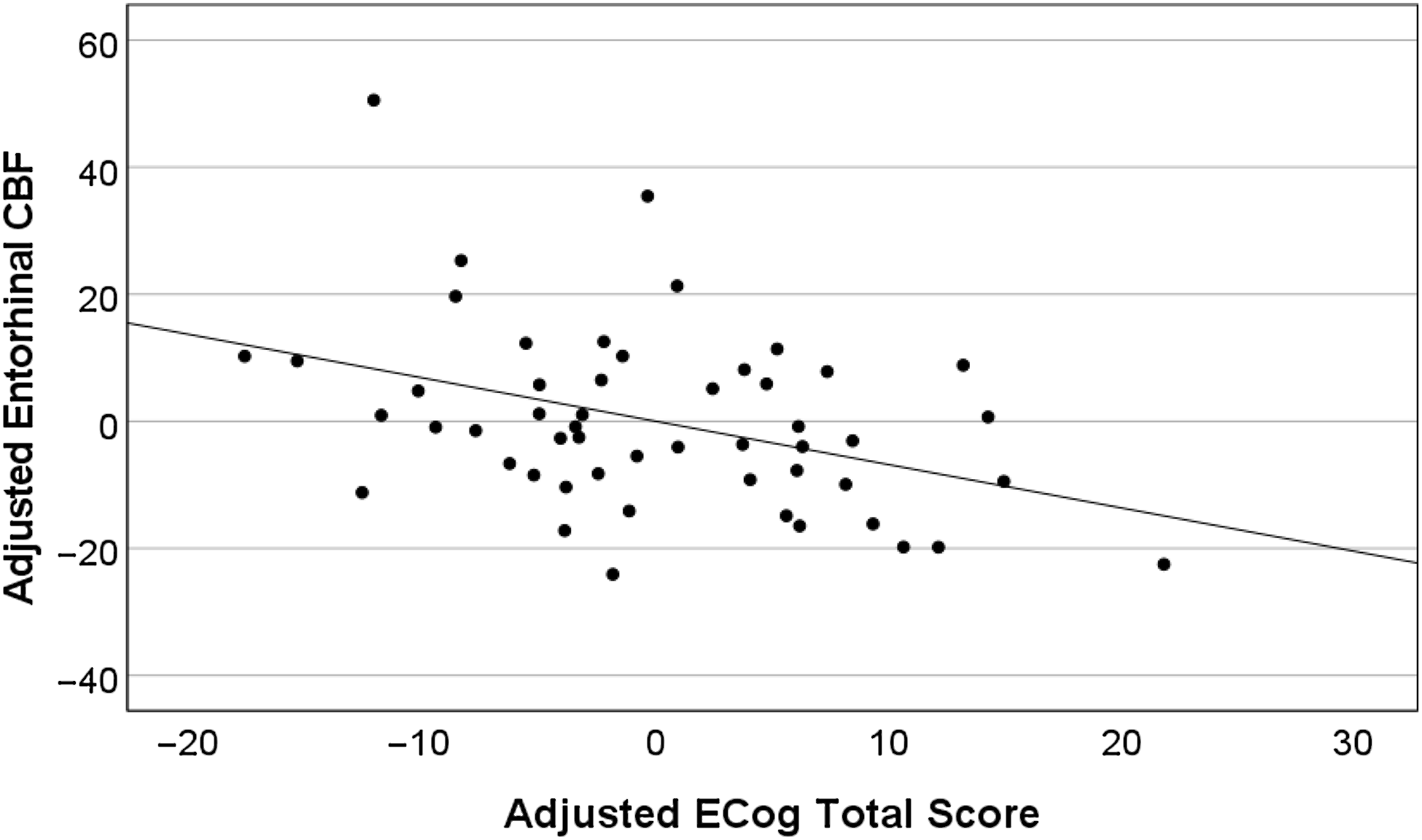
Figure 2. Higher ECog total scores predict lower entorhinal CBF in the total sample (n = 52). Partial regression plot depicts residual values adjusted for age, years of education, sex, and modified GDS scores.
Exploratory analysis: ECog, objective memory, and CBF stratified by CVD risk
Lower and higher FSRP score groups did not significantly differ in years of education, m-GDS scores, memory performance, ECog total scores, or CBF (hippocampal, parahippocampal, entorhinal). The sex distribution and age were statistically different between the lower and higher FSRP score groups. The higher FSRP score group had significantly greater FSRP% stroke risk scores and systolic blood pressure (Table 3). There was a significant association of greater ECog scores with lower memory performance (Figure 3A) and entorhinal CBF (Figure 3B) only for those in the higher FSRP score group. Memory performance and entorhinal CBF were not associated with ECog scores in the lower FSRP score group (Table 4 ). Results did not change when using the total GDS scores instead of the m-GDS scores as a covariate.
Table 3. Demographic characteristics of the lower (n = 21) and higher (n = 31) FSRP score groups
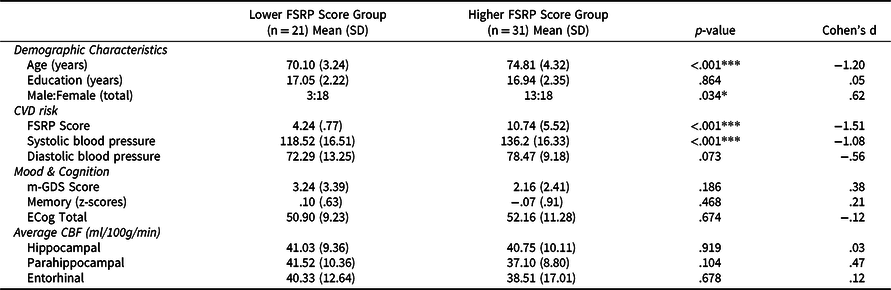
Note. *p < .05, **p < .01, ***p < .001. P-values were derived from independent samples t-tests and χ2 tests. All comparisons based on df = 50. Abbreviations: CVD = cardiovascular (risk); m-GDS = modified Geriatric Depression Scale; FSRP = Framingham Stroke Risk Profile; CBF = cerebral blood flow; ECog = Everyday Cognition Scale. All values listed are means (standard deviations) unless otherwise indicated.
Table 4. Regression models of ECog predicting memory and CBF stratified by CVD risk group

Note. *p < .05, **p < .01, ***p < .001. Stratified regression models were fully adjusted for age, sex education, and modified GDS scores. B (SE) denotes unstandardized coefficient and corresponding standard error. β denotes the standardized coefficient. Abbreviations: FSRP = Framingham Stroke Risk Profile; CBF = cerebral blood flow.
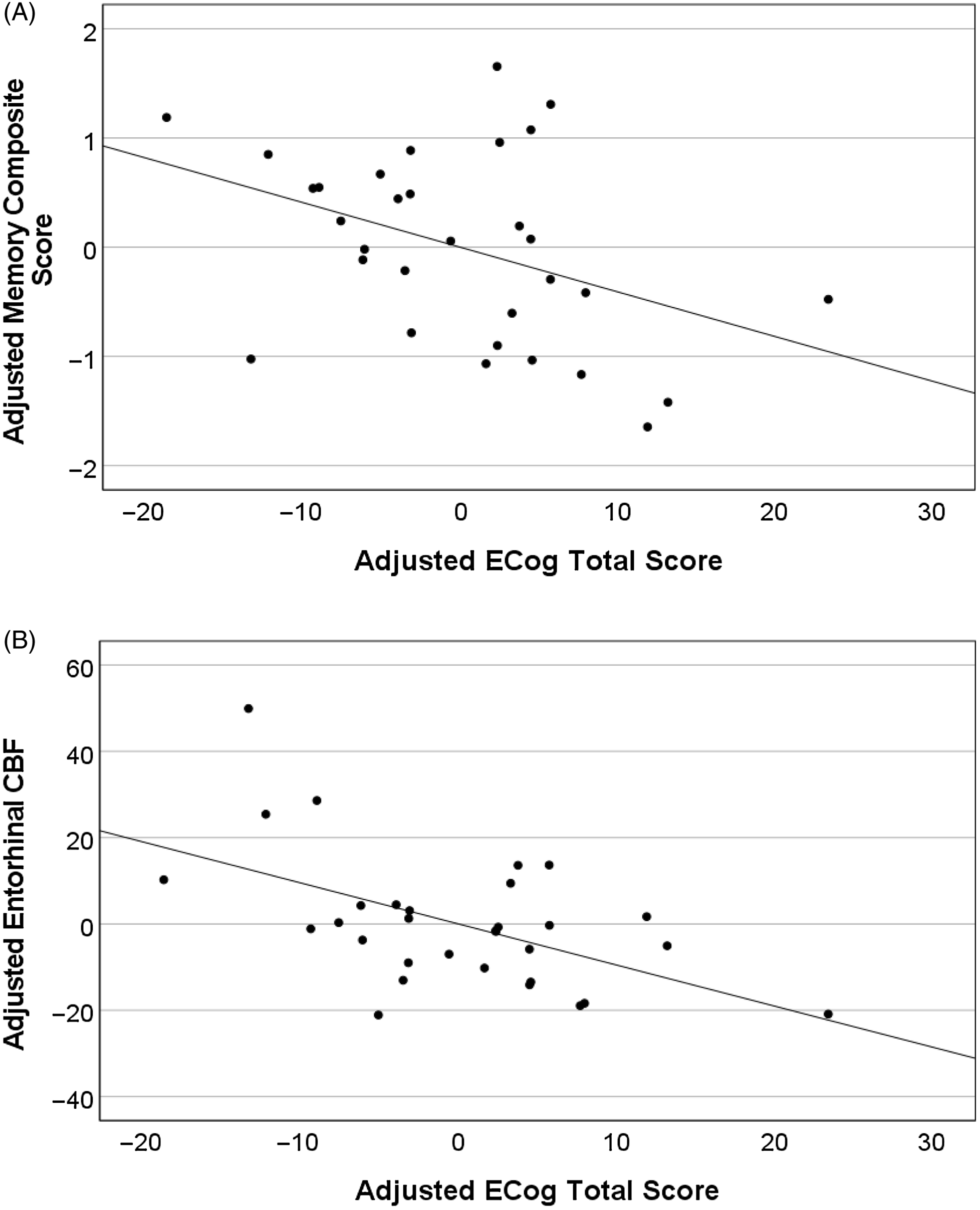
Figure 3. Higher ECog total scores predict lower memory and entorhinal CBF in the higher FSRP score group (n = 31). Partial regression plots depict residual values adjusted for age, years of education, sex, and modified GDS scores.
Discussion
This study investigated the association of SCD severity with objective memory performance and CBF in areas implicated in preclinical AD in older cognitively normal research volunteers. We also explored if those with greater CVD risk severity showed a stronger correlation of ECog scores with memory performance and CBF. We found that greater SCD severity, measured with the ECog, was associated with lower memory performance in our sample of cognitively normal older adults, after controlling for demographic characteristics and mood. This is consistent with other studies (Brailean et al., Reference Brailean, Steptoe, Batty, Zaninotto and Llewellyn2019; Corlier et al., Reference Corlier, Shaw, Hayes-Larson, Mungas, Farias, Glymour and Mayeda2020; Farias et al., Reference Farias, Park, Harvey, Simon, Reed, Carmichael and Mungas2013; Kielb et al., Reference Kielb, Rogalski, Weintraub and Rademaker2017; Li, Yue, et al., Reference Li, Yue and Xiao2022), and highlights that SCD severity in community-based samples may be sensitive to concurrent memory difficulties, even in individuals who are cognitively normal, non-medical help-seeking, and have overall low CVD risk profiles.
We also found an association between greater SCD severity and lower entorhinal CBF across the total sample, even after adjusting for demographics and mood. The entorhinal cortex is thought to be one of the first regions to be affected in AD and a strong predictor of disease progression from MCI to AD (Khan et al., Reference Khan, Liu, Provenzano, Berman, Profaci, Sloan, Mayeux, Duff and Small2014; Zhou et al., Reference Zhou, Zhang, Zhao, Qian and Dong2016). In fact, reduced CBF in the MTL has been predictive of faster rates of cognitive decline, neurodegeneration and white matter hyperintensity progression (Bangen et al., Reference Bangen, Thomas, Sanchez, Edmonds, Weigand, Delano-Wood and Initiative2021), and decline in daily functioning (i.e., instrumental activities of daily living) (Sanchez et al., Reference Sanchez, Thomas, Edmonds, Bondi, Bangen and Initiative2020). Dysfunction in the entorhinal cortex is thought to precede dysfunction in the hippocampal circuit (Khan et al., Reference Khan, Liu, Provenzano, Berman, Profaci, Sloan, Mayeux, Duff and Small2014), a brain region that is associated with hallmark memory deficits observed in AD (Babcock et al., Reference Babcock, Page, Fallon and Webb2021; Chen et al., Reference Chen, Farrell, Rundle, Chan, Moore, Wig and Park2021; Setti et al., Reference Setti, Hunsberger and Reed2017). Reductions in CBF have been associated with increased Aβ production and are thought to be an early risk marker of AD (Hays et al., Reference Hays, Zlatar and Wierenga2016; Korte et al., Reference Korte, Nortley and Attwell2020; Park et al., Reference Park, Hong, Lee, Ji, Jung, Yoon, Won, Song and Kim2019; Wierenga et al., Reference Wierenga, Hays and Zlatar2014; Zlokovic, Reference Zlokovic2011). As such, our findings provide evidence that SCD severity is not only associated with objective memory, but also with one of the earliest brain signatures of AD in a cognitively normal, community-based sample. These findings are consistent with recent literature showing that CBF alterations in the MTL are an independent risk factor for SCD (Li, Jiang et al., Reference Li, Jiang, Zou, Zhang, Sun, Jia, Li and Xu2022), and that, compared to healthy controls, those with SCD show lower CBF in areas of the MTL (Yang et al., Reference Yang, Rong, Cao, Wu, Zhao, Xie and Liu2021).
Consistent with our hypothesis, exploratory stratified analyses within FSRP score groups suggest that the relationship of SCD severity with memory performance and entorhinal CBF in our sample of cognitively normal older adults may be driven by those with higher risk for developing AD by virtue of their CVD burden. That is, the association of greater SCD severity with worse memory performance and lower entorhinal cortex CBF was only significant within the higher FSRP score group. As previously noted, SCD is associated with an increased risk for dementia (Jessen, Reference Jessen2014; Mendonça et al., Reference Mendonça, Alves and Bugalho2016; Mitchell et al., Reference Mitchell, Beaumont, Ferguson, Yadegarfar and Stubbs2014; Molinuevo et al., Reference Molinuevo, Rabin, Amariglio, Buckley, Dubois, Ellis, M., Hampel and Rami2017) for some individuals. Similarly, those with greater CVD burden also have an increased risk of developing AD (Song et al., Reference Song, Pan, Xu, Qi, Buchman, Bennett and Xu2021). Our findings suggest that SCD severity in individuals with a higher CVD burden may be more closely associated with cognition and brain markers of preclinical AD than in those with lower CVD burden. These exploratory results shed light on a potential variable that may help increase the clinical utility of SCD reporting in cognitively normal and overall healthy individuals, although larger studies with more diverse individuals (i.e., with greater CVD risk and from different ethnic and socioeconomic backgrounds) are needed to replicate these findings. It is important to keep in mind that not everyone who reports SCD will progress to AD since SCD reporting may reflect other conditions such as depression, anxiety, or other neurologic disorders (Jessen et al., Reference Jessen, Amariglio, Van Boxtel, Breteler, Ceccaldi, Chételat, Dubois, Ellis and Van Der Flier2014; Molinuevo et al., Reference Molinuevo, Rabin, Amariglio, Buckley, Dubois, Ellis, M., Hampel and Rami2017; Slavin et al., Reference Slavin, Brodaty, Kochan, Crawford, Trollor, Draper and Sachdev2010; Zlatar et al., Reference Zlatar, Moore, Palmer, Thompson and Jeste2014). As such, finding individual characteristics that increase the clinical utility of SCD to predict preclinical AD and later progression warrants further study. Alternatively, our findings could also indicate that cognitively normal individuals with a higher CVD burden may also be more aware of subtle changes in their memory performance and/or may be at greater risk for memory difficulties.
Limitations of this study include a cognitively normal older adult community-based sample that was mostly Caucasian/White, female, and highly educated, limiting the generalizability of our findings. Individuals in our sample generally reported minimal to no depressive symptoms and reported low SCD severity. Similarly, we had strict inclusion criteria, excluding individuals with a history of vascular events (e.g., myocardial infarction, transient ischemic attacks, stroke) and diabetes, which are components of the FSRP score we used to characterize CVD risk. This resulted in an overall healthy sample with low CVD risk burden. Thus, our results cannot be generalized to individuals with high CVD burden, a diagnosis of SCD, and/or clinical samples seeking medical help for cognitive decline. Since CVD risk and other health conditions may influence SCD reporting (Gu et al., Reference Gu, Fischer, Saposnik and Schweizer2013; Molinuevo et al., Reference Molinuevo, Rabin, Amariglio, Buckley, Dubois, Ellis, M., Hampel and Rami2017; Rabin et al., Reference Rabin, Smart and Amariglio2017; Sajjad et al., Reference Sajjad, Mirza, Portegies, Bos, Hofman, Koudstaal and Ikram2015), future research with larger and more diverse samples, including individuals with high CVD risk burden is warranted. Moreover, our small sample size may have obscured potential associations of SCD with CBF in other MTL regions due to lack of power. Notably, this study was cross-sectional, and therefore, we cannot determine if SCD predicts changes in objective cognition or CBF over time. Future longitudinal studies would be beneficial in elucidating these important relationships. Lastly, inclusion of other measures of CVD risk (including relevant variables such as hyperlipidemia, hypercholesterolemia, body mass index, renal function) (Bosomworth, Reference Bosomworth2011) and other markers of brain health would be beneficial to increasing our understanding of how CVD risk may impact the relationship of SCD severity with memory and CBF.
In summary, findings provide evidence that SCD severity is associated with objectively measured cognition and CBF in regions implicated in preclinical AD, and further suggest that those with greater CVD risk may be driving this relationship. Findings are aligned with previous studies suggesting that the ECog can be used as a clinically valuable prescreening tool (Corlier et al., Reference Corlier, Shaw, Hayes-Larson, Mungas, Farias, Glymour and Mayeda2020; Farias et al., Reference Farias, Park, Harvey, Simon, Reed, Carmichael and Mungas2013; Rueda et al., Reference Rueda, Lau, Saito, Harvey, Risacher, Aisen, Saykin, Farias and Initiative2015; Shokouhi et al., Reference Shokouhi, Conley, Baker, Albert, Kang, Gwirtsman and Newhouse2019). In this case, the ECog seems particularly sensitive to memory performance and neural markers of preclinical AD in individuals with greater CVD risk. Future studies should investigate if individuals who present with greater CVD burden and SCD severity have a higher chance of progression to MCI and AD over time.
Acknowledgements
We acknowledge the University of California, San Diego’s Strategic Enhancement of Excellence through Diversity (SEED) Fellowship to Marina Zaher Nakhla. We thank all research participants for volunteering their time and effort to propel scientific knowledge. Finally, we thank the research assistants at the University of California, San Diego’s WISE Lab for their help with data collection and entry. Study data were collected and managed using REDCap electronic data capture tools hosted at the University of California, San Diego CTRI [UL1TR001442].
Funding
Contents are solely the responsibility of the authors and do not necessarily represent the official views of the NIH. Zvinka Z. Zlatar received support from the National Institutes on Aging (NIA) Grants K23AG049906 and R01AG066657. Katherine J. Bangen received support for this paper from VA Clinical Science R&D Merit Award 1I01CX001842, NIA R01 AG063782, and the Alzheimer’s Association AARG-18-566254.
Conflicts of interest
The authors report no conflicts of interest.