Introduction
Alzheimer’s disease (AD) is the sixth leading cause of death in the United States (U.S.), with more than 6 million people in the nation currently diagnosed with the disease (Alzheimer’s Association, 2022). As life expectancy increases, the prevalence of AD is also expected to increase in the coming years, with the number of people with AD in the U.S. projected to reach almost 13 million by 2050 (Alzheimer’s Association, 2022). In addition to the development of pharmacological and nonpharmacological interventions for AD, early and accurate identification of AD with biomarkers (e.g., amyloid and tau markers) and cognitive measures have become a key goal in the fight against the disease. Understanding how performance on cognitive tests is related to AD biomarkers is of significant value to clinicians given that cognitive tests are faster to administer, less invasive, more accessible, and cheaper than many current techniques used to assess biomarker changes in AD.
Prior research on AD suggests that semantic networks are disrupted early in the AD process (Chan et al., Reference Chan, Butters and Salmon1997; Pineault et al., Reference Pineault, Jolicoeur, Grimault, Bermudez, Brambati, Lacombe, Villalpando, Manuel, Marie-Jeanne and Joubert2018). Behaviorally, disruption of semantic networks in AD can manifest as inefficient or poor performance on cognitive tests of semantic fluency, such as tests of category fluency (Troyer et al., Reference Troyer, Moscovitch and Winocur1997). The category fluency (animals) test is widely used and studied in AD research, and performance on this measure is believed to be supported by semantic networks (Lezak et al., Reference Lezak, Howieson, Loring and Fischer2004; Weakley & Schmitter-Edgecombe, Reference Weakley and Schmitter-Edgecombe2014). While examining the total score on category fluency animals can help researchers assess semantic fluency and semantic access, some groups have examined the qualitative aspects of category fluency, which includes clustering (i.e., stating words sequentially that are all contained within a subcategory, such as domestic animals; Fagundo et al., Reference Fagundo, López, Romero, Guarch, Marcos and Salamero2008; Brucki & Rocha, Reference Brucki and Rocha2004; Troyer et al., Reference Troyer, Moscovitch and Winocur1997). Worse performance on both quantitative and qualitative aspects of category fluency have been seen in patients with mild dementia due to AD (Fagundo et al., Reference Fagundo, López, Romero, Guarch, Marcos and Salamero2008; Gomez & White, Reference Gomez and White2006); several studies, for example, have found that relative to healthy aged adults people with AD generated fewer category clusters and exhibited less switches between semantic subcategories (Haugrud et al., Reference Haugrud, Crossley and Vrbancic2011, Liampas et al., Reference Liampas, Folia, Zoupa, Siokas, Yannakoulia, Sakka, Hadjigeorgiou, Scarmeas, Dardiotis and Kosmidis2022, Tessaro et al., Reference Tessaro, Hermes-Pereira, Schilling, Fonseca, Kochhann and Hübner2020). The total score on animal fluency, however, may not be sensitive enough to differentiate people in the preclinical and earliest clinical stages of the disease from healthy adults (Haugrud et al., Reference Haugrud, Crossley and Vrbancic2011; Troyer et al., Reference Troyer, Moscovitch and Winocur1997, Reference Troyer, Moscovitch, Winocur, Leach and Freedman1998). Examining both qualitative and quantitative aspects of animal fluency together may improve the utility of this test in identifying people at increased risk for AD dementia, including people in the preclinical stage and earliest clinical stages of the disease.
Studying the preclinical period of AD, or the period where there are evident abnormalities in AD biomarkers without clinically significant objective cognitive impairment (Jack et al., Reference Jack, Bennett, Blennow, Carrillo, Dunn, Haeberlein, Holtzman, Jagust, Jessen, Karlawish, Liu, Molinuevo, Montine, Phelps, Rankin, Rowe, Scheltens, Siemers, Snyder, Sperling and Silverberg2018; Sperling et al., Reference Sperling, Aisen, Beckett, Bennett, Craft, Fagan, Iwatsubo, Jack, Kaye, Montine, Park, Reiman, Rowe, Siemers, Stern, Yaffe, Carrillo, Thies, Morrison-Bogorad and Phelps2011), remains a significant challenge. Autosomal dominant AD (ADAD) is a rare, genetically deterministic form of AD due to mutations in one of three genes – the Amyloid Precursor Protein [APP], Presenilin1, and Presenilin 2 (Fuller et al., Reference Fuller, Cronin-Golomb, Gatchel, Norton, Guzmán-Vélez, Jacobs, Hanseeuw, Pardilla-Delgado, Artola, Baena, Bocanegra, Kosik, Chen, Tariot, Johnson, Sperling, Reiman, Lopera and Quiroz2019; Ryman et al., Reference Ryman, Acosta-Baena, Aisen, Bird, Danek, Fox, Goate, Frommelt, Ghetti, Langbaum, Lopera, Martins, Masters, Mayeux, McDade, Moreno, Reiman, Ringman, Salloway and Bateman2014). To the degree to which ADAD and sporadic AD have similar clinical and biomarker similarities, studying ADAD carriers and noncarrier family members presents a unique opportunity to investigate the biological and cognitive markers of the disease in nondemented individuals destined to develop AD dementia in the future (Fuller et al., Reference Fuller, Cronin-Golomb, Gatchel, Norton, Guzmán-Vélez, Jacobs, Hanseeuw, Pardilla-Delgado, Artola, Baena, Bocanegra, Kosik, Chen, Tariot, Johnson, Sperling, Reiman, Lopera and Quiroz2019; Morris et al., Reference Morris, Weiner, Xiong, Beckett, Coble, Saito, Aisen, Allegri, Benzinger, Berman, Cairns, Carrillo, Chui, Chhatwal, Cruchaga, Fagan, Farlow, Fox, Ghetti and Buckles2022).
In this study, we leverage data from a homogenous ADAD kindred with the Presenilin1 E280A (PSEN1) mutation from Colombia, South America (see Fuller et al., Reference Fuller, Cronin-Golomb, Gatchel, Norton, Guzmán-Vélez, Jacobs, Hanseeuw, Pardilla-Delgado, Artola, Baena, Bocanegra, Kosik, Chen, Tariot, Johnson, Sperling, Reiman, Lopera and Quiroz2019 for details) to investigate if category fluency clustering is associated with markers of AD brain pathology (i.e., mean cortical amyloid and regional tau burden) in the preclinical and early stages of ADAD. This study is the first, to our knowledge, that examines category fluency clustering in people with ADAD mutations who are destined to develop AD clinical syndrome with virtually 100% certainty and noncarrier family members. We hypothesized we would find a significant correlation between category fluency scores (total score and clustering score) with age – which is a proxy for disease progression in ADAD (Fuller et al., Reference Fuller, Cronin-Golomb, Gatchel, Norton, Guzmán-Vélez, Jacobs, Hanseeuw, Pardilla-Delgado, Artola, Baena, Bocanegra, Kosik, Chen, Tariot, Johnson, Sperling, Reiman, Lopera and Quiroz2019) – and AD brain pathology, indicating a relationship between increased AD brain pathology and semantic disruption in the preclinical and earliest stages of the disease.
Methods
Ethics approval
A research ethics committee at the University of Antioquia (Colombia) and the Partners Human Research Committee (Boston) approved this study. Participants gave written informed consent before participating in the study. Study procedures were executed in compliance with the Helsinki Declaration standards for research with human subjects.
Participants and study design
Twenty-nine nondemented carriers of the Presenilin1 (PSEN1) E280A ADAD mutation (7 carriers with mild cognitive impairment) and 32 noncarrier family members from Colombia were recruited (Table 1). These participants traveled from Medellín, Colombia to Boston, MA to undergo positron emission tomography (PET) amyloid and tau imaging. Participants and the study staff were both blinded to individuals’ PSEN1 genetic status.
Table 1. Demographic, Cognitive, and PET Data of Sample (n = 61)
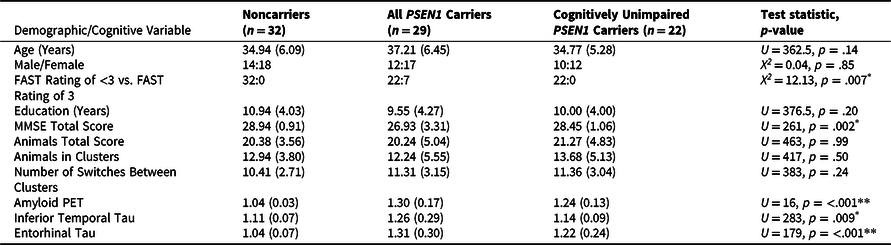
*p < .01, **p < .001.
Notes: Test statistics and p-values were generated for the comparison between noncarriers and all PSEN1 E280A carriers. The cognitively unimpaired carrier group is a subset of the full group of mutation carriers. Abbreviations: MMSE = Mini-Mental State Examination; FAST = Functional Assessment Staging Scale; PET = positron emission tomography; PSEN1 = presenilin1.
Procedures
Participants in this study live in the region of Antioquia, Colombia and completed clinical and cognitive testing at the University of Antioquia in Medellín. Spanish-speaking neuropsychologists (including author AB) administered all cognitive tests. The Functional Assessment Staging Scale (FAST, Sclan & Reisberg, Reference Sclan and Reisberg1992) was used to measure clinical impairment in participants, with values of 1 indicating “no cognitive impairment,” 2 indicating “subjective cognitive concerns,” 3 indicating “early mild cognitive impairment.” Participants also took a Spanish version of the Mini-Mental State Examination (MMSE, Folstein et al., Reference Folstein, Folstein and McHugh1975) to measure global cognitive functioning. Cognitively unimpaired individuals had to demonstrate no cognitive impairment on the Consortium to Establish a Registry for Alzheimer’s Disease neuropsychological battery (CERAD) word list recall and visuospatial memory tests, a MMSE score of 26 or greater, a clinical diagnostic rating scale (CDR) score of 0, and a Functional Assessment Staging Test (FAST) score of 2 or less, to be included in this study. Individuals with mild cognitive impairment (MCI) were diagnosed based on Petersen et al.’s (Reference Petersen, Caracciolo, Brayne, Gauthier, Jelic and Fratiglioni2014) criteria, which include subjective cognitive concerns, mild impairment in memory tests, intact activities of daily living, and a FAST score of 3.
In addition to other cognitive measures, all participants in this study took the Category Fluency (Animals) test (Lezak et al., Reference Lezak, Howieson, Loring and Fischer2004). This cognitive test asks participants to generate the names of as many animals they can think of in one minute and is considered a gold-standard cognitive test of semantic fluency and semantic access (Lezak et al., Reference Lezak, Howieson, Loring and Fischer2004). The total score of correctly named animals in the one-minute period is commonly used as an outcome measure in studies of cognition (Troyer, Reference Troyer2000). Beyond the total score, however, researchers can also examine words that are produced inside the same subcategory, as a qualitative approach to better understand cognitive strategies that are used during fluency performance (Fagundo et al., Reference Fagundo, López, Romero, Guarch, Marcos and Salamero2008). For example, some individuals may efficiently “cluster” animals that are related together (e.g., stating several domestic animals in sequence, followed by wild animals, etc.). A less efficient approach, conversely, may result in more switching between clusters (i.e., participants alternating frequently between categories of related animals).
In the present study, we used the following a priori categorization of animals into six main clusters, which was used previously by Fagundo et al. (Reference Fagundo, López, Romero, Guarch, Marcos and Salamero2008) for the study of patients with AD and was found to be sensitive to early AD: wild land animals, animals that live in the water, animals found on a farm, birds, pets (non-farm), and insects. The appendix provides a more detailed description of our clustering approach. Two co-authors who are native Spanish speakers and trained in neuropsychological assessment (AB and ABC) referenced the raw data forms for the Category Fluency (Animals) test for each participant in this study and identified the following variables: the total score of the test (total animals correctly generated in 1 minute); the number of clusters and switches generated by the participant; and the number of items contained within clusters. For our analyses, we primarily were interested in examining the total score of the test (which is commonly examined in other studies) and the total number of items contained within clusters, which were defined as two or more animals belonging to the same subcategory (see the appendix); we also examined the number of switches between clusters, but we expected that conceptually this variable would have an attenuated range given that the number of switches would inherently be a smaller number than the number of items contained within clusters. We also choose not to adjust for the number of items contained within clusters by the total score of the participant, as conceptually we believe that participants generating more words within clusters would be more efficient and, therefore, typically attain a higher total score.
As previously mentioned, participants travelled from Medellín, Colombia to Boston, Massachusetts as part of the Massachusetts General Hospital COLombia-BOSton (COLBOS) longitudinal study of biomarkers of ADAD. While in Boston these participants underwent PET scanning of amyloid-beta and tau, as has been previously described (Guzman-Velez et al., 2020; Quiroz et al., Reference Quiroz, Sperling, Norton, Baena, Arboleda-Velasquez, Cosio, Schultz, Lapoint, Guzman-Velez, Miller, Kim, Chen, Tariot, Lopera, Reiman and Johnson2018). In brief, 11C Pittsburgh compound B (PiB) was used as the tracer to identify the bilateral distribution volume ratio (DVR) of amyloid-beta in-vivo for each region of interest (ROI) using the Logan graphical method (Logan et al., Reference Logan, Fowler, Volkow, Wolf, Dewey, Schlyer and Gatley1990), with the cerebellar gray used as reference tissue. In-vivo tau was identified using Flortaucipir, with uptake expressed in FreeSurfer ROIs as the standardized uptake value ratio (SUVR) to the cerebellum, as has been done previously (Johnson et al., Reference Johnson, Schultz, Betensky, Becker, Sepulcre, Rentz, Mormino, Chhatwal, Amariglio, Papp, Marshall, Albers, Mauro, Pepin, Alverio, Judge, Philiossaint, Shoup, Yokell and Sperling2016).
Statistical analysis
Due to the non-normally distributed data in this sample, non-parametric Mann–Whitney U tests were used to assess group differences between carriers and noncarriers on demographics, as well as category fluency total score, category fluency clustering (i.e., the number of words contained within semantic clusters), and category fluency switching. Spearman correlations were used to assess associations between category fluency total score, category fluency clustering, demographic variables (e.g., age and education), and PET measurements of cortical amyloid-β (DVR) and regional tau (standardized uptake value ratios [SUVr] of entorhinal and inferior temporal cortices). These correlations were analyzed in noncarrier family members, all PSEN1 E280A mutation carriers (n = 29), and in the sub-set of mutation carriers who were cognitively unimpaired (n = 22). Correlation coefficients between carriers and noncarriers were compared using Fisher z transformations. These analyses were performed in SPSS v. 27.
For exploratory whole-brain analyses, Pearson correlations were used to assess the relationship between semantic memory scores and vertex-wise amyloid-β and tau PET within carriers. PET images were normalized to standard (MNI) space and projected onto the average surface, and vertex-wise values were sampled at the midpoint of the gray matter. Partial volume correction was applied using the extended Muller-Gartner method implemented in FreeSurfer (Greve et al., Reference Greve, Svarer, Fisher, Feng, Hansen, Baare, Rosen, Fischl and Knudsen2014). Results were displayed as –log10(p), significant at cluster-wise p < 0.05 (minimum cluster extent = 100 mm2) after false discovery rate (FDR) correction for multiple comparisons. These correlations and visualizations were performed in R v. 4.0.3; clustering and multiple comparisons corrections were performed using FreeSurfer v.6 tools.
Results
Sample characteristics
Descriptive statistics for the full sample’s demographic, cognitive, and PET imaging data are outlined in Table 1. Carriers and noncarriers did not differ on age or education, nor the proportion of males-to-females in each group (all p’s > .05). Carriers did have worse performance on the MMSE and greater neocortical amyloid-β and regional tau burden in temporal regions than noncarriers (all p < .01). The PSEN1 carriers and noncarriers did not differ on the total number of animals generated, the number of animals contained within clusters, the number of switches between clusters, nor the number of switches between categories of animals.
Association between semantic clustering and age in PSEN1 E280A carriers
Age was more strongly negatively associated with the number of animals contained in clusters in all PSEN1 carriers compared to noncarrier family members (all carriers: ϼ = −.34, p = .02 vs. noncarriers: ϼ = .11, p = .46; comparison: z = 2.24, p = .01). Among the cognitively unimpaired carriers only, age was also negatively associated with the number of animals contained in clusters at threshold significance, and this correlation was significantly stronger than the magnitude of the correlation in noncarriers (cognitively unimpaired carriers: ϼ = −.42, p = .05 vs. noncarriers: ϼ = .11, p = .46; comparison: z = 1.89, p = .03).
Association between semantic clustering, semantic fluency total score, and brain pathology in PSEN1 E280A carriers
Fewer animals contained in clusters was more strongly negatively associated with greater amyloid- burden (all carriers: ϼ = −.45, p = .01 vs. noncarriers: ϼ = .15, p = .43; comparison: z = 2.35, p = .009) and more entorhinal tau (all carriers: ϼ = −.59, p = .001 vs. noncarriers: ϼ = −.13, p = .49; comparison: z = 2.05, p = .02), but not more inferior temporal tau (all carriers: ϼ = −.12, p = .52 vs. noncarriers: ϼ = .19, p = .29; comparison: z = 1.17, p = .12) in all PSEN1 mutation carriers relative to noncarrier family members. Among the cognitively unimpaired carriers only, fewer animals contained in clusters was significantly negatively associated with entorhinal tau, though this correlation was not significantly stronger than the relationship seen in noncarriers (cognitively unimpaired carriers: ϼ = −.43, p = .05 vs. noncarriers: ϼ = −.13, p = .49; comparison: z = 2.05, p = .02). Similarly, in cognitively unimpaired carriers the items contained in clusters was not significantly associated with greater amyloid (ϼ = −.20, p = .38), or inferior temporal tau (ϼ = .02, p = .94).
Regarding the total score of animal fluency (i.e., total score irrespective of clustering), in all PSEN1 carriers lower animal fluency total score was more negatively associated with higher levels of entorhinal tau (all carriers: ϼ = −.46, p = .01 vs. noncarriers: ϼ = .25, p = .43; comparison: z = 2.86, p = .002) relative to noncarriers. Though all PSEN1 mutation carriers also exhibited a significantly stronger negative association between inferior temporal tau and animal fluency total score (all carriers: ϼ = −.31, p = .10 vs. noncarriers: ϼ = .15, p = .43; comparison: z = 1.76, p = .04), the correlation between inferior temporal tau and animal fluency total score was not significant itself in the group of all mutation carriers. No differences were found between all of the carriers and noncarriers in the relationship between animal fluency total score and neocortical amyloid (all carriers: ϼ = −.28, p = .15 vs. noncarriers: ϼ = −.12, p = .52; comparison: z = 0.62, p = .27). The total score of animal fluency was negatively correlated at threshold significance with age in the cognitively unimpaired carriers, and this relationship was stronger than the correlation observed in noncarriers (cognitively unimpaired carriers: ϼ = −.42, p = .05 vs. noncarriers: ϼ = .25, p = .43; comparison: z = 2.86, p = .009). However, the category fluency total score was not significantly correlated with neocortical amyloid (ϼ = −.06, p = .79), entorhinal tau (ϼ = −.32, p = .15), or inferior temporal tau (ϼ = −.09, p = .69) among only the cognitively unimpaired mutation carriers.
Exploratory whole-cortex analysis in PSEN1 carriers of amyloid and tau PET vs. animals contained within clusters revealed that amyloid in medial-parietal regions, as well as in frontal and lateral regions, was significantly negatively correlated with animal clustering after FDR correction (Figure 1, left panels). The relationship between tau and animal clustering in carriers, however, showed a lone cluster in the right medial temporal lobe that survived FDR correction (Figure 1, right panels).
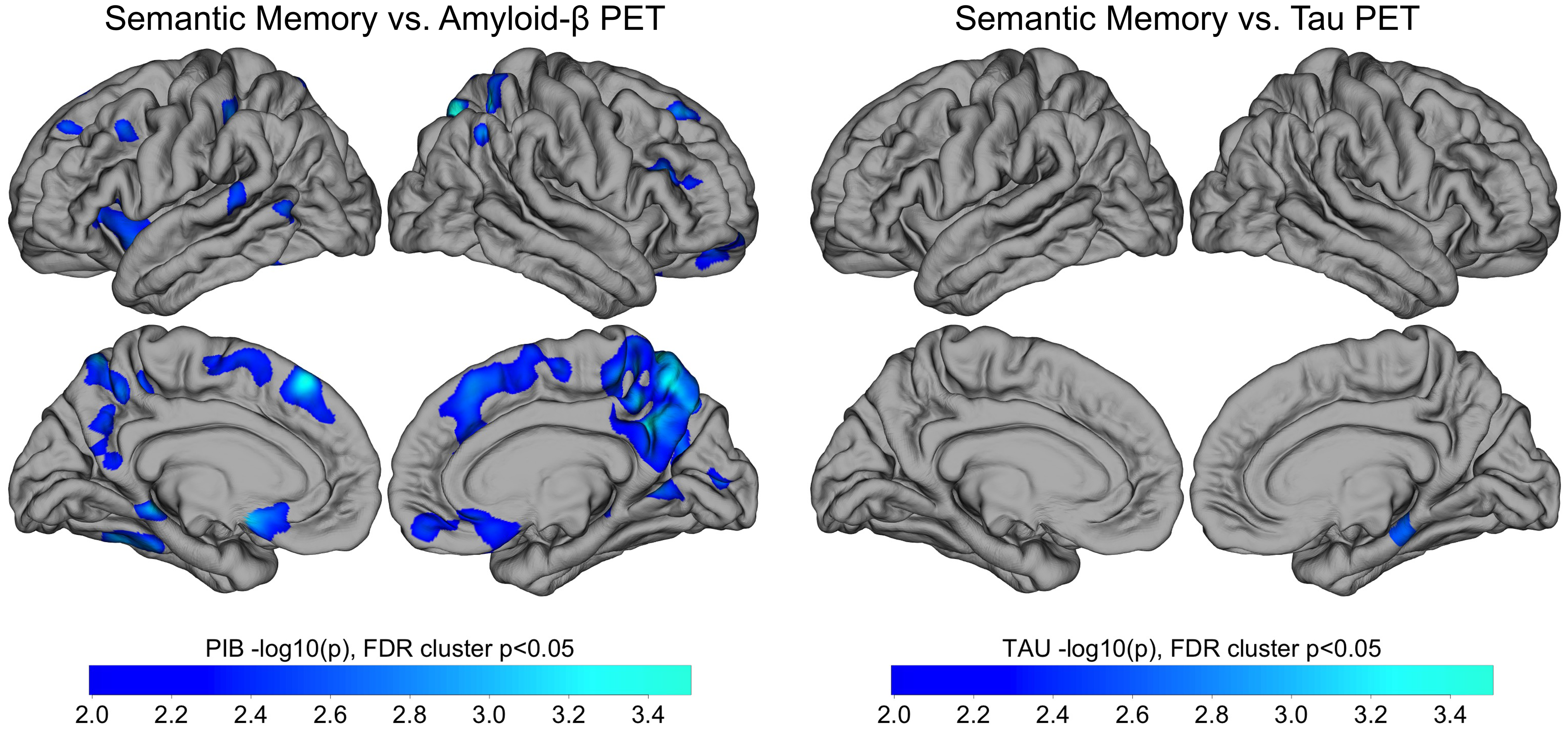
Figure 1. Whole Cortex Analysis of the Association of Category Fluency (Animals) Clustering with Amyloid and Tau PET. Whole-cortex analysis of amyloid PET (left) and tau PET (right) vs. semantic memory. Pearson correlations were performed between Category Fluency and amyloid-β (left) and tau (right) PET within all carriers (n = 29). Results are displayed as -log10(p), significant at cluster p < .05 after false discovery rate (FDR) correction. Notes: PET = positron emission tomography; PIB = Pittsburgh compound B.
Discussion
There is a growing need to understand how cognitive and biological markers of AD are related. In this study, we examined how the total score and clustering on a semantic fluency measure (Category Fluency Animals; Lezak et al., Reference Lezak, Howieson, Loring and Fischer2004) differed between nondemented carriers of the PSEN1 E280A ADAD mutation and noncarrier family members. We also evaluated how the total score and clustering score on the animal fluency task were related to in-vivo AD pathology. Contrary to our hypotheses, nondemented PSEN1 ADAD carriers and age- and education-matched noncarrier family members did not differ on animal fluency total score or clustering. Relative to noncarriers, however, ADAD carriers exhibited a stronger negative association between category fluency clustering with amyloid and tau protein levels. We specifically found that higher levels of neocortical amyloid and tau proteins were associated with fewer items listed within clusters, though this relationship was only seen when examining both cognitively unimpaired mutation carriers and carriers with MCI as a group. Category fluency clustering was also more strongly negatively correlated with age – a proxy for disease progression in ADAD (Fuller et al., Reference Fuller, Cronin-Golomb, Gatchel, Norton, Guzmán-Vélez, Jacobs, Hanseeuw, Pardilla-Delgado, Artola, Baena, Bocanegra, Kosik, Chen, Tariot, Johnson, Sperling, Reiman, Lopera and Quiroz2019) – in both nondemented ADAD carriers and in cognitively unimpaired carriers alone relative to noncarrier family members. These findings suggest a potential impact of AD pathophysiology on semantic networks that may underpin efficient word retrieval performance and semantic access in the preclinical and early stages of the disease.
Prior research using the animal fluency test (and clustering) suggests that semantic networks are impaired due to AD. In one study, participants with AD produced significantly smaller mean cluster sizes compared to control participants, and reduced clustering on the category fluency task was conceptualized as a possible deficit in the semantic network and the temporal lobe (Weakley & Schimitter-Edgecombe, 2014). In other recent studies, people with AD generated fewer category clusters and exhibited less switches between semantic subcategories relative to healthy controls (Haugrud et al., Reference Haugrud, Crossley and Vrbancic2011, Liampas et al., Reference Liampas, Folia, Zoupa, Siokas, Yannakoulia, Sakka, Hadjigeorgiou, Scarmeas, Dardiotis and Kosmidis2022, Tessaro et al., Reference Tessaro, Hermes-Pereira, Schilling, Fonseca, Kochhann and Hübner2020). Our current findings in this ADAD sample do not align with this prior research, as we did not see any significant behavioral differences between animal fluency total score nor clustering when comparing nondemented ADAD carriers and noncarrier family members. We speculate that our results do not align with these prior studies because two-thirds of our sample of PSEN1 E280A mutation carriers were cognitively unimpaired, whereas these prior studies examined differences on category fluency total score and clustering between healthy controls and individuals with MCI or dementia due to sporadic AD. In our study, we did find that the greater amyloid and tau burden in the brain was negatively correlated with animal fluency clustering in this sample, potentially indicating that in vivo AD pathological change may have subtle impacts early in the disease process on how individuals approach category fluency tasks. To our knowledge, few prior studies done on the topic of category fluency clustering have investigated how AD biomarker levels are related to category fluency clustering (one notable exception is Loewenstein et al., Reference Loewenstein, Curiel, DeKosky, Bauer, Rosselli, Guinjoan, Adjouadi, Peñate, Barker, Goenaga, Golde, Greig-Custo, Hanson, Li, Lizarraga, Marsiske and Duara2018). In this study we importantly were able to examine the associations of these category fluency variables with neocortical amyloid-beta and tau in medial temporal regions in the preclinical and early stages of the disease. Understanding how performance on the animal fluency test – a gold-standard cognitive measure – is related to in-vivo AD biomarkers is a crucial step in understanding the relationship between the cognitive and biological changes in AD and provides a foundation for future research in this area.
A key benefit of conducting this study with ADAD carriers and noncarriers versus people with sporadic AD is that sporadic AD is believed to be etiologically heterogeneous, and thus it is difficult to study relationships between biological and cognitive markers of sporadic AD in the preclinical stage of the disease. In ADAD, however, we can study carriers of ADAD mutations many decades before the onset of clinical symptoms (Fuller et al., Reference Fuller, Cronin-Golomb, Gatchel, Norton, Guzmán-Vélez, Jacobs, Hanseeuw, Pardilla-Delgado, Artola, Baena, Bocanegra, Kosik, Chen, Tariot, Johnson, Sperling, Reiman, Lopera and Quiroz2019). In the present study, the ADAD carriers and noncarrier family members were on average about 8 years younger than the median expected age of onset of MCI for this cohort at 44 years old (Acosta-Baena et al., Reference Acosta-Baena, Sepulveda-Falla, Lopera-Gómez, Jaramillo-Elorza, Moreno, Aguirre-Acevedo, Saldarriaga and Lopera2011).
This study, like others, has its limitations, including our use of a single-trial category fluency task (animals), which may attenuate the possible performances of the participants on this cognitive measure relative to an expanded category fluency task that includes multiple categories. Although the category of “animals” is considered to be common knowledge, using a three-trial category fluency test may be advisable as knowledge in different categories can be heavily influenced by culture and levels of education. Future studies should consider implementing three-trial category fluency (e.g., animals, vegetables, and fruits), in conjunction with phonemic fluency and object naming tests, to fully capture both the total score aspect of fluency tests in ADAD, as well as how people with ADAD mutations organize their approach to generation of words on fluency and language tasks.
Another limitation of this study is the relatively small sample size of the carrier and noncarrier groups, which limits statistical power and may confound our analyses. Previous studies from our group using PET imaging have used a similar sample size (e.g., Quiroz et al., Reference Quiroz, Sperling, Norton, Baena, Arboleda-Velasquez, Cosio, Schultz, Lapoint, Guzman-Velez, Miller, Kim, Chen, Tariot, Lopera, Reiman and Johnson2018) as our PET sub-sample. Future research would benefit from a larger sample and from studying this question with other AD biomarkers (e.g., plasma neurofilament light; Quiroz et al., Reference Quiroz, Zetterberg, Reiman, Chen, Su, Fox-Fuller, Garcia, Villegas, Sepulveda-Falla, Villada, Arboleda-Velasquez, Guzmán-Vélez, Vila-Castelar, Gordon, Schultz, Protas, Ghisays, Giraldo, Tirado and Lopera2020). A larger sample would also confer the ability for future studies to use more sophisticated statistical techniques that could examine the ability to identify carrier status early in the disease course based off category fluency clustering performance, such as discriminant analysis or logistic regression. It is also important to acknowledge, however, the rarity of ADAD, which makes the current sample size a large representative sample of its kind.
In conclusion, by investigating the relationship between brain pathology and performance on category fluency tests we found a significant correlation between levels of amyloid and tau on PET and the number of items nondemented ADAD mutation carriers listed within clusters, even though carriers and noncarriers did not differ in the total numbers of animals produced nor the number of animals contained within clusters. These findings suggest there is an association between brain AD pathophysiology and performance on certain cognitive tasks that tap into semantic abilities. Continued research that elucidates the relationships between imaging markers and cognitive performance in AD will prove fruitful as we seek to identify cognitive tasks (and sub-components of these tasks) that have high sensitivity in the detection of AD and are easy to administer, thereby increasing access to accurate AD diagnostics and potential enrollment into clinical trials.
Acknowledgements
The authors thank the PSEN1 Colombian families for contributing their valuable time and effort, without which this study would not have been possible. We thank the research staff of the Group of Neuroscience of Antioquia for their help coordinating study visits to Boston.
Funding statement
DY was supported with funding from the Boston University Undergraduate Research Opportunities Program. JTFF was supported by the US National Institute on Aging (F31AG062158-03). CVC was supported by a grant from the Alzheimer’s Association (AA Research Fellowship). YTQ was supported by grants from the National Institute on Aging ([R01 AG054671, RF1AG077627], the Alzheimer’s Association, and Massachusetts General Hospital ECOR).
Conflicts of interest
YTQ serves as consultant for Biogen. All other authors have nothing to disclose.
Appendix A A priori category fluency clustering groups
The table below shows the six a priori category fluency clustering groups used in this study (based on Fagundo et al., Reference Fagundo, López, Romero, Guarch, Marcos and Salamero2008). These groups were used to determine clusters when participants generated two or more animals in the same group in sequence, and switches when participants alternated between animals in these predetermined groups.
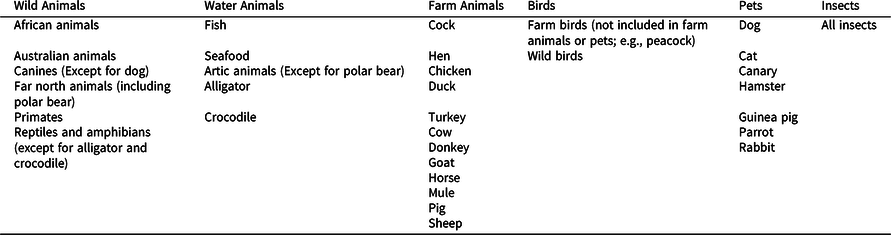