Impact statement
Pharmacoepigenetics studies how epigenetic mechanisms impact disease states, and the response to drugs. We summarize work in these areas and propose an approach to move the field forward by using publicly available data to identify pharmacoepigenetic interactions. These interactions promise an improved understanding of how to deliver more precise personalized medicine.
Introduction
Variation in genetics can lead to variation in the response to drugs. Pharmacogenetics (PGx) is the field of research that characterizes this relationship by examining how genetic variation correlates with pharmacological parameters such as pharmacokinetics and pharmacodynamics. In PGx, the simplified paradigm for understanding a gene–drug interaction is as follows: first, recognizing regions of genetic variation, second, identifying key functional changes such as single nucleotide polymorphisms (SNPs), and insertion and/or deletions (indels), and third, mapping these onto a drug response phenotype. This process represents a base case PGx scenario. However, there are cases where individuals have several variant genes of interest, called pharmacogenes. The effect from each pharmacogene complicates the analysis of how a single gene variant impacts drug response. PGx studies have been successful in explaining and predicting differences in drug response (Ross et al., Reference Ross, Anand, Joseph and Paré2012).
Genetic variation typically accounts for approximately 10–30% of observed differences in individual responses to drugs (Ross et al., Reference Ross, Anand, Joseph and Paré2012). Researchers have proposed that epigenetic effects, which modify gene expression without altering the genetic code, may also contribute to variation in drug response (Berger et al., Reference Berger, Kouzarides, Shiekhattar and Shilatifard2009; Gomez and Ingelman-Sundberg, Reference Gomez and Ingelman-Sundberg2009; Kacevska et al., Reference Kacevska, Ivanov and Ingelman-Sundberg2011; Ivanov et al., Reference Ivanov, Kacevska and Ingelman-Sundberg2012; Ingelman-Sundberg et al., Reference Ingelman-Sundberg, Zhong, Hankinson, Beedanagari, Yu, Peng and Osawa2013; Kim et al., Reference Kim, Han, Burckart and Oh2014; He et al., Reference He, Chevillet, Liu, Kim and Wang2015; Stefanska and MacEwan, Reference Stefanska and MacEwan2015; Cascorbi and Schwab, Reference Cascorbi and Schwab2016). Epigenetic mechanisms include DNA methylation (DNAm), hydroxymethylation, histone modification, chromatin architecture changes, and noncoding RNAs (although RNAs are not always considered epigenetic factors) (Kelly et al., Reference Kelly, De Carvalho and Jones2010). Epigenetic principles have been extensively studied in recent years, and a thorough review of these principles in the context of health and disease is given by Zhang et al. (Reference Zhang, Qianjin and Chang2020).
Epigenetic variants have been found near genes and gene regulators, which control the metabolism of drugs, suggesting a role for epigenetic mechanisms in modulating pharmacokinetics and pharmacodynamics (Kacevska et al., Reference Kacevska, Ivanov and Ingelman-Sundberg2012; He et al., Reference He, Chevillet, Liu, Kim and Wang2015; Shi et al., Reference Shi, Li, Song, Xu, Huo, Shen, Xing, Cui, Li, Zhao, He and Qin2017). Pharmacoepigenetics, is the field that studies how epigenetic variability impacts variability in drug response. We can use a similar approach as with PGx to study this field. First, we identify variation in epigenetic markers, second, we select key epigenetic biomarker(s) in regions of variance, and third, we map these biomarker(s) to a drug response phenotype.
We introduce the term forward pharmacoepigenetics to describe situations where the existing epigenetic state dictates response to drugs (Csoka and Szyf, Reference Csoka and Szyf2009; Cascorbi, Reference Cascorbi2013). However, as in PGx, there are more complex cases where epigenetics and drug response interact. Drugs can also modulate the epigenetic profile in a manner we call reverse pharmacoepigenetics, where compounds target epigenetic mechanisms to alter gene expression (Csoka and Szyf, Reference Csoka and Szyf2009; Kelly et al., Reference Kelly, De Carvalho and Jones2010; Lötsch et al., Reference Lötsch, Schneider, Reker, Parnham, Schneider, Geisslinger and Doehring2013; Ivanov et al., Reference Ivanov, Barragan and Ingelman-Sundberg2014; Figure 1). With some drugs, this is a side effect, but a subset of epigenetic drugs, which we will refer to as epi-drugs, are designed to alter epigenetic markers and are used to treat cancers, immune disorders, and mental health disorders (Peedicayil, Reference Peedicayil2014; Furtado et al., Reference Furtado, Dos Santos Luciano, Silva Santos, Furtado, Moraes and Pessoa2019; Licht and Bennett, Reference Licht and Bennett2021).

Figure 1. (a) Forward case of pharmacoepigenetics where basal DNAm influences drug response. Preexisting DNAm markers (Me) in the promoter region of the gene can down-regulate gene expression. This decreases the amount of gene product available for interaction with a drug. Since the DNAm affects the drug response phenotype, we call this forward pharmacoepigenetics. (b) Reverse case where drug changes DNAm and this in turn affects response phenotype. Here the drug is altering the methylation status of the gene promoter region, which leads to changes in downstream gene expression. Since the drug is affecting the DNAm, we call this reverse pharmacoepigenetics. In this scenario methylation at the promoter region downregulates gene expression, but this is not always the case. Created with BioRender.com.
Biomarkers, such as methylation patterns, are used to identify whether an epi-drug will be effective for a specific patient (Treppendahl et al., Reference Treppendahl, Kristensen and Grønbæk2014; Majchrzak-Celińska and Baer-Dubowska, Reference Majchrzak-Celińska and Baer-Dubowska2017). Therefore, much of the literature in the field of pharmacoepigenetics analyzes biomarkers related to epi-drugs. One class of biomarker analyses identifies which patient cohorts respond best to certain epi-drugs (Berdasco and Esteller, Reference Berdasco and Esteller2019; Incorvaia et al., Reference Incorvaia, Fanale, Badalamenti, Brando, Bono, De Luca, Algeri, Bonasera, Corsini, Scurria, Iovanna, Russo and Bazan2020). Another application seeks biomarkers that are indicative of epi-drug efficacy against a specific disease (Cheng et al., Reference Cheng, Kuo, Alakus, Frazer, Harismendy, Wang and Tseng2014; Alag, Reference Alag2019; Morel et al., Reference Morel, Jeffery, Aspeslagh, Almouzni and Postel-Vinay2020). These studies have generated a large volume of data following advances in epigenetic sequencing technology (Zhou et al., Reference Zhou, Li, Zhang, Lowdon, Rockweiler, Sears, Madden, Smirnov, Costello and Wang2015; Luo et al., Reference Luo, Hitz, Gabdank, Hilton, Kagda, Lam, Myers, Sud, Jou, Lin, Baymuradov, Graham, Litton, Miyasato, Strattan, Jolanki, Lee, Tanaka, Adenekan, O’Neill and Cherry2020; Boix et al., Reference Boix, James, Park, Meuleman and Kellis2021). The sequencing methods are reviewed in Cazaly et al. (Reference Cazaly, Saad, Wang, Heckman, Ollikainen and Tang2019). Computational methods to predict biomarkers and patient responses from the available information are emerging (Cazaly et al., Reference Cazaly, Saad, Wang, Heckman, Ollikainen and Tang2019). For example, machine learning (ML)-based algorithms trained on functionally validated pharmacogenomic biomarkers joined with clinical measures, predicted selective serotonin reuptake inhibitor (SSRI) remission and response in patients with major depressive disorder (Athreya et al., Reference Athreya, Neavin, Carrillo-Roa, Skime, Biernacka, Frye, Rush, Wang, Binder, Iyer, Weinshilboum and Bobo2019).
While epi-drug biomarker studies are important for improved patient diagnosis and treatment, they are not the focus of this review. Instead, we focus on the relationship between classical drug response and epigenetic variation (Gomez and Ingelman-Sundberg, Reference Gomez and Ingelman-Sundberg2009; Cascorbi, Reference Cascorbi2013; Lauschke et al., Reference Lauschke, Zhou and Ingelman-Sundberg2019). In PGx, researchers typically identify key SNPs from regions of genetic variance and map how they relate to the drug response phenotype. In pharmacoepigenetics, few studies have reported on how epigenetic variation relates to classical drug response. We find that (1) epigenetic variants can be indicative of disease and are varied throughout the population, (2) epigenetic variation can be summarized by key biomarkers, which predict diagnosis and prognosis, and (3) epigenetic variation impacts nonepigenetic drug response. We also demonstrate how publicly available data can be used to examine all aspects of the relationship between epigenetic variance and classical drug response to further our understanding of human biology and improve our treatment of disease.
Epigenetic variation in disease, drugs, and drug response
Epigenetic variation
Of the epigenetic mechanisms, DNA methylation (DNAm) is the most studied, and has been implicated in several disease phenotypes. DNAm at the promoter region of a gene is more likely to downregulate gene expression, while methylation in the body of the gene is more likely to increase expression (Jjingo et al., Reference Jjingo, Conley, Yi, Lunyak and Jordan2012). Cancers, immune diseases, and diabetic kidney disease are linked to differential methylation (Husquin et al., Reference Husquin, Rotival, Fagny, Quach, Zidane, McEwen, MacIsaac, Kobor, Aschard, Patin and Quintana-Murci2018; Kato and Natarajan, Reference Kato and Natarajan2019; Ochoa-Rosales et al., Reference Ochoa-Rosales, Portilla-Fernandez, Nano, Wilson, Lehne, Mishra, Gao, Ghanbari, Rueda-Ochoa, Juvinao-Quintero, Loh, Zhang, Kooner, Grabe, Felix, Schöttker, Zhang, Gieger, Müller-Nurasyid, Heier, Peters, Lehtimäki, Teumer, Brenner, Waldenberger, Ikram, van Meurs, Franco, Voortman, Chambers, Stricker and Muka2020). Mental illnesses including schizophrenia, bipolar disorder, major depressive disorder, Alzheimer’s, and autism may also be associated with variant methylation patterns (Tyrka et al., Reference Tyrka, Price, Marsit, Walters and Carpenter2012; Cacabelos and Torrellas, Reference Cacabelos and Torrellas2015; Andari et al., Reference Andari, Nishitani, Kaundinya, Caceres, Morrier, Ousley, Smith, Cubells and Young2020; Zhou et al., Reference Zhou, Li, Wang, He, Xia, Sweeney, Kopp, Liu and Chen2021). Based on these data, we focus the scope of our review on the relationship between DNAm and drug response. There are relationships between drug response and other epigenetic mechanisms, which are outlined in Kim et al. (Reference Kim, Han, Burckart and Oh2014), He et al. (Reference He, Chevillet, Liu, Kim and Wang2015), and Cascorbi and Schwab (Reference Cascorbi and Schwab2016).
Epigenetic variation relevant to disease can vary across ethnicities. Nielsen et al. (Reference Nielsen, Hamon, Yuferov, Jackson, Ho, Ott and Kreek2010) found that DNA methylation of the l-opioid receptor gene (OPRM1) promoter region varied across African American, Hispanic, and Caucasian ethnic groups. This was done in the context of a heroin addiction study, and in addition to higher methylation levels in former heroin addicts compared to controls, and there was a significant difference across ethnicities in both users and control subjects. Epigenetic polymorphisms of the gene CYP2D6 are present among Chinese Tibetan, Mongolian, Uygur, and Han populations (Qi et al., Reference Qi, Yin, Zhang and Wang2020). CYP2D6 is important for susceptibility to cardiovascular disease and drug metabolism. These studies demonstrate that methylation patterns vary across different populations in genes relevant to disease phenotype and drug response.
Key epigenetic biomarkers predict diagnosis and prognosis
For diseases with an epigenetic component, it is useful to identify key biomarkers representing differential epigenetic states associated with disease diagnosis, prognosis, and treatment efficacy. Duruisseaux et al. (Reference Duruisseaux, Martínez-Cardús, Calleja-Cervantes, Moran, Castro de Moura, Davalos, Piñeyro, Sanchez-Cespedes, Girard, Brevet, Giroux-Leprieur, Dumenil, Pradotto, Bironzo, Capelletto, Novello, Cortot, Copin, Karachaliou, Gonzalez-Cao, Peralta, Montuenga, Gil-Bazo, Baraibar, Lozano, Varela, Ruffinelli, Palmero, Nadal, Moran, Perez, Ramos, Xiao, Fernandez, Fraga, Gut, Gut, Teixidó, Vilariño, Prat, Reguart, Benito, Garrido, Barragan, Emile, Rosell, Brambilla and Esteller2018) identified key DNAm biomarkers that were predictive of response to anti-programmed death 1 (anti-PD-1) treatment in nonsmall-cell lung cancer patients. In an epilepsy study, a methylation signature defined temporal lobe epilepsy and predicted drug resistance in patients (Xiao et al., Reference Xiao, Nobre, Piñeiro, Berciano-Guerrero, Alba, Cobo, Lauschke and Barragán2020).
Computational models can aid in identifying epigenetic biomarkers, which correlate to disease. For example, three ML programs were trained on the sequence of DNA with CG repeats (CpG sites). Researchers used these three programs (a complexity-optimized classifier, a decision tree, and a miRNA expression-based decision tree) to predict paclitaxel-sensitive and resistant breast cancer tumors (Bomane et al., Reference Bomane, Gonçalves and Ballester2019). ML programs have also used drug similarity data and cancer cell similarity matrices to predict the sensitivity of various cancer cell lines to novel drugs. The performance of this ML prediction model using DNAm was comparable to that of experimentally based information from oncogene mutation and gene expression data (Yuan et al., Reference Yuan, Chen and Wang2020). These studies demonstrate that biomarkers represent epigenetic variance in a manner, which can be used to predict disease phenotype, prognosis, and treatment efficacy.
Epigenetic variation impacts nonepigenetic drug response
Epigenetic profiles vary in the population, are associated with several diseases, and can be represented by biomarkers to identify and predict disease phenotypes. Epigenetic alterations can also influence the response to nonepigenetic drugs. For example, methylation patterns in patients with Fragile X syndrome correlate with a differential response to a mGluR5 antagonist, which may alleviate some symptoms of the disorder (Jacquemont et al., Reference Jacquemont, Curie, des Portes, Torrioli, Berry-Kravis, Hagerman, Ramos, Cornish, He, Paulding, Neri, Chen, Hadjikhani, Martinet, Meyer, Beckmann, Delange, Brun, Bussy, Gasparini, Hilse, Floesser, Branson, Bilbe, Johns and Gomez-Mancilla2011). In another hereditary disease, hyperhomocysteinemia, there is a consistent association between methylation levels of Betaine–Homocysteine S-Methyltransferase and folate therapy efficacy (Li et al., Reference Li, Yang, Zhao, Zhang, Ren, Yue, Du, Godfrey, Huang and Zhang2019).
Differences in methylation status correlate with the presence and severity of several mental health disorders. Methylation status also impacts response to anti-psychotic treatment (Swathy et al., Reference Swathy, Saradalekshmi, Nair, Nair and Banerjee2018). One example of this relationship is that DNA methylation status in the interleukin-11 gene predicts clinical response to antidepressants (Powell et al., Reference Powell, Smith, Hackinger, Schalkwyk, Uher, McGuffin, Mill and Tansey2013). Another study done on the Chinese Han population found that response to the anti-psychotic risperidone could be explained by both SNPs in key genes and CpG islands in the promoter or gene coding regions of those genes (Shi et al., Reference Shi, Li, Song, Xu, Huo, Shen, Xing, Cui, Li, Zhao, He and Qin2017). Specifically, examining methylation in CYP3A4, CYP2D6, ABCB1, HTR2A, and DRD2 genes revealed seven significant CpG sites within the promoter or coding regions of these genes. Zhou et al. (Reference Zhou, Li, Wang, He, Xia, Sweeney, Kopp, Liu and Chen2021) expand on the correlative relationship between DNAm profiles and clinical response to antipsychotic drugs in a retrospective study.
Most of the genes identified in these studies are involved in drug metabolism. Absorption, distribution, metabolism, and excretion (ADME) genes often vary in expression level. This variation impacts drug pharmacokinetics. Cytochrome P450 (CYP) genes are ADME genes and there is evidence that some unexplained variance in their level of function is attributable to methylation (Kacevska et al., Reference Kacevska, Ivanov and Ingelman-Sundberg2012; Shi et al., Reference Shi, Li, Song, Xu, Huo, Shen, Xing, Cui, Li, Zhao, He and Qin2017; Xiong et al., Reference Xiong, Yang, Li, Ma, Zhao, Wang, Li, Zheng, Zou, Zong, Kang, Jia, Li, Zhang and Bao2022). Polymorphisms in CYP450 and another gene, ABCB1, and their respective DNA methylation statuses significantly altered risk for steroid-induced osteonecrosis in the femoral head in Chinese populations (Huang et al., Reference Huang, Zhan, Hu, Yang, Cheng, Han and Yue2020). Moreover, methylation of ABCB1 also had a significant effect on aspirin resistance in Chinese ischemic stroke patients (Xu and Wang, Reference Xu and Wang2022). Furthermore, methylation of CYP1A1 was shown to modulate stable warfarin dosage in Chinese patients (He et al., Reference He, Wu, Yan, Liu, Zhao, Xie, Ge and Ye2021).
CYP3A4 is another cytochrome P450 family member that exhibits high interindividual variation in hepatic expression. Much of the variability in CYP3A4 remains unexplained. However, there exist highly variable CpG methylation sites in adult livers, which correspond to important CYP3A4 transcription factor binding sites at the proximal promoter. This suggests that the variance in the expression of CYP3A4 in adult livers may be due to methylation of the proximal promoter region (Kacevska et al., Reference Kacevska, Ivanov and Ingelman-Sundberg2012).
Epigenetic and pharmacogenomic resources
Several publicly available resources provide useful epigenetic and pharmacogenomic information. These resources derive from initiatives to aggregate molecular association studies to create data resources that are publicly available (The GTEX Consortium, 2020; Min et al., Reference Min, Hemani, Hannon, Dekkers, Castillo-Fernandez, Luijk, Carnero-Montoro, Lawson, Burrows, Suderman, Bretherick, Richardson, Klughammer, Iotchkova, Sharp, Al Khleifat, Shatunov, Iacoangeli, McArdle, Ho, Kumar, Söderhäll, Soriano-Tárraga, Giralt-Steinhauer, Kazmi, Mason, McRae, Corcoran, Sugden, Kasela, Cardona, Day, Cugliari, Viberti, Guarrera, Lerro, Gupta, Bollepalli, Mandaviya, Zeng, Clarke, Walker, Schmoll, Czamara, Ruiz-Arenas, Rezwan, Marioni, Lin, Awaloff, Germain, Aïssi, Zwamborn, van Eijk, Dekker, van Dongen, Hottenga, Willemsen, Xu, Barturen, Català-Moll, Kerick, Wang, Melton, Elliott, Shin, Bernard, Yet, Smart, Gorrie-Stone, Shaw, Al Chalabi, Ring, Pershagen, Melén, Jiménez-Conde, Roquer, Lawlor, Wright, Martin, Montgomery, Moffitt, Poulton, Esko, Milani, Metspalu, Perry, Ong, Wareham, Matullo, Sacerdote, Panico, Caspi, Arseneault, Gagnon, Ollikainen, Kaprio, Felix, Rivadeneira, Tiemeier, van IJzendoorn, Uitterlinden, Jaddoe, Haley, McIntosh, Evans, Murray, Räikkönen, Lahti, Nohr, Sørensen, Hansen, Morgen, Binder, Lucae, Gonzalez, Bustamante, Sunyer, Holloway, Karmaus, Zhang, Deary, Wray, Starr, Beekman, van Heemst, Slagboom, Morange, Trégouët, Veldink, Davies, de Geus, Boomsma, Vonk, Brunekreef, Koppelman, Alarcón-Riquelme, Huang, Pennell, van Meurs, Ikram, Hughes, Tillin, Chaturvedi, Pausova, Paus, Spector, Kumari, Schalkwyk, Visscher, Davey Smith, Bock, Gaunt, Bell, Heijmans, Mill and Relton2021; Battram et al., Reference Battram, Yousefi, Crawford, Prince, Babaei, Sharp, Hatcher, Vega-Salas, Khodabakhsh, Whitehurst, Langdon, Mahoney, Elliott, Mancano, Lee, Watkins, Lay, Hemani, Gaunt, Relton, Staley and Suderman2022; Ruiz-Arenas et al., Reference Ruiz-Arenas, Hernandez-Ferrer, Vives-Usano, Marí, Quintela, Mason, Cadiou, Casas, Andrusaityte, Gutzkow, Vafeiadi, Wright, Lepeule, Grazuleviciene, Chatzi, Carracedo, Estivill, Marti, Escaramís, Vrijheid, González and Bustamante2022; Xiong et al., Reference Xiong, Yang, Li, Ma, Zhao, Wang, Li, Zheng, Zou, Zong, Kang, Jia, Li, Zhang and Bao2022). We focus on DNAm as an epigenetic marker for which putative pharmacoepigenetic relationships can be elucidated using available data.
The Genetics of DNA Methylation Consortium (GoDMC) is an international collaboration that aggregated data from >30,000 study participants to provide associations between genetic variants and DNAm sites in the general population known (Min et al., Reference Min, Hemani, Hannon, Dekkers, Castillo-Fernandez, Luijk, Carnero-Montoro, Lawson, Burrows, Suderman, Bretherick, Richardson, Klughammer, Iotchkova, Sharp, Al Khleifat, Shatunov, Iacoangeli, McArdle, Ho, Kumar, Söderhäll, Soriano-Tárraga, Giralt-Steinhauer, Kazmi, Mason, McRae, Corcoran, Sugden, Kasela, Cardona, Day, Cugliari, Viberti, Guarrera, Lerro, Gupta, Bollepalli, Mandaviya, Zeng, Clarke, Walker, Schmoll, Czamara, Ruiz-Arenas, Rezwan, Marioni, Lin, Awaloff, Germain, Aïssi, Zwamborn, van Eijk, Dekker, van Dongen, Hottenga, Willemsen, Xu, Barturen, Català-Moll, Kerick, Wang, Melton, Elliott, Shin, Bernard, Yet, Smart, Gorrie-Stone, Shaw, Al Chalabi, Ring, Pershagen, Melén, Jiménez-Conde, Roquer, Lawlor, Wright, Martin, Montgomery, Moffitt, Poulton, Esko, Milani, Metspalu, Perry, Ong, Wareham, Matullo, Sacerdote, Panico, Caspi, Arseneault, Gagnon, Ollikainen, Kaprio, Felix, Rivadeneira, Tiemeier, van IJzendoorn, Uitterlinden, Jaddoe, Haley, McIntosh, Evans, Murray, Räikkönen, Lahti, Nohr, Sørensen, Hansen, Morgen, Binder, Lucae, Gonzalez, Bustamante, Sunyer, Holloway, Karmaus, Zhang, Deary, Wray, Starr, Beekman, van Heemst, Slagboom, Morange, Trégouët, Veldink, Davies, de Geus, Boomsma, Vonk, Brunekreef, Koppelman, Alarcón-Riquelme, Huang, Pennell, van Meurs, Ikram, Hughes, Tillin, Chaturvedi, Pausova, Paus, Spector, Kumari, Schalkwyk, Visscher, Davey Smith, Bock, Gaunt, Bell, Heijmans, Mill and Relton2021). Epigenome-wide association studies (EWAS) characterize the association between DNAm and phenotypic outcomes such as aging and smoking, but also pharmacogenomic (PGx) outcomes. Over 2,500 such studies are hosted on the EWAS catalog (Battram et al., Reference Battram, Yousefi, Crawford, Prince, Babaei, Sharp, Hatcher, Vega-Salas, Khodabakhsh, Whitehurst, Langdon, Mahoney, Elliott, Mancano, Lee, Watkins, Lay, Hemani, Gaunt, Relton, Staley and Suderman2022), and the EWAS Atlas (Xiong et al., Reference Xiong, Yang, Li, Ma, Zhao, Wang, Li, Zheng, Zou, Zong, Kang, Jia, Li, Zhang and Bao2022), and the GTEx project (The GTEX Consortium, 2020). To further elucidate the pathway from DNAm to phenotype, association studies between methylation and gene expression can provide insightful information (Ruiz-Arenas et al., Reference Ruiz-Arenas, Hernandez-Ferrer, Vives-Usano, Marí, Quintela, Mason, Cadiou, Casas, Andrusaityte, Gutzkow, Vafeiadi, Wright, Lepeule, Grazuleviciene, Chatzi, Carracedo, Estivill, Marti, Escaramís, Vrijheid, González and Bustamante2022), as do omics quantitative trait loci (QTL) data (The GTEX Consortium, 2020). In Table 1, we present publicly available resources that provide summary statistics of these molecular associations that could help identify causal pathways in the context of pharmacoepigenetics as subsequently discussed for the anti-psychotic compound clozapine (section “Synthesizing available pharmacoepigenetic data to investigate pharmacoepigenetic interactions”).
Table 1. Public resources with quantitative molecular interaction information that directly or indirectly involve DNAm

Note: Resources to query interaction information (1–5) as displayed in Figure 2 are outlined for genetic variants, PGx outcome (e.g., differential drug response, ADR), DNAm at CpG sites, gene expression, and omics levels. Each interaction involves two of these entities and to facilitate navigation, we explicitly name them (i.e., genetic variant – DNAm level) while also mentioning the common name of the intended analysis (i.e., mQTL). Information about the rs2472297-CYP1A1/CYP1A2 – clozapine example is provided when available. This list of resources is not exhaustive as we focused on large databases with user-friendly interfaces. As such, references supporting the clozapine examples also stem from other resources.
In section “Synthesizing available pharmacoepigenetic data to investigate pharmacoepigenetic interactions,” we illustrate the use of these resources to examine the epigenetics of clozapine. There are several relevant resources. dMEM, or the database of Epigenetic Modifiers, maintains the genomic information of about 167 epigenetic cancer target modifiers and proteins including DNAm and histone modification and microRNAs (Singh Nanda et al., Reference Singh Nanda, Kumar and Raghava2016). Consortia such as NIH Roadmap Epigenomics and the Encyclopedia of DNA Elements (ENCODE) provide data portals containing thousands of sequencing-based genome-wide epigenetic datasets (Fingerman et al., Reference Fingerman, McDaniel, Zhang, Ratzat, Hassan, Jiang, Cohen and Schuler2011; Luo et al., Reference Luo, Hitz, Gabdank, Hilton, Kagda, Lam, Myers, Sud, Jou, Lin, Baymuradov, Graham, Litton, Miyasato, Strattan, Jolanki, Lee, Tanaka, Adenekan, O’Neill and Cherry2020). Another epigenetic resource, Epimap, combines 10,000 epigenomic maps across 800 samples, which annotate chromatin states, high-resolution enhancers, enhancer modules, upstream regulators, and downstream target genes (Boix et al., Reference Boix, James, Park, Meuleman and Kellis2021).
Genome browsers such as the Washington University Epigenome Browser, offer a visualization platform that integrates consortia data for browsing and downloading (Zhou et al., Reference Zhou, Li, Zhang, Lowdon, Rockweiler, Sears, Madden, Smirnov, Costello and Wang2015). The UCSC genome browser offers a GTEx track, which allows users to examine epigenetic variation and gene expression in a variety of human tissues (Kent et al., Reference Kent, Sugnet, Furey, Roskin, Pringle, Zahler and Haussler2002; Navarro Gonzalez et al., Reference Navarro Gonzalez, Zweig, Speir, Schmelter, Rosenbloom, Raney, Powell, Nassar, Maulding, Lee, Lee, Hinrichs, Fyfe, Fernandes, Diekhans, Clawson, Casper, Benet-Pagès, Barber, Haussler, Kuhn, Haeussler and Kent2021).
Aside from databases examining epigenetic markers, there are databases that provide drug-response information. The PharmGKB provides curated drug label annotations, clinical guideline annotations, FDA annotations, genetic variant annotations, curated pathways, and annotations for hundreds of drugs (Whirl-Carrillo et al., Reference Whirl-Carrillo, Huddart, Gong, Sangkuhl, Thorn, Whaley and Klein2021). DGIdb (Drug–Gene Interaction database) provides information on drug–gene interactions and druggable genes from publications, databases, and other web-based sources (Freshour et al., Reference Freshour, Kiwala, Cotto, Coffman, McMichael, Song, Griffith, Griffith and Wagner2021). DrugBank offers chemical, pharmacological, and pharmaceutical data with comprehensive drug target information (Wishart et al., Reference Wishart, Feunang, Guo, Lo, Marcu, Grant, Sajed, Johnson, Li, Sayeeda, Assempour, Iynkkaran, Liu, Maciejewski, Gale, Wilson, Chin, Cummings, Le, Pon, Knox and Wilson2018). Additionally, the human enhancer drug database (HEDD) integrates epigenetic drug datasets obtained from laboratory experiments and manually curated information. HEDD incorporates five kinds of datasets: (1) drug, (2) target, (3) disease, (4) high-throughput, and (5) complex datasets (Qi et al., Reference Qi, Wang, Wang, Jin, Yang, Wu, Li, Zhao, Du, Song and Wang2016). Pharmacogenomic variation nomenclature is standardized within the PharmVar consortium as a centralized pharmacogene variation data and pharmacogenomic nomenclature repository (Gaedigk et al., Reference Gaedigk, Casey, Whirl-Carrillo, Miller and Klein2021).
Synthesizing available pharmacoepigenetic data to investigate pharmacoepigenetic interactions
Clozapine is commonly prescribed for schizophrenia. There is a wealth of information, which can be assembled about it from existing epigenetic and PGx resources. We illustrate how pharmacoepigenetic mechanisms can be hypothesized by integrating different datasets (Figure 2 and Table 1).
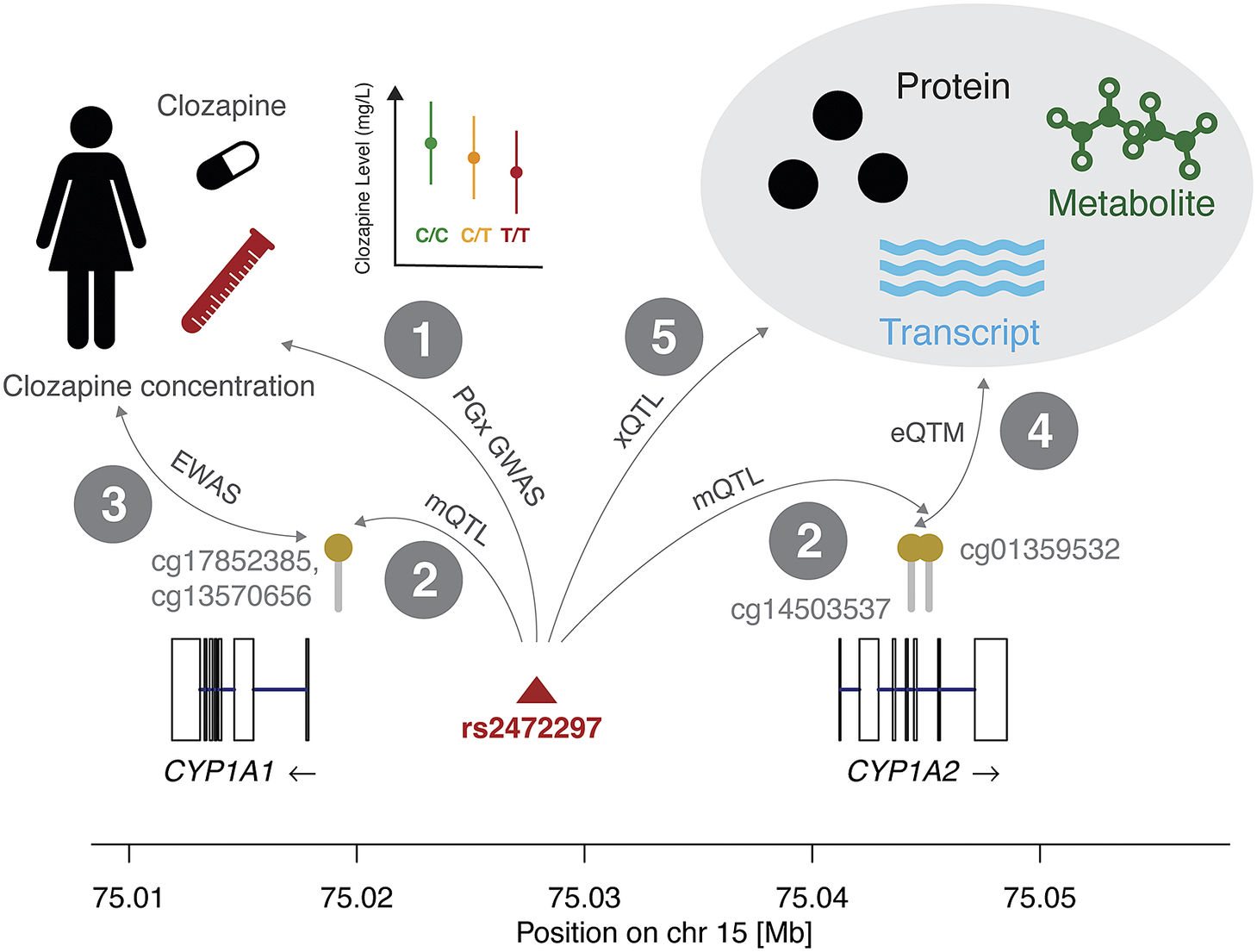
Figure 2. Combining molecular interaction resources can detect putative causal mechanisms that determine differential drug responses because of DNAm. We show interactions between genetic variants (SNPs), methylation status at CpG sites, drug response, expression levels, and other omics measures. In interaction 1, we report the association between the reduction in clozapine concentration and the minor allele of rs2472297 (Pardiñas et al., Reference Pardiñas, Nalmpanti, Pocklington, Legge, Medway, King, Jansen, Helthuis, Zammit, MacCabe, Owen, O’Donovan and Walters2019). In interaction 2, we present the CpG sites in vicinity of the CYP1A1 and CYP1A2 genes whose methylation levels are under the genetic influence of rs2472297 (GoDMC mQTL study). This suggests that epigenetic mechanisms may affect clozapine concentration. Interaction 3 indicates the association results that could be expected from an EWAS on clozapine concentration, however, such data is currently not available for this compound. Interaction 4 represents the link between methylation and expression levels (eQTM) that could support the role of CYP1A1 or CYP1A2 as mediators in this hypothetical epigenetic mechanism. Interaction 5 represents genetic associations to omics data such as mRNA expression, protein levels, and metabolite levels, which could further provide mechanistic insights and elucidate downstream effects of methylation on clozapine concentration through other omics layers. This figure is accompanied by Table 1 with public resources to query quantitative information corresponding to these interactions. The molecular mechanism depicted here is based on the significant effect of rs2472297 on clozapine metabolite plasma concentration (Pardiñas et al., Reference Pardiñas, Nalmpanti, Pocklington, Legge, Medway, King, Jansen, Helthuis, Zammit, MacCabe, Owen, O’Donovan and Walters2019). As outlined above, support for other interactions is often missing and we detail the degree of evidence in Table 1.
Clozapine has high interindividual differences in plasma clozapine concentration at a given dose and the risk of serious adverse drug reactions (ADR) at high concentrations can make its use challenging (Molden, Reference Molden2021). A recent PGx genome-wide association study (GWAS) (N = 2,989) identified a single genetic variant (rs2472297) associated with plasma clozapine concentration located between CYP1A1 and CYP1A2 (interaction 1, Figure 2).
Consulting the GoDMC methylation QTL (mQTL) resource, we associated this SNP to three CpG sites, two (cg13570656, cg17852385) located in the proximity of the transcription start site of CYP1A1 and one in the CYP1A2 intron 5–6 (cg01359532; interaction 2). To the best of our knowledge, no epigenome-wide association study (EWAS) on clozapine plasma concentration has been reported, which could relate methylation status to observed interindividual concentrations (interaction 3). Previous studies provided evidence for inverse correlations between DNAm and CYP1A2 mRNA levels in liver (Ghotbi et al., Reference Ghotbi, Gomez, Milani, Tybring, Syvänen, Bertilsson, Ingelman-Sundberg and Aklillu2009; Bonder et al., Reference Bonder, Kasela, Kals, Tamm, Lokk, Barragan, Buurman, Deelen, Greve, Ivanov, Rensen, van Vliet-Ostaptchouk, Wolfs, Fu, Hofker, Wijmenga, Zhernakova, Ingelman-Sundberg, Franke and Milani2014), among which is an inverse correlation between the intronic cg14503537 and CYP1A2 (interaction 4) (Bonder et al., Reference Bonder, Kasela, Kals, Tamm, Lokk, Barragan, Buurman, Deelen, Greve, Ivanov, Rensen, van Vliet-Ostaptchouk, Wolfs, Fu, Hofker, Wijmenga, Zhernakova, Ingelman-Sundberg, Franke and Milani2014). However, no significant correlation between any of the previously listed mQTL CpG sites and CYP1A1/CYP1A2 was found in either liver or blood (Bonder et al., Reference Bonder, Kasela, Kals, Tamm, Lokk, Barragan, Buurman, Deelen, Greve, Ivanov, Rensen, van Vliet-Ostaptchouk, Wolfs, Fu, Hofker, Wijmenga, Zhernakova, Ingelman-Sundberg, Franke and Milani2014; Ruiz-Arenas et al., Reference Ruiz-Arenas, Hernandez-Ferrer, Vives-Usano, Marí, Quintela, Mason, Cadiou, Casas, Andrusaityte, Gutzkow, Vafeiadi, Wright, Lepeule, Grazuleviciene, Chatzi, Carracedo, Estivill, Marti, Escaramís, Vrijheid, González and Bustamante2022). Likewise, no expression QTL (eQTL) data involving these two genes was reported for rs2472297 in the GTEx project (interaction 5) (The GTEX Consortium, 2020).
While the data may suggest a relationship between rs2472297 and clozapine concentration through DNAm, the information is too sparse to exclude horizontal pleiotropy (i.e., the SNP affecting DNAm and clozapine concentration independently). However, DNAm may be a consequence of the lower gene expression due to the proximal SNP. Given the absence of a genetic association with expression levels, further studies would be necessary to corroborate this hypothetical mechanism. Even this simple example with clozapine demonstrates the complex space of possible direct and indirect pathways, including forward and reverse directionalities, and warrants caution when integrating and interpreting molecular associations.
Synthesizing available pharmacoepigenetic data to investigate the DNAm landscape of CYP genes
CYPs play a major role in the metabolism of a large fraction of drugs and have notable variance in their expression, explained by both genetic and nongenetic factors. Studies previously discussed (section “Epigenetic variation impacts nonepigenetic drug response”) identify methylation near CYP promoter regions as a possible cause for differential drug response. We searched the GoDMC database to assess the DNAm landscape in 10 major CYP genes involved in drug metabolism (Min et al., Reference Min, Hemani, Hannon, Dekkers, Castillo-Fernandez, Luijk, Carnero-Montoro, Lawson, Burrows, Suderman, Bretherick, Richardson, Klughammer, Iotchkova, Sharp, Al Khleifat, Shatunov, Iacoangeli, McArdle, Ho, Kumar, Söderhäll, Soriano-Tárraga, Giralt-Steinhauer, Kazmi, Mason, McRae, Corcoran, Sugden, Kasela, Cardona, Day, Cugliari, Viberti, Guarrera, Lerro, Gupta, Bollepalli, Mandaviya, Zeng, Clarke, Walker, Schmoll, Czamara, Ruiz-Arenas, Rezwan, Marioni, Lin, Awaloff, Germain, Aïssi, Zwamborn, van Eijk, Dekker, van Dongen, Hottenga, Willemsen, Xu, Barturen, Català-Moll, Kerick, Wang, Melton, Elliott, Shin, Bernard, Yet, Smart, Gorrie-Stone, Shaw, Al Chalabi, Ring, Pershagen, Melén, Jiménez-Conde, Roquer, Lawlor, Wright, Martin, Montgomery, Moffitt, Poulton, Esko, Milani, Metspalu, Perry, Ong, Wareham, Matullo, Sacerdote, Panico, Caspi, Arseneault, Gagnon, Ollikainen, Kaprio, Felix, Rivadeneira, Tiemeier, van IJzendoorn, Uitterlinden, Jaddoe, Haley, McIntosh, Evans, Murray, Räikkönen, Lahti, Nohr, Sørensen, Hansen, Morgen, Binder, Lucae, Gonzalez, Bustamante, Sunyer, Holloway, Karmaus, Zhang, Deary, Wray, Starr, Beekman, van Heemst, Slagboom, Morange, Trégouët, Veldink, Davies, de Geus, Boomsma, Vonk, Brunekreef, Koppelman, Alarcón-Riquelme, Huang, Pennell, van Meurs, Ikram, Hughes, Tillin, Chaturvedi, Pausova, Paus, Spector, Kumari, Schalkwyk, Visscher, Davey Smith, Bock, Gaunt, Bell, Heijmans, Mill and Relton2021).
GoDMC provides cis and trans-mQTL information based on the analysis of 420,509 DNAm sites. We restricted our search to DNAm sites located 50kB up- and downstream of the CYP gene of interest and downloaded available data including the average DNAm level of these sites in the general population, mQTLs in cis influencing their DNAm levels, the minor allele frequency (MAF) of these mQTLs, and the estimated heritability of DNAm levels based on cis-mQTLs. In Table 2, we summarized this information. The full query results can be found in Supplementary Table S1.
Table 2. Summary of DNAm variation for 10 CYP genes

Note: For each gene, we include the chromosome, number of DNAm sites found 50 kB up- and downstream the gene boundaries, minimum and maximum mean DNAm levels, maximum mQTL minor allele frequency (MAF), and the minimum and maximum DNAm cis-heritability values. The mean DNAm level indicates the average methylation status of a given DNAm site in the studied population. The MAF corresponds to the MAF of the top mQTL (mQTL most significantly associated with that DNAm site) and the DNAm cis-heritability is the extent of DNAm variation explained by genetic variation in proximity of the gene which we derived by summing up the explained variance of independent cis-mQTLs. Only DNAm sites available in the GoDMC resource (whole blood) are reported with the study population being of European ancestry. Full query results with detailed mQTL association information can be found in Supplementary Table S1.
DNAm levels in CYP genes range from 1.8% up to 97.9%. As a comparison, genome-wide DNAm levels are reported on average 52% across the ~420,000 tested CpG sites. However, only 21% of the CpG sites are in transcription factor-binding regions (Min et al., Reference Min, Hemani, Hannon, Dekkers, Castillo-Fernandez, Luijk, Carnero-Montoro, Lawson, Burrows, Suderman, Bretherick, Richardson, Klughammer, Iotchkova, Sharp, Al Khleifat, Shatunov, Iacoangeli, McArdle, Ho, Kumar, Söderhäll, Soriano-Tárraga, Giralt-Steinhauer, Kazmi, Mason, McRae, Corcoran, Sugden, Kasela, Cardona, Day, Cugliari, Viberti, Guarrera, Lerro, Gupta, Bollepalli, Mandaviya, Zeng, Clarke, Walker, Schmoll, Czamara, Ruiz-Arenas, Rezwan, Marioni, Lin, Awaloff, Germain, Aïssi, Zwamborn, van Eijk, Dekker, van Dongen, Hottenga, Willemsen, Xu, Barturen, Català-Moll, Kerick, Wang, Melton, Elliott, Shin, Bernard, Yet, Smart, Gorrie-Stone, Shaw, Al Chalabi, Ring, Pershagen, Melén, Jiménez-Conde, Roquer, Lawlor, Wright, Martin, Montgomery, Moffitt, Poulton, Esko, Milani, Metspalu, Perry, Ong, Wareham, Matullo, Sacerdote, Panico, Caspi, Arseneault, Gagnon, Ollikainen, Kaprio, Felix, Rivadeneira, Tiemeier, van IJzendoorn, Uitterlinden, Jaddoe, Haley, McIntosh, Evans, Murray, Räikkönen, Lahti, Nohr, Sørensen, Hansen, Morgen, Binder, Lucae, Gonzalez, Bustamante, Sunyer, Holloway, Karmaus, Zhang, Deary, Wray, Starr, Beekman, van Heemst, Slagboom, Morange, Trégouët, Veldink, Davies, de Geus, Boomsma, Vonk, Brunekreef, Koppelman, Alarcón-Riquelme, Huang, Pennell, van Meurs, Ikram, Hughes, Tillin, Chaturvedi, Pausova, Paus, Spector, Kumari, Schalkwyk, Visscher, Davey Smith, Bock, Gaunt, Bell, Heijmans, Mill and Relton2021). Across the CYP genes, the frequency and location of DNAm sites with respect to the transcript exons are gene-specific (Figure 3). Seven out of the 10 assessed genes (CYP1A1, CYP2B6, CYP2C19, CYP2D6, CYP3A4, CYP3A5, and CYP4F2) have at least one DNAm site in the promoter region whereas only intronic and/or exonic DNAm sites were observed for CYP1A2 and CYP2C9.

Figure 3. Visualization of DNAm profiles for 10 CYP genes. This data was generated from public resources to demonstrate the wealth of epigenetic information available about important drug metabolism genes. Each box represents one CYP gene with the name and strand orientation. The exon/intron architecture is outlined and aligned to the position on their respective chromosomes. DNAm sites are drawn above (gray bars with yellow dots) relative to their location on the gene together with their average DNAm level (green bars). The height of the left black bar indicates a DNAm level of 1 (i.e., 100% methylated). DNAm sites very close to each other may appear as a single bar and for visualization purposes, DNAm sites distant to the gene body were omitted. DNAm site positions and DNAm level information are from the GoDMC resource (whole blood).
We focused on CYP-DNAm sites that are under genetic control with heritability ranging from <0.1% up to 97%. Genetic variants influencing these DNAm levels were found to be both rare (MAF < 5%) and very common (MAF > 40%). GoDMC shows that genetic variants influence 45% of the assessed DNAm sites. This suggests that CYP genes could harbor additional DNAm sites likely to be under environmental control. The DNAm data we are presenting here was derived from whole blood in European participants and differences in the liver, the most relevant tissue for CYP enzymes, as well as population-specific differences, are expected. While further research is needed to attribute DNAm sites to PGx effects and determine whether epigenetic marks are functional intermediates or consequences of differential gene activity, this qualitative assessment may help prioritizing candidate genes to conduct further research. For example, CYP2D6 metabolizes several common drugs and is polymorphic in epigenetic profiles in some populations (Qi et al., Reference Qi, Yin, Zhang and Wang2020). Understanding the epigenetic landscape of the gene, including which genes are under genetic control may allow scientists to better prioritize which of these sites to pursue with biological experiments.
Challenges in pharmacoepigenetics
Our current understanding of pharmacoepigenetics paired with available resources can generate hypotheses about interactions between epigenetics and classical nonepigenetic drug response. However, some of the information necessary to reconstruct or predict pharmacoepigenetic relationships is not yet available, as seen in the clozapine example.
Challenges in the field go beyond unavailable data. Epigenetic mechanisms are important for cancer progression, yet, studying pharmacoepigenetics in the context of cancer is challenging. In cancer cells, it is difficult to identify population-level genetic or epigenetic differences because the cancer epigenome is different from the host’s somatic epigenome and cancer cells are rapidly evolving. Furthermore, in the setting of active treatment, it is challenging to track which markers are basal to the patient tissue, are cancer-specific, or are a result of a pharmacological action. The tissue-specificity of epigenetic signals also means that obtaining disease-relevant samples from patients via blood draw is often not possible, limiting the current clinical applications of epigenetic profiles. Nevertheless, epigenetics marks affect cancer drug response and prognosis. Therefore, understanding these relationships remains an important area of research that is difficult to examine at the population and epigenome level of analysis.
Establishing causal relationships between changes in epigenetic state and a given phenotype is not only challenging in cancer. Environmental factors, disease states, and drugs can affect epigenetic markers, and likewise, marker levels may alter drug response and disease prognosis. Additionally, epigenetic states are dynamic and change throughout an individual’s lifetime. As such, determining the cause and consequence of observed associations between epigenetic markers, medical conditions, and drug responses is not always straight-forward. In some cases, twin studies are used to determine causality, but much remains to be uncovered regarding the directionality of the relationship between epigenetics and drug response (Bell and Spector, Reference Bell and Spector2012).
To navigate these complexities and disentangle observed associations, causal inference methods such as Mendelian randomization (MR) techniques can consolidate forward or reverse causality (Porcu et al., Reference Porcu, Sadler, Lepik, Auwerx, Wood, Weihs, Sleiman, Ribeiro, Bandinelli, Tanaka, Nauck, Völker, Delaneau, Metspalu, Teumer, Frayling, Santoni, Reymond and Kutalik2021a). MR makes use of genetic instrumental variables and has been successful in identifying putative causal relationships between gene expression and complex traits using eQTL and GWAS. Analogous application to mQTL data can reveal DNAm-to-trait and even DNAm-to-gene expression-to-trait pathways (Sadler et al., Reference Sadler, Auwerx, Lepik, Porcu and Kutalik2022; Figure 1). Equivalently, MR can be used to test whether altered methylation levels are responsible for observed PGx effects by leveraging PGx GWAS (Auwerx et al., Reference Auwerx, Sadler, Reymond and Kutalik2022). While EWASs of the PGx phenotype of interest can provide evidence of putative mechanisms, these results should be interpreted with caution considering the challenges associated with determining causality. Indeed, it was found that observed DNAm-to-trait and gene expression-to-trait correlations were more likely to arise due to reverse causality (i.e., trait-induced) (Min et al., Reference Min, Hemani, Hannon, Dekkers, Castillo-Fernandez, Luijk, Carnero-Montoro, Lawson, Burrows, Suderman, Bretherick, Richardson, Klughammer, Iotchkova, Sharp, Al Khleifat, Shatunov, Iacoangeli, McArdle, Ho, Kumar, Söderhäll, Soriano-Tárraga, Giralt-Steinhauer, Kazmi, Mason, McRae, Corcoran, Sugden, Kasela, Cardona, Day, Cugliari, Viberti, Guarrera, Lerro, Gupta, Bollepalli, Mandaviya, Zeng, Clarke, Walker, Schmoll, Czamara, Ruiz-Arenas, Rezwan, Marioni, Lin, Awaloff, Germain, Aïssi, Zwamborn, van Eijk, Dekker, van Dongen, Hottenga, Willemsen, Xu, Barturen, Català-Moll, Kerick, Wang, Melton, Elliott, Shin, Bernard, Yet, Smart, Gorrie-Stone, Shaw, Al Chalabi, Ring, Pershagen, Melén, Jiménez-Conde, Roquer, Lawlor, Wright, Martin, Montgomery, Moffitt, Poulton, Esko, Milani, Metspalu, Perry, Ong, Wareham, Matullo, Sacerdote, Panico, Caspi, Arseneault, Gagnon, Ollikainen, Kaprio, Felix, Rivadeneira, Tiemeier, van IJzendoorn, Uitterlinden, Jaddoe, Haley, McIntosh, Evans, Murray, Räikkönen, Lahti, Nohr, Sørensen, Hansen, Morgen, Binder, Lucae, Gonzalez, Bustamante, Sunyer, Holloway, Karmaus, Zhang, Deary, Wray, Starr, Beekman, van Heemst, Slagboom, Morange, Trégouët, Veldink, Davies, de Geus, Boomsma, Vonk, Brunekreef, Koppelman, Alarcón-Riquelme, Huang, Pennell, van Meurs, Ikram, Hughes, Tillin, Chaturvedi, Pausova, Paus, Spector, Kumari, Schalkwyk, Visscher, Davey Smith, Bock, Gaunt, Bell, Heijmans, Mill and Relton2021; Porcu et al., Reference Porcu, Sadler, Lepik, Auwerx, Wood, Weihs, Sleiman, Ribeiro, Bandinelli, Tanaka, Nauck, Völker, Delaneau, Metspalu, Teumer, Frayling, Santoni, Reymond and Kutalik2021a).
As more population-level epigenetic data emerges, there is an opportunity to elucidate potential pharmacoepigenetic interactions from publicly available data by (1) identifying pharmacogenes with unexplained variability, (2) locating key epigenetic biomarkers near the gene or regulatory region, and (3) referencing epigenetic databases to hypothesize relationships (such as those outlined in Figure 1) between biomarkers like DNAm and phenotypes such as drug response. It will be critical to validate such predicted interactions via biological and clinical experiments to generate a deeper understanding of epigenetic effects and drug response. While there is a great deal of information already available, more is required to fully examine pharmacoepigenetic influences on drug response. Additional pharmacoepigenetic studies on classical nonepigenetic drugs both in vitro and with patient populations would help address these gaps. Employing novel computational approaches to identify or predict pharmacoepigenetic relationships paired with biological validation allows us to fully materialize the promise of pharmacoepigenetics as a powerful tool for understanding biological mechanisms and developing effective interventions.
Open peer review
To view the open peer review materials for this article, please visit http://doi.org/10.1017/pcm.2023.6.
Supplementary material
The supplementary material for this article can be found at https://doi.org/10.1017/pcm.2023.6.
Financial support
D.A.S. was supported by the Stanford Biochemistry Department, the NSF Graduate Research Fellowship Program ID: 2019286895. M.C.S. was supported by the Swiss National Science Foundation (310030-189147) and would like to thank the Fulbright Program for funding her research stay at Stanford University. R.B.A. was supported by NIH GM102365 HG010615 and the Chan-Zuckerberg Biohub.
Competing interest
The authors have no conflict of interest to disclose.
Comments
No accompanying comment.