Cognitive impairment post-stroke can result in significant physical and psychological consequences for the individual. Stroke is one of the leading causes of adult disability, and in the past decade, hospitalization due to stroke-related cognitive impairment has increased by 35%.1 However, as many as 77% of individuals with stroke-related cognitive impairment may go undiagnosed by cognitive screening measures.Reference Chan, Khan, Oliver, Gill, Werring and Cipolotti2 The Montreal Cognitive Assessment (MoCA)Reference Nasreddine, Phillips and BÃcdirian3 is a widely used cognitive screening measure and has been recommended by the National Institute of Neurological Disorders and Stroke-Canadian Stroke Network for use with stroke populations.Reference Hachinski, Iadecola and Petersen4 Although cognitive impairment varies depending on stroke location and severity, several studies have found that individuals with cerebrovascular conditions are most likely to be impaired on MoCA items involving executive functions, fluency, recall, and attention.Reference Price, Jefferson, Merino, Heilman and Libon5–Reference Pendlebury, Markwick, Jager, Zamboni, Wilcock and Rothwell7
Despite the MoCA’s demonstrated use for cerebrovascular conditions, it lacks a processing speed metric, a function often affected in stroke populations.Reference Cees De Groot, De Leeuw and Oudkerk8,Reference Pendlebury, Cuthbertson, Welch, Mehta and Rothwell9 Speed of information processing can be easily assessed by tests that can be administered in under 2 minutes. The Symbol-Digit Modalities Test (SDMT)Reference Smith10 is a widely used test of processing speed that requires visual scanning, psychomotor speed, attention, and learning. Dong and colleaguesReference Dong, Slavin and Chan11 demonstrated that when the MoCA was supplemented with the SDMT, it improved accuracy for vascular cognitive impairment (VCI) screening.
Traditionally, a single cut point of 26 is used on the MoCA to form dichotomous classifications of impaired or not impaired.Reference Nasreddine, Phillips and BÃcdirian3 An alternative methodReference Nakas, Alonzo and Yiannoutsos12–Reference Swartz, Cayley and Lanctôt14 uses two cut points to classify individuals as low, indeterminate, or high probability of cognitive impairment. This allows for greater certainty of those classified as low or high probability of impairment, but leaves a subgroup whose classification is unknown and requires further assessment. Indeed, in the vascular sample studied by Swartz and colleaguesReference Swartz, Cayley and Lanctôt14, a sizeable 62% scored in this indeterminate range.
The Present Study
In the present study, we sought to confirm and expand on previous findings of the clinical utility of the MoCA in detecting cognitive impairment using a diagnostic accuracy study design. First, we aimed to validate Swartz and colleagues’Reference Swartz, Cayley and Lanctôt14 three-category classification approach against a gold-standard neuropsychological assessment in a different sample of vascular pathology, post-acute ischemic stroke. A detailed comprehensive neuropsychological battery with standardized tests was used to assess and characterize cognitive impairment.Reference Nasreddine, Phillips and BÃcdirian3,Reference Hachinski, Iadecola and Petersen4,Reference McLaughlin, Sunderland and Beaton17 Second, to improve diagnostic classification and reduce the number of people in the indeterminate category, we conducted two additional analyses. First, based on the literature showing specific deficits in executive function and processing speed in vascular pathology,Reference Price, Jefferson, Merino, Heilman and Libon5–Reference Pendlebury, Markwick, Jager, Zamboni, Wilcock and Rothwell7 we examined whether subdomain scores on the MoCA would provide additional predictive utility over and above the total score. Second, we included the SDMT with the MoCA to determine whether adding a simple, rapidly acquired measure of processing speed improved predictive utility. Screening conducted in the acute phase can result in reversible cognitive impairment,Reference Sivakumar, Kate, Jeerakathil, Camicioli, Buck and Butcher15 as such, we sought to examine cognition at a more stable time point post-stroke.
Method
Participants
This study was approved by the Research Ethics Boards at Baycrest Health Sciences and at York University. Participants were part of the Ontario Neurodegenerative Disease Research Initiative (ONDRI), a longitudinal, multidisciplinary research study investigating common profiles among five neurodegenerative conditions.Reference Farhan, Bartha and Black16 The cerebrovascular disease (CVD) cohort was used in the present cross-sectional study. All participants completed various assessment platforms including genomics, neuroimaging, ocular function, and gait and balance, as well as neuropsychological testing. Demographic, clinical, and neuropsychological data obtained from the baseline assessment were used for this study. All participants were administered the MoCA as part of the screening procedure, and the neuropsychological battery (including the SDMT) was administered within 8 weeks of initial screening with the MoCA. If the participant had recently completed the MoCA for clinical purposes, efforts were made to administer alternate forms. Post-data collection, MoCA data underwent a rigorous cleaning and curation process that was overseen by a clinical neuropsychologist. Cleaning ensured that values were in range and that items were scored consistently. A working group of four individuals (including three neuropsychologists) highly familiar with the MoCA was established to reach a consensus and resolve any inconsistencies in scoring that required subjective judgment (i.e. Abstraction, Verbal Fluency). Neuropsychology data underwent rigorous monitoring and preprocessing.Reference McLaughlin, Sunderland and Beaton17 All ONDRI data underwent a data evaluation procedure using multivariate outlier detection to identify anomalous observations, providing guidance on additional areas of possible error.Reference Sunderland, Beaton and Fraser18
All participants provided informed consent and met extensive eligibility criteria for the larger ONDRI study.Reference Farhan, Bartha and Black16 Participants in the CVD cohort were post-acute ≥ 3 months and also met the following inclusion criteria: (a) proficient in speaking and understanding English, with self-ratings of 7 or more (corresponding to “good”) for both speaking and understanding English on the Language Experience and Proficiency Questionnaire,Reference Marian, Blumenfeld and Kaushanskaya19 (b) 8 or more years of formal education, (c) post-acute ischemic stroke or silent stroke that was documented on MRI or CT, (d) mild-moderate stroke severity defined by scores of 0–3 on the modified Rankin Scale1 (MRS),Reference Rankin20 and (e) a MoCA score of at least 18. Exclusion criteria included a history of dementia prior to the stroke, large cortical strokes, severe cognitive impairment, aphasia, inability to write, or severe functional disability limiting ability to perform the assessment. Data versions 2018SEP18 and 2018AUG17 were used for this study.
Participant Characteristics
Participant characteristics are provided in Table 1. There were 161 participants with 32% women and 68% men. Participants’ age ranged from 54 to 85 years and were in their late 60s on average and had some university education (M = 14.6 years).
Table 1. Participant Characteristics (n = 161)

Note. MoCA = Montreal Cognitive Assessment; NIHSS = National Institutes of Health Stroke Scale; NP impairment = impaired status on neuropsychological battery; QIDS-SR = Quick Inventory of Depressive Symptoms - Self Report.
Assessments
MoCA
The MoCA is a screening tool that assesses multiple cognitive domains and is completed in under 10 minutes.Reference Julayanont and Nasreddine21 Individuals with ≤ 12 years of education are given an additional point up to a maximum score of 30.Reference Nasreddine, Phillips and BÃcdirian3
Symbol-Digit Modalities Test
The SDMT is a measure of processing speed and incidental learning.Reference Smith10 Participants are shown a key at the top of the page that pairs nine symbols with corresponding digits (1–9). They are then presented with rows of just symbols below for which they use the key to write the corresponding digit. The score is the total number of items filled in correctly in 90 seconds (max = 110). Uchiyama and colleaguesReference Uchiyama, Delia and Dellinger22 added an incidental learning component to the test featuring 15 symbols in which all 9 original symbols are included at least once. Participants are asked to fill in the number associated with each symbol from memory, without the assistance of the key, immediately following the coding trial. The score is the number of items recalled correctly (max = 15). If a symbol is presented more than once and the participant responds correctly on one trial and incorrectly on another, they receive a point for the correct trial. Overall, two scores are obtained for the SDMT: coding and learning. For those with a physical impairment potentially impacting performance (e.g., tremor, paralysis, weakness, or injury), an oral version of the test is administered. Eight individuals (5%) were administered the oral version of the SDMT, and the remaining 153 (95%) completed the written version.
Neuropsychological Assessment
The neuropsychological assessment consisted of a standardized battery administered to all participants. For the purpose of characterizing areas of cognitive impairment in individual participants, the tests were categorized into five cognitive domains based on a principle component analysis as shown in Table 2. Test scores were normalized based on age and/or education using published norms and converted to standardized scores (z-scores, t-scores, scaled scores, and/or percentiles), in most cases. Participants were deemed cognitively impaired on the neuropsychological battery if they obtained a standardized score that was lower than 1.5 standard deviations (SDs) below the normative mean on at least two test scores within one or more domains.Reference Jak, Bondi and Delano-Wood23 On two tests (SDMT incidental learning; Semantic Probe), normative data were not available, so we derived cutoff scores based on the available data while taking into consideration the test designs and estimated false-positive rates. To minimize fatigue, participants were offered breaks at scheduled intervals (approximately every 30–45 minutes of testing). Participants were permitted to take rest breaks as needed throughout the test session. In addition, participants were required to take a substantial break (i.e., at least 30 minutes) if testing continued for more than 3 hours. If any participant was unable to finish the testing in one session, additional sessions were scheduled on subsequent days.Reference McLaughlin, Sunderland and Beaton17
Table 2. Neuropsychological Battery

Note. DKEFS = Delis-Kaplan Executive Functions System; WASI-II = Wechsler Abbreviated Scale of Intelligence – II.
Statistical Analyses
Multiple linear regression analyses were performed to calculate the effects of age, education, sex, and depression on MoCA scores. To determine diagnostic accuracy, we identified individuals with a positive MoCA result (i.e., an impaired score, < 26) versus a negative result (i.e., a normal score, ≥ 26) and compared this to their performance on the neuropsychological tests. We then used receiver operating characteristic (ROC) curve analyses to maximize sensitivity and specificity to a) determine an optimal single cut point and b) to determine two cut points: the lowest value that achieved at least 90% sensitivity and the highest value that achieved at least 90% specificity. Participants scoring below the high sensitivity cut point were classified as high probability for cognitive impairment, and participants scoring above the high specificity cut point were classified as low probability. Participants scoring between the two cut points were classified as indeterminate or unknown probability of cognitive impairment. Positive and negative likelihood ratios, predictive values, and overall classification accuracies were calculated for all cut point analyses.
To improve classification for the indeterminate group, a second analysis was conducted using discriminant function analysis.Reference James, Witten, Hastie and Tibshirani24,Reference Brown, Wicker, Tinsley and Brown25 A predictive model was built to correctly classify participants as impaired or not impaired as determined by their performance on the neuropsychological battery. We examined two sets of variables to create two separate discriminant functions. First, we examined the predictive value of distinct MoCA subdomain items, rather than the total MoCA score, to determine if the distributed weighting of subdomains would better predict group membership relative to the coarser single score. Second, we examined the utility of the SDMT, paired with the total MoCA score, to determine whether the additional metrics of processing speed and incidental learning would improve diagnostic accuracy relative to the MoCA total score alone. Participants from the high and low probability of cognitive impairment groups were used first as the learning data to develop the discriminant functions, and then the models were tested on the indeterminate group. The cross-validated classification method was used to indicate the final number of cases correctly identified by each function. This method employs the leave-one-out technique in which one case is systematically held out and the discriminant analysis is performed on the remaining sample. Then, the excluded case is classified into one of the groups based on the discriminant function and the procedure is repeated on each case of the sample until all cases are classified. This results in a more conservative estimate of the number of cases correctly identified by the function.Reference Brown, Wicker, Tinsley and Brown25 All statistical analyses were conducted in SPSS Version 22.0.
Results
Neuropsychological Data
Of the 161 participants, 95 (59%) met the criteria for cognitive impairment (≥ 1.5 SD below the normative mean on ≥ two test scores in at least one domain) on the neuropsychological battery. Forty-six participants (29%) were impaired in two or more cognitive domains, and 6 (4%) were impaired in three or more domains.
MoCA Performance
The mean MoCA score was 25.2 (3.0), with 75 (47%) participants scoring below the traditional cut point of 26 and 86 (53%) participants scoring at or above 26. In a multiple regression model (R 2 = .069, p < .001) with age, sex, years of education, and depression as main effects, only age was a significant predictor of MoCA scores, where older participants (β = −0.106, p < .001) had lower MoCA scores. Sex (β = .068, p = .891), years of education (β = .093, p = .243), and depression scores (β = −.212, p = .596) did not significantly predict MoCA scores. Table 3 presents a count of the number of participants who were cognitively impaired or intact based on the neuropsychological battery as a function of MoCA score.
Table 3. Number of Participants Impaired vs. Not Impaired on the Neuropsychology Battery as a Function of MoCA Score

Note. MoCA = Montreal Cognitive Assessment.
Next, we examined neuropsychological performance of the MoCA-negative (≥ 26) and MoCA-positive (≤ 25) groups. Of the 86 individuals in the MoCA-negative group, 34 (40%) were impaired in at least one neuropsychological domain. Regarding the areas of impairment, 26 (30%) participants demonstrated impaired speed, 19 (22%) had impaired memory, 2 (2%) had impaired visual spatial processing, 1 (1%) had impaired language, and 1 (1%) had impaired attention. Of these 34 who were impaired on at least one domain, 12 (35%) were impaired in two or more domains, and 2 (17%) of those 12 participants were impaired in three or more domains on the neuropsychological battery.
Of the 75 individuals in the MoCA-positive (≤ 25) group, 61 (81%) were impaired in at least one of the neuropsychological domains. Thirty-nine (52%) were impaired in speed, 49 (65%) were impaired in memory, 10 (13%) were impaired in language, 1 (1%) was impaired in visual spatial, and no individual was impaired in the attention domain. Of these 75 individuals, 34 (45%) were impaired in two or more domains, and 4 (12%) of those 34 were impaired in three or more domains.
Diagnostic Accuracy Analysis
Using the traditional cut point of 26, the MoCA’s sensitivity was 64% and specificity was 79% in the present sample. Our ROC analysis indicated that the optimal single cut point, which maximized sensitivity and specificity, was 27. The optimal two cut points were 27 (to maximize sensitivity at 92%) and 24 (to maximize specificity at 91%). All results involving cut points are summarized in Table 4. Using two cut points, 27% of participants scored ≤ 23 and were classified as high probability for cognitive impairment, and 24% of participants scored ≥ 28 and were classified as low probability for cognitive impairment. The remaining 48% of participants scored in the intermediate range of 24 to 27 and were classified as indeterminate probability for cognitive impairment. Figure 1 displays the proportion of individuals in each category and their cognitive status based on the neuropsychological assessment.
Table 4. Diagnostic Accuracy Analysis and Optimal Cut Points
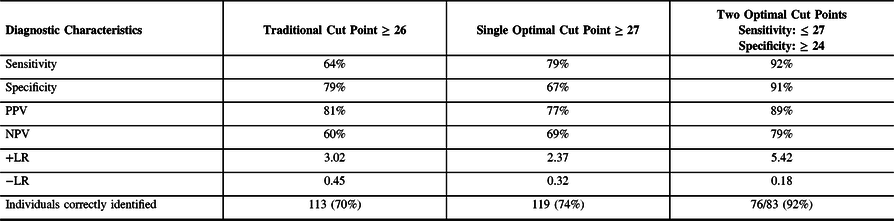
Note. +LR = positive likelihood ratio; −LR = negative likelihood ratio; NPV = negative predictive value; PPV = positive predictive value.
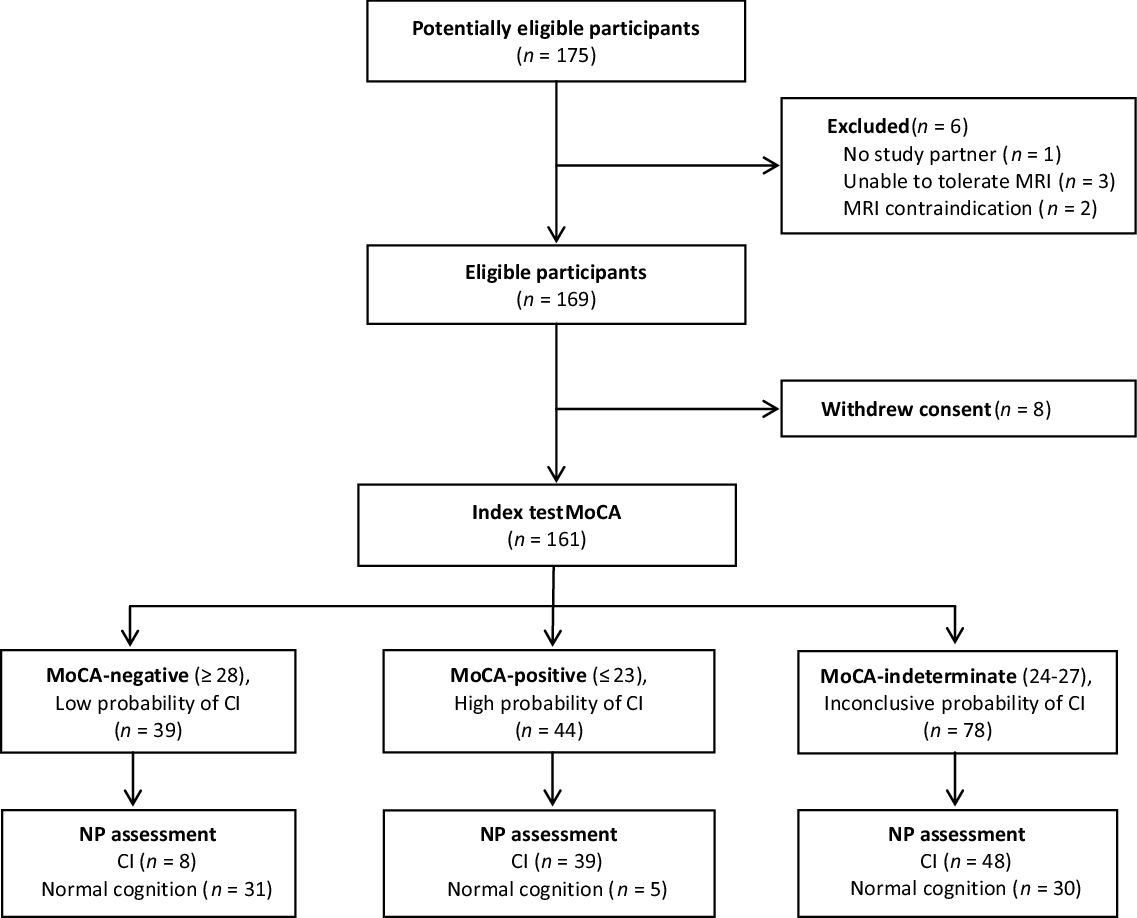
Figure 1: Flow Diagram of Participant Classification by the MoCA (Index) and Neuropsychological Assessment (Reference).
Discriminant Function Analysis
MoCA subdomain scores
Eight MoCA variables were created by grouping scorable items into their respective cognitive domains, including attention (10 points), immediate recall (10 points), delayed recall (15 points), visual construction (5 points), abstraction (2 points), fluency (25 points), object naming (3 points), and orientation (6 points). With participants from the high and low probability of cognitive impairment groups, the discriminant function using MoCA variables was significant, Λ = .57, χ 2(8) = 43.67, p < .001, accounting for 43% of between-group variability. The structure matrix correlation coefficients reveal the strength of each variable in the model with the function. Items of attention (r = .61), immediate recall (r = .34), delayed recall (r = .55), language fluency (r = .53), visual construction (r = .48), abstraction (r = .45), and orientation (r = .30) were significant group predictors, whereas object naming was not, r < .30.Reference Brown, Wicker, Tinsley and Brown25 The cross-validated classification showed that overall, 65 of 83 (78%) cases in the high and low groups were correctly identified based on the predictors in the model. Next, in order to test the predictive utility of the model, we conducted a discriminant function analysis on the indeterminate MoCA group alone. The discriminant function for the indeterminate group was not significant, Λ = .87, χ 2 (8) = 10.15, p = .254, and accounted for 13% of between-group variability.
Symbol-Digit Modalities Test
The SDMT was removed from the neuropsychological battery before conducting these analyses to avoid issues of circularity. There were 33 (21%) individuals who were impaired on the SDMT in our full sample, and two participants no longer met criteria for cognitive impairment on the neuropsychological battery when the SDMT was removed.
We entered two subscores from the SDMT in the discriminant function analysis, a coding subscore (normative-based z-score) and a learning subscore (raw total score). Using the high and low probability of cognitive impairment groups, the discriminant function was significant, Λ = .44, χ 2 (3) = 65.01, p < .001, accounting for 56% of between-group variability. Analysis of the structure matrix scores revealed that the MoCA total score (r = .88), SDMT coding score (r = .62), and SDMT learning score (r = .52) were all significant group predictors. The cross-validated classification showed that overall, 69 of 83 (83%) cases were correctly identified. Next, we tested this model for the indeterminate group alone. This discriminant function analysis was also significant, Λ = .88, χ 2 (3) = 9.62, p < .022, accounting for 12% of between-group variability. Analysis of the structure matrix revealed that the SDMT coding score, SDMT learning score, and the MoCA total score were all significant predictors of group membership for the indeterminate group, rs = .89, .62, and .51, respectively. The cross-validated classification showed that overall, 51 of 78 (65%) cases were correctly identified for this group. Thus, across the entire sample, 127/161 (79%) cases were correctly identified as determined by performance on the larger neuropsychological battery.
Discussion
The present study evaluated the clinical utility of the MoCA by using a three-category approach to maximize classification for cognitive impairment and combining it with the SDMT. First, we tested two cut points that maximize the MoCA’s sensitivity and specificity to higher than 90% for post-acute stroke individuals. This means that the cognitive status determined by performance on the neuropsychological battery was correctly identified by using the MoCA total score for more than 90% of individuals within the low and high probability of cognitive impairment groups. However, we were still uncertain of the probability of cognitive impairment for almost half of our sample in the intermediate group. We found that pairing the SDMT, a brief test of processing speed, with the MoCA improved classification for the indeterminate group, such that most cases in that group (65%) were correctly identified. Examining individual subdomains of the MoCA did not significantly improve its ability to discriminate cognitive impairment for the indeterminate group.
Our findings confirm and extend those of Swartz and colleagues,Reference Swartz, Cayley and Lanctôt14 who reported similar cut points (23 and 27) and a large indeterminate group in a sample of 400 individuals from a stroke clinic. The slightly different samples in the two studies (post-acute stroke vs. individuals referred to a stroke prevention clinic) suggest that the cut points are generalizable, at least within populations with vascular-related concerns. We expand on these previous findings by demonstrating improved diagnostic accuracy when adding a measure of processing speed to the MoCA.
Processing speed is affected in many neurological disordersReference Cees De Groot, De Leeuw and Oudkerk8,Reference Lezak27 and has been identified as a possible reason for the MoCA’s reduced sensitivity for vascular-related cognitive impairment.Reference Chan, Khan, Oliver, Gill, Werring and Cipolotti2,Reference Pendlebury, Markwick, Jager, Zamboni, Wilcock and Rothwell7 The addition of the SDMT to the MoCA directly addresses this issue by including a domain likely to be impaired in this population. This approach was first demonstrated by Dong and colleaguesReference Dong, Slavin and Chan11 who demonstrated improved accuracy of VCI screening by supplementing the MoCA with the SDMT. Our study further refines this approach by introducing a staged method whereby the SDMT is administered to individuals scoring in the intermediate range on the MoCA (24–27) and whose cognitive status is therefore not certain after assessment with the MoCA alone. The SDMT also measures other cognitive domains in addition to processing speed, including divided attention, learning, working memory and complex visual scanning.Reference Smith10,Reference Lezak27 Because the test uses both verbal and nonverbal materials, the SDMT incidental learning trial may be even more sensitive to subtle memory problems than other memory measures utilizing a single kind of material. Although some studies have reported impaired verbal and nonverbal learning in vascular dementia,Reference Sachdev, Brodaty and Valenzuela28 the use of the incidental learning trial has not been implemented in other studies using the SDMT.Reference Pendlebury, Markwick, Jager, Zamboni, Wilcock and Rothwell7,Reference Dong, Slavin and Chan11,Reference Sachdev, Brodaty and Valenzuela28
There are a number of clinical implications of our findings. Where clinical resources are limited and to avoid unnecessary referrals, employing an additional 90-second processing speed measure such as the SDMT can increase confidence in detecting cognitive impairment post-stroke. This approach is both practical and efficient as an addition to a screening test. When patients score in the intermediate range (24–27) on the MoCA and score more than 1.5 SDs below normative data on the SDMT coding, they are highly likely to have cognitive impairment and do not require further testing. The SDMT is an easily administered and quickly scored test with age-and education-corrected norms that are readily available and provide sensitive information that can be reliably used to triage patients. To optimize the use of the SDMT incidental learning component in this manner, there is a need for age- and education-corrected norms. Ideally when there are concerns about cognition, testing should occur after the acute phase, which is typically 3 months post-stroke, when cognition has stabilized and is less likely to fluctuate.Reference Sivakumar, Kate, Jeerakathil, Camicioli, Buck and Butcher15 The screen can be conducted in either specialized stroke clinics, general practitioner’s office, or any setting where individuals who have had a stroke in the past (> 3 months) are now concerned about cognitive complaints and need to be triaged for more specialized care. In a clinical setting, false positives may pose a costly outcome when busy clinics and expensive treatments are used for individuals who do not need them. Similarly, false negatives can be detrimental in providing appropriate treatment for those who most need it. As such, the three-category approach to identify individuals with and without cognitive impairment directly addresses these issues by effectively triaging individuals who are at low, indeterminate, and high probability of cognitive impairment.
There are some caveats to our design that should be noted. First, participants may have been higher functioning than those typically seen in the general clinic by virtue of the study requirements: they had to have sufficient motor, language, hearing, and visual functioning to complete neuropsychological testing. Similarly, only individuals who scored ≥ 18 on the MoCA were included, and as such we were unable to test diagnostic accuracy across the full range of cognition post-stroke. Additionally, we used a definition of cognitive impairment that includes those with even mild impairment, namely, ≥ 1.5 SD on two or more tests in a single domain. Thus, there would be a higher prevalence of cognitive impairment in our sample than in other studies that use a more conservative definition (i.e., ≥ 2 SD). Lastly, the cognitive domains used in our definition of cognitive impairment were based on a principal components analysis conducted on this sample, which restricts the generalizability of the results to other diseases.
Conclusions
The MoCA’s sensitivity and specificity are maximized to higher than 90% by using two cut points to stratify post-stroke individuals into groups of low, indeterminate, and high probability of cognitive impairment. This is a practical and efficient method to increase certainty of classification for the indeterminate group while also expanding the domains assessed by pairing the MoCA with the SDMT. This process has the potential to improve the efficiency of limited and expensive resources, which is an important goal within the healthcare system.
Acknowledgments
This work was completed on behalf of the Ontario Neurodegenerative Disease Research Initiative (ONDRI). The authors would like to acknowledge the ONDRI Founding Authors: Robert Bartha, Sandra E. Black, Michael Borrie, Dale Corbett, Elizabeth Finger, Morris Freedman, Barry Greenberg, David A. Grimes, Robert A. Hegele, Chris Hudson, Anthony E. Lang, Mario Masellis, William E. McIlroy, Manuel Montero-Odasso, David G. Munoz, Douglas P. Munoz, J.B. Orange, Michael J. Strong, Stephen C. Strother, Sean Symons, Maria Carmela Tartaglia, and Lorne Zinman.
Sources of Funding
This research was conducted with the support of the Ontario Brain Institute, an independent non-profit corporation, funded partially by the Ontario government. The opinions, results, and conclusions are those of the authors and no endorsement by the Ontario Brain Institute is intended or should be inferred. RHS receives personal support for research from a Clinician-Scientist Phase II Award from Heart & Stroke and by the Department of Medicine at Sunnybrook HSC.
Statement of Authorship
KZ, AKT, JBR, RHS, MAB, and LT conceived and designed the study. KZ, AKT, JBR, KMS, and DB analyzed and interpreted the data. KZ drafted the manuscript and AKT, JBR, RHS, KMS, MAB, LT, PMM, DK, and DB revised the manuscript for intellectual content. BP, BL, DJS, DD, AH, JM, and The ONDRI Investigators contributed to the acquisition of data. All authors approved the manuscript.
Disclosures
None.