1. Introduction
In this keynote, we introduce a blueprint of a representation-based model of individual lexical units and their development, which we call the Ontogenesis Model of the L2 Lexical Representation (OM). Since we do not assume that the native and nonnative representationFootnote 1 differ in their basic dimensions, the core of the model can also account for L1 lexical representations and their ontogenesis.
The OM attempts to encompass the multifaceted character of individual lexical representations and is based primarily on the synthesis of current findings on lexical processing and vocabulary training. It subsumes several dimensions of lexical encoding and describes their ontogenetic development, which starts with their emergence and ends – optionally – with their being forgotten. Lexical representations dynamically move along these ontogenetic curves. At its optimum, the representation is properly encoded and fully specified. We propose that while most L1 representations reach their optima and stay around it, most L2 lexical representations do not reach their optima (i.e., stay fuzzy) – at least in some dimensions – and move more dynamically along the ontogenetic curve (cf. the dynamic character of the interlanguage and the general experience of learners in using or not using (up to forgetting) their non-native languages).
As its name indicates, the model has two crucial properties. First, the OM addresses primarily the properties and aspects of the L2 lexical units and their acquisition. Thus, while most existing models, such as the Distributed Feature Model, BIA+, or Multilink focus on the relationship and interactions between the L1 and L2 and can be best described as models of the bilingual lexicon, the OM is best described as a model of the nonnative lexicon (which can also be adapted as a model of a native lexicon). It is concerned with lexical entries that contain an L2-specific component (typically, at least an L2 word form) and relations between them.Footnote 2 The OM thus focuses on the L2 dimension of the bilingual models and addresses L2 representations from a more L2-centered perspective.
Second, in contrast to the above-mentioned models, the OM also seeks to capture the developmental aspects of L2 representations, starting with their emergence and optionally continuing towards their optimum and/or attrition and forgetting. While much research has been devoted to the development of proficiency in L2 speakers, to our knowledge there is no comprehensive model that would focus entirely on the development of individual L2 representations and their components as the OM does. Memory-based approaches that to some degree attempt this step, such as the Complementary Learning Systems (CLS) hypothesis, adapted for L2 by Lindsay and Gaskell (Reference Lindsay and Gaskell2010), or the Episodic L2 hypothesis by Forster and colleagues (Forster & Jiang, Reference Forster and Jiang2001; Forster & Witzel, Reference Forster and Witzel2012) are not concerned with the internal structure of lexical representations or with the interaction between their components, and often concentrate either on their form or meaning. Other models address the development of particular aspects of L2 lexical representations. For example, the Second Language Linguistic Perception (L2LP) model (Escudero, Reference Escudero2009; Yazawa, Whang, Kondo & Escudero, Reference Yazawa, Whang, Kondo and Escudero2020) explores the acquisition of phonologically contrastive sounds in L2 by modelling differential sensitivity to phonetic cues in L1 and L2 speakers. Connectionist approaches focus on computational modelling of the processing mechanisms underlying learning, but leave aside the parameters of the learner and the acquisitional context. The OM is essentially interconnectable with other perspectives and can serve as a reference model in language instruction.
The OM is multidimensional. It has several components described in detail below (dimension of linguistic domains, dimension of mappings, and dimension of networks) and illustrates how they interact and relate to each other. In general terms, the model is inspired by the research that explores the “depth of vocabulary knowledge” (Nation, Reference Nation2001; Read, Reference Read, Bogaards and Laufer2004) in that it also focuses on the acquisition of various properties of lexical representations. However, while sharing such an integrative approach to lexical representations, the OM is based primarily on evidence from online methods and thereby captures the dynamic interaction of the lexical representation's components during processing. While the research employing these methods enables more direct and precise insights into the structure of lexical entries and their processing, it typically targets only singular aspects of lexical representations. One of the OM's purposes is to offer a synthesizing framework that brings these findings together and places them in a context that helps to understand and explain their relations and how they affect each other during their development.
One of the crucial concepts of the OM is fuzziness, which is viewed as a central property of the L2 lexicon. Fuzziness refers to inexact or ambiguous encoding of different components or dimensions of the lexical representation that can be caused by several linguistic, cognitive, and learning-induced factors. These factors include, among others, changes in neural plasticity, the complexity of mapping L2 semantic representations on the existing L1 semantic representations and of mapping L2 forms on the semantic representations, and problems with L2 phonological encoding. Fuzziness in different components of lexical encoding also interacts with input frequency, leading to different outcomes, as exemplified in the OM. The OM illustrates how fuzziness accounts for various effects reported in the previous research that had not been theoretically interconnected, such as phonological categorization difficulties leading to problems with word recognition (Darcy, Dekydtspotter, Sprouse, Glover, Kaden, McGuire & Scott, Reference Darcy, Dekydtspotter, Sprouse, Glover, Kaden, McGuire and Scott2012; Darcy, Daidone & Kojima, Reference Darcy, Daidone and Kojima2013), lexical confusions (Cook & Gor, Reference Cook and Gor2015), reliance on sublexical processing in word recognition (Gor & Cook, Reference Gor and Cook2020), incorrect or weak form-meaning mappings (Cook, Pandža, Lancaster & Gor, Reference Cook, Pandža, Lancaster and Gor2016; Ota, Hartsuiker & Haywood, Reference Ota, Hartsuiker and Haywood2009), engagement of new representations in a semantic network (Bordag, Kirschenbaum, Tschirner & Opitz, Reference Bordag, Kirschenbaum, Tschirner and Opitz2015; Bordag, Kirschenbaum, Rogahn, Opitz & Tschirner, Reference Bordag, Kirschenbaum, Rogahn, Opitz and Tschirner2017a; Bordag, Opitz, Rogahn & Tschirner, Reference Bordag, Opitz, Rogahn and Tschirner2018), or comprehension difficulties in L1 reading (Perfetti, Reference Perfetti2007). The OM relates fuzziness to the concept of the optimum, which refers to the ultimate attainment of a representation (or its individual components), i.e., the highest level of its acquisition, when the representation is properly encoded and no longer fuzzy. The optimum represents a range of settings in different aspects of lexical encoding rather than a fixed unique value (see below).
In the blueprint of the model, we focus primarily on the initial stages of acquisition in the phonological, orthographic, and semantic domains and the corresponding mappings and networks, while the empirical evidence for the later acquisition stages is covered in less detail.Footnote 3 Although we address L2-specific characteristics of L2 representations, we also refer to L1 evidence where appropriate, especially when L2 evidence is minimal. Overall, the OM aims to provide a consolidated framework for more or less disconnected research strands that is informed by and consistent with existing empirical evidence. We hope that the model will offer a basis for future studies by making it possible to pinpoint, more exactly, which ontogenetic aspect of lexical properties they are targeting and how the other properties are – or are not – affected by it. This will help to establish how the status of the lexical representation in the model's space changes through its development or a particular treatment, and which methods are successful in addressing individual aspects of lexical ontogenesis.
In the following, we first give an overview of the whole model, including the figures that illustrate it. The subsequent sections address the individual aspects of the model and discuss the corresponding evidence.
1.1 Model overview
The first dimension of the model is the dimension of linguistic domains. The domains correspond to the various types of word knowledge that need to be represented. What types of linguistic knowledge are encoded in the word is a question that prompts different answers by various researchers. Most refer to semantic, phonological, and orthographic knowledge (cf. also BIA+). Grammatical and morphological knowledge are sometimes mentioned as well, either subsumed under the semantic or the form category (cf. Perfetti, Reference Perfetti2007 or Nation, Reference Nation2001), or listed separately (Read, Reference Read, Bogaards and Laufer2004; Caramazza, Reference Caramazza1997). In the current OM version, we focus on the semantic, phonological, and orthographic components of the lexical representations. In the model, each domain is represented by its own ontogenetic curve that captures its degree (or the depth) of acquisition: in other words, its quality. The onset of acquisition may not be the same for all domains, since e.g., the emergence of an orthographic representation may follow the emergence of a phonological representation. Figure 1 shows how linguistics domains are modelled in the OM.
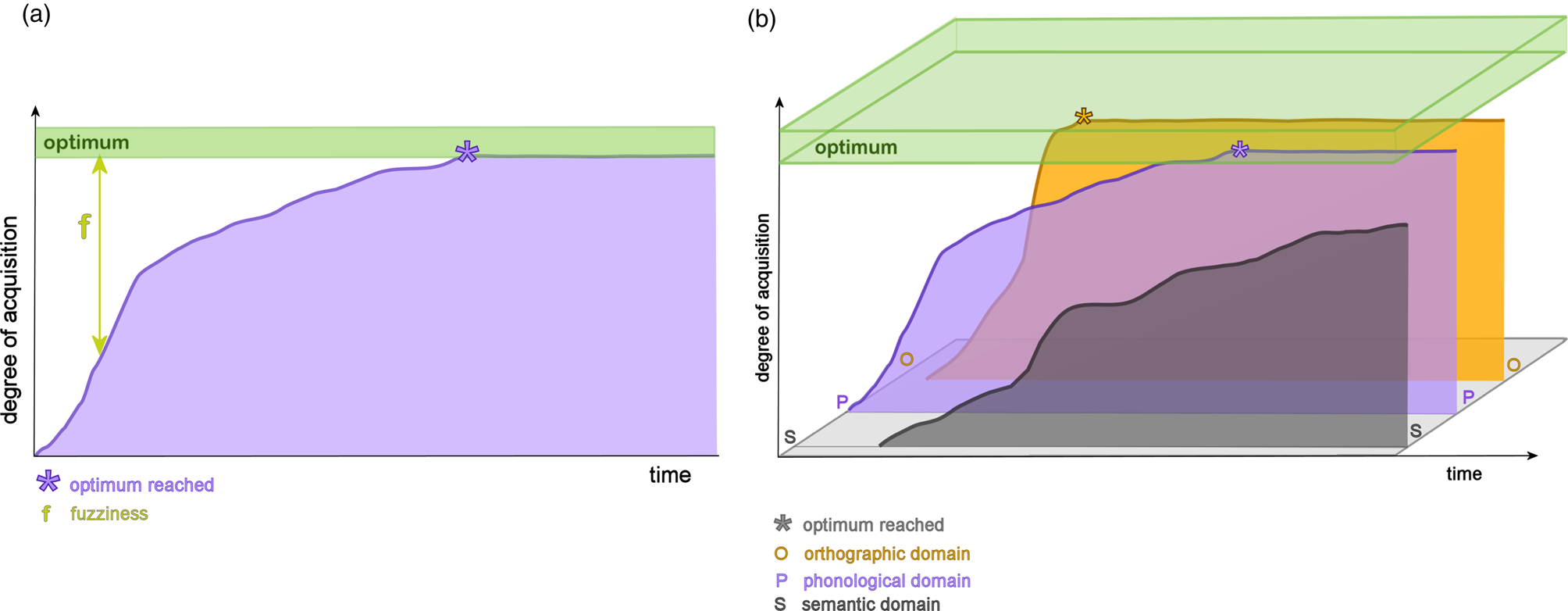
Figure 1 Figure 1a depicts an example ontogenetic curve in one domain. Over time, the degree of acquisition increases while, simultaneously, the degree of fuzziness decreases till the optimum range (shaded green) is reached (asterisks, meeting the optimum's lower bound).
Figure 1b shows the ontogenetic curves of all three domains in a three-dimensional graph (semantic in front, phonological and orthographic behind). Domains may have different onsets (here, the emergence of the phonological representation starts before the orthographic and semantic representations), different slopes (here the orthographic representation has a steeper slope) and that they may (here: phonological and orthographic) or may not (here: semantic) reach their optima.
The second dimension of the model is the dimension of mappings between the representations of different domains in a lexical entry. In contrast to the properties of lexical representations in terms of the dimension of linguistic domains and the dimension of networks, the mapping between the domain components of a representation has not usually been considered in the context of the question “what is involved in knowing a word” (cf. e.g., the corresponding list in Nation, Reference Nation2001, p.27 or Read, Reference Read, Bogaards and Laufer2004). In a fully specified lexical representation, all domains are well interconnected, which enables its easy retrieval. Before such a stage is reached, the strength of the mapping links can vary on a continuum from weak to robust. We assume that the mapping between the different domains of an L2 representation is typically weaker than in L1 (or yet non-existent). The importance of well-interconnected/mapped linguistic domains is highlighted in the Lexical Quality Hypothesis (LQH) developed for L1 reading (Perfetti & Hart, Reference Perfetti, Hart, Verhoeven, Elbro and Reitsma2002; Perfetti, Reference Perfetti2007), because only when all domains are able to be activated with relative synchronicity can reliable word identification and its automatic retrieval during reading be expected. Ehri (Reference Ehri2014) refers to these mappings as “connections that are activated to bond the identities of words in memory”. In Figure 2, we show how the dimension of mappings is modelled in the OM.Footnote 4
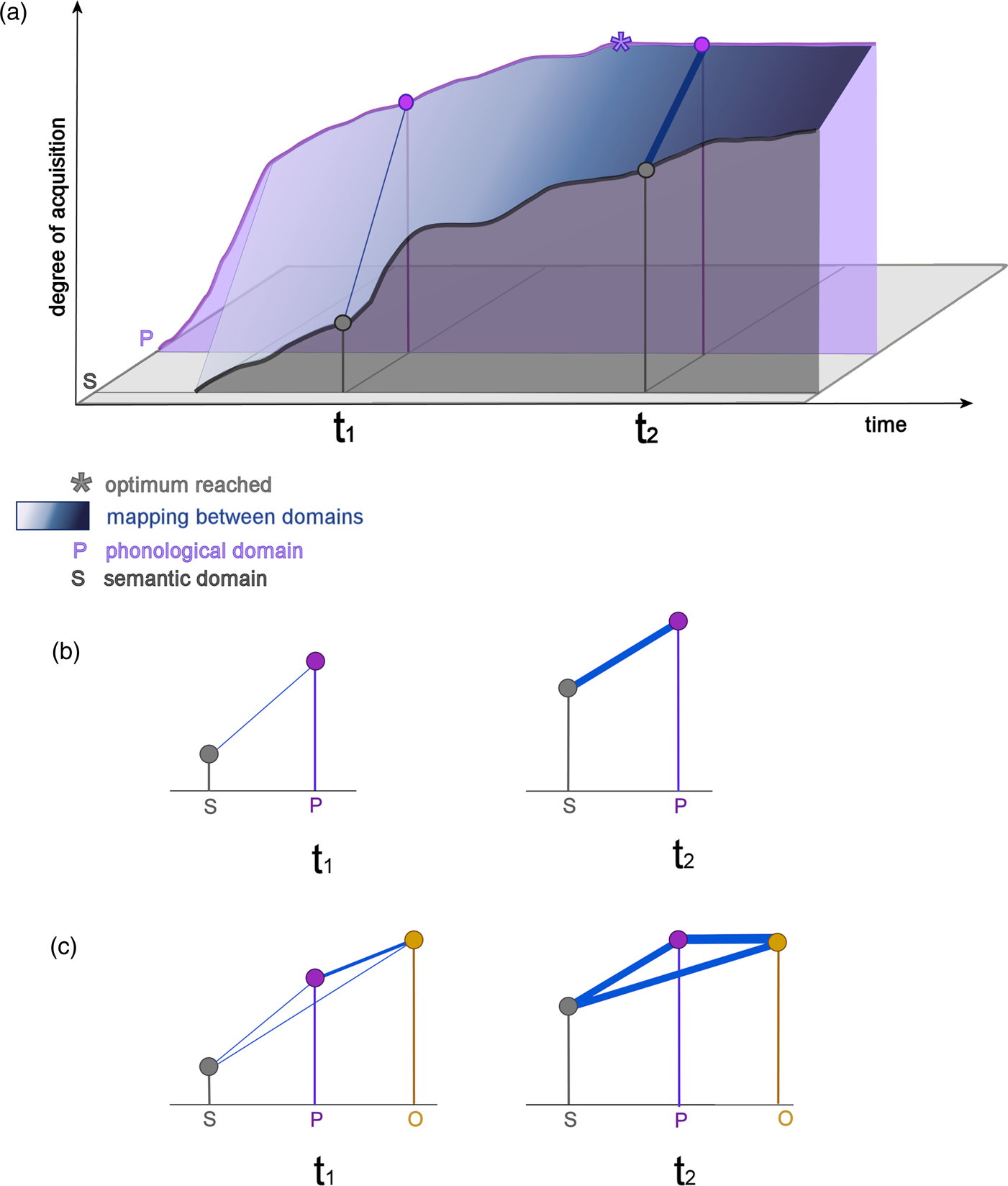
Figure 2 Figure 2a plots again (cf. Figure 1) the semantic (in front) and phonological domains curves (for clarity, the domain of orthography is omitted here). The mapping between these domains is depicted as links between the curves that grow more and more robust over time – in the graph the links produce a continuous surface that grows darker as the links grow more robust. t1 and t2 on the x-axis represent two different time points of the development. The mapping between the domains at these particular timepoints is shown by a highlighted mapping link (purple).
In 2b, the cross-sections at timepoints t1 and t2 are depicted. While at t1 the mapping between the semantic to the phonological representation is still weak (a thin line), it is more pronounced at time t2 (thicker line).
Figure 2c adds the third domain, orthography, to the cross-sections of graph 2b for illustration.
The third dimension is the dimension of networks. A representation can be well integrated in the network or only loosely with just a few connections; similar to the mapping links, also these connections may vary in strength. In the research on the depth of vocabulary knowledge, network knowledge (Read, Reference Read1993; Reference Read, Bogaards and Laufer2004) refers to how a given lexical representation is interconnected with other units in the given language (called IntraNetwork in the OM). Some descriptions refer to this aspect with the terms “associations”, “use” or “collocations” (Nation, Reference Nation2001, p.7) – knowledge about which words are used together or which associate or collocate is implemented as strong links between these lexical representations. Since the OM views the networks from the perspective of an individual lexical representation, each domain in the representation has its own network. The number of connections in a network depends primarily on two factors: the number of lexical representations at a particular time and the quality of their encoding. Figure 3 shows how the dimension of networks is modelled in the OM.Footnote 5
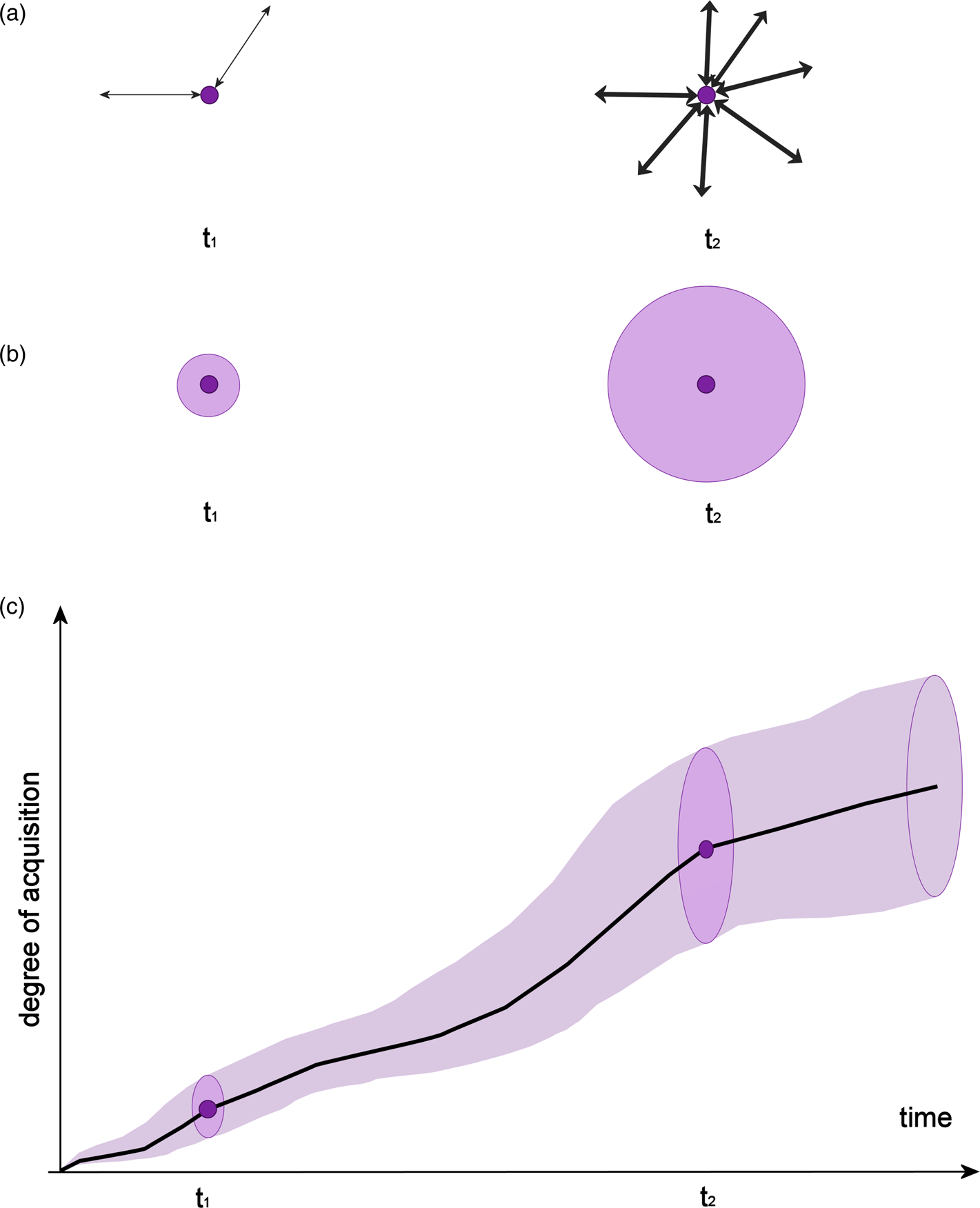
Figure 3 Figure 3a is a schematic representation of network integration at two timepoints. At t1, the representation has only few and weak connections (indicated by fewer, thinner arrows) to other representations, at t2, it is better integrated (indicated by more and thicker arrows).
Figure 3b is an abstraction of 3a, which is used in the OM when modelling this dimension. The circle with a smaller radius represents weaker, more fuzzy integration into the network.
Figure 3c shows an ontogenetic domain curve (e.g., semantic) with gradual network integration. Depicted over time, the circles representing network integration yield a cone-like structure around the curve in the three-dimensional space; its radius grows as the representation becomes better integrated in the corresponding network.
Connections between the nonnative and native lexical units are usually not discussed with regard to the depth of L2 lexical knowledge or the quality of L2 lexical representations, but are addressed separately in the models of the bilingual lexicon. In the OM, they are called InterNetwork and could be depicted as an additional cone at a given domain. The ontogenesis of both networks differs depending on the domain and other factors, such as the context of lexical acquisition or type of training.
A lexical representation is multidimensional and thus comprises all the multiple representational components outlined above. Their acquisition is not an all-or-nothing process (Gyllstad, Reference Gyllstad, Bardel, Lindquist and Laufer2013), but rather an ontogenetic process. Each representational component is typically acquired gradually and cumulatively.
In previous research, it was especially in the acquisition of meaning where this ontogenetic aspect was considered (cf. “precision of meaning” as an extra aspect in Read, Reference Read, Bogaards and Laufer2004, or the stages in the Vocabulary Knowledge Scale of Paribakht & Wesche, Reference Paribakht, Wesche, Coady and Huckin1997) and the acquisition of the IntraNetwork (Wilks & Meara, Reference Wilks and Meara2002, Reference Wilks, Meara, Daller, Milton and Treffers-Daller2007). In many cases, however, the mental lexicon is viewed from a holistic perspective, especially by studies focusing on the size of the lexicon that approach word knowledge as an either-or issue, disregarding the variation and individual differences between the lexical representations.
The Depth of individual word knowledge model (DIWK) by Wolter (Reference Wolter2001), which can be seen as one of the predecessors of the OM, assumes that “words in the mental lexicon are acquired individually and, as such, undergo developmental shifts separately from other words in the mental lexicon”. In the OM, we go one step further and assume that not whole individual words, but the components of each dimension, undergo their own developmental shifts; however, we assume they do not happen separately, but may interact with each other, e.g., the orthographic representation positively or negatively affects the development of the phonological representation (cf. Hayes-Harb, Nicol & Barker, Reference Hayes-Harb, Nicol and Barker2010; Showalter, Reference Showalter2020).
Each dimension of a lexical representation and its components thus emerge, develop, and may be subject to attrition individually. What can be described as “complete acquisition” also differs for each dimension and its components. Within the OM, we refer to acquisition optima that are the same for L1 and L2 speakers of the same dialect. In general, the optimum is modelled as a range, but there are also differences between the optima of different domains and dimensions. In the dimension of domains, the optimum for orthographic representations is described by Perfetti and Hart (Perfetti & Hart, Reference Perfetti, Hart, Verhoeven, Elbro and Reitsma2002) as a precisely specified orthographic form that encodes an exact spelling. The optimum for phonological representations could be described by analogy as a precisely specified phonological form that encodes correct pronunciation. For these two domains, the optimum range is rather narrow, as the properties of the representations at their ultimate acquisition stage can be typically exactly defined. However, the range for the optima in the phonological domain may include the encoding of stylistic and regional variants of pronunciation. In the orthographic domain, the range may include the encoding of regional spellings, as in “colour” and “color”. The definition of the optimum for semantic representations is challenging per se, since word meanings are not fixed; they develop and change both as a reaction to changes within the mental lexicon (e.g., related meanings emerge) and as a reaction to changes in the outside world. When we approximate the optimum of a semantic representation, it is elaborate and specific (cf. Read, Reference Read, Bogaards and Laufer2004), and covers all core senses of the word. Importantly, while the phonological and orthographic optima are typically the same for all speakers of the same dialect, the semantic optimum may differ to some degree for individual speakers (e.g., specific senses may be available only to experts in particular fields), although the core meaning of each semantic representation should have the same or a very similar optimum (with the encoding of the same semantic features) for all native speakers. For these reasons, the optimum range for the semantic domain is broader.Footnote 6
In the dimension of mappings, the optimum range is reached when the linguistic domains of lexical representations are well interconnected or when orthographic, phonological, and semantic elements “have been amalgamated” (Niolaki et al., Reference Niolaki, Vousden, Terzopoulos, Taylor, Sephton and Masterson2020, p.591). The links between the individual domains of a representation are established and sufficiently strong so that the whole information about the lexical entry can be easily retrieved at an encounter with any domain.
In the dimension of networks, the IntraNetwork optimum can be defined as a rich network of (adequately strong) connections with other relevant representations in a given domain. As will be elaborated in the section Dimension of networks, it is not only the number and strength of connections that is relevant, but also their type (phonological vs. syntagmatic and paradigmatic connection, cf. Wolter & Yamashita, Reference Wolter and Yamashita2015). In contrast to the optimum in the phonological and orthographic domains, the optimum of the IntraNetwork cannot be exactly pinpointed and subsumes a broader range. The optimum for InterNetwork is not defined (see Dimension of networks for the reasoning).Footnote 7
The ontogenesis of lexical representations comprises different stages with respect to all its dimensions and their components. The existence of lexical representations starts with their emergence. The optimum may or may not be reached, and similarly the process of attrition may or may not start. The concept of an optimum is closely related to that of fuzziness. When a component of a dimension is not at its optimum, it is more or less fuzzy. Fuzzy representations are imprecise. In the domains of phonological or orthographic representations, this means that the encoded pronunciation/spelling is incomplete with some segments not fully specified or that it contains one or more (temporarily or permanently) incorrect phonemes/graphemes. A meaning representation is fuzzy when it contains only a few semantic features, so that the speaker has a vague and imprecise idea of what the word means, or when incorrect semantic features are included and borders between individual semantic representations not clearly set. Fuzziness in the dimension of mappings refers to missing or only very weak links between the linguistic domains. In the IntraNetwork, fuzziness is related to a limited number of connections, inappropriate connections, or overrepresented/underrepresented connections of some type. Crucially, fuzzy, i.e., inaccurate or low-resolution encoding in one or more domains has a cascading effect on the robustness of the mappings between different domains and the strength of connections in the networks. The degree of fuzziness varies, but the closer a representation is to its optimum, the closer is the degree of fuzziness to zero. A representation is fuzzy not only before reaching its optimum, but may become fuzzy also at subsequent stages, e.g., due to attrition, neurological damage, or disease.
Thus, while other frameworks, e.g., connectionist models, model non-optimal representations via, e.g., non-final weights and optimize them via re-weighting of connections due to new input (i.e., with increasing input frequency), fuzziness in the OM refers to imprecise lexical encoding due to a broader range of linguistic and cognitive factors and the learning conditions (see Gor et al., in preparation).
The OM assumes that fuzziness is a more pervasive phenomenon in the L2 mental lexicon compared to the L1 in all its dimensions and their components. In the following sections we illustrate this claim and show that this L1-L2 difference may be responsible for the effects that have not been considered in this light and were ascribed to different sources. We further believe that individual variation at multiple levels, from the differences between the components of a dimension up to the differences between learners’ lexical performance, can be illuminated from the OM perspective.
Figure 4 models all three dimensions of the OM (linguistic domains, mapping, network integration) and their development over time.
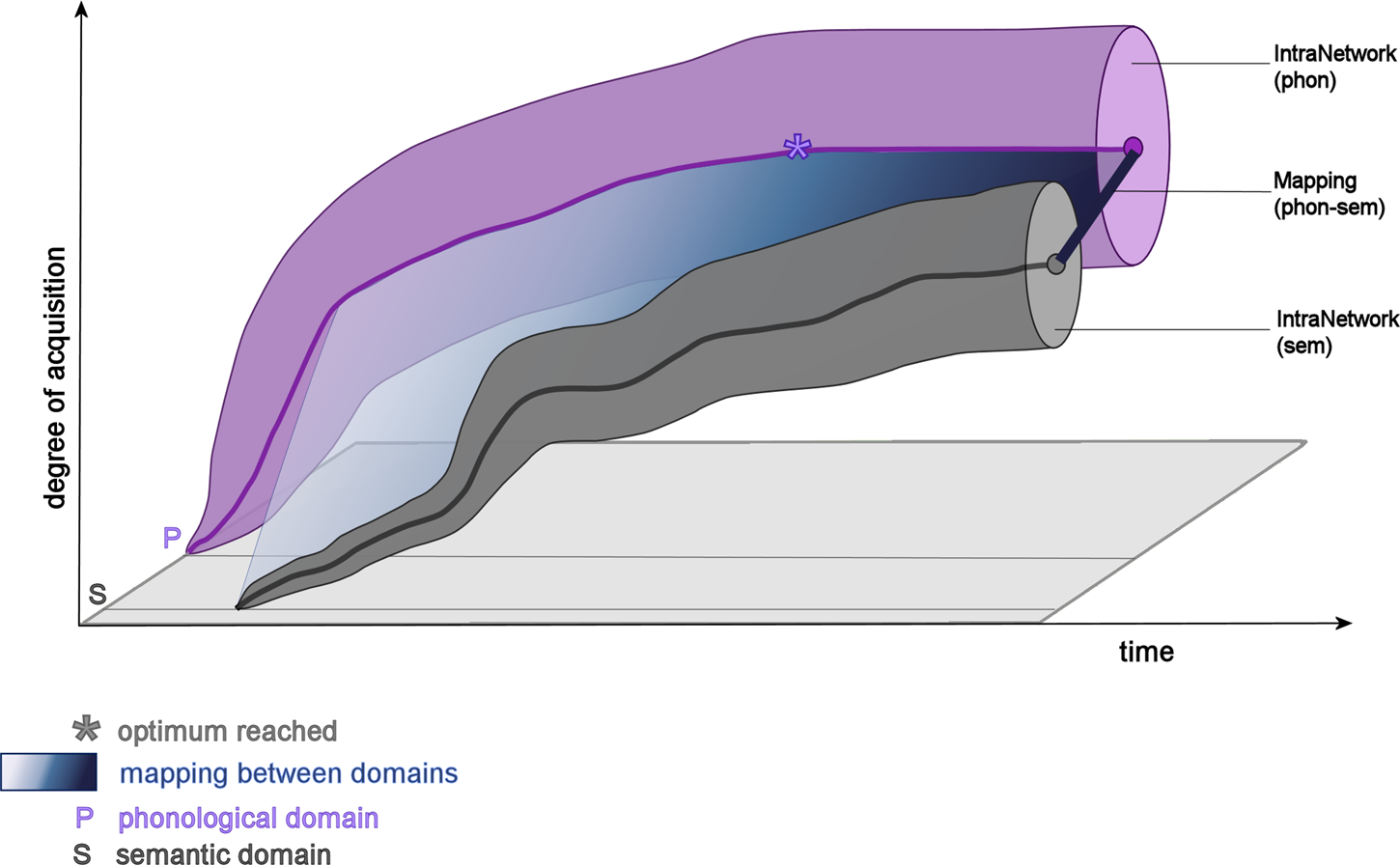
Figure 4 In this figure, all three dimensions (linguistic domains, mapping, IntraNetwork) are modelled, as they develop overtime. The orthographic domain is not represented for the sake of clarity. (For the same reason, the optimum range and colouring of the space under each curve are not depicted either.)
2. Dimension of linguistic domains
The current version of the model focuses on three domains that constitute a lexical entry and correspond to the three types of word knowledge that are in the foreground of acquisition research. As an example, LQH (Perfetti & Hart, Reference Perfetti, Hart and Gorfein2001; Perfetti & Hart, Reference Perfetti, Hart, Verhoeven, Elbro and Reitsma2002; Perfetti, Reference Perfetti2007) posits that lexical representation quality depends on phonology, orthography, semantics and (in Perfetti, Reference Perfetti2007) morpho-syntax. It defines lexical quality as “the extent to which a mental representation of a word specifies its form and meaning components in a way that is both precise and flexible,” (Perfetti, Reference Perfetti2007, p.359) and shows how, e.g., the accuracy and efficiency of word recognition and higher skills such as reading comprehension are impacted if information in any domain is missing or imprecise. It thus recognizes fuzziness as a decisive factor characterizing the ontological development of lexical representations at the dimension of domains.
The development of the representations in each domain does not proceed independently from each other. Rather, the three domains may scaffold on each other in lexical acquisition – robust encoding in one domain may contribute to a decrease of fuzziness in others and to better learning outcomes overall (see also Dimension of mappings). Several studies support the assumption that knowledge in the individual domains is not acquired in isolation, although not all imaginable types of mutual influence have been explored. As an example, there is significant evidence that knowledge from the orthographic domain affects the acquisition of phonological representations (e.g., Showalter, Reference Showalter2020), as well as research suggesting that semantics may provide a learning advantage in new word form learning (Angwin et al., Reference Angwin, Phua and Copland2014; Havas, Taylor, Vaquero, de Diego-Balaguer, Rodríguez-Fornells & Davis, Reference Havas, Taylor, Vaquero, de Diego-Balaguer, Rodríguez-Fornells and Davis2018). In addition, novel word learning experiments that manipulated the properties of word form and meaning revealed that words with nonnative phonology were more difficult to learn than words with native phonology, even if the meanings represented by pictures were of familiar objects. Similarly, words referring to new, nonexisting concepts (pictures of novel objects) were more difficult to retain than those referring to familiar concepts, even when combined with easily pronounceable word forms in native phonology (Havas et al., Reference Havas, Taylor, Vaquero, de Diego-Balaguer, Rodríguez-Fornells and Davis2018). Therefore, problems with either phonological or semantic encoding lead to weaker word learning outcomes.
A substantial amount of research addressing more than one dimension or domain, exploring initial acquisition and employing online methods is related to the Complementary Learning Systems (CLS) account (Lindsay & Gaskell, Reference Lindsay and Gaskell2010), either by targeting it directly or by referring to it. According to the account, an episodic memory trace is formed first (supported by the medial temporal lobe), which is sufficient for explicit recognition and recall of novel lexical items (corresponds to the domain dimension in the OM). Through the process of consolidation, the new lexical item then becomes a cortical representation that is integrated into the existing phonological and semantic networks in a way that allows interaction between the new and older items as revealed, e.g., through semantic priming effects and engagement in lexical competition (corresponds to the IntraNetwork dimension in the OM). Although most of this research has been performed in L1, it also translates into L2 word learning (cf. Lindsay & Gaskell, Reference Lindsay and Gaskell2010 for discussion), although not without limitations.Footnote 8
One difference between L2 knowledge acquisition from the phonological or orthographicFootnote 9 domains (both referred to as the word form), on the one hand, and from the semantic domain, on the other, is that the ontogenesis of the former almost always involves the establishment of a new representation (with the exception of cognates), whereas existing semantic representations created during L1 acquisition can be employed when establishing an L2 entry. This ontogenetic difference can have consequences for knowledge acquisition in other dimensions and may explain the processing differences observed in CLS research with respect to the need for consolidation (or the lack thereof) to observe the expected effects. For example, an existing semantic representation already has connections with other representations and may thus immediately engage within the IntraNetwork. In contrast, a new word form representation (and also a new semantic representation if it needs to be established) has to be connected with other representations first, before it can engage with them.
Thus, when word form learning is addressed in the CLS-related research (typically, using RT and ERP methods), the effects of domain knowledge (e.g., word recognition effects) appear immediately after learning, while the effects of the IntraNetwork engagement (i.e., the priming effects showing the level of lexical integration) appear typically with a delay (e.g., Dumay & Gaskell, Reference Dumay and Gaskell2007; Reference Dumay and Gaskell2012; Bakker, Takashima, van Hell, Janzen & McQueen, Reference Bakker, Takashima, van Hell, Janzen and McQueen2014 in L1; Havas et al., Reference Havas, Taylor, Vaquero, de Diego-Balaguer, Rodríguez-Fornells and Davis2018 in early L2). In contrast, when the learning of meaning is explored, both the domain knowledge effects and the IntraNetwork semantic engagement effects are often observed directly after learning (Mestres-Missé, Rodriguez-Fornells & Münte, Reference Mestres-Missé, Rodriguez-Fornells and Münte2007, Borovsky, Kutas & Elman, Reference Borovsky, Kutas and Elman2010; Borovsky, Elman & Kutas, Reference Borovsky, Elman and Kutas2012; Lindsay & Gaskell, Reference Lindsay and Gaskell2013, but see Henderson, Devine, Weighall & Gaskell, Reference Henderson, Devine, Weighall and Gaskell2015). As discussed below, these findings concerning the difference between available, pre-existent representations in the semantic domain and the initial absence of representations in the phonological or orthographic domains may result in acquisition following different ontogenetic curves.
In the following sections, we address the evidence that relates specifically to the individual domains.
2.1 Phonological and orthographic domains
Word form is often the first and only new piece of information about a word that learners encounter and internalize (Ecke, Reference Ecke2015; Jiang & Zhang, Reference Jiang and Zhang2021). Previous research showed that the initial word form representation can emerge remarkably fast (cf. the CLS-related research). For instance, results of an eye-tracking study by Godfroid and colleagues (Godfroid et al., Reference Godfroid, Ahn, Choi, Ballard, Cui, Johnston, Lee, Sarkar and Yoon2018) suggest that – compared to meaning – a novel word form is recognized faster. Word forms may be a shallower type of word knowledge that can be acquired more easily through simple repetition (Nation, Reference Nation2001), while the mapping of that knowledge to other domains (e.g., to its meaning) leads to better word retention in memory (Takashima, Bakker, van Hell, Janzen & McQueen, Reference Takashima, Bakker, van Hell, Janzen and McQueen2017).
At the same time, various factors have been identified that affect the ease, efficiency, and accuracy in the encoding of a new form representation and its stability, and thus shape the ontogenetic curve of the new word form, especially in its initial stages. For example, the size of the lexicon to which a new word form would be added has been shown to co-determine its ease of acquisition, because it defines the repertoire of already available phoneme sequences or letter strings to which the new word form can conform (e.g., Ellis, Reference Ellis2002; Bordag, Kirschenbaum, Rogahn & Tschirner, Reference Bordag, Kirschenbaum, Rogahn and Tschirner2017b). Similarly, vocabulary training studies exploring early stages of word form encoding and memorization have shown that L1 schema-inconsistent knowledge (e.g., words with L2 phonology when the L2 lexicon is very small or virtually nonexistent) is more dependent on consolidation compared to pseudowords that conform to L1 phonology and phonotactics (Bakker et al., Reference Bakker, Takashima, van Hell, Janzen and McQueen2014; Bakker, Takashima, van Hell, Janzen & McQueen, Reference Bakker, Takashima, van Hell, Janzen and McQueen2015). Havas and colleagues (Havas et al., Reference Havas, Taylor, Vaquero, de Diego-Balaguer, Rodríguez-Fornells and Davis2018) claim that, during L2 word learning, neocortical systems can only activate an approximate representation of a new phonological form and hence are less effective in supporting hippocampal encoding in long-term memory. The ontogenetic curve of such representations is thus likely to rise less steeply than of those with familiar phonology and phonotactics (see Figure 5a). Other L2 novel word learning studies (e.g., Escudero, Hayes-Harb & Mitterer, Reference Escudero, Hayes-Harb and Mitterer2008; Escudero, Simon & Mulak, Reference Escudero, Simon and Mulak2014; Hayes-Harb & Masuda, Reference Hayes-Harb and Masuda2008) further show how phonological categorization difficulties that involve nonnative phonemes reduce the efficiency of phonological encoding.
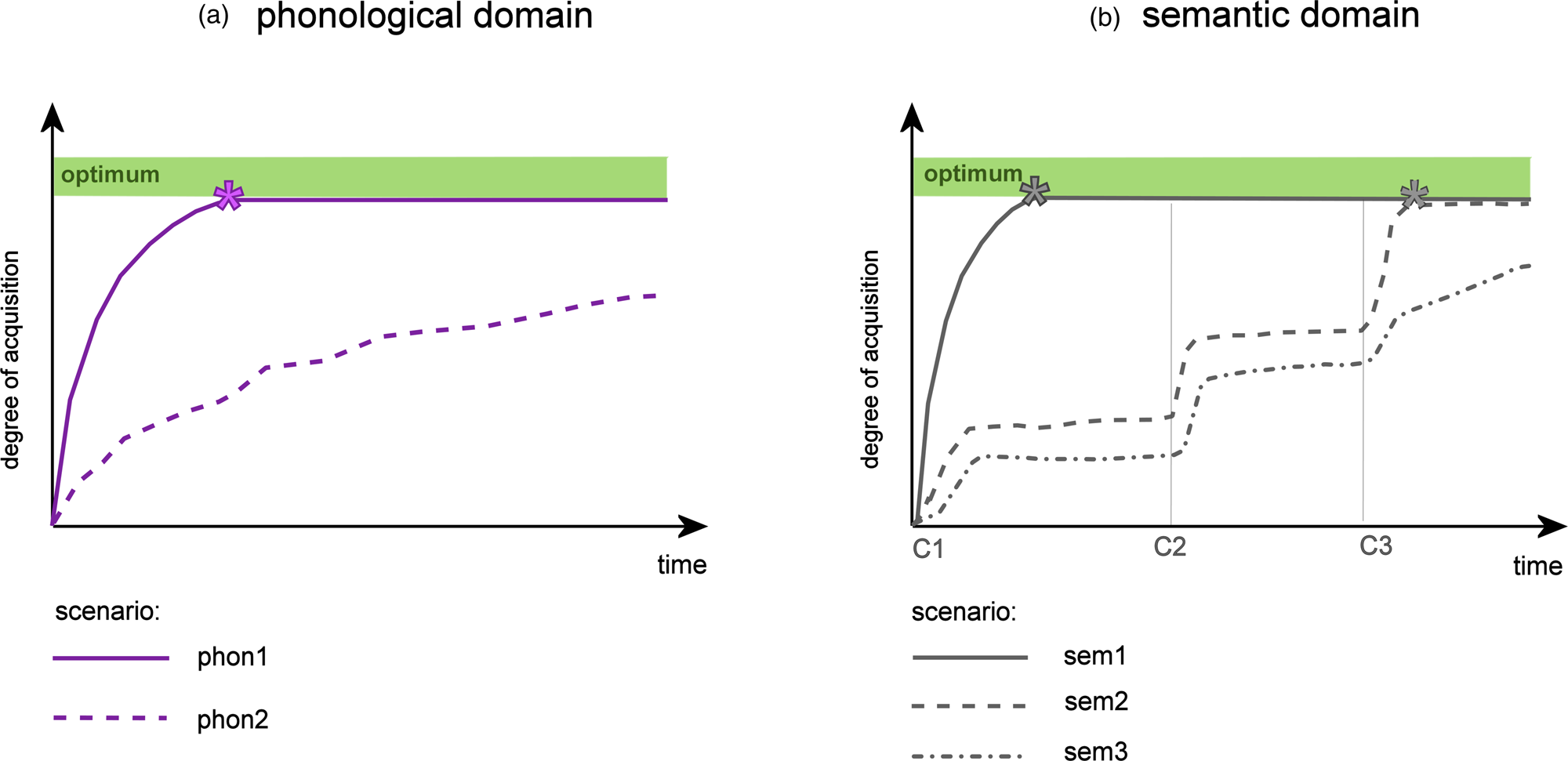
Figure 5 Phonological domain (a): In scenario phon1, the phonotactics of the new word in L2 (e.g., /tɪʃ/ German Tisch ‘table’) are in accordance with the L1 phonotactics (e.g., English). The ontogenetic curve is thus rather steep and may reach the optimum quickly.
In scenario phon2, in contrast, the new word form is not supported by the phonotactics of L1 (e.g., /knɔp͜͜f/ consonant clusters in Knopf ‘button’) and accordingly, the acquisition of the phonological form proceeds slower, the representation may stay fuzzy, and the optimum may not be reached.
Semantic domain (b): In scenario sem1, the L2 word, for instance, ‘dandelion’ is learned via an explicit translation equivalent (e.g., in word lists: Löwenzahn (in L1 German) - dandelion (in L2 English)). The corresponding semantic representation of ‘dandelion’ can be easily identified and the new L2 word form can be mapped directly on it.
In scenario sem2, the new word ‘dandelion’ is, e.g., acquired incidentally through multiple exposures in texts. At context C1, e.g., the word appears in a context that allows the learner to infer that it is a flower. At C2, e.g., the context provides information about the color of the flower, context C3, e.g., information about its form and time of blooming etc. If enough and sufficient information is accumulated, the learner can identify the corresponding semantic representation of dandelion and map it to the new word form (optimum).
In scenario sem3, the learner gradually infers information about the new word's meaning similar to scenario sem2. In this case, the learner is not familiar with the flower (has no semantic representation) and needs to gradually create a new semantic representation for the new word form. The approximation to the optimum may take longer, alternatively, the representation may stay fuzzy.
In addition to internal word form properties, external factors such as the type of learning (incidental vs. intentional) determine which word form properties become crucial for the establishment of the initial form representations and thus also define the ontogenetic curve. For example, Bordag and colleagues (Bordag et al., Reference Bordag, Kirschenbaum, Rogahn and Tschirner2017b) show that high phonotactic probability has primarily positive effects on intentional learning of new words from definitions, while low phonotactic probability has primarily positive effects on their initial incidental acquisition during reading. These effects conform to the understanding of the differences between the two types of word learning. In intentional word learning from definitions, L2 words with easily encoded orthographic form are better retained. In incidental word learning, words with unusual form are more salient and more easily detected.
Although the factors described above affect the shape of the ontogenetic curve, the main difference between the L1 and L2 word form representations does not seem to be in the shape of their ontogenetic curves in general (cf. the difference between the L1 and L2 ontogenetic curves of semantic representations), but rather because L2 forms more often than L1 forms fail to reach their optima. Problems with form encoding lead to fuzzy lexical representations and low accuracy in word retrieval from memory (Escudero et al., Reference Escudero, Hayes-Harb and Mitterer2008). Importantly, form encoding has two unique properties within lexical representations: novel L2 word learning starts with the form, and the form needs exact encoding (or to reach its optimum) for the lexical entry to function properly. This is why fuzzy form representations have such a detrimental impact on L2 lexical processing.
The idea that phonological encoding in L2 lexical representations is not as robust as in L1 lexical representations has been explored within the linguistic representations-based approach. One account focuses on the difficulties with phonological encoding of lexical items resulting from problems with phonetic categorization of L2 contrasts that are absent in L1 (Pallier, Colomé & Sebastián-Gallés, Reference Pallier, Colomé and Sebastián-Gallés2001; Darcy et al., Reference Darcy, Dekydtspotter, Sprouse, Glover, Kaden, McGuire and Scott2012, 2013). The phonetic categorization performance on a problematic L2 contrast is compared to the rate of lexical confusions in minimal pairs of words differentiated by this contrast. For example, Darcy et al. (Reference Darcy, Daidone and Kojima2013) tested American learners of German on how they categorized German front and back rounded vowels. While they were highly accurate in a categorization task, they showed a very high error rate in the auditory lexical decision task in rejecting pseudowords created by swapping the front and back rounded vowels (e.g., König ‘king’ with /ø/ vs. pseudoword *Konig with /o/). The research led to the formulation of the lexical coding deficiency hypothesis, according to which the deficit is located at the lexical coding level and not the perceptual level.
While phonological categorization difficulties clearly lead to fuzziness in the phonological encoding of L2 words, the fuzzy lexical representations (FLR) account (Cook & Gor, Reference Cook and Gor2015; Gor & Cook, Reference Gor and Cook2020; Gor, to appear; Gor et al., in preparation), with which the OM aligns, assumes that fuzziness is a more general property of L2 lexical representations that are often characterized by imprecise encoding of their components. In this respect, the OM differs from the activation-based approaches to lexical representations, such as the lexical entrenchment account (Brysbaert, Lagrou & Stevens, Reference Brysbaert, Lagrou and Stevens2017; Diependaele, Lemhöfer & Brysbaert, Reference Diependaele, Lemhöfer and Brysbaert2013). While the OM also acknowledges lexical frequency as a crucial factor in shaping robust lexical representations, it focuses on the quality of lexical encoding driven by linguistic factors and interacting with lexical frequency. According to the FLR account, less familiar L2 words have only approximate phonological representations in the mental lexicon (i.e., they are below their representation optimum), which creates uncertainty at the processing level and blurs the boundaries between similar-sounding word forms. This fuzziness then manifests itself in lexical confusions and nonnative patterns in lexical competition (cf. also earlier studies on confusions between similar L2 word forms by Meara, Reference Meara1983 or Sunderman & Kroll, Reference Sunderman and Kroll2006).
Lexical confusions that are not rooted in difficulties in encoding particular non-native contrasts were demonstrated for example by Cook et al. (Reference Cook, Pandža, Lancaster and Gor2016, Experiment 1) using a cross-modal translation judgment task: L2 speakers were slower to reject an auditory Russian prime (e.g., /malako/) when it was presented with an English translation not of itself (i.e., ‘milk’), but of a similar-sounding Russian word (i.e., ‘hammer’ which is /malatok/ in Russian) compared to a control condition. By using Levenshtein's distance measure of phonological overlap between two similar-sounding words (Levenshtein, Reference Levenshtein1966), it was found that lexical confusions were triggered by more dissimilar words in L2 than in L1.
Therefore, fuzziness in the phonological encoding has a strong impact on L2 lexical processing.
In auditory word recognition, words compatible with the input are activated together with similar-sounding words and compete with them for selection (Marslen-Wilson, Reference Marslen-Wilson1987). L1 speakers quickly resolve lexical competition between properly encoded phonological neighbors. In contrast, lexical competition in L2 is weak (see Gor & Cook, Reference Gor and Cook2020; Gor, to appear). While irrelevant word forms in the L2 lexicon can be activated due to their fuzzy encoding, e.g., ‘flesh’ can be spuriously activated when ‘flash’ was heard, this phantom activation does not lead to increased lexical competition (Broersma, Reference Broersma2012), because lexical competition in L2 is generally weak, in particular for confusable words. In L1, strong form-based lexical competition manifests itself as inhibition in phonological priming experiments with word-initial overlap (Slowiaczek & Hamburger, Reference Slowiaczek and Hamburger1992; Dufour & Peereman, Reference Dufour and Peereman2003). In contrast, in L2 learners, low-frequency or low-familiar primes produce facilitation instead of inhibition (Cook & Gor, Reference Cook and Gor2015; Gor & Cook, Reference Gor and Cook2020). The reversal of the priming effect from inhibition to facilitation is a sign of weak form-based competition. According to FLR, when the L2 lexical competitors are weak, sublexical facilitation provides a processing boost to the target that has the onset overlapping with the prime. Crucially, FLR does not assume different processing mechanisms in L1 and L2, but interprets the processing differences as a consequence of a higher degree of fuzziness in L2.
The processing of orthographically similar words in L2 shows the same pattern as the processing of phonologically similar words that can be attributed to the same source – a higher degree of fuzziness in L2 orthographic representations resulting in weak lexical competition. In visual masked priming experiments, L2 participants show facilitation for orthographically related word pairs, e.g., scandal-SCAN (Heyer & Clahsen, Reference Heyer and Clahsen2015; see also Diependaele, Duñabeitia, Morris & Keuleers, Reference Diependaele, Duñabeitia, Morris and Keuleers2011; Li, Taft & Xu, Reference Li, Taft and Xu2017). Following the same logic as for phonological facilitation, orthographic overlap between the prime and the target leads to an orthographic processing boost rather than to inhibition caused by lexical competition. Such an interpretation also agrees with the LQH (Perfetti & Hart, Reference Perfetti, Hart, Verhoeven, Elbro and Reitsma2002; Perfetti, Reference Perfetti2007) that focuses on the quality of orthographic encoding as part of lexical encoding. For orthographic representations, it assumes that in fully specified forms (optima), all letters are constants, while in low-quality, fuzzy representations some letters are variable. Current research indicates that the concept of orthographic depth (e.g., Schmalz, Marinus, Coltheart & Castles, Reference Schmalz, Marinus, Coltheart and Castles2015), which refers to the transparency of the grapheme-phoneme correspondences, may also affect the degree of fuzziness of orthographic forms – with languages that have a deeper orthography tending towards a higher degree of orthographic fuzziness (e.g., Share, Reference Share2004a; Seymour, Aro, Erskine & Collaboration with COST Action A8 Network, Reference Seymour, Aro and Erskine2003 in L1, van Daal & Wass, Reference van Daal and Wass2017 in L2).
2.2 Semantic domain
Most models assume that semantic representations consist of component features represented as nodes in a complex connectionist network (e.g., Caramazza, Hillis, Rapp & Romani, Reference Caramazza, Hillis, Rapp and Romani1990; Taylor, Devereux & Tyler, Reference Taylor, Devereux and Tyler2011; Vigliocco, Vinson, Lewis & Garrett, Reference Vigliocco, Vinson, Lewis and Garrett2004). An overlap between semantic representations then corresponds to the number of shared component features. Shared semantics across languages are assumed by theoretical models of bilingualism (e.g., the Revised Hierarchical Model, Kroll & Stewart, Reference Kroll and Stewart1994; the BIA+ model, Dijkstra & van Heuven, Reference Dijkstra and van Heuven2002; and Green's convergence hypothesis, Green, Reference Green, Van Hout, Hulk, Kuiken and Towell2003); however, languages map their words onto the conceptual store in different ways. Semantics of individual words differ along a continuum that has translation equivalents whose semantics completely overlap in two given languages at the one end, and words whose semantic representation exists only in one of the languages at the other (cf. Distributed Feature Model, Van Hell & De Groot, Reference van Hell and de Groot1998 or the model of Duyck & Brysbaert, Reference Duyck and Brysbaert2004). Between these extremes, there are translations with a different degree of semantic equivalence, i.e., with different degrees of overlap between their semantics (Bordag et al., Reference Bordag, Opitz, Rogahn and Tschirner2018; De Groot, Reference De Groot2011; Pavlenko, Reference Pavlenko and Pavlenko2009). However, it is important to note that differences in individual meanings between L1 and L2 do not have to be registered by L2 learners, who are not in close contact with L2 and its culture and/or are not highly proficient. They may simply map the L2 word onto the pre-existing semantic representation without ever noticing that the translation equivalence is incomplete (cf. Jiang, Reference Jiang2002). In addition, lexical representations also differ with respect to the number of their senses with L2 representations typically comprising fewer senses compared to the richly populated L1 representations (cf. the Sense Model by Finkbeiner, Forster, Nicol & Nakamura, Reference Finkbeiner, Forster, Nicol and Nakamura2004).
During L1 acquisition, the semantic store needs to be established, both with respect to the component features and semantic representations. In L2 acquisition, this information is already available, with multiple scenarios of how the ontogenesis of the new representation might evolve in the initial stages (see Figure 5). In the simplest ontogenetic scenario, L2 word forms can be directly linked to existing semantic representations (possibly via the L1 form for novice learners, De Groot, Dannenburg & van Hell, Reference De Groot, Dannenburg and van Hell1994; see Bordag et al., Reference Bordag, Kirschenbaum, Rogahn, Opitz and Tschirner2017a for a detailed description), as it is typically the case when L1-L2 vocabulary pairs are learnt, and the translation equivalency is known (or assumed). In this case, there is a sudden rise of the semantic ontogenetic curve towards the optimum and the engagement with other semantic representations may start almost immediately (Figure 5b, scenario sem1). In more complex ontogenetic scenarios, learners need to discover the equivalency and highly fuzzy semantic representations comprising: for example, only very general features (e.g., some kind of blossoming flower instead of a dandelion), or a specific but incomplete set of features may emerge. This is typically the case when the meaning needs to be inferred in incidental vocabulary acquisition and depends on the input quality with respect to the available cues (Ellis & Collins, Reference Ellis and Collins2009) (Figure 5b, scenario sem2). In another scenario, the word in L2 refers to a completely new concept, whereby a new semantic representation needs to be established (similarly to L1 acquisition) and the ontogenetic curve rises towards its optimum only gradually (Figure 5b, scenario sem3). After a word form is matched to an existing representation, it may turn out that the translation equivalence is incomplete and that partially different semantic features correspond to the words in the two languages. Consequently, the mappings need to be restructured and the semantic representations of the L2 adjusted adequately. This process may characterize the ontogenesis of semantic representations across their lifespan as speakers encounter them in new contexts and/or they gain new senses (or lose them) (Beck, McKeown & Kucan, Reference Beck, McKeown and Kucan2002; Bloom, Reference Bloom2000). Accordingly, the semantic representation needs to be both precise and flexible, which supports the modelling of the optimum as a range. The sketched scenarios result in differently shaped ontogenetic curves in the OM. Except for the case when the translation equivalency is known and the new L2 word form can be mapped on the existing semantic representation at its optimum, there is abundant space for fuzziness regarding the establishment of L2 semantic representations at the dimension of domains.
As mentioned at the beginning of this section, while the majority of studies observe the engagement effects at the word form level only after a period of consolidation, the effects of semantic engagement are often observed directly after training. In the OM this observation is primarily modelled as different ontogenetic curves of development of semantic representations. Bordag and colleagues (Bordag et al., Reference Bordag, Kirschenbaum, Tschirner and Opitz2015, 2017a, 2018) and Elgort (Reference Elgort2011) demonstrate how different semantic priming effects in L2 emerge depending on whether a new L2 word form was linked directly to an existing representation (facilitation), or whether a new semantic representation was established (inhibition in Bordag et al., Reference Bordag, Kirschenbaum, Rogahn, Opitz and Tschirner2017a, 2018; no priming effect in Elgort, Reference Elgort2011; cf. also e.g., Dagenbach, Carr & Barnhardt, Reference Dagenbach, Carr and Barnhardt1990 for L1). Except for the case of cognates, employing a pre-existing word form representation in a similar way when establishing a new lexical entry is typically not possible. The ontogenetic curve for most word forms thus proceeds in a similar way, i.e., by establishing a new word form representation (gradual, but rather steep rise towards the optimum). In contrast, additional factors like the pre-existence of a given semantic representation or the probability of its quick identification play a role in the acquisition of semantic domain knowledge and shape its ontogenetic curve. Thus, semantic acquisition strongly depends on the availability of cues – it is at its maximum when learning translation equivalents, lower when learning from definitions, least when inferring from context, depending on the number of exposures, etc. The variability of semantic ontogenetic curves during initial acquisition is thus higher and covers larger extremes. Measuring semantic acquisition is therefore highly sensitive to the properties of the items employed in a particular study and to the training tasks, since they can initiate acquisition along different ontogenetic scenarios resulting in different patterns of results (cf. also Kaczer et al., Reference Kaczer, Bavassi, Petroni, Fernández, Laurino, Degiorgi, Hochmann, Forcato and Pedreira2018). This highlights the importance of considering both the type of acquired knowledge (i.e., which linguistic domain is involved) and the ontogenesis of individual words along all their dimensions (and their components) – in a given time, different representations can be in different ontogenetic stages (cf. DIWK in Wolter, Reference Wolter2001).
2.3 Domains conclusion
As discussed, for each domain the onset, steepness of the ontogenetic curve and closeness of the reached maximum to the optimum will depend on a set of factors. At the same time, some generalizations are possible, even if they capture only idealized main tendencies and are subject to exceptions. Table 1 attempts to make such generalizations for the three domains.
Table 1. Domain-internal factors shaping the developmental trajectory for the form and meaning domains of lexical representations.

As evident from the table, in both form modalities, the encoding is unidimensional, and for it to be accurate requires all the segments and their sequences to be exactly represented. Accordingly, if phonological categorization and sequencing (subject to phonotactic constraints) in L2 is problematic for specific phonemes or phoneme clusters, the optimum can be never reached even if it is approximated more over time. At the same time, the quality of phonological and orthographic encoding for an individual lexical representation will also interact with its phonological or orthographic neighbors (see Dimension of networks) – ‘good enough’ phonological encoding may allow the representation to be functional if the word is not confusable with other similarly sounding words (cf. ‘zebra’ with no neighbors vs. ‘sheep’ with a close phonological neighbor ‘ship’).
Semantic representations follow different ontogenetic curves depending on their semantic properties and the learning conditions – the variability of their curves is larger than that of the form ontogenetic curves. Admittedly, the optimum for the semantic domain is only an approximation in the current version of the OM, since the reality is more complex. The optimum could, for instance, be modelled as consisting of two sub-optima, one for the number of senses and one for the preciseness and richness of semantic representation of each sense (i.e., which component features it comprises). The current OM version is based primarily on the latter.
3. Dimension of mappings
In the OM, the dimension of mappings refers to the links between different domains of the lexical representation. As mentioned in the introduction, a strong and stable mapping between different domains is a prerequisite for an easy retrieval of a given representation (Perfetti & Hart, Reference Perfetti, Hart, Verhoeven, Elbro and Reitsma2002; Perfetti, Reference Perfetti2007). The LQH refers to the knowledge captured by the dimension of mappings as ‘constituent binding’ and ‘synchronicity’ (Perfetti, Reference Perfetti2007, p. 360). The most explored types of mappings to date are the form-meaning mappings that include phonology-to-meaning and orthography-to-meaning mappings, and the mappings between two form modalities: phonological and orthographic. This section starts with discussing the links between word form and meaning that constitute the essence of lexical encoding, organization, and processing, and further addresses the links between phonological and orthographic representations.
3.1 Form - meaning mapping
The link between a word form and its semantic representation is built over repeated occurrences of the same form referring to the same meaning by the lexical system that captures the association between the form and context in which it occurs (Taft, Reference Taft, Assink and Sandra2003). Given the reduced exposure to L2, it can be assumed that the mapping links connecting word forms with their semantic representations are weaker than in L1. For example, the weaker links hypothesis claims that the source of the weaker links between semantics and phonology in each lexical system of a bilingual speaker is due to reduced practice that is divided between two languages of a bilingual (Gollan et al., Reference Gollan, Montoya, Cera and Sandoval2008)
Moreover, one of the fundamental differences between L1 and L2 is that phonological and their corresponding semantic representations are typically established more or less simultaneously in L1 and are integrated into a gradually evolving network in each domain. In contrast, lexical acquisition in L2 often involves the establishment of a new form representation and its mapping onto an existing semantic representation. Simultaneous development of the phonological and semantic networks in L1 might foster a stronger mapping between the representations in the two domains in L1 compared to L2.
Besides being generally weaker, a further source of mapping problems in L2 may reside in encoding difficulties in the relevant domains. Vocabulary training experiments capture the initial stages of the mapping process for novel lexical representations. They report that any encoding complexities in each of the domains, form or meaning, lead to mapping problems in L2 and lower word learning rates (Angwin, Phua & Copland, Reference Angwin, Phua and Copland2014; Takashima et al., Reference Takashima, Bakker, van Hell, Janzen and McQueen2017; Havas et al., Reference Havas, Taylor, Vaquero, de Diego-Balaguer, Rodríguez-Fornells and Davis2018).
At the same time, a richer lexical representation, in which both the form and meaning are encoded – and therefore, a mapping between them can be potentially established – is more robust overall. Semantics enhances word learning, and word forms learned together with meanings are better retained in memory than those learned without meanings (Angwin et al., Reference Angwin, Phua and Copland2014; Takashima et al., Reference Takashima, Bakker, van Hell, Janzen and McQueen2017).
The mapping between form and meaning can be fuzzy, i.e., below the optimum, for two main reasons: a) the links between the word form and its meaning are weak, b) the links are unclear – when the speakers are unsure of the meaning of a given word form, or alternatively, they oscillate between two (or more) word forms, often phonological neighbors, for a particular meaning. In the most extreme case, an L2 learner may establish incorrect links between word forms and meanings: for example, when a Russian learner of English uses the words ‘arm’ and ‘hand’ interchangeably (because the same word ‘ruka’ is used for both in Russian). However, a more common scenario is when fuzzy mappings arise as a consequence of fuzzy encoding in one or more of the linguistic domains of the lexical representation.
The construct of weak mappings was developed in the RHM (Kroll & Stewart, Reference Kroll and Stewart1994), according to which L2 words have weak direct links to meanings, in particular, in less proficient speakers, and go through connections to L1 words to access the word meaning level. As an extension of this approach and of the weaker links hypothesis (Gollan et al., Reference Gollan, Montoya, Cera and Sandoval2008), the OM focuses on the fuzzy mappings driven by unfaithful or low-resolution encoding in individual L2 linguistic domains and that lead to lexical confusion within the L2 system. Also, the mappings in the OM are construed as the properties of individual lexical items, while the models mentioned above are more concerned with the generalizable properties of L2 lexical representations belonging to the networks domain or with L1-L2 connections.
Lexical confusions in L2 have been well documented as they occur quite often (Meara, Reference Meara1983; Jiang & Zhang, Reference Jiang and Zhang2021). Given the pervasiveness of fuzzy phonological representations in L2, phonological-semantic confusions are the most common ones (e.g., 58% of errors in Cook & Gor, Reference Cook and Gor2015 were confusions with similar-sounding words unrelated in meaning).
Unfaithful encoding in the form domain can result in fuzzy mappings and form–meaning confusions especially with forms involving non-native contrasts, in particular if they have numerous and/or high-frequency phonological neighbors. Corresponding evidence can be gleaned from visual world eye-tracking experiments when two objects whose names are compatible with the same word onset are present in the visual display. Until the disambiguation point is reached, participants typically alternate their looks to either object. Such experiments can test L2 speakers’ sensitivity to a particular phonological contrast. Cutler and colleagues show that L1 speakers of Japanese are slower than English speakers in deciding that the word they hear is ‘rocket’ and not ‘locker’ because the /r/-/l/ contrast is difficult to discriminate (Cutler et al., Reference Cutler, Weber and Otake2006) – an indication that the mappings between the two word forms and their meanings are fuzzy (see also Weber & Cutler, Reference Weber and Cutler2004; Escudero et al., Reference Escudero, Hayes-Harb and Mitterer2008). Another source of evidence is given by lexical confusions that occur in L2 speakers – when a word in sentence context is replaced by its minimal pair (Chrabaszcz & Gor, Reference Chrabaszcz and Gor2014, Reference Chrabaszcz and Gor2017). Importantly, persistent difficulties with non-native contrasts and the ensuing mapping difficulties occurring despite high input frequency cannot be exclusively attributed to frequency effects (cf. entrenchment) and justify the necessity of approaches focusing on the quality of lexical representations such as the fuzziness hypothesis (cf. Reference Gor, Cook, Bordag and ChrabaszczGor et al. in preparation).
Fuzziness in L2 form-meaning mappings that has its origin in unfaithful encoding of phonological forms transcends difficulties with non-native contrasts and can be generalized more broadly to similar-sounding words. According to Cook and colleagues (Reference Cook, Pandža, Lancaster and Gor2016), fuzzy phonological representations of two similar-sounding words can be merged into one fuzzy representation or be loosely linked to their respective meanings (e.g., /'pɛrət/ for both ‘parrot’ and ‘parent’). Fuzzy phonological representations may then lead to variable and unfaithful form-meaning mappings and to the retrieval of an incorrect semantic representation in lexical access resulting in lexical confusion. Importantly, fuzzy form-meaning mappings may produce a temporary confusion that is later resolved, but causes a processing delay. For instance, an auditory pseudo-semantic priming experiment demonstrated how two auditorily confusable words (e.g., /malatok/ ‘hammer’ and /malako/ ‘milk’ in Russian) produced semantic confusion (Cook et al., Reference Cook, Pandža, Lancaster and Gor2016, Experiment 2). The authors report an inhibition effect in English-speaking learners of Russian when the word pairs were ‘cow-hammer’ rather than the expected ‘cow-milk’, with unrelated word pairs as a baseline. The increase in response times reflected temporary activation of an incorrect form-meaning mapping that took additional time to be resolved.
Orthographic-semantic mappings show the same pattern of results in L2 word learning as phonological-semantic mappings, in particular, in languages with deep orthography (see Perfetti, Reference Perfetti2007). One reason for these mapping difficulties is given by complex orthographic-phonological correspondences. L2 learners need to learn to link the orthographic form of numerous homophones to their semantic representations and to overcome the orthographic processing routines trained by the use of L1 (see Veivo & Järvikivi, Reference Veivo and Järvikivi2013).
The other reason is that phonology is typically automatically activated when word orthography is processed, and phonological encoding problems associated with orthographic encoding problems interfere with form-meaning mappings. This covert influence of fuzzy phonological representations on orthographic-semantic mappings was illustrated in visual word processing. In a semantic relatedness task, Japanese learners of English were likely to say that the words ‘key’ and ‘rock’ were semantically related, presumably because they associated the ‘key-lock’ word pair instead (Ota, Hartsuiker & Haywood, Reference Ota, Hartsuiker and Haywood2009). Thus, their difficulties with the /r/-/l/ contrast percolated to the level of orthographic encoding and, ultimately, to orthographic-semantic mappings. Similar results were observed in a visual semantic categorization task with different English phonological contrasts and L1 Spanish and Japanese participants (Ota, Hartsuiker & Haywood, Reference Ota, Hartsuiker and Haywood2010). Additionally, in the homophone condition, pure confusions in orthographic-semantic mapping were observed: the participants accepted ‘see’ as corresponding to the definition “A body of water” (cf. ‘sea’).
While the previous examples focused on the fuzziness in form representations as the source of unfaithful form-meaning mappings, the locus of fuzziness leading to loose form-meaning mappings can reside in fuzzy semantic representations as well (Bordag et al., Reference Bordag, Opitz, Rogahn and Tschirner2018). If the meaning of a newly encountered L2 word is fuzzy because it is novel, i.e., it does not exist in L1, and/or had to be inferred from the context, the form-meaning mapping also becomes unstable. Using novel words as primes, Bordag and colleagues report inhibition instead of the expected facilitation in semantic priming – an effect that indicates a delay in establishing a mapping link between an unambiguous form and a fuzzy meaning.
3.2 Phonology - Orthography
While modality-specific (phonological and orthographic) form encoding can be treated separately, there is clearly an interface between them, and when both are available, they are co-activated via the corresponding mapping links (e.g., Peleg, Edelist, Eviatar & Bergerbest, Reference Peleg, Edelist, Eviatar and Bergerbest2016). However, the mutual influence of orthographic and phonological forms is not straightforward. On the one hand, orthographic information has been shown to support phonological encoding of novel L2 words when graphic-phonological correspondences were L1-congruent (Hayes-Harb et al., Reference Hayes-Harb, Nicol and Barker2010; Showalter, Reference Showalter2020). This is consistent with the Dual-coding theory (Sadoski, Reference Sadoski2005), which predicts better memorization for words learned with orthographic information, since lexical representations become more robust with each additional source of information. On the other hand, Best and Tyler (Reference Best and Tyler2007) suggest that the use of a common grapheme in L1 and L2 may lead L2 learners to “equating” phoneme categories, even when their phonetic realizations are quite different. In addition, Berthelsen, Horne, Shtyrov, and Roll (Reference Berthelsen, Horne, Shtyrov and Roll2020) refer to “spelling pronunciations” as an example of when exposure to orthography leads to less target-like realizations in pronunciation (see also Bassetti, Reference Bassetti2017). Depending on the grapheme-phoneme relationship between the L1 and L2 and within L2, simultaneous acquisition of orthographic information may thus move the phonological representation closer to or further away from its optimum (and vice versa). Furthermore, the effect of L1 orthography on spoken word recognition in L2 is modulated by L2 proficiency and word familiarity (Veivo & Järvikivi, Reference Veivo and Järvikivi2013).
In orthographic-phonological mappings, L2 learners tend to map incorrect spellings to phonological representations of words. Thus, a pseudohomophone effect was demonstrated in a masked priming study with Finnish learners of French when the prime was a pseudohomophone (a nonword pronounced the same as the target French word) and the target a spoken French word (Veivo & Järvikivi, Reference Veivo and Järvikivi2013). This effect again shows that learners have a fuzzy representation of L2 orthographic forms and incorrectly associate nonwords with spoken word forms.
Overall, these findings indicate a relatively strong link between the orthographic and phonological forms also in L2. Similarly to the joint acquisition of phonological forms and meanings in L1 and contrary to “supplementary” acquisition of word forms (that are mapped onto already existing meanings) in L2, phonological and orthographic forms are often acquired simultaneously or in close proximity in L2, which may contribute to the development of strong links between these two domains. However, depending on the relationship between the two forms (mediated by shallow vs. deep orthography) and their quality of encoding (i.e., how close they are to their optima), strong mappings between them might be advantageous or disadvantageous. It is a topic for future research to explore whether the mapping between phonological and orthographic forms is equally strong in L1 and L2, at least in cases when the involved domain representations are close to their optima in both L1 and L2.
To summarize, the development of mappings between different domains, and, especially, form-meaning mappings in L2 lexical representations crucially depends on the proper encoding in each corresponding domain and on the simultaneity of emergence of the representations that are to be linked. The optimum is achieved when all domains of a given lexical representation are reliably mapped onto each other.
4. Dimension of networks
Each lexical entry can comprise representations from the three domains, and each representation is interconnected with other representations of the same type. Each domain representation can thus develop its own, idiosyncratic network of connections to other representations. Together they constitute the phonological, orthographic, and semantic networks in the mental lexicon.
L2 lexical units can be connected with both L1 and L2 representations. In contrast to models of the bilingual lexicon (RHM, BIA+, Multilink), the OM focuses on the L2 networks, and is centered on individual lexical representations (i.e., explores the domain networks of one lexical entry). The model sees a word's lexical integration as a gradual ontogenetic process, in which connections to other representations grow in number and strength until the optimum is potentially reached. The optimum in this dimension can be described as an adequately rich network of appropriate connections. Fuzziness in this dimension then refers primarily to an inadequate number of connections to other representations (typically too few) and/or to their inadequate strength (typically too weak), as well as inappropriate connections (e.g., an erroneous connection between the phonological forms of through and dough due to the influence of orthography).
During acquisition, the ontogenesis of a word's lexical integration into a network proceeds in a unique way in each of its domains with respect to the quantity and quality of its connections, and its developmental trajectory (i.e., the start and pace of their acquisition). The important points of the developmental process include its onset relative to the onsets in other dimensions or domains, whether and when the optimum is reached and, potentially, its attrition (e.g., connections weakening due to reduced language use).
The number of network connections within an entry's domain depends not only on the individual representation, but also on the number of other representations in the network. At this point, the OM approach of viewing the networks from the perspective of a single lexical unit meets with other approaches that typically take the outer perspective and discuss the networks as wholes, e.g., a network of phonological forms. Research within this latter perspective reveals that emerging (here L2) networks are less dense than established (here L1) networks and that an increase in network density is linked to higher proficiency (for L2: Wilks & Meara, Reference Wilks and Meara2002, Reference Wilks, Meara, Daller, Milton and Treffers-Daller2007; Wilks, Meara & Wolter, Reference Wilks, Meara and Wolter2005) and especially to vocabulary growth (for L2: see Zareva, Reference Zareva2007). Due to its lower connectivity, the general level of fuzziness in an L2 network is higher (which contributes to reduced competition in L2 compared to L1, cf. Broersma, Reference Broersma2012). With increasing vocabulary size, this fuzziness is reduced – there are more opportunities for connections, and as a result each representation in the network needs better resolution in order to survive with a more densely inhabited and interconnected space leading to stronger competition (cf., for instance, Talamas, Kroll & Dufour, Reference Talamas, Kroll and Dufour1999).
Research based on word association (WA) tasks and priming studies indicates that the form network has a special status in L2. One of the oldest and most persistent findings of WA research shows that a word triggers associations on different linguistic levels. Regarding the organization of the L2 lexicon, many studies find that WA tasks trigger more phonological responses in (early) L2 (house-mouse), while in adult L1, WA tasks more often trigger cross-domain, i.e., semantic responses (e.g., paradigmatic: honey-bee; or syntagmatic: bird-fly) leading to a so-called form-prominence in L2 (Jiang & Zhang, Reference Jiang and Zhang2021; for an overview see Fitzpatrick & Thwaites, Reference Fitzpatrick and Thwaites2020). Morphological priming studies with an orthographic control condition supply further evidence for the special status of the form network in the L2 lexicon: they reveal reliable, purely form-based, orthographic priming effects in L2, while these effects are typically much weaker or missing in L1. For instance, Heyer and Clahsen (Reference Heyer and Clahsen2015) observe priming for purely form-related items (career-car) only in L2, while priming effects of the same size were found both in L1 and in L2 for morphologically/semantically related items (darkness-dark) (see also Li, Jiang & Gor, Reference Li, Jiang and Gor2017; however, for contrary findings see Diependaele et al., Reference Diependaele, Duñabeitia, Morris and Keuleers2011). Other research lines bring evidence for L2 form-prominence, too (e.g., eye-tracking study on text reading by Bordag, Opitz, Polter & Meng, Reference Bordag, Opitz, Polter and Meng2021).
From the OM's perspective, the described findings indicate several possible kinds of fuzziness that might be at play. For example, an L2 phonological representation might be (more strongly) connected to phonological forms that are irrelevant or less relevant in L1 (e.g., car and career or parent and parrot due to fuzzy phonological representations). In addition, the connection from an L2 form representation to other L2 form representations might be disproportionately strong relative to the weak mapping link between the form and corresponding semantic representation. As described in Dimension of mappings, a joint, simultaneous development of the phonological and semantic networks in L1 results in strong mapping between the representations in the two domains in L1, while the mapping is usually weak in L2 that develops with an already pre-existing semantic network. As a consequence, activation spreads more easily within the L2 form network than through the weak mapping link to the semantic network (the weak mapping link can serve as a bottleneck for activation to spread among other semantic representations). The activation that reaches the semantic network is thus reduced due to more diffuse spreading in the form network and the mapping bottleneck, which may result in weaker and/or limited in scope activation at the semantic level (cf. weaker sensory-motor effects in L2 compared to L1, e.g., Zhang, Yang, Wang & Li, Reference Zhang, Yang, Wang and Li2020).
Moreover, in WA studies, L2 participants produce L2 word forms that they can say/write down, while they may not have the corresponding L2 forms in their lexicon for the semantic representations they could possibly associate. Alternatively, the mapping link between the semantic representation and the corresponding L2 phonological form might be too weak to enable an easy form retrieval based on activation from the semantic network. Indeed, with growing L2 form network/lexicon, the number of paradigmatic responses increases and the proportion of phonological and cross-domain responses becomes more native-like (cf. Fitzpatrick & Thwaites, Reference Fitzpatrick and Thwaites2020). The so-called form prominence may thus be – at least to some degree – also a result of deficits/fuzziness in the form network: a word triggers propagation of activation throughout the shared semantic network that would result in native-like associations; however, there are no L2 phonological forms available for them. In general, these considerations suggest an explanation of the seemingly paradoxical situation that word forms and their networks have a prominent status in L2, though L2 speakers are not very efficient encoders of the word form (see Dimension of linguistic domains).
Importantly, the larger reliance on word forms in L2 is thus not mutually exclusive with the assumption that L2 learners use the same (well-developed) semantic network as in their L1 and thus have the same semantic base that contributes to the L1 speakers’ ability to provide cross-domain responses in WA tasks (i.e., the form prominence does not imply better developed form network compared to the semantic network). At the same time, the L2 difference between “pre-existent” semantic and “to be developed” phonological networks is not absolute (see Dimension of domains). In the case of cognates or even false friends, an existing form representation may be shared between languages and does not have to be established anew (Costa et al., 2000; Hoshino & Kroll, Reference Hoshino and Kroll2008). Moreover, recent research on closely related languages such as Czech and Slovak (Kříž, Reference Kříž2020) indicates that when a substantial proportion of the phonological lexicons overlaps, the existing phonological network is used also in L2 processing. Regular deviations between the languages are then handled by productive rules (e.g., “if ‘ú’ in Slovak, change it into ‘ou’ to make a Czech word”: pavúk → pavouk; ‘spider’). Or else, fuzzy L2 representations are created based on the L1 representations with variable settings for the original L1 phonemes/graphemes or their combinations that are not perceived as L2-like in a given phonotactic context (e.g., in Kříž’ experiments, Slovak participants would recognize the word slon ‘elephant’ as an L2 Czech word in a lexical decision task, but would produce it incorrectly as *slún or *slun in picture naming).
If a to-be-added lexical unit can employ an existing representation or a part of it to build upon, this has significant consequences for the slope of the ontogenetic curve, which then steeply rises. This holds not only for the ontogenetic curve of a given domain representation, but also for the ontogenetic trajectory of the network dimension: the identification of an existing semantic representation, to which a new L2 form is then linked, implies that the L2 lexical representation also inherits the semantic network associated with the given semantic representation.Footnote 10 As discussed already in the Dimension of linguistic domains, CLS-related research often shows early semantic engagement effects, while form engagement effects typically appear only after a period of consolidation. According to the OM, this difference arises due to the necessity to establish the phonological network around a newly added phonological representation, while the existing semantic network of the semantic representation (in L1) can be employed basically immediately. Bordag and colleagues (2015), for instance, show that incidentally acquired new words engage with semantically related representations after just three previous occurrences in texts and without any consolidation period, if they could be mapped on existing semantic representations. Similarly, when Bordag and colleagues (2018) manipulated the semantic content of new words in L2, they found (immediate) semantic facilitation in priming only for novel words that could rely on stable, earlier established representations (via L1) and not for novel words with recently established semantic representations (i.e., with novel meanings).
In most cases, however, the acquisition of a new word in L2 (at least in typically explored language pairs) comprises a mapping of a new L2 form representation on an already existing (more or less equivalent) meaning representation. In L2 acquisition, it is thus primarily within the form network that new representations have to be established and interconnected with other units.
In the above paragraphs, we focused on how a word form is connected to other word forms within the L2 form network and on its engagement within the semantic network. However, a new L2 form representation is connected not only to other, previously established, L2 form representations, but also to L1 forms. The OM thus differentiates between two subnetworks within the form network: an IntraNetwork and an InterNetwork. The IntraNetwork refers to the connections between a given L2 form and other L2 forms, as discussed above. The InterNetwork refers to cross-language connections, i.e., the connections between a given L2 form and L1 forms. Since OM is primarily a model of L2 representations, it is the IntraNetwork that is in the center of its focus and the InterNetwork is discussed only briefly.
The IntraNetwork and InterNetwork of a given form can differ in their robustness, i.e., number and strength of their connections. We assume that the robustness and thus the relative dominance of the IntraNetwork or InterNetwork depends strongly on the learning conditions and language use. For example, while incidental learning of words or vocabulary learning from definitions promotes the development of the IntraNetwork, vocabulary learning with the help of L1 translation equivalents or learning in settings where both languages are used promotes the development of the InterNetwork. Similarly, using the L2 only in a monolingual L2 setting promotes the development of the IntraNetwork, while translating or interpreting between two languages promotes the development of the InterNetwork.
In addition, teaching methods may also influence which types of InterNetwork connections are established. Vocabulary learning with translation equivalents promotes the correspondent connection between the two translations. On the other hand, the keyword method may promote connections to formally similar L1 forms. For example, a Czech learner of Hebrew might be encouraged to remember the Hebrew word goral, ‘fate’ by associating it with the Czech word horal, ‘highlander’ (since highlanders have hard fate). A method which might ease the encoding of the form representation might thus lead to non-native complexities in the network dimension potentially increasing the fuzziness and hindering fluency of processing (when trying to say the word fate in Hebrew, the Czech learner might – at least in the initial stages – need to make a detour and access the semantic representation of highlander and its phonological form horal to finally reach the phonological form goral).
The InterNetwork connections between the translation equivalents are highlighted especially by the RHM (but see also the Inhibitory Control Model, Green, Reference Green1998). Based on a series of translation experiments, Kroll and colleagues assume a strong link between an L1 form and its meaning, while the access to the meaning in L2 is primarily mediated via the L1 translation equivalent (in production) until the learner acquires sufficient skill in the L2 to access meaning directly (i.e., until a strong link between the L2 form and the corresponding semantic representation develops) (e.g., Kroll & Stewart, Reference Kroll and Stewart1994; Sholl, Sankaranarayanan & Kroll, Reference Sholl, Sankaranarayanan and Kroll1995, for a discussion see Brysbaert & Duyck, Reference Brysbaert and Duyck2010, and Kroll, van Hell, Tokowicz & Green, Reference Kroll, van Hell, Tokowicz and Green2010). Other models of bilingual processing, such as the Multilink model and its predecessor BIA+, assume that L1 and L2 form representations of translation equivalents are not connected directly, but mediated through a shared semantic representation.
The InterNetwork that is targeted by models and studies on bilingual lexicon transgresses the concepts of the OM that are primarily designed to handle the L2 lexicon. For example, it is unclear what could be seen as an acquisition optimum for the InterNetwork. On the one hand, the optimum as the subnetwork's complete absence would help to reduce interference and unintended code-switching that are clearly an unwished result of the L1-L2 interaction. On the other hand, it would also reduce positive transfer that might ease the acquisition. In addition, for e.g., interpreting and translating purposes such a state definitely could not be seen as optimal. Thus, it is also unclear which ontogenetic developments of the InterNetwork could be characterized as progress and which as deficits or regression. In terms of the concept of fuzziness, L1 interference could probably be considered to increase its level, but in the case of positive transfer it could also lead to a reduction of fuzziness. In general, the OM is not designed to handle the IntraNetwok aspects.
5. Conclusion
In the previous sections, we introduced the OM, a model of the development of a lexical representation in L2 that takes into account its complex multicomponent nature. Different domains develop at their own pace, depending on their properties, as they interact with the learning context, and more often than not they stay below their optimum. Inaccurate or low-resolution encoding in one or more domains produces fuzzy lexical representations. This fuzziness at the level of linguistic domains has a cascading effect on the robustness level of the mappings between different domains and the strength of connections between different lexical representations in the IntraNetwork as much as it is influenced by the links to other domains and other lexical representations in the networks. We have reviewed the findings reported in different strands of research on lexical acquisition and processing, and in particular, vocabulary training studies, to show that the OM has the potential to provide a generalized approach to the interpretation of the results that have been treated separately and to move us forward in building a model of L2 lexical acquisition and processing.
We should acknowledge that the OM is based on limited evidence in a few areas of research, and accordingly it invites further studies that would finesse the methodologies gauging the level of fuzziness in L2 lexical representations as opposed to processing difficulties, and explore the factors that mitigate the successful encoding of lexical representations and their integration in the lexical networks. These factors include the learning context (or the training conditions), as well as the individual properties of the lexical representation and the learner. Longitudinal studies would be especially welcome in the study of the ontogenesis of lexical representations; however, experiments targeting lexical configurations that are expected at particular ontogenetic stages under different scenarios would be equally helpful. In addition, some research areas, in particular neurolinguistic evidence, are not discussed in the current publication due to space reasons, although such evidence has been considered when developing the model. A direct inclusion of neurolinguistic findings in the model is on the agenda for future work. Moreover, since the model focuses on L2 representations, it does not address the bilingual aspects of processing that are the focus of other models (e.g., BIA+ and Multilink), such as L1 - L2 interactions or control.
The OM is a functional, theoretical model based on current empirical evidence that hopes to inform various areas of language study, among them: for example, computational modelling of language processing or language instruction. The OM also encourages research on yet unexplored aspects of L2 lexical representations: the relation between the ontogenetic curves for lexical representations (and their components) and the developmental trajectories for L2 proficiency, the role of language typology, the downward curve characteristic of attrition, and the properties of lexical representations in different populations of L2 speakers, including those with language deficits and age-related decline. In general terms, the OM fosters research into individual variation in lexical representations and their ontogenesis. Furthermore, the data invoked to illustrate the workings of the OM come from testing perception rather than production. It is important to understand what parallelisms and asymmetries the lexical processing in these two modalities involves, how they differentially contribute to the development of lexical representations, and which lexical properties are differentially engaged in production as opposed to perception.
Acknowledgments
The development of the model and the writing of this manuscript were supported by funding from the Slavic and East European Language Resource Center (SEELRC) at Duke University and from the German Research Foundation (Deutsche Forschungsgemeinschaft, DFG). We thank Bracha Nir for the initial creative impetus, Anna Chrabaszcz, Svetlana Cook, Harald Clahsen, Marc Brysbaert and two anonymous reviewers for their helpful comments on the earlier version of the manuscript and Emelie Wey for her assistance in preparing the manuscript for publication.
Competing interests
The authors declare none.