CLINICIAN’S CAPSULE
What is known about the topic?
Bed boarding is one of the major contributors to emergency department overcrowding.
What did this study ask?
What are the characteristics of patients with prolonged boarding times, and what are the impacts on patient-oriented outcomes?
What did this study find?
Patients who were older, sicker, and had isolation and telemetry requirements experienced longer boarding times, and longer inpatient length of stay even after correcting for confounders.
Why does this study matter to clinicians?
Organization-wide interventions to improve efficiency and flow are required to mitigate the burden of bed boarding.
INTRODUCTION
Over the past several decades, emergency department (ED) overcrowding has become a chronic, worsening problem in Canada and internationally. This overcrowding leads to a detrimental effect on patient care, ED efficiency, and patient and provider satisfaction. 1 , Reference Affleck, Parks and Drummond 2 Previous studies have shown a link between increased ED overcrowding and worse clinical outcomes, increased risk of in-hospital mortality, higher costs, and longer times to treatment.Reference Bernstein, Aronsky and Duseja 3 - 8
The cause of ED overcrowding is thought to be a combination of input, throughput, and output factors.Reference Bernstein, Aronsky and Duseja 3 , Reference Hoot and Aronsky 4 , Reference Villa-Roel, Guo and Holroyd 9 , Reference Asplin, Magid and Rhodes 10 Input factors encompass causes of patient inflow to the ED (e.g., non-urgent visits due to the lack of access to primary care, “frequent flyer” patients). Throughput factors are capacity-related issues or operational bottlenecks and inefficiencies within the ED (e.g., inadequate staffing or physical space). Output factors reflect bottlenecks or inefficiencies in other parts of the institution – or within the local healthcare and social system as a whole – that have upstream effects on ED operations and flow (such as bed availability within inpatient units).Reference Hoot and Aronsky 4 , 8
Within Ontario, roughly 10% of patients who present to the ED are admitted to the hospital. Recent data show a median time to inpatient bed for these patients of 3.7 hours and a 90th percentile time to inpatient bed of 23.3 hours (Figure 1). 11 , 12 Recently, there has been a great deal of interest within the public, the media, and the government on the issue of delays in the transfer of admitted patients out of the ED – otherwise known as bed boarding. Bed boarding is felt to negatively impact ED flow and efficiency, lead to adverse effects for the patients themselves, and is believed to be one of the chief drivers of ED overcrowding.Reference Affleck, Parks and Drummond 2 , Reference Hoot and Aronsky 4 , 8 , Reference Asaro, Lewis and Boxerman 13

Figure 1 Schematic representation of different metrics measured during the course of a patient’s ED journey.
The impact of ED wait times for admitted patients on clinically oriented outcomes has been explored in multiple studies.Reference Bernstein, Aronsky and Duseja 3 - Reference Liew and Kennedy 6 , Reference Singer, Thode, Viccellio and Pines 14 - Reference Richardson 16 However, there are few Canadian studies that have explored the characteristics, drivers, and patient-oriented outcomes associated with ED wait times for those patients admitted to the hospital but boarded in the ED while awaiting a bed on the inpatient unit.Reference Huang, Thind, Dreyer and Zaric 5 We seek to explore the nature and impact of prolonged ED wait times through a series of exploratory and regression analyses. Our study objectives are to 1) describe the patient-specific characteristics of boarded patients stratified by inpatient service, 2) identify the patient population with the most prolonged ED wait times, 3) within this patient population identify patient-specific characteristics associated with prolonged wait times, and 4) determine any association between prolonged ED wait times and two clinically relevant patient-oriented outcomes of a) inpatient length of stay (IP LOS) and b) inpatient mortality. Within our study, we use two definitions of ED wait times: 1) total ED length of stay (ED LOS) and 2) total boarding time (or time to bed [TTB]) after a decision to admit.
METHODS
Study site
Our centre is a very high volume community hospital, with >135,000 ED visits per year and a 13% admission rate for the 2015-2016 fiscal year. Ontario Ministry of Health data show that, for the 2015-2016 fiscal year, roughly 3.5% of our total ED patient population had ED LOS>24 hours, whereas roughly 24.9% of our admitted patient population had ED LOS>24 hours. 11 Within the subgroup of admitted patients, the median boarding time (i.e., TTB) was 5.2 hours, whereas the 90th percentile boarding time was 30.7 hours. 11
Study design and data collection
We conducted a retrospective single-centre observational study of patients admitted to a hospital inpatient unit through the ED during the period between January 1, 2015 and December 31, 2015. Patient data were accessed from electronic patient and administrative hospital records through our Decision Support unit. Collected variables included patient demographics and clinical characteristics (including telemetry requirements, isolation status, and comorbidity level), 17 - Reference Ghanny 19 ED wait times, IP LOS, and inpatient mortality. The comorbidity level (ranging from level 0 to level 4) is an index developed by the Canadian Institute of Health Information (CIHI) to measure a patient’s resource consumption during his or her hospitalization relative to that of other patients within the same major clinical category. Patients with higher comorbidity levels have higher relative resource consumption:
Level 0: the coded comorbidities on the episodes have no or <1.25 times impact on resource consumption.
Level 1: the coded comorbidities on the episodes have 1.25 up to 1.5 times more resource consumption.
Level 2: the coded comorbidities on the episodes have 1.5 up to 2 times more resource consumption.
Level 3: the coded comorbidities on the episodes have 2 up to 3 times more resource consumption.
Level 4: the coded comorbidities on the episodes have 3 or more times more resource consumption.
ED wait times comprised total ED LOS, calculated in hours from the time of triage to the time when the patient physically left the ED, and TTB, calculated in hours from the time of the decision to admit to the time that the patient physically left the ED for an inpatient bed (see Figure 1). Our study population included all patients admitted to the following admitting services: medicine, surgery, pediatrics, and critical care. The admitting service was determined by the unit of admission, as well as the admitting service as described by the coded hospital data. Patients who were admitted to an inpatient unit from the ED but were ultimately discharged from the ED (without having been physically transferred to an inpatient bed) were included for all analyses of total ED LOS, and were categorized under their admitting service. These patients were excluded from the analysis of TTB (boarding time) given that they never reached an inpatient bed.
Data analysis
The mean total ED LOS and TTB were calculated for each admitting service. Previous years’ trends (obtained from the Ontario Ministry of Health’s iPORT Access tool) with respect to ED wait times show that these wait times were highly right-skewed, and that mean or median wait times did not fully capture the experience of those patients with more extreme prolonged wait times. 20 We therefore performed further analyses on those patients with prolonged ED LOS and prolonged TTB for each service. Prolonged ED LOS was determined a priori as≥24 hours, and prolonged TTB was determined a priori as≥12 hours. Using logistic regression, odds ratios (OR) were calculated for prolonged ED LOS and prolonged TTB as a function of the patient’s admitting service using the surgical service as the reference.
Further exploratory analysis was conducted to identify the major predictors of prolonged ED LOS and TTB. Both within the literature and within our initial preliminary analyses, patients admitted to the medicine service experienced the greatest delays in transfer to inpatient beds.Reference Ye, Zhou and He 21 Therefore, we conducted these secondary analyses only within those patients admitted to medicine, to eliminate any confounders relating to admission service. We sought to determine the impact of patient age, patient comorbidity, requirement for an isolation bed or telemetry bed, and day of the week of patient registration on ED LOS and TTB. For patient age, we first conducted a linear regression analysis to determine the existence of an association between age and 1) ED LOS and 2) TTB. We then categorized patient age into four categories based on the quartile cut-offs of our sample population. Our age categories were ages less than 56, ages 56 to 69, ages 70 to 80, and ages≥81. Using logistic regression analysis, we calculated unadjusted and adjusted OR for prolonged ED LOS and prolonged TTB for each variable. Our adjusted model included all variables of isolation status, telemetry requirement, age category, and comorbidity level.
To determine whether prolonged ED wait times were associated with clinically relevant outcomes, we first used logistic regression to calculate OR for inpatient mortality as a function of both TTB and ED LOS for patients admitted to the medicine service. Secondly, unadjusted and adjusted mean total IP LOS were calculated for those with prolonged ED LOS and TTB versus those without prolonged ED LOS and TTB. One-way analysis of variance (ANOVA) tests were performed to determine the statistical significance of the difference in mean IP LOS among those with prolonged ED LOS and TTB versus those without. Further analysis using multivariate linear regression was conducted to adjust group means for comorbidity level, age category, isolation status, and telemetry requirements.
All analyses were conducted using SAS statistical software program version 9.4 for Windows, SAS Institute Inc. (Cary, North Carolina).
Ethics approval was obtained through the William Osler Health System Research Ethics Office.
RESULTS
The initial study population included 18,250 unique inpatient admissions. Of these, 13,872 admissions were to the four services of interest, and 4,319 admissions were to other services. Of the 13,872 admissions in the cohort, 1,053 (7.6%) admission events were ultimately discharged from the ED without reaching an inpatient bed. Fifty-nine admission events had missing data on admission unit or service. Descriptive characteristics of patients, total ED LOS, and total TTB by admission service appear in Table 1. The one-way ANOVA test showed a statistically significant difference between group means (p<0.0001) for mean ED LOS and mean TTB among patients admitted to the four different services. Patients admitted to Medicine had a mean ED LOS of 25.6 hours and a mean TTB of 15.9 hours. Pair-wise comparisons showed this difference to be significantly (p<0.0001) greater than ED wait times for patients admitted to the other three services (see Table 1). As well, there is a significant difference in odds of prolonged ED LOS and prolonged boarding time (i.e., TTB) as a function of admitting service, with patients admitted to medicine having a far greater odds of prolonged wait time in the ED:
OR (confidence interval [CI]) for prolonged ED LOS: medicine, 29.5 (23.2-37.4); critical care, 2.7 (2.0-3.7); pediatrics, 0.5 (0.3-0.7); and OR (CI) for prolonged TTB: medicine, 31.4 (25.5-38.7); critical care, 3.6 (2.9-4.7); pediatrics, 0.7 (0.5-1.0).
Table 1 Baseline characteristics of sample population
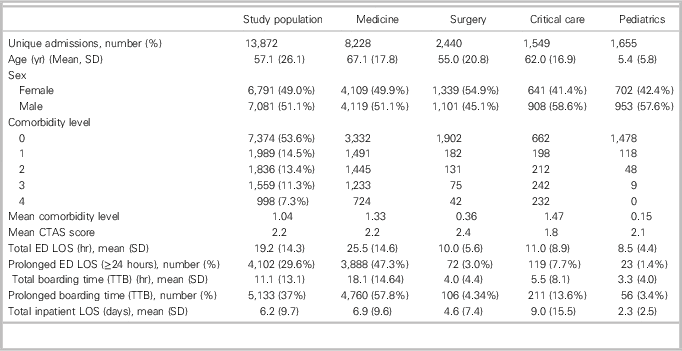
CTAS=Canadian Triage Acuity Scale; ED=emergency department; LOS=length of stay; SD=standard deviation; TTB=time to bed.
We found the distribution of wait times among the cohort of patients admitted to the four services to be highly right-skewed. Figure 2 shows the distribution of TTB for our population (the distribution of total ED LOS can be found in Supplementary Table 1 and Supplementary Figure 1). This figure shows that the total cumulative patient-hours of boarding time for all patients whose boarding times were greater than the median boarding time (N=6,936 patients) were nearly eight times higher than the cumulative patient-hours of boarding time of those patients whose boarding times were less than the median.

Figure 2 Distribution of boarding times (TTB), 2015.
When examining patients admitted to the medicine service, we found that the odds of prolonged wait in the ED (either as prolonged ED LOS or prolonged TTB) were significantly greater for those under isolation, for those under telemetry, for older patients, and for those with a greater comorbidity burden. The direction and magnitude of ORs for these patient-specific factors were similar for both exposure variables of prolonged TTB (Table 2) and ED LOS (see Supplementary Table 1). The day of the week of admission was also associated with significant differences in odds of prolonged ED wait times (both ED LOS and TTB) for those who presented to the ED on Sundays and Mondays (see Table 2 and Supplementary Table 1).
Table 2 Unadjusted and adjusted odds ratios for prolonged boarding time (TTB) as a function of patient characteristics
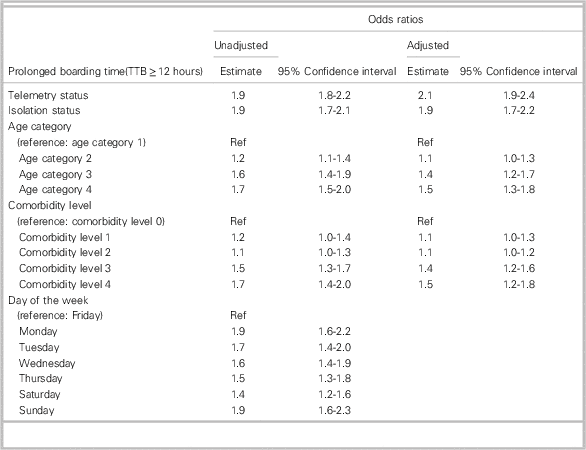
TTB=time to bed.
For those patients admitted to the Medicine service, the logistic regression analysis of the association between inpatient mortality and prolonged ED wait times revealed no statistically significant greater odds of mortality for those with prolonged ED LOS (OR: 0.9, 95% CI: 0.7-1.0) or prolonged TTB (OR: 0.9, 95% CI: 0.8-1.1). There was, however, a statistically significant greater mean IP LOS for those who had prolonged wait times in the ED (Table 3). This increased IP LOS and persisted even after correcting for age category, isolation status, telemetry requirements, and comorbidity level.
Table 3 Unadjusted and adjusted mean inpatient length of stay for patients with prolonged ED wait times
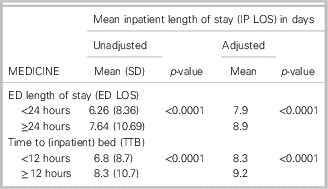
DISCUSSION
Findings of the most recent studies on the issue of ED overcrowding have challenged some of the conventional wisdom around root causes of ED congestion. With respect to input factors, it is commonly believed by both the lay public and, to some degree, hospital administrators and policymakers that ED overcrowding is partly due to lack of timely access to primary care. However, studies have in fact shown that the volume of low-complexity, low-acuity patients has a negligible impact on overall ED flow and operations.Reference Schull, Kiss and Szalai 22 - Reference Sprivulis, Grainger and Nagree 24 As well, improved access to primary care has not been consistently correlated with improved ED overcrowding metrics.Reference Nagree, Ercleve and Sprivulis 23 , Reference Thijssen, Kraaijvanger and Barten 25 , Reference Glazier, Zagorski and Rayner 26 For those patients with chronic ambulatory care sensitive conditions, a recent CIHI report showed that these patients represented a very small (4%) proportion of ED users, were judged to be of higher acuity than the overall ED patient population, and had a much higher rate of inpatient admission, suggesting that care within the ED is in fact very appropriate for such patients. 27 Studies have similarly found throughput issues to be a less significant barrier to ED flow than previously believed.Reference Asaro, Lewis and Boxerman 13 , Reference Khare, Powell, Reinhardt and Lucenti 28 - Reference Kheirbek, Beygi and Zargoush 32
In fact, current research suggests that hospital-wide issues – rather than factors internal to the ED such as ED staffing and resources – may be more important in determining ED efficiency and throughput. 8 , Reference Asplin, Magid and Rhodes 10 , 27 , Reference Innes, Grafstein and Scheuermeyer 33 - Reference Mustafa, Gilligan and Obu 36 Consequently, interventions that have had the most positive impact on ED congestion are those that target hospital-wide capacity and operations.Reference Villa-Roel, Guo and Holroyd 9 , Reference Viccellio, Santora and Singer 15 , Reference Innes, Grafstein and Scheuermeyer 33 , Reference Zocchi, McClelland and Pines 37 - Reference Brick, Lowes and Lovstrom 43
The TTB metric is an important adjunct to the ED LOS metric, because it reflects resource and capacity factors and operational issues that lie outside of the ED. The prolonged ED wait times of the more complex patients (such as those admitted to the medicine service) highlight the variability of wait times as a function of the patient’s resource utilization and burden of illness. In fact, within our study, the greatest mediator of prolonged ED wait times appears to be the admitting service. Patients admitted to the Medicine service wait far longer in the ED than patients admitted to other services. Even within Medicine patients, those with the greatest comorbidity burden and more specialized resource needs (i.e., those requiring a telemetry or isolation bed) are shown to wait the longest in the ED. A similar relationship between age and comorbidity and higher ED LOS was found in other studies.Reference Ye, Zhou and He 21 , Reference Brick, Lowes and Lovstrom 43 - Reference Moy, Coffey and Moore 45 However, to our knowledge, few studies have attempted to quantify the impact of clinical and operations-related factors such as admission service, telemetry requirements, and isolation status on ED wait times. These wide disparities in wait times, in part, are likely a reflection of the complexity of the patient population (with pediatric and surgical patients being less complex and having more predictable total inpatient lengths of stay, than those admitted to Medicine), operational issues impacting the different organizational structures within these services, and systemic challenges in addressing the needs of more medically complex patients. Given the variability in the evidence, recommendations and practice patterns around isolation and telemetry requirements for admitted patients, this presents an area for potential intervention by clinicians and hospital administrators through auditing their current admissions practices and adopting institution-wide policies and pathways that are more in line with existing capacity.Reference Chen and Hollander 46 , Reference Schull and Redelmeier 47
With respect to actual clinical outcomes, we found no statistically significant association between prolonged ED LOS and TTB and increased inpatient mortality. Within our sample population, those patients admitted to the medicine service were older, sicker, and had higher inpatient mortality rates than those admitted to the surgery and pediatric services. They also were disproportionately represented among those patients with prolonged ED LOS and TTB, confounding the association between ED wait times and inpatient mortality. Previous associations between inpatient mortality and increased ED LOS may have been driven in part by the confounding of admission service as a driver of both increased ED LOS (and increased boarding times) and increased inpatient mortality.Reference Singer, Thode, Viccellio and Pines 14 , Reference Richardson 16 , Reference Jo, Jeong and Jin 48 , Reference Sprivulis, Da Silva and Jacobs 49
Nonetheless, even when analysing only those patients admitted to the medicine service, and thereby eliminating the confounding effect of different admitting services, we found a positive correlation between increased ED wait times and greater total IP LOS. This association is likely multifactorial, reflecting both widespread organizational inefficiencies and wider health systems factors that impact the transfer of patients into the unit and discharge of patients out of the unit, in addition to any potential deterioration that the patient may suffer while waiting in a suboptimal ED environment. Finally, the finding of the extremely right-skewed distribution of boarding times is worthy of further exploration because it indicates that the issue of ED congestion and bed boarding is likely driven by a very small proportion of the total number of patients who present to the ED, and has implications for performance monitoring and process- and operations-level improvement.
Several limitations exist in this study. Firstly, it is a single-site study at a centre with a particularly high patient volume and consequent ED wait times. Other limitations include those endemic to observational studies of large databases, namely coding and measurement errors, effects of hidden confounders, and the identification of statistically significant but clinically inconsequential effects due to the large sample size. Finally, the variable of comorbidity level used in our study was developed primarily to provide a relative index of cost of resource consumption as a function of the patient’s comorbidity burden. The inclusion of “facility” and “age” into the CIHI comorbidity level regression model impedes generalizability and may lead to collinearity with the age category variable in our model. However, one major advantage of comorbidity level as an indicator of comorbidity burden is that it measures resource utilization (particularly within a Canadian context) as opposed to other indices of comorbidity (e.g., Charleson Comorbidity Index), which are used to predict mortality, and include only a small number of comorbid conditions.
CONCLUSION
In summary, the findings of our study show that bed boarding within our centre is highly unequally distributed and overwhelmingly concentrated in the medicine service. As well, those patients who have higher resource requirements tend to have greater boarding times, and these prolonged boarding times are associated with greater IP LOS. Finally, the highly right-skewed distribution of ED boarding times indicates that ED overcrowding and congestion are likely greatly driven by a relatively small proportion of the total number of patients who visit the ED. These findings – while requiring further exploration and research – highlight the pitfalls of more universal, untargeted interventions to resolve the issue of ED overcrowding.
Acknowledgements
The authors would like to thank Shaun Ghanny (Manager, Decision Support, WOHS), Desa Marin (Manager, Decision Support, WOHS), Craig Homan (Case Mix Team, CIHI), Ravi Bhargava (WOHS Research Office), and Vibeeshan Jegatheeswaran for their contributions to this manuscript.
No external funding was secured for this study. Institutional support was provided by William Osler Health System, McMaster University Department of Medicine, and the University of Toronto, Department of Family and Community Medicine.
Competing interests: None declared.
Supplementary materials
For supplementary material/s referred to in this article, please visit https://doi.org/10.1017/cem.2018.18