1. Introduction
Rapid aging of populations across the developed world presents a challenge in delivering care to and maintaining quality of life for elders. Recent technological developments provide an avenue towards addressing this gap. According to the World Health Organization, the proportion of the global population which is elderly (i.e., aged
$\geq$
60 years) will nearly double between 2020 and 2050 from 12% to 22%. By 2050, the number of individuals aged over 60 years is expected to reach 2.1 billion [1]. As the proportion of the population which is elderly expands, so does the demand for rehabilitation and social care, which play an important role in maintaining function and mediating motor and cognitive decline in older adults. Today, more older adults are aging in independent living arrangements than have historically, resulting in increased loneliness and social isolation. In 2020, about 27% (14.7 million) of all older adults in the USA lived alone [2].
At the same time, there are long-standing shortages of therapists and other clinicians in developing nations and growing shortages in developed nations, especially in rural areas [3–Reference Al-Shamsi10]. This supply-demand imbalance poses a particular challenge for patients who are in the sub-acute or chronic phases of an injury which has led to impairment. For example, in the United States, about 795,000 patients every year suffer from stroke and an estimated 7.6 million people over 20 years old living post-stroke [Reference Tsao, Aday, Almarzooq, Alonso, Beaton, Bittencourt, Boehme, Buxton, Carson, Commodore-Mensah, Elkind, Evenson, Eze-Nliam, Ferguson, Generoso, Ho, Kalani, Khan, Kissela, Knutson, Levine, Lewis, Liu, Loop, Ma, Mussolino, Navaneethan, Perak, Poudel, Rezk-Hanna, Roth, Schroeder, Shah, Thacker, VanWagner, Virani, Voecks, Wang, Yaffe and Martin11]. After discharge from acute care, 50–80% of stroke survivors have impairment which may manifest as limited upper extremity range of motion or strength, spasticity, challenges with gait, aphasia, etc. [Reference Lawrence, Coshall, Dundas, Stewart, Rudd, Howard and Wolfe12]. Rehabilitation for any of these impairments requires significant amounts of both patient and therapist time and effort.
Challenges to care delivery and social connectedness have been exacerbated during the Coronavirus Disease 2019 (COVID-19) pandemic, which has led to lockdowns and limitations of physical and social contact. The pandemic has limited physical interactions between older adults and their caregivers, physicians, physical therapists, etc. In addition to the direct loss of care, loneliness, and abandonment (due to a loss of social contact) in older adults can also lead to the development of several health conditions such as cognitive impairments [Reference Holwerda, Deeg, Beekman, van Tilburg, Stek, Jonker and Schoevers13], coronary heart diseases, and stroke [Reference Valtorta, Kanaan, Gilbody, Ronzi and Hanratty14]. For portions of the pandemic, outpatient rehabilitation centers, including those serving geriatric populations, closed and most health institutions which did remain open limited outside visitors. This led to elder care staff becoming increasingly burdened. Disruptions have limited access to rehabilitation facilities, group activities, and periodic clinical assessments and interventions, negatively affecting the motivation of patients for their rehabilitation and physical functions [Reference Reddy, Resnik, Freburger, Ciolek, Gifford, Whitten and Baier15]. To be effective in driving convalescence, rehabilitation requires a great deal of repetition, consistent involvement, and interactions over a long period of time.
Given these challenges, there is a clear need to develop strategies to continue offering rehabilitation services and social interactions to address both changing demographics and living situations and possible unforeseen circumstances, such as the complete social isolation during the COVID-19 pandemic.
1.1. Telepresence systems
Telehealth has been used progressively in the healthcare sector for decades [Reference Nesbitt16], utilizing telepresence technology such as videoconferencing, instant messaging, phone calls, and telepresence robots to maintain communication between patients and providers, and extend the geographic reach of providers. The first quarter of 2020, corresponding to the onset of the pandemic, witnessed a 50% growth in the number of telehealth encounters, compared to the first quarter of the previous year [Reference Koonin, Hoots, Tsang, Leroy, Farris, Jolly, Antall, McCabe, Zelis, Tong and Harris17]. Telehealth enables patients to receive healthcare remotely, bypassing barriers to care, such as travel, allowing for more encounters with clinicians and, as a result, better patient outcomes and continuity of care. Telehealth can also serve as a platform for mental online health services, which can mitigate the burden of mental health issues, such as burnout, depression, and anxiety, due to patient isolation and loneliness [Reference Zhou, Snoswell, Harding, Bambling, Edirippulige, Bai and Smith18]. While the necessity for telehealth in rural towns and other resource-limited places having deficient access to local practitioners is frequently emphasized, the recent pandemic produced by the contagious COVID-19 virus has highlighted the potential and importance of telehealth more broadly [Reference Latifi and Doarn19].
Telehealth can be used to deliver neuromotor rehabilitation remotely. Previous studies have described the experience of therapists with telerehabilitation systems to facilitate interventions such as exercise, stretching, and regular assessments of function as well as social interactions with older adults [Reference Chumbler, Quigley, Li, Morey, Rose, Sanford, Griffiths and Hoenig20–Reference Dodakian, McKenzie, Le, See, Pearson-Fuhrhop, Quinlan, Zhou, Augsberger, Tran, Friedman, Reinkensmeyer and Cramer22]. Telemedicine appointments, which can be used as a tool to improve patient experience, were also shown to facilitate the evaluation of motor function and range of movement in patients with arthroscopic orthopedic knee surgery [Reference Abel, Baldwin, Chuo, Ganley, Kim, Wells and Giordano23]. In a randomized controlled study, telehealth-based physical training visits were tested with 143 patients undergoing total knee arthroplasty and compared to traditional physical therapy with 144 patients. The telerehabilitation program demonstrated comparable clinical outcomes and patient engagement to usual care, while reducing the rehospitalization rates and leading to lower healthcare costs [Reference Bettger, Green, Holmes, Chokshi, Mather, Hoch, de Leon, Aluisio, Seyler, Del and Gaizo24]. Patient engagement and motivation to do prescribed exercises at home was reported to be improved by frequent interaction with clinicians over telepresence [Reference Dodakian, McKenzie, Le, See, Pearson-Fuhrhop, Quinlan, Zhou, Augsberger, Tran, Friedman, Reinkensmeyer and Cramer22].
Although telehealth has become ubiquitous and well-perceived in clinical settings [Reference Almathami, Win and Vlahu-Gjorgievska25], it is not without limitations. Almathami et al. described the potential barriers to telehealth-based home online consultation, including internal factors like poor body language and communication due to the lack of physical contact, negative perceptions by patients of telehealth privacy and security, and possible technical challenges such as network latency, Internet disruption, and difficult to use systems [Reference Almathami, Win and Vlahu-Gjorgievska25]. Other limitations of the technology are limited field of view, low display screen resolution, and the projection of three-dimensional movement and space into two-dimensional screen-based representations, which affect the patient’s perception of the presence of the therapist and decrease spatial reasoning for both participants [Reference Johnson, Rae, Mutlu and Takayama26, Reference Sirkin, Venolia, Tang, Robertson, Kim, Inkpen, Sedlins, Lee, Sinclair, Campos, Graham, Jorge, Nunes, Palanque and Winckler27]. These barriers might lower patient motivation and compliance, leading them to avoid telehealth, which, when no other alternative exists, could negatively impact care outcomes.
1.2. Socially assistive robots (SARs)
Another technical area generating excitement as a tool to fill gaps in care is socially assistive robotics. Socially assistive robots (SARs) have been developed to assist individuals with disabilities, provide platforms for social interventions, and promote interactive engagement [Reference Feil-Seifer and Matarić28]. The most prominent benefit of using SARs over various virtual interaction tools is the physical presence inherent in a robot. When compared to engaging with a virtual robot, Kiesler et al. found that people who interact physically with a robot are more engaged and follow instructions better [Reference Kiesler, Powers, Fussell and Torrey29]. According to Bainbridge et al., robots having a physical presence for interactions are crucial for the user’s trust and motivation, particularly for activities that entail discomfort [Reference Bainbridge, Hart, Kim and Scassellati30]. By allowing users to participate in various types of social engagement both within and outside the care centers, social robots might combat both emotional and social loneliness in assisted living [Reference Pirhonen, Tiilikainen, Pekkarinen, Lemivaara and Melkas31].
Several social robots have been developed for upper extremity rehabilitation in older adult patients [Reference Kachouie, Sedighadeli, Khosla and Chu32]. For example, Bandit, a humanoid social robot, is designed to promote upper extremity physical exercise in older adults autonomously, using a computer vision system [Reference Tapus, Tapus and Mataric33]. Bandit has also been tested for different tasks, including delivering social aid, playing cognitive games, and encouraging physical therapy. During exercise sessions, the robot performs upper-limb motions and encourages imitation from patients. In testing, older adults have engaged well with the robot, performing tasks at a high level across interactions and have rated the entire system as enjoyable and useful and the humanoid robot as helpful, socially attractive, and a good companion [Reference Fasola and Matarić34].
Beyond physical rehabilitation, development is also focused on cognitive and psychological care. Systems have been built and tested to treat patients with stroke, mild traumatic brain injury, and dementia [Reference Tapus, Tapus and Mataric33, Reference Matarić, Tapus, Winstein and Eriksson35].
1.3. Social robot-augmented telepresence
Advances in social robotics and telepresence are each intriguing but have limitations. To address challenges with classical telepresence for rehabilitation, we propose social robot-augmented telepresence (SRAT). In SRAT, a social humanoid robot is combined with a standard telepresence system (screen, camera, microphone). Using a SRAT system, a healthcare provider could connect with patients remotely and leverage the humanoid robot to lead and/or monitor exercises. The central theses of such an approach are that:
-
1) the social robot, by being physically present, could motivate patients and communicate physical activities with patients in a way that cannot be done via a screen alone,
-
2) having the clinician’s expertise within interactions is critical to maintaining high-quality care,
-
3) robots alone, without clinician control, are not yet ready for deployment in challenging environments, and
-
4) neither clinicians nor patients are yet prepared to hand over care to a robot without direct, active clinician oversight.
Combining telepresence and social robotics is not a new concept. However, what we propose, to maintain the operator and the social robot as separate social entities, does appear to be novel. Other systems, such as Telenoid, a telecommunication robot with a humanoid torso and a soft outer skin material, act as conduits for the operator, rather than adding a new social entity to interactions. Telenoid has nine degrees of freedom and can perform activities such as communication, looking around, and hugging, by synchronizing with the operator’s motions. This proved an effective method of interaction, results show that Telenoid was able to induce positive impression in the elderly with mild dementia during active communication, and in those suffering from severe dementia during physical interaction [Reference Kuwamura, Yamazaki, Nishio and Ishiguro36, Reference Sorbello, Chella, Calí, Giardina, Nishio and Ishiguro37].
To evaluate the potential of SRAT, we developed an exemplar system, Flo, which was previously known as Lil’Flo (Fig. 1) [Reference Sobrepera, Lee and Johnson38]. The Flo system is used throughout the remainder of the trial as a tool to test SRAT.

Figure 1. Flo, an example of a social robot-augmented telepresence system.
1.4. The use of social robots in long-term deployments
In general, deploying social robots for long-term use in any situation is often perceived as challenging, due to several factors such as logistics, maintenance, quality requirements, etc. Specifically for social robots whose interactions with users are important, one of the challenges is that there might be a novelty effect. Although SARs can be designed with appealing appearance and have been shown to produce enjoyment and benefits in short term, after the novelty effect wears off, the interest of users in using SARs in long-term interactions might decrease. This can cause a decay in users’ perception and interaction with robots [Reference Céspedes, Raigoso, Múnera and Cifuentes39]. To maintain engagement over the long term, it is critical that SARs are functionally relevant to users [Reference De Graaf, Allouch and Van Diik40]. Additionally, environments in which robots are deployed can be unstructured and sometimes include unpredictable changes, which challenge the long-term interaction between robot and people. Even harder still is deploying them in healthcare environments where requirements for data safety, infection prevention, and hardware safety can pose challenges to researchers. As a result, not many studies deploy social robots in the wild in long-term healthcare use. Those studies focused on long-term deployments in healthcare settings, with repeated interactions, stand out and we take inspiration from them.
In recent studies, a patient-robot interface was developed for cardiac rehabilitation, including a sensor interface to monitor physiological parameters of a user during exercises on the treadmill, and a robot module incorporating a NAO robot delivering interactions with the user, and aiming to provide motivation support, performance monitoring, online feedback, and warning [Reference Céspedes, Irfan, Senft, Cifuentes, Gutierrez, Rincon-Roncancio, Belpaeme and Múnera41, Reference Casas, Senft, Gutiérrez, Rincón-Rocancio, Múnera, Belpaeme and Cifuentes42]. Addressing the long-term effect of a socially assistive robot, the authors conducted a controlled 18-week longitudinal study. The long-term effects were proved by the lower rate of dropouts in robot-assisted group comparing to control condition and the maintenance of patient-robot interaction throughout the program. The same group of researchers also combined NAO robot and Lokomat gait therapy in a 15-session rehab program. Similarly, positive perception and improvement of gait performance were found, as the effect of cognitive support provided by the social robot [Reference Céspedes, Raigoso, Múnera and Cifuentes39]. The presence of social robot during therapies can facilitate physical distancing and promote the continuity of rehabilitation during the pandemic.
Pulido, et al. deployed the NAOTherapist with eight pediatric patients (six with obstetric brachial plexus palsy, two with infantile cerebral palsy) in a longitudinal study [Reference Pulido, Suarez-Mejias, Gonzalez, Ruiz, Ferri, Sahuquillo, Vargas, Infante-Cossio, Calderon and Fernandez43]. Participants completed on average 12 half-hour sessions with a social robot in which they played a pose mirroring game and a pose repetition game (the robot showed a sequence of poses which then had to be repeated). The system was programmed by a therapist to operate autonomously with their own patients, under their supervision. The therapist was able to modify the activities to suit the patient, but not able to move beyond the programmed activities. The therapist and two physiatrists rated the system as having high utility and responded positively in open-ended questions.
Feingold-Polak, et al. described an even larger study with 11 post-stroke patients who completed a total of 306 rehab interactions with a social robot over a 5–7 week period [Reference Feingold-Polak, Barzel and Levy-Tzedek44]. The social robot autonomously directed subjects through a variety of gamified ADL (activities of daily living) focused therapies. Subjects were positive on the interactions across a variety of metrics.
However, our unique proposed use of social robots, to augment telerehab interactions, provides an interesting opportunity for a different focus in a longer-term study. Our system enables us to focus on how the therapist uses the robot, because the robot is controlled in real time by the therapist and they have significant flexibility to change how it is acting, we can observe how they use the system, how their patients respond to it, and how they feel about using it.
This study will also represent the first time, to our knowledge, testing the idea of SRAT over multiple interactions with either patients or clinicians.
1.5. Objectives
The primary objective of this pilot study was to understand how clinicians and patients would use a novel SRAT system during rehab interactions in a natural environment. We sought to understand both the modes of interaction and the response to the interactions.
Specifically, we evaluated:
-
1) How do clinicians choose to use the system?
-
2) How well do patients react to the system?
-
3) How useful do patients and clinicians find the system?
-
4) What challenges arise while using the system?
2. Methods
This study was approved by the University of Pennsylvania Institutional Review Board. The Trinity Health Mid-Atlantic Institutional Review board deferred to the University of Pennsylvania for ethics review.
2.1. Robotic system – flo
The robotic system is comprised of a Kobuki drive base, two RealSense D415 cameras, a screen, speakers, a microphone, and a fisheye camera. The base houses an Intel NUC with a solid-state drive and 16 gigabytes of RAM, on which the entire system runs. A humanoid robot, with a head, face, torso, and arms, is attached to the mobile telepresence system to augment telerehabilitation encounters (Fig. 2a). The humanoid incorporates the chest of the XYZ Robotics Bolide robot, an commercialized edutainment robotics platform, along with motors and a control board (Fig. 2b). These motors are housed by a custom exoskeleton and are serially controlled and interfaced with the Robot Operating System (ROS), allowing for the real-time control and capture of the humanoid’s arms in ROS. The humanoid’s arms can perform movements and gestures to promote interactions. The humanoid can speak, using AWS Polly to synthesize speech, controlled by the operator or automated within games [Reference Sobrepera, Lee and Johnson38]. The face on the humanoid, which uses LED matrices, can be changed to alter the emotional state it is communicating [Reference Sobrepera, Kina and Johnson45]. Regarding the height of the robotic system, the middle of the humanoid’s face, the middle of the screen, and the top of the robot are about 72, 90, and 132 cm, respectively. The system is controlled remotely via a web interface (Fig. 3) using a plays and scripts model.

Figure 2. (a) A close-up shot of the Flo humanoid robot. (b) The computer-aided design of the humanoid with a cutaway to show the internals of the arms, torso, and head. It exposes the orientation of the motors, the internals of the head, and the LED matrices. The pictures are used with permission from the authors of [Reference Sobrepera, Lee and Johnson38].
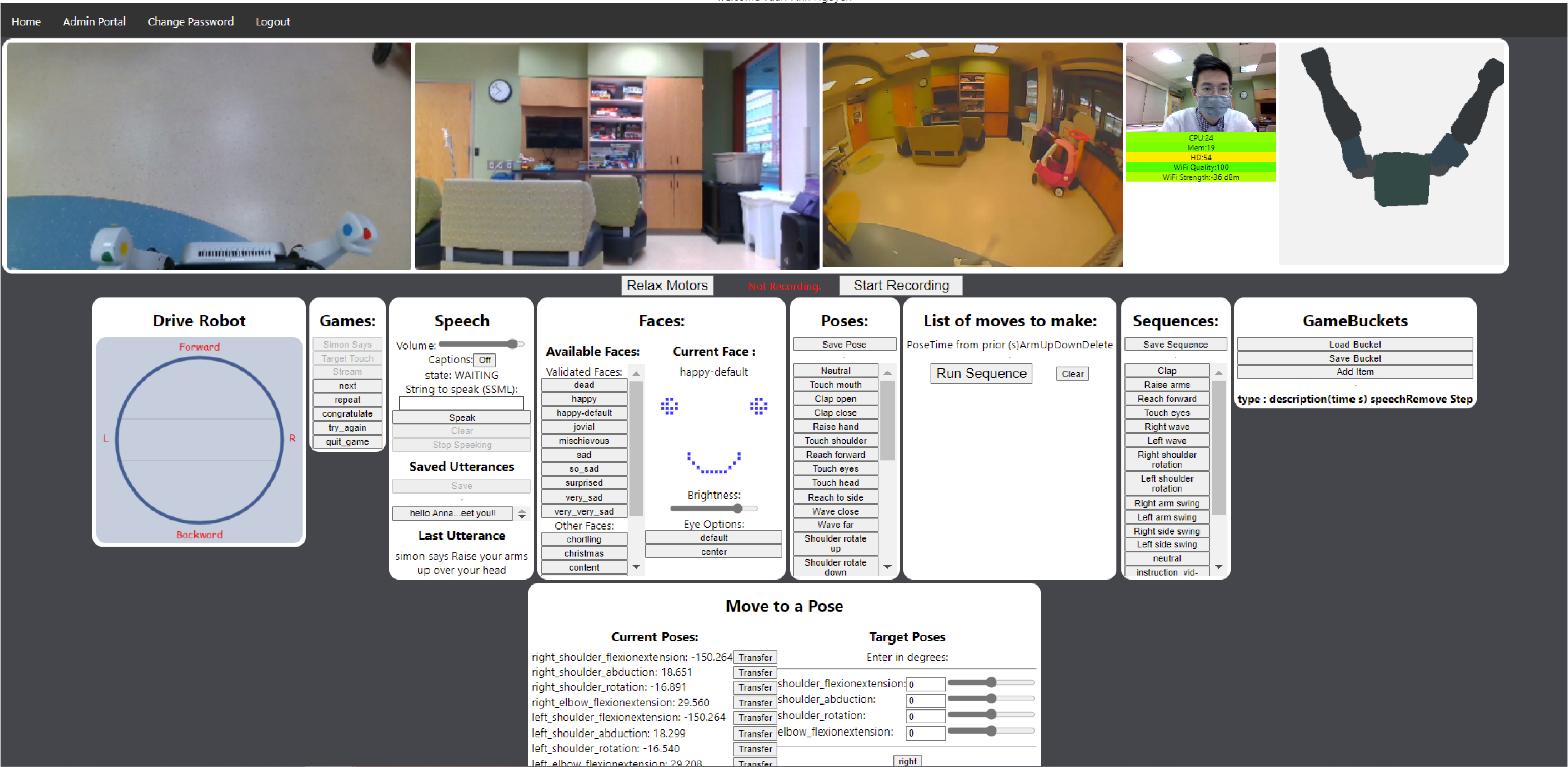
Figure 3. The web interface for operating the robot. On the top from left are the three video feeds from the robot, the operator’s video, system stats for the computer on the robot, and a 3D model of the humanoid’s position. In the middle from left are modules for driving the robot, running the games on the robot, commanding the robot to speak and monitoring what it is saying during activities (also heard over the audio feed), controlling the robot’s face, recording poses and inserting them into sequences, manipulating and running sequences, saving sequences, and constructing game buckets. On the bottom row is a module for directly viewing the humanoid robot’s joint angles and controlling them.
A clinician can control the system using any computer with a Chromium-based web browser and a webcam. There are two primary games included in the system, a “Simon says” game and a target touch game. They are both run from “game buckets,” collections of poses and sequences of poses, along with speech commands, which can be randomly ordered and executed by either game engine to produce activities which are unique at runtime. The operator can manually drive the robot, speak through the speaker, make the humanoid speak in its own voice through the speaker, change the humanoid robot’s face, make the humanoid robot move its arms to prerecorded poses, make the humanoid robot move through pre-arranged sequences of prerecorded poses, record new poses, arrange new sequences, play a “Simon says” game, play a target touch game, and set up new buckets for use in the games. The operator can see four video feeds, straight ahead (Fig. 4A), facing down (Fig. 4B), a fisheye camera, and their own video which is shown on the mobile telepresence system (Fig. 4D).

Figure 4. A therapist operating the SRAT system and presenting rehabilitation interactions with a patient: A) Camera facing forward towards the patient, B) Camera facing downward (for close-up interactions), C) Third-person camera recording the whole space where the interaction took place, D) Camera on operator’s computer to record the remote operator. Subjects provided media releases for publication of images.
In the “Simon says” game, the robot first reads out instructions to the patient, telling them that they should do what the robot does, mirrored, when and only when the command begins with “Simon says.” The robot then guides the patient through a series of poses and dynamic movements, instructing them both verbally and by arm motions. The commands from the game bucket are randomly combined using left and right arm actions to create unique bi-manual motions each time the activity is executed.
For the target touch game, the robot has two colored dots on each hand, one which can be seen when the robot’s hand is down (lower dot) and one when it is up (upper dot). The robot tells the patient that they will be told a sequence of colored dots to touch and which of their hands to use for each dot. For each set of points, the robot performs a pose which shows two dots to the subject (one on each of the robot’s hands) and delivers instructions for a sequence of between one and four touches, randomly selecting which subject hand/dot sequence to use and how long the sequence should be. A sample instruction prompt for a set of points is “Left hand red, right hand yellow,” which asks the subject to touch red dot using left hand and touch yellow dot using right hand. The robot then tells the subject to go. This is continued, with the arms moving and new sets of point/hand sequences being randomly generated, until the lower dots are touched three times each, using the patient’s arm of the same side, and the upper dots have been touched three times each for each of the patient’s hands, for a total default of eighteen touches. The parameters for the range of number of touches per set as well as total number of touches per dot can be set by the operator.
In surveys of 351 practicing therapists in the United States, therapists reported that they believed SRAT would significantly improve communication, motivation, and compliance during telerehab interactions when compared to traditional telepresence [Reference Sobrepera, Lee, Garg, Mendonca and Johnson46]. Building on the reported perceived utility of SRAT, in this study, Flo, an exemplar SRAT, was deployed in a geriatric care and rehabilitation setting. Therapists operated the robot with their existing patients, who have varied levels of cognitive and motor function. This study was designed to be an uncontrolled study in which natural interactions could develop. Clinicians and patients had the latitude to define their own interactions. We will therefore report our findings and observations. The hope is that the reader will gain increased insight into what may and may not be feasible with such a system. Reports of usability metrics and how the robot was used will hopefully highlight future design directions. Reports of acceptance metrics will clarify whether this direction of development is worth pursuing. Initial trends in perception of the system over multiple interactions by therapists and among different classes of patients should be interesting to the reader excited about further developing this new space.
2.2. Experimental procedure
This pilot study took place at a Program of All-inclusive Care for the Elderly (PACE) center. Patients in the center receive the full spectrum of care in one place, including primary care, rehabilitation, and eye care as well as social interaction with staff and other patients. All of the patients live in the community and are transported to the center by a fleet of buses for anything ranging from as needed to daily care. Patients treated by the center have a wide range of levels of function and diverse sources of impairment. Trials with patients took place between May 17, 2021, and June 03, 2021.
2.2.1. Pre-trial testing
Prior to the trials, the robot was brought to the clinical site, where it was tested in several possible trial locations. The quality of Wi-Fi signal and navigability was assessed. During these tests, it was observed by a member of the clinical team that the robot was not loud enough for patients treated at the center, so the speaker system on the robot was upgraded.
A pilot trial was completed using the robot with subjects in a laboratory environment to refine the system and interactions which could be completed with the robot [Reference Sobrepera, Lee, Garg and Johnson47].
2.2.2. Subject recruitment and selection
Our goal was to recruit patients to complete a total of twenty-five interactions with the robot. Therapists also needed to be recruited to operate the robot.
A member of the research team presented the project to physical therapists at the clinical facility. Several therapists expressed interest in participating and felt that their patients would qualify.
Potential patients were identified with the help of the staff and rehab teams at the clinical facility and were all the existing patients with the therapists who operated the robot in the study. Patients were required to be able to sit on their own or with the help of an assistive device, but without the help of a person and had to have at least partial voluntary upper-limb function and be able to follow simple two-step instructions.
2.2.3. Clinician consenting
At the time of the trial start, two physical therapists and one therapy assistant were available to participate. Consent to participate was gained from all three.
2.2.4. Subject consenting
From subjects who were able to give consent, a member of the study team gained consent. For subjects not able to provide consent, their representatives were contacted, and consent was gained remotely using a REDCap e-consent while on a phone call with a study team member. Assent was then gained from the subject by a member of the study team, in person.
Both the subjects and representatives (when appropriate) were able to agree to one or both of an optional release to use images and videos from the trial in publications, presentations, and other media and an optional release to use data collected for future research.
2.2.5. Pre-training survey
Prior to training to use the robot, the therapists took a survey (Table III (see Supplementary material)). This survey asked several questions about the therapists’ prior experience with various forms of technology, their experience doing various tasks by telepresence, how they feel about telepresence, demographic information, and whether they were excited to use the system they were about to be trained on.
2.2.6. Clinician training
Prior to the interactions with patients, the clinician participants were trained to use the system. They began by watching a short training video for operating the robot (https://youtu.be/mcdf0Qx6ujs). They then demonstrated basic competency with the system’s functions, following a training sign off, which required them to login to the system, drive the robot out of the room and back, say a new phrase with the robot, say a recorded phrase with the robot, use the recorded poses on the robot to make the left arm extend in front of the robot, use the recorded sequences to make the robot wave, record a new pose on the robot by relaxing the motors and positioning the arms manually, manually move the arms using angle commands, make a new sequence with 3 or more recorded poses using both arms, create a new game bucket with at least 3 items, turn on and off recording, and disconnect from the robot and reconnect. Throughout training, the therapists were free to ask a team member any questions.
2.2.7. Post-training survey
After the training session, the therapists were asked to fill out a usability survey. The survey is shown in Table IV (see Supplementary material). The survey had a question about excitement to use the system as well as six questions from the NASA Task Load Index (TLX) [Reference Hart and Staveland48] to determine the physical and cognitive load of the clinicians while training on the robot. (Cognitive load is an important metric in usability.) There were then two sets of questions comparing interactions with the Flo system vs. classical telepresence (CT) and between the Flo system and in-person interactions along seven axes of interest: communication between the therapist and patient, patient motivation, patient compliance, patient adherence, patient enjoyment, patient understanding, and the therapist’s ability to assess the patient.
2.2.8. Robot interaction with patients
Patients were guided to an unused activity room in the center by one of the therapists or a study team member. They were seated in an independent chair or in their wheelchair in an open space. Prior to the patients’ arrival, the robot was present on the edge of the room in a sleep state with a system information display on the screen of the robot and a sleeping face on the humanoid. The study team member activated recording cameras and left the area for an adjacent room/hallway.
The clinician, from a separate room (their office/cubicle), logged into the robot and drove it up to the patient. The clinician introduced the robot and proceeded with an interaction (Figs. 4 and 5). The clinicians were asked to use at least one of the two pre-programmed activities; either the “Simon says” game or the target touch game. Beyond this requirement, the clinicians were free to use the robot in whatever way they felt best for their patient, for however long they felt appropriate, and could blend conversation with themselves with conversation from the humanoid via the synthesized voice to help their patients achieve their rehab goals.

Figure 5. Example interactions between patients and the humanoid robot controlled remotely by therapists. Subjects provided media releases for publication of images.
2.2.9. Video data collection
During every experiment, video was recorded (Fig. 4). Two cameras on the robot recorded video, one forward facing for interactions, like “Simon says,” which occur at a distance, and one downward facing, for close-up interactions, like the target touch activity. The video from the remote operator was also recorded. The audio was also recorded, along with data on the robot’s operations. During most trials, a third-person camera also recorded video (Fig. 4C).
2.2.10. Survey data collection postrobot interaction
Patients. After each interaction, the patients completed a survey on the interaction. The survey was completed on an iPad using REDCap. A study team member assisted the subjects in completing the surveys. The post-interaction survey can be seen in Table V (see Supplementary material). The questions on mental demand, physical demand, performance, effort, and frustration were drawn from the NASA TLX. The questions asking how well the subject understood what they should do, if they wanted to have the interaction again, how safe they felt during the interaction, and how much they enjoyed the interaction are all custom designed for this survey based on prior experience of the authors [Reference Johnson, Sobrepera, Kina and Mendonca49] and modified from the Self-Assessment Manikin [Reference Bradley and Lang50]. The second block of questions in Table V (see Supplementary material) is all drawn from the Intrinsic Motivation Index (IMI) [Reference Ryan51–Reference Plant and Ryan53]. Selected questions were used based on a previous pilot study with a similar patient population. The normal IMI scale of seven elements was reduced to five elements. In previous testing, seven elements have been shown to be confusing to subjects. The questions on likability of Flo are drawn from the Godspeed Questionnaire number 3, likability [Reference Bartneck, Kulić, Croft and Zoghbi54]. For the Godspeed questions, the interviewer explained to the subject that they were being asked to discuss the humanoid portion of the robot (the robot was given different names by different subjects).
Clinicians. After a clinician completed all the robot interactions that they were doing in one day, they responded to a usability survey. The end of day survey can be seen in Table VI (see Supplementary material). The first five questions of the survey are drawn from the NASA TLX, followed by an added question in the same style on enjoyment. These are followed by six custom questions which are designed to understand the quality of the interaction between the patients and therapists along specific axes important to high-quality rehab. Surveys were completed by the clinicians using a web survey served by REDCap, at their convenience. At the conclusion of the study, the clinicians received a final survey to complete at their convenience, the text of which can be seen in Table VII (see Supplementary material).
2.2.11. Chart information
Chart information was collected for each subject. The following were collected: age, gender, diagnoses, reason for treatment, cognitive assessment results (if available), motor assessment results (if available).
2.3. Data analysis
Demographics of both the therapists and the patients are described.
2.3.1. Activities used
To determine which activities were used during the trial, a single study team member reviewed all videos. The activities used along with notes on how they were applied, what did and did not work, and how interactions developed are presented and discussed.
2.3.2. Patients
Surveys completed by patients who have no cognitive impairment, mild cognitive impairment, or moderate cognitive impairment were analyzed. Surveys completed by subjects with severe cognitive impairment were discarded. The custom questions and TLX questions are reported and apparent impacts from level of impairment and changes between subjects’ first and subsequent trials are observed. The responses to the questions drawn from the Intrinsic Motivation Inventory were analyzed to determine internal consistency among the questions. Questions which had a correlation of less than 0.6 on the expected theme were dropped. Cross-loading was not considered. Correlation was measured and necessary questions dropped iteratively until all questions correlated well (>=0.6) with their expected themes. For the questions taken from Godspeed III: Likability, the responses for the individual questions were averaged.
2.3.3. Therapists
Surveys completed by the therapists were analyzed for any patterns and are described. For questions which were asked both at the beginning and end of the experiment, the data were analyzed to find any pattern of change. For questions which were asked after every day of participation, the pattern of change day to day was evaluated.
3. Results
3.1. Subjects
3.1.1. Patients
Eleven patients participated in the trial, completing 23 sessions. Their demographic information along with the number of trials they completed is shown in Table I. Three subjects suffer from severe cognitive impairment. All three were able to complete their sessions and engage with the therapist remotely and with the robot. They were not able to accurately fill out surveys, so their survey responses were discarded. The remaining eight subjects completed a total of 14 trials; six subjects completed two interactions and the remaining two completed one trial each.
Table I. Patient demographics.

3.1.2. Therapists
Two physical therapists (PT) and one physical therapy assistant (PTA) participated in the trial. Their information along with information on their experience with various forms of technology in general and for healthcare specifically can be seen in Table II. All three subjects had previously made video calls and both therapists had made video calls for healthcare. All three are highly experienced and have practiced for five or more years. The physical therapists have a high level of experience with computers and tablets. All three have a high level of experience with phones. None have much experience with robots. The PTs were positive about using video calls for healthcare, and the PTA was neutral. One PT was positive towards robots, the remaining two therapists were neutral. Two of the therapists had previously seen a presentation on the Flo system during recruitment.
Table II. Information on clinicians and their responses to questions in pre-training survey Table III (see Supplementary material).

Activities which can be done using telepresence. Prior to completing any activities with the Flo system, including training, the therapists rated a variety of common tasks on whether they could be done via telepresence (Fig. 6). The consensus of the therapist group was that telepresence can be used to deliver activities of daily living (ADL) practice, cognitive assessments, discussion about radiology results, and medical prescriptions. Subject 401 has previous experience doing several activities remotely. All three participants agreed that orthotics assessments cannot be done remotely. One participant each believes that stretching, environmental adaptation, and cognitive exercises cannot be done remotely, using telepresence.
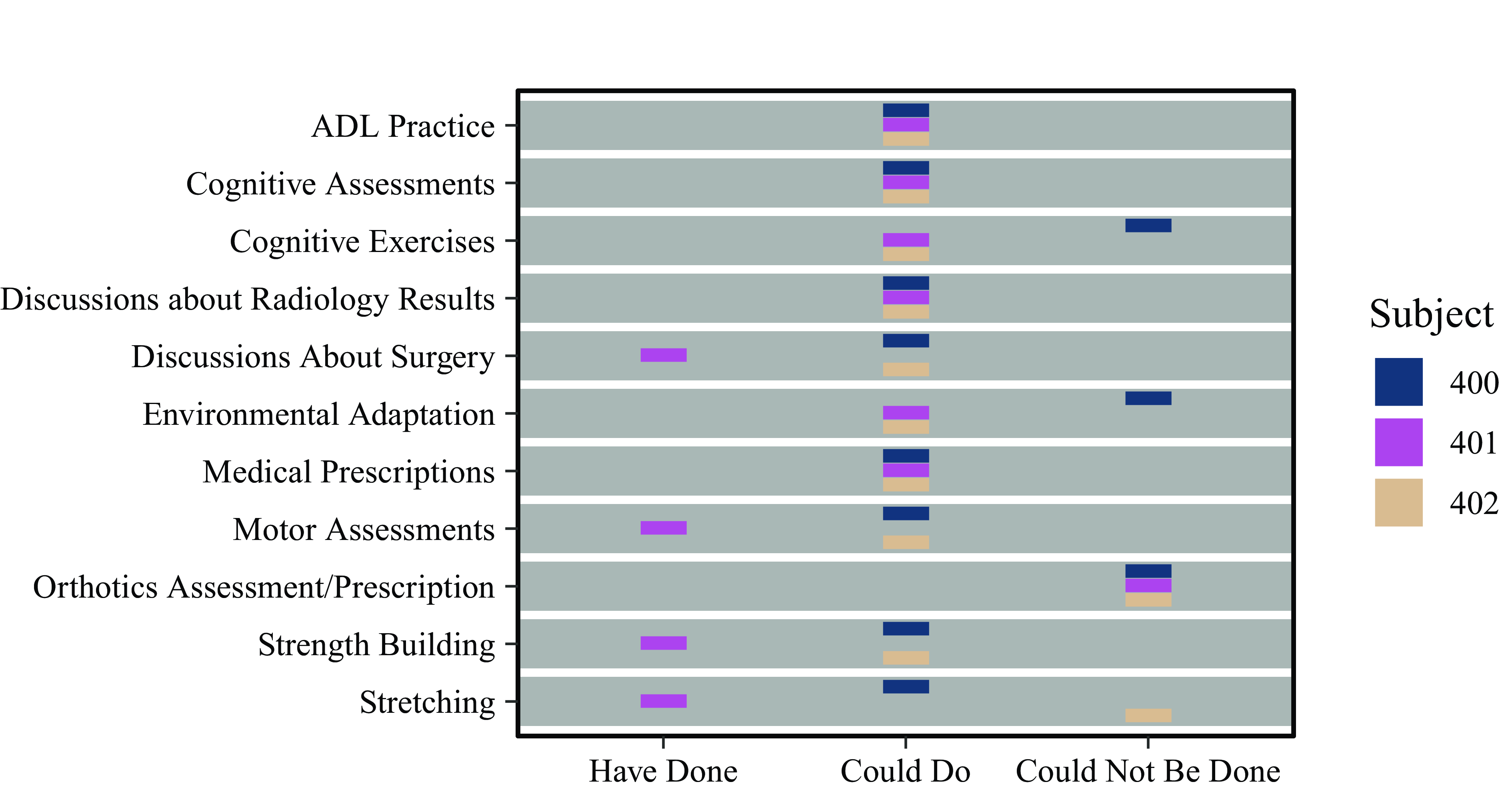
Figure 6. Activities which each participant had done remotely, believed could be done remotely, or believed could not be done remotely, prior to the trial.
3.2. Therapist training
All three therapists were able to successfully complete the training procedure. Responses provided post-training, to the questions comparing SRAT to CT can be seen in Fig. 14 and the responses comparing SRAT to in-person interaction can be seen in Fig. 15. The TLX showed low frustration and physical effort and neutral values for the remaining scores. Subject 400 reported being concerned that they would be a slow pilot.
After the training session, S401 requested help in setting up a Tai-Chi activity on the robot, specifically the “gathering the qi” sequence, modified for seated practice. In which the subject is instructed to breathe in as they raise their arms, extended, out to the side and then overhead and breathe out as they push their arms down through the middle of their chest, towards their navel. A member of the study staff helped S401 create the new poses required, by moving the robot’s arms and recording their positions throughout the motions and creating a new sequence from those poses.
3.3. Interactions
Reviewing the videos from all the interactions shows a clear pattern of interaction development. Each of the therapists developed their own style of operating the robot, which was unique, even though they worked together and communicated regularly.
3.3.1. Therapist 400
Subject 400’s interactions lasted an average of about 13 min (min: 9 min, max: 20 min). In all S400s interactions, they used both the “Simon says” and target touch games. In most of the interactions led by S400, the introduction was short, the robot would say “hello” “how are you” and then the participants jumped straight into a game, sometimes the patient decided the order. On three instances the robot was used to ask the subject about their level of pain prior to beginning the activities. During the “Simon says” activity, S400 would do some of the activities themselves to help demonstrate. For certain activities, they would interrupt the game to push the subject harder by encouraging them to achieve greater range of motion or by adding a modification, for example: having a subject touch the back of their head after touching the top of their head as part of the game or having a subject lift their arms to the side with their thumbs up. During “Simon says” the operator liked to joke with the subject, tell them when they were doing a good job, and use the robot’s “congratulate,” “try harder,” and “gotcha” functionality to build engagement. When appropriate, during “Simon says,” S400 would ask the patient if they were experiencing any pain and would comment on improvements in their range of motion. The target touch activity was run as programmed. In most cases, S400 tried to make the subjects reach as far as possible by placing the robot far from the subjects, even placing it at an offset angle to force reaching 45 degrees away from forward. In one case S400 moved the robot halfway through the target touch activity to the other side of a patient, to fully engage both sides of their upper extremities. For some trials, S400 also used the “gathering the qi” activity, which had been pioneered by S401, sometimes with, sometimes without the breathing component. Finally, the robot and operator would briefly say goodbye.
3.3.2. Therapist 401
Subject 401’s interactions lasted an average of about 25 minutes (min: 21 minutes, max: 30 minutes). They began each interaction with a “personalization activity” in which they tried to build rapport in the three-way interaction between the patient, therapist, and robot. This always began with the robot asleep. The therapist would introduce the robot as “our therapy robot” and encourage the patient to wake it up. Once the patient yelled “wake up” or similar at the robot, the therapist would make the robot show a smiling face and provide some excited commentary. For each patient’s first interaction, the therapist would turn the robot’s face to a frown and ask the patient what emotion the robot was displaying. The therapist would say that the robot had forgotten its name due to a power outage or computer failure and ask the patient to create a new name for the robot. This name was then used for the remainder of the trial. The therapist would ask the robot what they thought about their new name and have the robot say they loved it and smile, and the therapist would say good job or similar. For most patients, after the first interaction, the same name continued to be used. In the case of one patient with severe dementia, the patient never remembered the robot and renamed it with a different name each interaction. The therapist would then introduce the idea of the patient and robot having a discussion (ex: “I would like <name of robot>to get to know you better” or “<robot name>do you have any questions for <patient name>”). They would then have the robot ask questions like: “what do you do for fun,” “what kind of music do you like,” “can you tell me a joke,” etc. The robot would then follow up saying things like “I like that too” or with additional questions like “who is your favorite musician” with the operator asking more complex follow-up questions, helping subjects to answer questions, and providing commentary like “you two are like a match made in heaven.” At some point, the therapist would also encourage the patient to ask the robot questions. In some interactions, the conversation included the robot telling a joke, which was generally accompanied by a significant delay as the therapist worked to concoct a joke and type it in.
In most, but not all, interactions, the therapist would then use the robot to check the patient’s orientation. The robot would be used to ask the patient questions like: “where are you,” “what is the date,” “what is the season,” “who is the governor,” and “who is the president,” all of which are designed to demonstrate how well the patient is aware of where/when they are and what is happening around them. In most interactions the robot would also be used to ask the patient if they had any pain and if so ask to rate it. The therapist and the robot would ask for details when relevant. For a few trials, the therapist used the faces on the robot as an alternative to a 1–10 pain scale, showing a sad, very sad, and extremely sad face and asking the patient which one they felt when their pain was bad.
For some subjects, throughout the conversational elements of the interaction, the therapist would have to clarify what the robot said. Because all these questions and responses were being typed in by the therapist, there were always delays, some of which were long. To cope with these, the therapist would say that the robot was thinking, which satisfied most of the patients. The length of the conversational segments of the trials varied but was around half of each trial.
The interactions then moved to a motor-focused component. The therapist would first tell the patients that they wanted them to mimic what the robot did. They then commanded the robot to move to poses and through sequences, waiting for the subject to complete each one. During this component of the interaction, the robot did little to no speaking. The therapist continued to speak, encouraging patients to push their range of motion and correcting their form. Throughout, they would provide commentary on how certain movements were useful for a functional activity and discuss what if any movements were causing pain. While discussing the motions, the robot was used as a source of motivating positive reinforcement by agreeing with the therapist that certain actions are a good idea/make sense. The next activity was either the “Simon says” game or “gathering the qi” activity. The “Simon says” game progressed as programmed with the operator talking through motions and using the robot’s built-in “congratulate” functionality. The tai-chi “gathering the qi” activity was introduced by the therapist, who in most cases told the patient that they could begin by just watching as the robot completed the motion (most subjects joined in on their own). The motion was repeated several times. If the patient was following along well, then coordinated breathing was added to the motion and it was repeated several more times. S401 only tried the target touch game once, which did not go well (the robot was too far from the patient), leading them to exit the game and not return.
They then concluded with the robot and therapist saying thank you and goodbye followed by the robot driving away.
3.3.3. Therapist 402
Subject 402 only completed one interaction, so there was not enough data to determine their style of operating the robot. The patient (500) who had already completed two interactions with the therapist 401 was not interested in the interaction and S402 was never able to fully engage them. During the interaction between this therapist and the patient 500, the patient did provide some deep perspectives on life, giving advice to the robot.
3.3.4. Overall challenges
There were three major areas that challenged the patients in completing the activities. The first was that the voice was not slow or clear enough for many of them. The second was that many were confused by the target touch activity telling them to use their left or right arm to do activities. They believed that they were supposed to touch a certain colored dot on the left/right hand of the robot. Finally, some subjects had a hard time understanding what the robot wanted them to do for a few specific actions during the “Simon says” activity as a result of the arms being slightly bulky and the hands’ mitten-like shape being unfamiliar and less anthropomorphic to the patients (Fig. 1).
3.4. Therapist test experience
The therapists performed the interactions over multiple days. Their cumulative experience is shown in Fig. 7. After each day of interactions, the therapists answered questions drawn from the NASA TLX along with a question on enjoyment. The development of their responses is shown in Fig. 8. Over time, their mental demand decreased, and performance increased. Other measures were stable, with low physical demand, moderate to high enjoyment, and moderate to low frustration and effort.

Figure 7. The cumulative interaction counts by therapist over time.

Figure 8. The therapists’ ratings on the TLX scale + a TLX-styled question on enjoyment. The testing day, on the x-axis, is the day which the therapist is experiencing (their first day using the system, second day, etc.), which is to say that the third day may not be the same date for each participant. A linear model is shown with the coefficient of determination for each plot. The 95% confidence region for possible linear models is shown in gray. Here enjoyment refers to the therapists’ level of enjoyment. 0 is very low (wording varies) and 100 is very high (wording varies).
They were also asked to rate the interactions from the day on six measures of interaction quality. Their responses are shown in Fig. 9. Ability to assess their patients, patient compliance, and patient motivation all show upward trends as the therapists completed more trials. On all metrics, on average, ratings are good to very good.

Figure 9. The therapists’ ratings on six measures of interaction quality.
3.4.1. Open ended responses
The therapists provided insights on their interactions via open-ended responses.
Subject 400 only provided an open-ended response at the end of the first day of the trial, reporting that the system was easier to use than expected. They also wished there were more options for games.
After the first day, in which S401 worked with one patient, S401 reported that the patient engaged well with what they referred to as a “personalization activity” in which they had the subject rename the robot. S401 had some difficulty remembering how to use the pre-programmed tai-chi activity and required help from a study team member. They stated their goal in this first encounter was to “establish multi-directional flow of conversation between the therapist, the participant, and the robot.” They reported some success but also some difficulty: “participant responded well to some points of communication, but I think I did forget to ask the participant to ask the robot questions.” On their second day of trials, they reported that their patient responded well to the personalization activity, but that they forgot to change the face on the robot to be happy until the end of the session. The high cognitive load of operating the robot was a challenge for the therapist to maintain consistent interactions. On their third day, they innovated another way to use the SRAT system, using the face on the humanoid to indicate different levels of comfort/discomfort as an alternative to an ordinal pain scale. They felt this would be useful for patients who do not understand traditionally delivered pain scales, which use numbers. On their eighth day, they reported various technical challenges with speech and arm control that required them to disconnect and reconnect to the robot multiple times.
After their sole session, S402 reported that they had some issues coordinating movements with the robot due to a lack of experience.
3.5. Patient test experience
The responses by the patients to the four custom questions asked after each interaction (“How much did you enjoy the interaction,” “How safe did you feel during the interaction,” “How well did you understand what you were supposed to do,” “Would you want to have this interaction again”) are shown in Fig. 10 averaged over multiple interactions for those subjects who repeated the trial. Subjects were positive on enjoyment, safety, and understanding. They were neutral-positive about whether they would want to do the interaction again. No clear pattern associated with disability is evident. Evaluating changes between first and second trials (Fig. 18; see Supplementary material), 503 and 504 both reported less positive on all the questions in their post-interaction survey of the first trial. For the second trial, 504 became much more positive, but 503 became more negative on whether they enjoyed the interaction and wanted to do it again. After their first trial, 509 and 511 said that they did not want to do the interaction again and were neutral on doing the interaction again respectively, but after their second trial, both said they would very much like to do the interaction again.

Figure 10. Patient responses to the four custom questions asked after the interactions. Values which are shown are subject-weighted (each subject’s responses are averaged over their one to two interactions to provide a subject value). A box plot is shown with center line median, ends of box at the first and third quartile, and whiskers extending to the furthest value, which is within 1.5 times the inter-quartile range (IQR) of the nearest quartile. Outliers beyond 1.5 IQR from the nearest quartile are indicated by points. An x indicates the mean. Actual values for each subject are shown in a dot plot with shapes and colors indicating motor and cognitive impairment.
In their responses to the selected questions from the NASA TLX (Effort, Frustration, Mental Demand, Performance, and Physical Demand), the patients were more mixed (Fig. 11). Although frustration was initially moderate for three subjects on their first interaction, it was very low for all subjects during their final interaction (Fig. 19; see Supplementary material) and was on average low. Performance was rated neutral to high by all subjects across all interactions and was on average high. Effort, mental demand, and physical demand were overall neutral but highly variable both subject to subject and between interactions for subjects who completed multiple trials. Evaluating subjects’ impairment in connection with responses to the NASA TLX questions (Fig. 11), one subject who is unimpaired cognitively, but has a severe motor impairment, rated effort, mental demand, and physical demand as very high. Patients with moderate cognitive impairment rated frustration on average as slightly higher than other subjects.
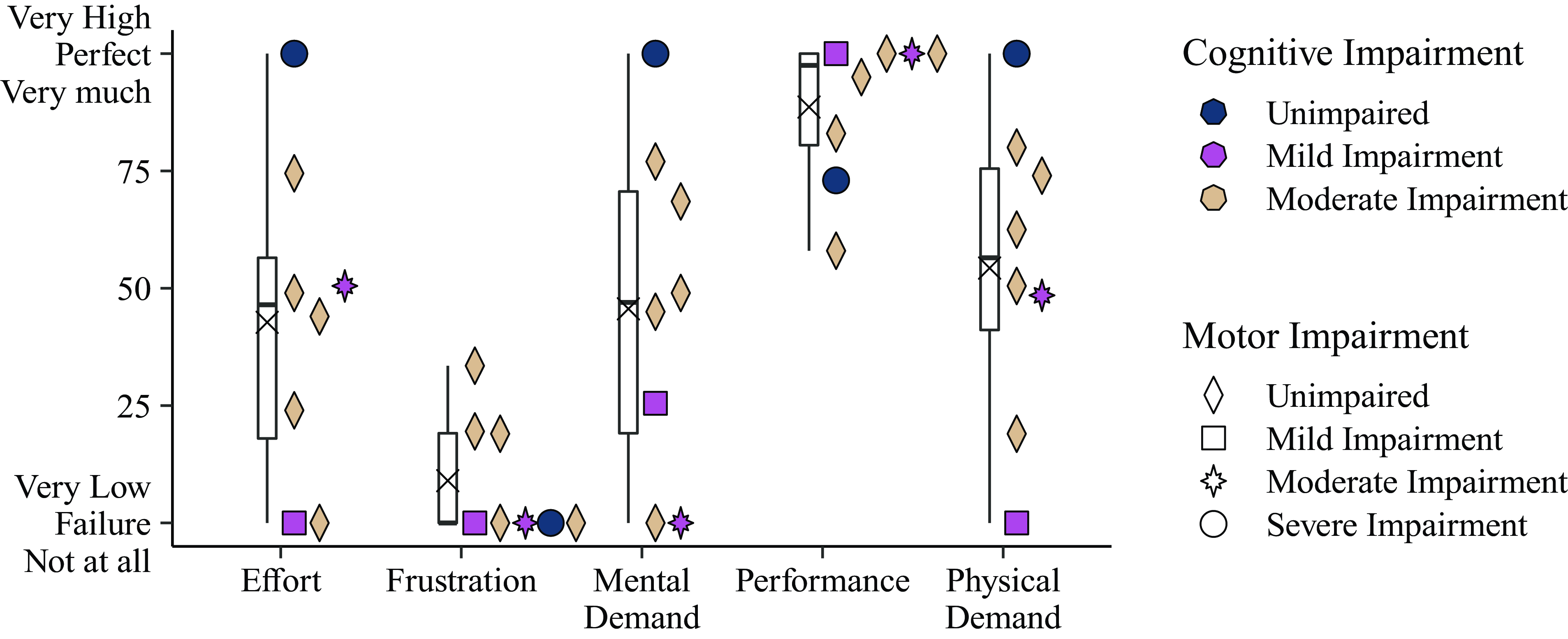
Figure 11. Patient responses to the NASA TLX, asked after the interactions.
Three questions were removed from the Intrinsic Motivation Index which did not correlate well with their assigned scales: “This was an activity that I couldn’t do very well,” “I would describe the activities as very interesting,” “I thought the activities were quite enjoyable.” In general, patients felt they had high competence working with the system (Fig. 12). Enjoyment was also high, with only two subjects giving less than the highest possible value on the two enjoyment questions. Pressure was low but variable, and value was high. Examining the subjects’ disabilities compared to their ratings, there is a suggestion that higher cognitive impairment might lead to decreased competence, enjoyment, and value, and increased pressure. Three subjects felt their competence improved between the first and second interaction, one felt their competence decreased (Fig. 20). The three subjects who improved their rating of competence between the first and second interactions also improved their rating of value. Two other subjects decreased their rating of value between the first and second sessions, one of whom had decreased their rating on competence.

Figure 12. Patient responses to the Intrinsic Motivation Inventory, asked after the interactions.
Finally, evaluating patients’ responses to the Godspeed Likability Scale, focused on the humanoid, all but one subject found the robot very likeable (Fig. 13) with almost no change between subjects’ first and second sessions (Fig. 21 (see Supplementary material)).

Figure 13. Patient responses to the Godspeed survey, section III, questions on Likability, asked after the interactions.
3.5.1. Patient open-ended responses
The patients were asked about their additional comments and thoughts at the end of the interactions. For all subjects, the researcher typed these notes while interviewing the subject. After the first interaction, most patients (n = 6) reported Flo system to be nice, interesting, and the activities to be fun and enjoyable.
It is kinda boring here. This was something different. At beginning, didn’t catch on at beginning but figured out it was fun. I hope I learned something. Will be happy to do it anytime (S501, severe cognitive impairment, aged 85)
It was nice. I understood exactly what was asked of me. I did my best. He [the robot] didn’t criticize me if I got it wrong. I really enjoyed it. Everything worked fine. Would be better if I could use my right arm too [subject’s right arm is severely impaired]. I liked “Simon says” and I liked the second part of it (S510, CVA, severe motor impairment, aged 69)
While some patients (n = 3) found the Flo system might be useful for their rehabilitation such as exercising and stretching arms, one patient was quite negative about it, reporting that the system is not patient-focused from their perspective, given their impairment.
The activities are too easy. Not effective. The program should be focused on the patient’s disability … General Simon says is not useful for my injury. If a patient has a walking disability, then it should be focused on that. (S504, CVA, Cognitive Deficit – Dementia, aged 74)
The patients continued to provide feedback after the second and third interaction. Most of the responses showed continuing positive attitudes towards the system and the interactions between the clinician and the patient delivered over SRAT. While one patient twice found it helpful for motion and exercising, another patient enjoyed having to use their mind.
It went well. I like it, I enjoy it. I enjoy doing what it says to do. Makes me stop and think. I like having to use my mind. [Interviewer: Anything that could be better?] No good as is. Second time doing it, it was good. (S511, CVA, Cognitive Deficit, Dementia, aged 67)
However, some patients (n = 2) did not think that it would help their impairments and did not want to work with the system again. One patient wanted the system to be more technically advanced in its capabilities and to be able to play music.
I wish it [the arms] moved faster […] I would like it if it moved to music […] for the rehab activities. I learned to type by listening to music. Music makes it more fun. (S504, CVA, Cognitive Deficit – Dementia, aged 74)

Figure 14. Responses by therapists to questions on the quality of interactions comparing SRAT-based telerehab to CT-based telerehab as rated post-training (circles, Table IV) and after the end of the trials (squares, Table VII).
3.6. Therapists’ opinions on social robot augmented telepresence vs alternatives
At the end of training and after completing all trials, the therapists were asked how they felt using Flo, an example of a SRAT system compared to classical telepresence (CT) (Fig. 14), recall that subjects 400 and 401 had previously done video calls for healthcare. After training, the therapists felt their ability to assess their patients’ function would be worse with the social robot, compared to classical telepresence alone, and that their patients would be less compliant via SRAT. However, they felt that the social robot would improve patient enjoyment and motivation. On other metrics, their feelings were mixed. After using the robot for all the interactions, the therapists did not change their opinion on their ability to assess patients (worse with SRAT) or adherence to treatment plans (no difference). They adjusted their opinion on compliance, concluding that there was no difference with/without the use of the social robot. They found that their patient’s level of enjoyment was in fact not better with SRAT.
When asked to comment in an open format on ways in which SRAT is better than classical video-based telepresence, and vice versa, one therapist found the novelty value of adding humanoid robot to telepresence.
Droid added novelty to therapy interactions, in a way that a third “personality” involved that the participant could get interested in (S401)
However, both therapists reported that operating Flo was difficult when compared to classical telepresence, specifically in the context of rehab interactions. One therapist found it too hard to adapt to changing interactions with the robot:
As a physical therapist you are constantly changing your treatment plan during the treatment session because of the natural “guess and test” that occurs. It is difficult to modify your treatment plan during the session with Lil’ Flo because of games and options that are pre-loaded. (S400)
The other therapist found it challenging to maintain a quick pace of information flow during interactions:
Communication lag with traditional telepresence is non-existent, whereas with the droid the therapist has to receive data from the participant, then type a response into the speech field, then click a button and wait for droid to output audio response which creates lag in communication and a slow churn of data exchange. Also the voice of the droid is monotoned which is absent in traditional telepresence. (S401)
The therapists were also asked to compare using SRAT to in-person rehab (Fig. 15). They initially reported an expectation that communication would be better in-person and found that to be true. Although they initially expected compliance to be worse via SRAT, they found that compliance was equivalent or better with SRAT than in person. When asked in an open format to say ways in which one was better than the other, S400 noted their inability to assess standing activities with the robot.
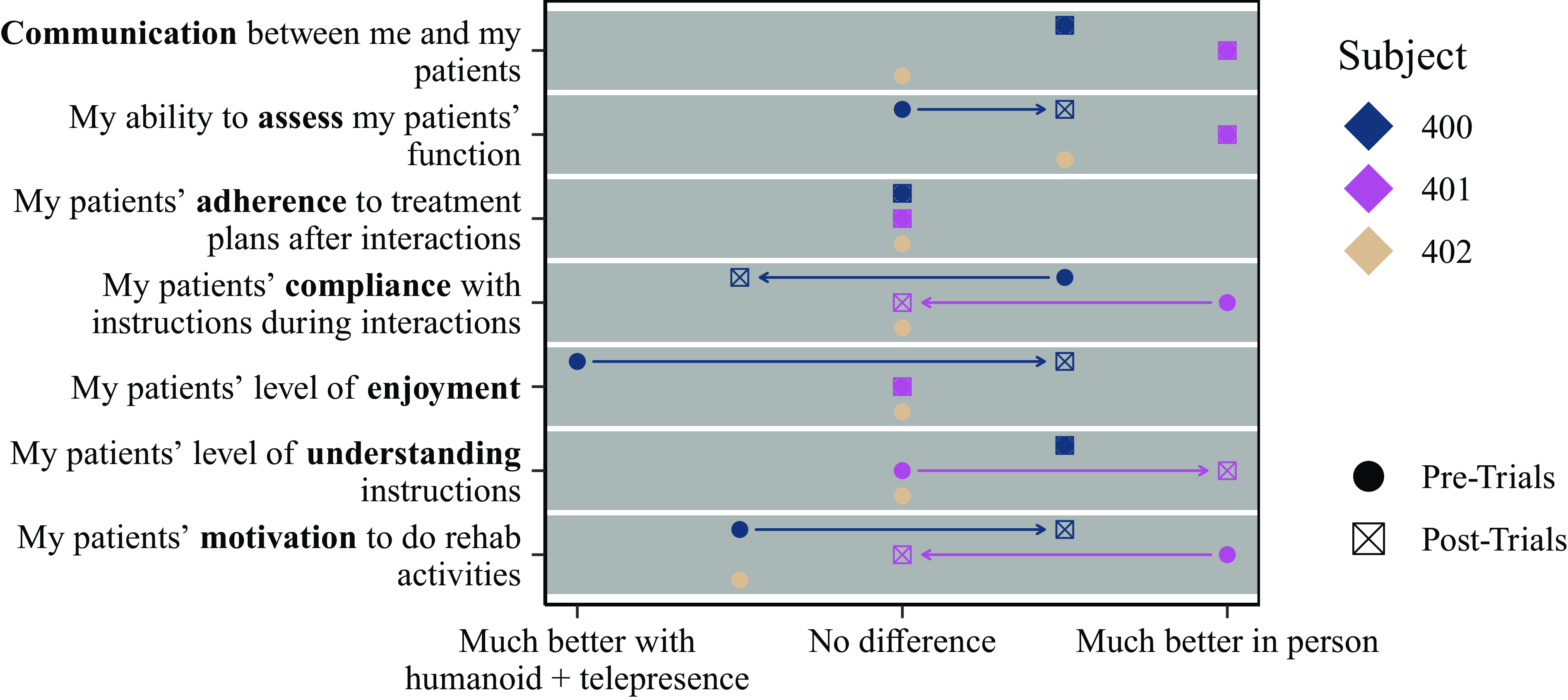
Figure 15. Responses by therapists to questions on the quality of interactions comparing SRAT-based telerehab to CT-based telerehab as rated post-training (circles, Table IV (see Supplementary material)) and after the end of the trials (squares, Table VII (see Supplementary material)).

Figure 16. Responses by therapists on tasks which they believe a SAR-augmented telepresence system would/would not be effective for (Table VII).

Figure 17. Responses after the interactions by therapists on where they believe that a SAR-augmented telepresence system would/would not be useful (Table VII).
3.7. Tasks which therapists believe SRAT would be useful for
When asked “How effective do you believe that Lil’Flo would be for the following activities” after completing the interactions, therapists provided the responses shown in Fig. 16. Although one therapist (S401) felt Flo would be effective for cognitive assessments and exercises as well as discussions about radiology results and surgery, the other therapist thought that Flo would be only fair for those tasks. The two therapists also disagreed on the effectiveness of Flo for orthotics assessment/prescription and strength building. The therapists did agree that Flo would be ineffective for medical prescriptions and stretching.
3.8. Therapist opinions on deployment opportunities for social robot augmented telepresence
Therapists were asked in which types of clinical environments they felt a SRAT system would be useful. Their responses are shown in Fig. 17. Subject 400 perceived the system as extremely useless for deployment in acute care settings, patient homes, and urban inpatient clinics, S401 rated those settings as neutral and above. The usefulness of SRAP in centers for special needs, pediatric acute care settings, and rural outpatient clinics was rated as neutral to useful by both the therapists. Subject 400 rated the implementation of the system in schools as extremely useful. On other settings, the ratings were mixed between useful and useless.
When asked if there were any other clinical settings in which SRAT would be particularly useful, S400 suggested “speech therapy settings” and S401 suggested “independent living centers with several residents in one building.” When asked for settings where SRAT would be particularly useless, S400 suggested “dementia care” and S401 suggested “urgent care.”
3.9. Final thoughts from the therapists
Finally, when asked for final thoughts from their experience, only one had additional comments:
The robot increased the difficulty of common clinical care provided by therapists, due to the demand of the droid on the therapist for constant input to perform any functions. (S401)
4. Discussion
We completed a pilot study with eleven patients who participated actively in rehabilitation interactions delivered by three therapists operating a SRAT system in a clinical setting. We sought to understand how the therapists and patients would use the SRAT system during uncontrolled hands-on interactions with the system. We collected video recordings of the interactions to determine how the system was used and survey responses to evaluate the feasibility of deploying SRAT systems and the benefits and challenges of the modality for enhancing telerehab interactions. This study is one of the first trials of SRAT that we are aware of (the other being [Reference Sobrepera, Lee, Garg and Johnson47]) and is also one of very few trials in the social robotics literature in which a social robot was used in a minimally prescribed protocol by practicing clinicians with their own patients. None of the therapists who participated are part of the design team (which does include other therapists) and had minimal prior exposure to the system. They were empowered to use the system how they saw fit. This qualitative and descriptive research study provides a rich view of how systems like this might be used in the future and highlights opportunities and challenges for the systems.
The most interesting result is certainly that interactions were able to be completed and that the system provided a framework on which different therapists could layer different types of interactions to meet their style of rehabilitation and their patients’ needs. Leveraging their years of experience practicing, the therapists crafted engaging interactions, showing that the SRAT platform gave them the tools to use their expertise and creativity. Both the therapists and the patients reported their performance in interactions as high, and they agreed that the patients’ enjoyment, motivation, understanding, and compliance were high to very high. They also agreed that communication between the therapists with the patients was fair to very good. These results show that among older adults like those studied here the patient experience being treated via SRAT is good.
The constant engagement of the therapists throughout the interactions – providing feedback and modifying activities – demonstrates the value of keeping the therapist in the loop during social robot interactions and provides cues for designers who wish to develop autonomous systems to augment the interactions which patients have with their therapists. Challenges the therapists faced in operating the system highlight opportunities for automation even with the therapist in the loop, key areas of possible improvement are:
-
1) making it easier to talk with the robot, possibly by using advanced text prediction that learns the types of things the therapist wants the robot to say and presents options, incorporating information on the state of the system and the interaction,
-
2) making it easier to modify activities on the fly,
-
3) enabling more activities to be easily implemented, and
-
4) automating interaction components, such as the robot’s face.
The goal should be to decrease the load on the therapist as much as possible while still placing them in control. This type of therapist-robot teaming to build the best interactions possible is promising.
Other opportunities for enhancing the system also became apparent. Subject 400, in moving the robot around the subject to make the target touch easier or harder, highlighted the benefits that a holonomic (or pseudo-holonomic [Reference Specian, Mead, Kim, Matarić and Yim55]) drive system could provide. Subject 401 provided several new activities which could be more tightly integrated into the system to provide an easier operator experience: the “gathering the qi” activity (abstracted to meditation and breathing activities more generally), the question-and-answer activity which helped the robot and patient build a rapport, and the orientation activity designed to discover how well the patient is aware of their surroundings. Both therapists used the robot to assess patients’ pain, clearly measuring and managing pain is an opportunity for development.
Challenges that were observed with subjects understanding the voice of the robot highlight a need to expose voice speech speed to the operator to enable them to slow down the robot’s speech and in general develop voice synthesis systems which are well suited for diverse population. Misunderstandings around which hand to use in the target touch game indicate that the game should be rethought to find a better way to communicate which of the patient’s hands should be used, especially for populations experiencing cognitive decline. A few poses in the “Simon says” activity were unclear to subjects due to the inability of the arms to perfectly reach their targets, longer, more slender arms, with hands that have a clear palm and back of hand would likely fix this problem.
There were several domains in which, when comparing SRAT to classical telepresence (CT) or face-to-face (FTF) interactions, therapists’ initial expectations, post-training, did not hold up in use. The most drastic and most disappointing consensus change was in enjoyment comparing SRAT to CT, where initially, the therapists expected the humanoid to add to their patients’ enjoyment but found that to not be true (Fig. 14). Recall, the therapists reported their patients’ enjoyment as on average good after the interactions (Fig. 9) and subjects reported that they very much enjoyed the interactions (Fig. 10). It is difficult at this stage of testing to understand whether patients would enjoy SRAT less than CT. Although both therapists had previously used telerehab for healthcare, those interactions were not necessarily well matched to the ones presented here. Controlled studies will be needed to shed more light on these questions.
Although initially the therapists expected their patients’ compliance with SRAT to be worse than CT, in practice they found no difference. This mirrors a shift in reported compliance by the therapists over the course of the trials. Initially, the compliance was rated as fair to good, but after S400’s first two days of trials and after S401’s first seven days of trials, they reported compliance as very good. These results agree with the results found in surveys of a larger number of therapists (n = 351), in which therapists expected SRAT to significantly improve compliance [Reference Sobrepera, Lee, Garg, Mendonca and Johnson46]. If this pattern holds up in future deployments, then the question will be how to help first time operators achieve high patient compliance.
Our expectation was that comparing therapists’ ability to assess their patients using SRAT vs. CT there should be either no difference or that SRAT’s ability to push patients to do activities would make it better for assessment. The therapists’ ratings on their ability to assess their patients’ function followed a similar trend to compliance, except that ability to assess patients stabilized on good, not very good. Ability to assess patients was rated as better to much better in CT or FTF than SRAT. One of the contributing challenges, mentioned in the open-ended questions, is that the SRAT system which incorporates the upper body humanoid social robot could not do any lower extremity assessments. Future iterations of SRAT for rehabilitation need legs to aid in seated lower extremity activities. In general, remote assessment is challenging. Developing systems, using computer vision and other technologies, to ease that challenge should be a focus of work for both CT and SRAT.
These patterns of improving interaction quality over multiple uses are also found in the amount of effort which the therapists had to expend during the trials. Initially, mental demand was moderate, and perceived performance was moderate. Over the course of the trials, the mental demand required of the therapists dropped and their perceived performance increased. This is to be expected with any new technology, but again, any ways in which design can make use easier on the therapist will lead to better care for patients.
Unsurprisingly, across most domains, the therapists expected in-person interactions to be better than SRAT. This is not a bad thing. If available, in-person sessions with a well-trained therapist will always be the best option.
The therapists suggested that the SRAT would be useful for rural care settings and pediatric-focused care settings, namely rural outpatient clinics, schools, centers for special needs, and pediatric acute care settings. Working with children makes sense, in prior studies SRAT has been very well received by children [Reference Sobrepera, Lee, Garg and Johnson47]. They were in agreement that SRAT can be useful for rural outpatient clinics. This idea makes sense in that rural areas are a natural application for all forms of telepresence given that patients living these areas experience lower access to care compared to other areas. Using social robots in rural settings is in accordance with findings reported by Orejana et al. [Reference Orejana, MacDonald, Ahn, Peri, Broadbent, Tapus, André, Martin, Ferland and Ammi56] that older adults with chronic health conditions in rural communities were positive and accepting towards an assistive robot and acknowledged its benefits as a companion. There was broad disagreement between the two therapists on other locations. Given the different styles of using the robot between the therapists, it is not surprising that they would see different locations as useful for the robot. Similarly, there was little consensus among the therapists on which activities SRAT would be effective for. After using the system, they did both agree that it would not be well suited for providing medical prescriptions or stretching. The survey also suggested the potential implementation of cognitive exercises and speech-language activities on the system besides the physical therapeutic use.
This study is limited by its sample size of three therapists and eleven patients. This limited sample size together with the small number of interactions delivered through SRAT system (23 rehab sessions) could have implications for the generalizability of our findings to broader populations. The consistency of responses to questions such as the patients’ level of enjoyment, asked multiple ways and responded to by both therapists and patients provides confidence in the results regarding patient experience. Regarding the therapists’ responses, the much smaller number of therapist participants (effectively two) severely limits conclusions which can be drawn from questions comparing SRAT to CT and FTF, where SRAT can be deployed, etc. However, the innovations that the therapists developed and challenges they faced provide important new knowledge to the field.
Due to the limited sample size, we examined whether the responses from our patients and therapists subjects agree with the findings of other literature. Despite the small sample size, our findings are supported. Pulido and colleagues used the NAO social robot with patients with CP and reported that although therapists were able to modify the activities programmed and tailor them to their patients, they found the pre-programmed activities limited [Reference Pulido, Suarez-Mejias, Gonzalez, Ruiz, Ferri, Sahuquillo, Vargas, Infante-Cossio, Calderon and Fernandez43]. The therapists in our study were able to guide all the 11 adult patients to complete the work with the SRAT. They were able to work with the pre-programmed exercises but also create new ones. They also suggested a more flexible user interface for the SRAT that allowed them to more easily introduce new tasks and customize existing ones. Our results also aligned with the survey results of 351 practicing therapists in the United States, who reported that an SRAT would significantly improve communication, motivation, and compliance during telerehabilitation interactions when compared to traditional telepresence [Reference Sobrepera, Lee, Garg, Mendonca and Johnson46, Reference Sobrepera, Lee, Garg and Johnson47]. The therapists in this pilot study maintained their enjoyment of the SRAT system and were able to significantly improve their performance in operating the robot and reported a decrease in the perceived mental demand needed to use it. They also reported that their ability to assess their patients’ functional ability and their patients’ compliance and motivation increased. Feingold-Polak and colleagues [Reference Feingold-Polak, Barzel and Levy-Tzedek44] described that their adult patients responded positively to the interaction with their social robot, Pepper. Similarly, the 8 patients in our pilot study who used the SRAT robot and were able to provide cogent feedback reported that, in general, they enjoyed interacting with the robot despite the high perceived effort and mental and physical demand. Patients also reported that they felt the robot was safe and likable. These results mimic the results reported in prior reviews on older adults using social robots [Reference Góngora Alonso, Hamrioui, de la Torre Díez, Cruz, López-Coronado and Franco57–Reference Getson and Nejat59]. Specifically, the systematic reviews by Pu et al. and Gongora Alonso et al. demonstrated the potential use and effectiveness of social robot interactions to enhance the older adults’ psychological well-being [Reference Góngora Alonso, Hamrioui, de la Torre Díez, Cruz, López-Coronado and Franco57, Reference Pu, Moyle, Jones and Todorovic58]. Moreover, the analysis of the deployment of commercialized socially assistive robots (SARs) during the COVID-19 pandemic by Getson et al. reflected the promising use of SARs to alleviate patients’ loneliness and help reducing the workload on caregivers.
In general, the pilot study allowed us to draw some insights on the hands-on experiences of the therapists and their augmented interactions with the patients, which motivates us to pursue future studies on the system. More importantly, Flo, which has been used throughout this work as an exemplar of SRAT, represents only one attempt at SRAT. The idea of placing social robots into telepresence interactions as third entities can take many forms. Thus, it is important that additional unique systems be developed and researched, so that the field can understand which features of these interactions generalize to SRAT and which are specific to the Flo platform. Our Flo system might not be the first socially assistive robot or the first telepresence platform deployed in healthcare facilities, but it is the first attempt to combine the two modalities. To our understanding, this is the first study evaluating the effectiveness of a SAR-augmented telepresence system implemented in clinical settings. This is also one of the few studies that present the effort of therapists to operate a robotic rehabilitation system to interact with their own patients and evaluate their hands-on experiences with the robot.
5. Conclusions
SRAT system was perceived to be useful in enhancing patient communication, motivation, and compliance in some rehabilitation settings, such as outpatient and rural rehabilitation facilities. In this study, we deployed a SRAT system in a physical rehabilitation clinic which treats elders in the community, in order to explore its utility during therapist-patient interactions in a natural environment. The system was operated in a minimally constrained study by therapists, with their own patients. Preliminary results from responses of 8 patients with normal cognitive function show that patient enjoyment, compliance, motivation, and performance were all high. Another important finding from 23 rehab sessions delivered through SRAT is that the therapists were able to use the system to complete “Simon says” and target touch activities, which were already programmed in, as well as new activities, such as a tai-chi derived “gathering the qi” relaxation activity, pain monitoring, and orientation testing. Previous studies and now this pilot study support the fact that the presence of social robots can alleviate the lack of physical contact during telepresence-based therapies and promote the continuity of rehabilitation. This ability is important for low-resource settings that lack enough skilled therapists and clinicians locally and can leverage remote experts to provide assessment and therapy. The SRAT system not only is a new strategy to mitigate the pandemic’s impact on older adults and society at large but may also play important role in facilitating continuous rehabilitation services and social interactions to address the changing demographics and living situations even after the pandemic is contained. The pilot study also provides the barriers and challenges in usability from the therapist’s perspective, such as high cognitive load of operating the robot, which have highlighted opportunities for future development in automation to enable therapists to more effectively work in a team with social robots and assist them with multiple tasks autonomously. Additionally, future studies should be performed in larger and multicenter scale and in different settings, such as pediatric care and speech and cognitive therapy, to fully assess the usability of the SRAT system and to get more precise insights on its effectiveness.
Acknowledgements
We would like to thank the therapists and patients from Mercy LIFE West Philadelphia who participated in this study, directly operated and interacted with the robot and provided feedback about the robot-augmented telepresence interactions. This paper is based on a chapter of the first author’s PhD dissertation, entitled “Social Robot Augmented Telepresence for remote assessment and rehabilitation of patients with upper extremity impairment.”
Author contributions
Michelle Johnson conceived the original idea for a telepresence-based social robot. Michael Sobrepera led the robot design, led the study design, conducted the experiment, and analyzed data. Michael Sobrepera and Anh Nguyen wrote the article. Michelle Johnson and Emily Gavin supervised the project and revised the manuscript. All authors have read and agreed to the published version of the manuscript.
Financial support
Funding for this project has been provided by the Department of Physical Medicine and Rehabilitation in the Perelman School of Medicine at the University of Pennsylvania, by the School of Engineering and Applied Science at the University of Pennsylvania, and by the Eunice Kennedy Shriver National Institute Of Child Health & Human Development of the National Institutes of Health under Award Number F31HD102165. The content is solely the responsibility of the authors and does not necessarily represent the official views of the National Institutes of Health. This work was also supported in part by Oracle Cloud credits and related resources provided by the Oracle for Research program.
Competing interests
The authors declare none.
Ethical approval
The authors assert that all procedures contributing to this work comply with the ethical standards of the relevant national and institutional committees on human experimentation and with the Helsinki Declaration of 1975, as revised in 2008.
Supplementary material
To view supplementary material for this article, please visit https://doi.org/10.1017/S026357472400002X.