INTRODUCTION
Campylobacter is a major contributor to the burden of enteric diseases in most developed countries [Reference Huang1–Reference Kirk3]. In Canada, more than 10 000 confirmed cases of campylobacteriosis were reported in 2014 [4]. Campylobacter is currently under study, especially with regard to the sources of exposure and the determinants of its temporal dynamics in human cases, peaking in the summer [Reference Lal5]. There are numerous known animal and environmental sources for Campylobacter. Poultry are widely acknowledged as the primary source for sporadic campylobacteriosis and water, as the most frequent source for the rare outbreaks [Reference Said6–Reference Pires10]. Improving our understanding of the dynamics of campylobacteriosis cases and its determinants is an important step toward the design of effective control measures and public health interventions to effectively reduce the incidence of infections.
The seasonal dynamics of campylobacterioses has been studied in many countries using varied methodologies. Few studies have simultaneously considered the dynamics in human cases and in potential source(s), including chicken (farm level or retail), or water. Some have linked the source contamination to the summer peak in cases [Reference Jore11–Reference Wei, Schupbach and Held14], but most did not find such temporal association and concluded that environmental factors or exposure behaviours may better explain the seasonal peak in human cases [Reference Louis15–Reference Tam17]. Several other studies either considered meteorological variables as a determinant of the summer increase in cases incidence, or studied the dynamics of human infections alone and suggested the same hypothesis: the potential role of meteorologically-driven exposure behaviours. Those would include food handling practices such as barbequing and environmental contact with natural waters [Reference White13–Reference Louis15, Reference Tam17–Reference Lal24].
Therefore, to better explain the seasonality of campylobacteriosis cases we considered both the temporal dynamics of known sources of campylobacteriosis and of the exposure behaviours that increase contact with those sources at the individual level. We also considered the sources/behaviours together in their impact on the cases dynamics, to help developing tools to attribute Campylobacter infections to their sources or main exposure determinants.
The FoodNet Canada program is designed to collect integrated data on enteric diseases and their sources in sentinel sites across Canada (currently three sentinel sites in Ontario, British Columbia and Alberta). This design allows collection of contamination and exposure data in the same geographic region and time period as the human cases [25]. Based on these data, the purpose of this study was to describe the dynamics of Campylobacter jejuni cases in a Canadian community and to explore the impact of potential determinants on seasonal variations by applying time series analyses. The potential determinants that were considered included source contamination levels (retail chicken and water samples) and exposure behaviours related to these exposure sources (barbequing and swimming in natural waters) that may represent the meteorologically driven factors that explain the increase in campylobacteriosis incidence in the summer.
MATERIAL AND METHODS
Data
Data were collected from May 2005 to December 2010 under the framework of FoodNet Canada surveillance activities in the Ontario sentinel site located in the Region of Waterloo (ROW).
Human cases
Data on campylobacteriosis were collected using the provincial laboratory-based surveillance system for reportable illnesses. In ROW, this system is complemented by a systematic follow-up of each reported enteric disease case by a public health inspector using a standardized questionnaire. Detailed information on demographics, disease symptoms and exposure to potential risk factors that have occurred prior to illness is collected for the 1–10 days prior to symptom onset. Ethics approval was provided through the ROW Public Health ethics review (approved 9 June 2005).
The focus of this analysis was on sporadic, domestically-acquired C. jejuni cases, C. jejuni being the most frequent species identified among cases (>96%). Outbreak-related (identified through epidemiological or lab-based evidence) and international travel related (travel date overlapping the incubation window) cases were removed from the database for the analyses [Reference Ravel26].
Sources contamination
Retail raw chicken breasts and water samples were routinely collected between 2005 and 2013 in ROW [27]. Raw chicken breasts were collected weekly from grocery stores. Skin-on chicken breasts were collected until 2007 and skinless chicken breasts were sampled from 2007 onwards, with an overlap of samples in 2007 [Reference Cook28]. Stores were selected based on a census of retail grocery store outlets operating within the ROW. Stores were categorized as large (large and medium-sized chain stores) or small (independently owned butcher and market shops). Three large stores and one small store were sampled weekly at random from the store census. Packaged meat was required to be fresh and weigh at least 500 g to ensure sufficient sample quantity for testing. Samples were shipped at refrigeration temperature (2–4 °C) within 24 h of collection to a laboratory for primary isolation, enumeration and subtyping. The Grand River watershed was sampled twice per month at five fixed locations upstream of the regional drinking-.water intake. The approximate number of samples per year was 120. At each sampling, water samples were collected from fast flowing portions of the river using 1 L sterile sampling bottles containing sodium thiosulfate. Chicken and water samples were tested for Campylobacter spp. as described on the FoodNet Canada website (http://www.phac-aspc.gc.ca/foodnetcanada/niedsp10-pnisme10/s03-eng.php).
Exposure behaviours
Information on frequency of exposure to barbequing and natural waters was extracted for all enteric cases reported to FoodNet Canada from the enhanced human case questionnaire. Data included information on attendance at a barbeque (barbequing) and swimming in natural waters (swimming). Barbequing was used as an indicator of potential exposure to C. jejuni through high-risk foods such as chicken by cross-contamination or undercooking, and swimming, as an indicator of potential exposure to C. jejuni through incidental ingestion of untreated surface water.
Information on all enteric disease cases was available for the whole study period, and thus used as a proxy indicator for general population exposures. Exposure data from healthy individuals in ROW was available for the period August 2009–July 2010 only [Reference David29]. Those data were used to validate the coherence with the enteric disease cases exposure frequencies for the common period (95% confidence interval overlap).
Data transformation
Data were transformed into monthly time series: the monthly prevalence of C. jejuni was calculated for chicken and water samples, and monthly frequencies of exposure were calculated for barbequing and swimming. Monthly intervals were used as a compromise between maximizing the number of time points and keeping a sufficient amount of data for each time point.
Trend and seasonality description
The seasonality and long-term trend of the series (number of human cases, prevalence in chicken meat and surface water, frequency of barbequing and swimming) were explored using monthplots and 12 month moving average smoothing.
The seasonality of each variable was modelled using Fourier series:

This type of seasonality model allows multiple peaks and troughs within the same period, year in our study [Reference Fischer30]. We proceeded with a backward method, testing the full model and keeping only the significant sine and cosine components in the final model.
The seasonal pattern of human campylobacteriosis cases was visually compared with (i) the seasonal patterns of C. jejuni prevalence in chicken and water and (ii) the seasonal patterns of the frequency of barbequing and swimming.
Link between cases dynamics and potential determinants
Statistical models adapted to time series analyses were used. The link between local source contamination or exposure behaviour frequencies and campylobacteriosis dynamics was first assessed on the raw series (observed data). Regression with autocorrelated errors was applied, that includes a temporal structure on the remainder in the form of an Auto Regressive Moving Average (ARMA) process [Reference Shumway and Stoffer31].
If a significant association was observed with the raw data (P < 0·05), indicating at least a coincidence in the trend and seasonality of the series, this link was further tested on the stationarized (i.e. de-seasonalized and de-trended) series. The series were stationarized by removing the modelled seasonality and differentiating when necessary. Stationarity was tested using the augmented Dickey–Fuller test.
The link between the stationarized series was further explored using transfer functions, to take into account potential delays in the link between the series. The method used is described by Haugh [Reference Haugh32], and consists in (i) whitening the series (applying an ARMA process so that the remainder is white noise), (ii) assessing the cross-correlations between the explanatory and output whitened series, (iii) including the significant lags into a regression with auto-correlated errors linking the output series to the explanatory series and (iv) estimating the model. Residuals were tested for stationarity and Gaussian distribution using the augmented Dickey–Fuller test and the Shapiro test. These models should allow identifying links between the sources or exposure behaviours and the campylobacteriosis cases dynamics independently of the seasonal variations.
At each step where source contamination or behaviours were found significant, both were included in a single multivariate regression model. Statistical analyses were performed using R (R 3.0.3, R Foundation for Statistical Computing, Vienna, Austria 2014).
RESULTS
The raw time series are presented in Figs 1a (cases and sources) and 1b (cases and behaviours). Sixty-eight time points were included from May 2005 to December 2010. The monthly number of human cases ranged from 2 to 21 (median 9) cases per month. The mean monthly prevalence of positive samples was 33% for retail chicken (range 0–68%), 7% for water (range 0–40%). The mean monthly frequency for barbequing was 0·21 episode per person-month (range 0–0·59) and 0·06 for swimming (range 0–0·44). For the common period (August 2009–July 2010), no significant differences were observed between the monthly behaviour frequencies collected on all cases of enteric diseases and on the interviewees from the ROW healthy population.

Fig. 1. (a) Time series of the monthly number of C. jejuni cases in the region of Waterloo (Ontario) from May 2005 to December 2010, and of the monthly prevalence of C. jejuni in the potential sources, i.e. retail chicken (median monthly number of samples =16, min = 6 and max = 39) and water (median monthly number of samples =10, min = 2 and max = 20), over the same period and in the same geographical area. (b) Time series of the monthly number of C. jejuni cases in the region of Waterloo (Ontario) from May 2005 to December 2010, and of the monthly frequency among all cases of enteric disease of risk behaviours linked to the potential sources, i.e. barbequing and swimming in natural waters, over the same period and in the same geographical area.
Seasonality is evident in each series, with varied peaks (Figs 2a , b ). The human cases peaked in summer (July), contamination of chicken meat was at its highest in the fall (September–October) with a secondary lower peak in March, and contamination of natural waters peaked in late fall (November). Barbequing and swimming were summer activities peaking in July and August, respectively.
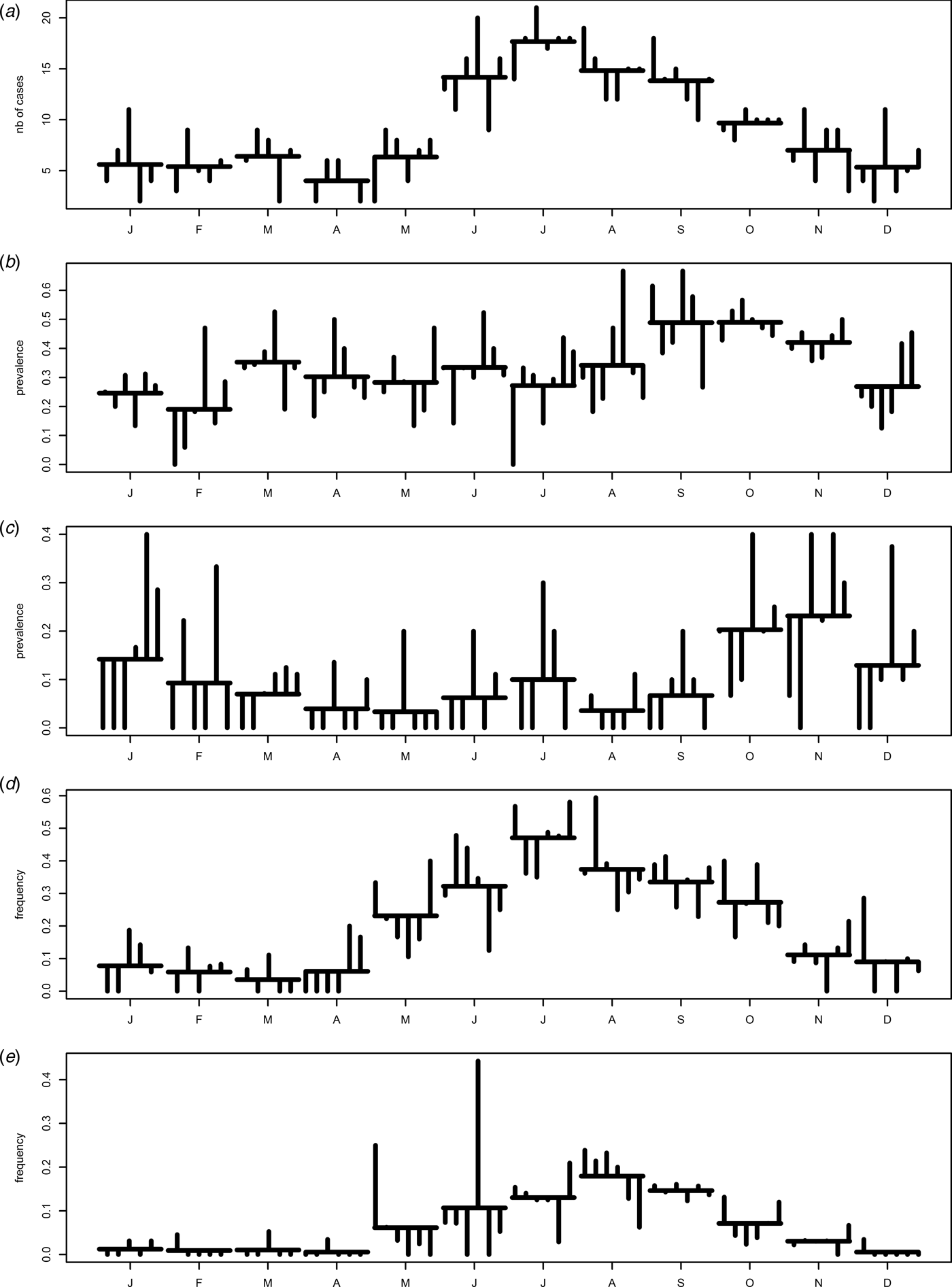
Fig. 2. Monthplots of the time series (2005–2010, region of Waterloo): (a) monthly number of C. jejuni cases, (b) monthly prevalence of C. jejuni in chicken at retail, (c) monthly prevalence of C. jejuni in water, (d) monthly frequency of barbequing in all the enteric cases, and (e) monthly frequency of swimming in natural waters in all the enteric cases.
Long term trend was stable for most series (Figs 3a , b ). A change in the mean level of prevalence was observed for water contamination between 2007 and 2009. That may reflect a laboratory change and sample volume increase (from 500 ml to 1 l) that happened in March 2008. Consequently, 2005–2007 water data were excluded.

Fig. 3. Trends in the time series (2005–2010, region of Waterloo): (a) monthly number of C. jejuni cases, (b) monthly prevalence in chicken at retail, (c) monthly prevalence in water, (d) monthly frequency of barbequing in all enteric cases, and (e) monthly frequency of swimming in natural waters in all enteric cases.
The final seasonality model for human cases (Fig. 4a ) included the significant terms cos(2πt × 1/12), sin(2πt × 1/12), cos(2πt × 2/12) and sin(2πt × 2/12). Cases peaked in August (17 cases) with a global peak to trough amplitude of 12 cases. The final model for chicken meat included three significant terms: cos(2πt × 1/12), sin(2πt × 1/12) and sin(2πt × 2/12). A primary peak in September (48%) and a secondary peak in April (32%) were observed for an amplitude of 25 points. The final model for natural water included one significant term, sin(2πt × 1/12) (Fig. 4a ) and showed one peak in December (22%) for an amplitude of 17 points.

Fig. 4. (a) Modelled seasonality through adjustment of a sinusoid to the data, for the number of C. jejuni cases (cos(2πt × 1/12) + sin(2πt × 1/12) + cos(2πt × 2/12) + sin(2πt × 2/12)), for the prevalence in chicken at retail (cos(2πt × 1/12) + sin(2πt × 1/12) + sin(2πt × 2/12)) and for the prevalence in water (sin(2πt × 1/12)). (b) Modelled seasonality through adjustment of a sinusoid to the data, for the number of C. jejuni cases (cos(2πt × 1/12) + sin(2πt × 1/12) + cos(2πt × 2/12) + sin(2πt × 2/12), for the frequency of barbequing (cos(2πt × 1/12) + sin(2πt × 1/12) + sin(2πt × 2/12)) and for the frequency of swimming in natural waters (cos(2πt × 1/12) + sin(2πt × 1/12) + sin(2πt × 2/12)).
The peak in chicken meat contamination occurs 2 months after the peak in human cases and the peak in water contamination precedes the secondary peak in human cases by 1 month. Considering the incubation period of Campylobacter (about 1 week) it is difficult to relate the increase in the human cases to any of these peaks.
The final seasonality models for barbequing and swimming (Fig. 4b ) were similar in structure. Three terms were included: cos(2πt × 1/12), sin(2πt × 1/12) and sin(2πt × 2/12) and both models demonstrate peaks in August (43% and 16%, respectively) for an amplitude of 38 and 16 points. These seasonal peaks visually matched the peak in cases.
Finally, it can be noted that, for the 2010 – early 2011 period (Fig. 5), seasonality of chicken meat contamination appears to shift, with a peak about 2 months later; additional data may clarify whether this represents a transient or permanent change in the seasonality of chicken contamination.

Fig. 5. Modelled seasonality and raw monthly data for the prevalence of C. jejuni in chicken at retail (2005–2010, region of Waterloo).
The individual regression models applied to the same raw series (Table 1) confirmed (i) the absence of a significant link between the dynamics of sources contamination and of human cases and (ii) the link between the dynamics of behaviour exposures and of human cases. The regression parameters for barbequing and swimming were similar when considered separately (P < 0·001 and P = 0·001) and together in the model (P < 0·001 and P = 0·02). This suggests a potential equivalent impact of the two exposures on cases dynamics. The contribution of barbequing may be higher due to its higher frequency in the population [Reference David29].
Table 1. Results for the regressions with autocorrelated errors the raw series, estimates, 95% confidence intervals and temporal error structure

a Significant models are bolded.
b ARMA (p, q): autoregressive moving average process of order p for auto-regressive process and order q for moving average process.
However, after stationarizing the series, when performing the transfer function analyses, no significant correlation was found between the cases series and either the barbequing or the swimming series, whatever the lag period considered (up to 12 months). This suggests that these behaviour exposures play a role in the summer increase of C. jejuni cases, but do not explain the baseline level of infection.
DISCUSSION
This study represents a first attempt to explore the impact of the temporal dynamics of C. jejuni contamination in both foodborne (retail chicken) and non-foodborne (surface water) sources on the temporal dynamics of human C. jejuni infection. No long-term trend could be detected in human campylobacteriosis but its modelled seasonality was similar to the seasonality observed in other studies, with a summer peak in incidence [Reference Lal5, Reference Wei, Schupbach and Held14, Reference Kovats19, Reference Naumova33, Reference Nylen34]. The temporal pattern of the contamination of the two sources explored was also in accordance with previous research.
More specifically, contamination of retail chicken meat samples peaked in the fall, which is consistent with previous studies that had identified late summer peaks for poultry flocks [Reference Wei, Schupbach and Held14, Reference Hartnack18, Reference Patrick20, Reference Guerin22, Reference Bang35, Reference Jorgensen36] and for retail chicken meat [Reference Meldrum16, Reference Boysen, Vigre and Rosenquist37, Reference Ishihara38]. This late peak has been explained by the cumulative effect of high temperatures on the contamination of poultry flocks, through its influence on flies’ abundance for example [Reference Patrick20, Reference Guerin22, Reference Ishihara39]. Other studies have reported a summer peak including a recent nationwide prevalence survey of retail chicken in Canada [40]. Our observation that the summer peak in human campyloacteriosis preceded the increase of contamination in chicken is consistent with similar findings based on retail chicken or chicken flock contamination [Reference Meldrum16, Reference Hartnack18, Reference Patrick20, Reference Nylen34, Reference Wilson41, Reference Williams42]. This common observation and our regression analyses conducted on raw time series provided no evidence for significant link between campylobacteriosis incidence and the prevalence of contaminated chicken. The analysis of the systematic enumeration of C. jejuni performed in all positive retail chicken samples did not show any obvious temporal pattern across the study period (results not shown). This finding similar to the results of Habib et al. [Reference Habib43] allows for the exclusion of a potential influence of an increase in the bacterial load in retail chicken meat during summer time. Hence, even though 65–69% of attributable domestically-acquired campylobacteriosis cases was estimated to be related to retail chicken meat in the same area (Ravel A, et al. Source attribution of human campylobacteriosis at the point of exposure by combining comparative exposure assessment and comparing subtypes comparison based on defined by comparative genomic fingerprinting. Submitted), the summer peak in human incidence does not appear to be driven by the contamination in retail chicken, similar to a recent conclusion in the USA [Reference Williams42]. Both findings from North America contrast with the positive impact of flock Campylobacter prevalence at slaughter on human campylobacterioisis incidence with 2 week lag demonstrated in Switzerland [Reference Wei, Schupbach and Held14]. In this study, the flock prevalence and the human campylobacteriosis incidence shared a similar large summer peak, which was not present in our study and may explain the different findings. In addition, the authors used a different methodology in modelling the number of human cases through an endemic and an autoregressive epidemic component including the flock prevalence as an independent variable. The role of chicken contamination in the increased incidence during the summer is still debatable and may exist in certain contexts.
This study also focused on surface water as source of campylobacteriosis since it has been suggested that the seasonality of Campylobacter infections may be driven by a fluctuation in Campylobacter persistence in environmental sources, notably water [Reference White13, Reference Louis15, Reference Mughini Gras44]. However, the absence of a significant link between C. jejuni cases and source water contamination, as well as the contamination peaks in the cooler months observed in the current study and in previous research [Reference Eyles45, Reference Wilkes46] do not support this hypothesis. As for chicken meat, the analysis of the systematic enumeration of C. jejuni performed in all positive water samples did not show any obvious temporal pattern on the study period (results not shown), similarly to the results of Habib et al. [Reference Habib43]. Hence, the summer peak in human incidence does not seem to be driven by either the surface water or the chicken meat contamination.
This study also explored the risk exposure behaviours associated with the sources studied, expanding upon previous research, which postulates that changes in behaviour may be a driver of seasonal fluctuation in campylobacteriosis [Reference Jore11, Reference Strachan12, Reference Meldrum16, Reference Spencer23]. Barbequing (a risk for chicken-borne contamination) and swimming in untreated (natural) recreational water (a risk for water-borne contamination) were studied, because they have been suggested as risk factors for infection with Campylobacter and other enteric pathogens [Reference Altekruse47–Reference French and Marshall49]. Our results have confirmed these hypotheses beyond the close match between the observed seasonal fluctuation in human cases and the measured seasonality in barbequing and swimming by a significant link between these behaviours, independently and simultaneously, and the incidence of C. jejuni infection on raw series. When considering both exposures simultaneously, their impact on the burden of human cases seems similar, in terms of relative impact, though swimming was reported by a relatively small proportion of the healthy individuals in the study area (11·7% when age- and gender-standardized to study population), and most frequently in younger population (5 to <15 years of age) and the age group that probably includes most parents of children with that age (30–45 years of age) [Reference David29]. This suggests that the role of this behavioural exposure may preferentially hit a specific sub-population, even if, recent Canadian estimates implicate waterborne transmission in 9·3% of domestic Campylobacter infections [Reference Butler, Thomas and Pintar50], so the potential connection between natural water exposure and illness should not be discounted without further consideration.
When analyzing deseasonalized series the link was no longer significant suggesting a role on the summer amplification of cases but not on the baseline level of contamination. This result leads to consider the series of events that leads to infection, namely (i) contaminated source, (ii) contact with source and (iii) contamination leading to infection and disease. To go further and explore the interplay between source contamination and amplification through exposure behaviours, it may be pertinent to consider compartmental models.
Time series are powerful tools for the analysis of longitudinal data. For example, it was used here to distinguish the impact on seasonality from the impact on baseline level of contamination. However, the routine collection of information on indicators of behaviour exposures over several years in a standardized way is a challenge. The FoodNet Canada enteric case questionnaires allowed for the routine collection of case data on barbequing and swimming over 6 years, not in the general population but for enteric cases other than Campylobacter. The comparison of exposure behaviour frequencies among the enteric cases and in the general healthy population suggests that the non-Campylobacter cases data are a suitable proxy for exposures in the general population.
To reflect behavioural exposure to chicken meat, barbequing was used as proxy. Barbequing represents a wider range of potential exposures to Campylobacter, including consumption of or cross contamination from various undercooked meats, and vegetables (e.g. potato salads). Barbequing also implies an outdoor setting and can include risks related to contact with insects and wildlife : seasonal increases in the exposure to flies have been suggested as a potential source of Campylobacter spp. [Reference Nichols51]. The time series data for pork meat and beef meat contamination over the study period and area showed a very low prevalence of Campylobacter contamination throughout the seasons [25], so that the contamination from chicken meat when barbequing seems most likely. However, even if barbequing was a proxy for overall meat exposures, the role of behaviour, rather than contamination of a particular source, remains valid. When considering the outdoor setting related risks, those cannot be excluded and may be further explored in future studies.
The data on human, food sources, water and exposures collected over long time periods from an integrated sentinel site based surveillance system were invaluable for the study. The weakness of the system though is the restricted number of cases in the geographically restrained area and population. Hence it was not possible to use weekly data, the number of cases per time point would have been too small. A finer time scale would have benefitted the precision of the results and the robustness of the conclusions. The low number of cases also made it difficult to break up the data by age and gender, even though children under five are known to have a specific exposure pattern, which is consistent with the observation in the dataset of a different temporal dynamics for children under five and people over five (data not shown).
Finally, it is difficult to extrapolate the results beyond the ROW to the rest of Canada as meteorological conditions, food contamination and behavioural habits may vary a lot from the West coast to the East coast. Within the FoodNet Canada framework, there is capacity for exploring this issue further, relying on data collected across the different sentinel sites. This would enable exploring how seasonality and the relative relationship between human infection, source contamination and risk exposure behaviours vary geographically and expand the conclusions to the national level.
Another perspective to strengthen the study findings could involve the integration of genetic fingerprinting data in order to consider differences in temporal patterns by sub-type [Reference Friedrich52, Reference McCarthy53]. Previous studies have suggested that specific sub-types of Campylobacter may be more or less susceptible to different pressures and thus be transmitted by different routes [Reference Jorgensen36, Reference Wilson41, Reference French and Marshall49]. Comparative genomic fingerprinting (CGF) is a powerful tool that can be used to implicate specific subtypes of Campylobacter in exploring source contamination and the relative impact of behaviours, a potential next step of this work [Reference Taboada54]. This method is currently being systematically applied on the isolates collected within the FoodNet-Canada sentinel sites. The use of methods such as CGF in conjunction with time series analysis of multiple sources could provide critical insight into source attribution for Campylobacter and other enteric pathogens. The combination of attribution methods is a promising way forward for risk assessments, as has been shown in the study by Mughini Gras et al. that used subtyping methodology to compare genetic similarities between human strains and those isolated from reservoirs including poultry and the environment in addition to a case control questionnaire and in which for example barbequing was identified as a risk factor for Campylobacter strains associated with ruminants [Reference Mughini Gras44].
These results highlight the need for public health interventions to target both the reduction of contamination (e.g. of chicken in the food chain) and modification of behaviours that affect the risk of exposure to Campylobacter. Information campaigns can be built from this to highlight ways in which the population can reduce their personal risk.
CONCLUSIONS
This study represents an exploration, in the Canadian context, of the interplay between source contamination and risk exposure behaviours to increase our understanding of the known but incompletely explained seasonality of human campylobacteriosis. While seasonal patterns were evident in the sources and behaviours explored, the summer increase in human incidence could only be significantly linked to risk exposure behaviours rather than sources contamination (swimming rather than surface water contamination and barbequing rather than retail chicken contamination), suggesting that this increase was the result of an amplification through more frequent exposure behaviours rather than the result of an increase of the reservoir contamination upstream in the farm to fork (source to tap) continuum. This is an indication that food handling education and information on risks related to recreational water sources could be an important way to reduce the increased infections in summer.
ACKNOWLEDGEMENTS
The study was funded by the Public Health Agency of Canada. The authors thank the Region of Waterloo Public Health for the follow-up of the reported cases. Thanks are also due to the Ontario Ministry of Health and Long Term Care's Toronto Public Health Laboratory (now the Ontario Agency for Health Protection and Promotion's Toronto Public Health Laboratory), Grand River Hospital Regional Microbiology Laboratory, Canadian Medical Laboratories, Gamma-Dynacare Laboratories, and Lifelabs for their work with and reporting of cases of disease caused by enteropathogens.
DECLARATION OF INTEREST
None.