I. Introduction
Artificial Intelligence (AI) is a rapidly advancing yet much misunderstood technology. Vastly different definitions of AI, ranging from AI as a mere tool to an intelligent being, give rise to contradicting assessments of the possibilities and dangers of AI. A clearer concept of AI is needed to come to a better understanding of the possibilities of responsible governance of AI. In particular, the relation of AI to the world we live in needs to be clarified. This chapter shows that AI integrates into the human lifeworld much more thoroughly than other technology, and that the integration needs to be understood within a wider picture.
The reasons for the unclear concept of AI do not merely lie in AI’s novelty, but also in the fact that it is an extraordinary technology. This chapter will take a fresh look at the unique nature of AI. The concept of AI here is restricted to computational systems: hard- and software that make up devices and applications which may but do not usually resemble humans. This chapter rejects the common assumption that AI is necessarily a simulation or even replication of humans or of human capacities and explains that what distinguishes AI from other technologies is rather its special relation to the world we live in.
The world we live in includes ordinary physical nature, which humans have been extensively changing with the help of technology – in constructive and in destructive ways. Human life is constantly becoming more bound to technology, up to the degree that the consequences of the use of technology threaten the most fundamental conditions of life on earth. Even small conveniences provided by technology, such as taking a car or plane instead of a bicycle or public transportation, matter more to most of us than the environmental damage they cause. Our dependence on technology has become so self-evident that a standard answer to the problems caused by technology is that they will be taken care of by future technology.
Technology is not only changing the physical world, however, and this chapter elaborates why this is especially true for AI. The world we live in is also what philosophers since Edmund Husserl have called the ‘lifeworld’ (Lebenswelt).Footnote 1 As the ‘world of actually experiencing intuition’,Footnote 2 the lifeworld founds higher-level meaning-formation.Footnote 3 It is hence not only a ‘forgotten meaning-fundament of natural science’Footnote 4 but the ‘horizon of all meaningful induction’.Footnote 5 The lifeworld is not an assumed reality behind experience, but the world we actually experience, which is meaningful to us in everyday life. Husserl himself came from mathematics to philosophy, and the concept of lifeworld culminates his lifelong occupation with the relation between mathematics and experience. He elaborates in detail how, over the course of centuries, the lifeworld became ‘mathematized’.Footnote 6 In today’s expression, we may say that the lifeworld becomes ‘digitized’.Footnote 7 This makes Husserl’s concept of lifeworld especially interesting for AI. While Husserl was mostly concerned with the universal structures of experience, however, this chapter will use the concept of lifeworld in a wider sense that includes social and cultural structures of experience, common sense, and language, as well as rules and laws that order common everyday activities.
Much of technology becomes integrated into the lifeworld in the sense that its use becomes part of our ordinary lives, for example in the forms of tools we use. AI, however, also integrates into the lifeworld in an especially intimate way: by intelligently navigating and changing meaning and experience. This does not imply human-like intelligence, which involves consciousness and understanding. Rather, AI makes use of different means, which may or may not resemble human intelligence. What makes them intelligent is not their apparent resemblance to human capacities, but the fact that they navigate and change the lifeworld in ways that make sense to humans. For instance, a self-driving car must ‘recognize’ stop signs and act accordingly, but AI recognition may be very different from human recognition.
Conventional high-tech, such as nuclear power plants, does not navigate the space of human meaning and experience. Even technologies that aim at changing meaning and experience, such as TV and the Internet, will look primitive in comparison to future AI’s active and fine-grained adaptation to the lifeworld. AI is set to disrupt the human lifeworld more profoundly than conventional technologies, not because it will develop consciousness and will, but because it integrates into the lifeworld in a way not known from previous technology. A coherent understanding of how AI technology relates to the world we live in is necessary to assess its possible uses, benefits, and dangers, as well as the possibilities for responsible governance of AI.
AI attends to and possibly changes not just physical aspects of the lifeworld, but also those of meaning and experience, and it does so in exceedingly elaborate, ‘intelligent,’ ways. Like other technology, AI takes part in many processes that do not directly affect the lifeworld. In contrast to other technology, however, AI integrates into the lifeworld in the just delineated special sense. Doing so had before been reserved to humans and animals. While it should be self-evident that AI does not need to use the same means, such as conscious understanding, the resemblance to human capacities has caused much confusion. It is probably the strongest reason for the typical conceptions of AI as a replication or simulation of human intelligence, conceptions that have misled the assessment of AI and lie behind one-sided enthusiasm and alarmism about AI. It is time to explore a new way of explaining how AI integrates into the lifeworld, as will be done in this chapter.
The investigation starts in Section II with an analysis of the two prevalent conceptions of AI in relation to the world. Traditionally and up to today, the relation of AI to the world is either thought to be that of an object in the world, such as a tool, or that of a subject that experiences and understands the world, or a strange mixture of object and subject. In particular, the concept of AI as a subject has attracted much attention. Already the Turing Test compares humans and machines as if they were different persons, and early visionaries believed AI could soon do everything a human can do. Today, a popular question is not whether AI will be able to simulate all intelligent human behaviour, but when it will be as intelligent as humans.
Section III argues that the subject and the object conception of AI both fundamentally misrepresent the relation of AI to the world. It will be shown that this has led to grave misconceptions of the chances and dangers of AI technology and has hindered both the development and assessment of AI. The attempt to directly compare AI with humans is deeply ingrained in the history of AI, and this chapter analyses in detail how the direct comparison plays out already in the setup of the Turing Test.
Section IV shows that the Turing Test allows for intricate exchanges and is much harder on the machine than it appears at first sight. By making the evaluator part of the experiment, the Turing Test passes on the burden of evaluation, but does not remove it.
The multiple roles of the evaluator are differentiated in Section V. Making the evaluator part of the test covers up the difference between syntactic data and the semantic meaning of data, and it hides in plain sight that the evaluator adds the understanding that is often attributed to the AI. We need a radical shift of perspective that looks beyond the core computation of the AI and considers how the AI itself is embedded in the wider system, which includes the lifeworld.
Section VI will map out further the novel approach to the relation of AI to lifeworld. It elaborates how humans and AI relate to the lifeworld in very different ways. The section explores how the interrelations of AI with humans and data enable AI to represent and simulate the lifeworld. In their interaction, these four parts constitute a whole that allows a better understanding of the place of AI.
II. The Object and the Subject Conception of AI
While in today’s discussions of AI there is a widespread sense that AI will fundamentally change the world we live in, assessments of the growing impact of AI on the world differ widely. The fundamental disagreements already start with the definition of AI. There is a high degree of uncertainty about whether AI is a technology comparable to objects such as tools or machines, or to subjects of experience and understanding, such as humans.
Like other technologies, AI is often reduced to material objects, such as tools, devices, and machines, which are often simply called ‘technology’, together with the software that runs on them. The technological processes in which material technological devices take part, however, are also called ‘technology.’ This latter use is closer to the Greek root of technology, technē (τέχνη), which refers to particular kinds of making or doing. Today, ‘technology’ is primarily used to refer to technological hard- and software and only secondarily to their use. To refer to the hard- and software that makes up an AI, this chapter will simply speak of an ‘AI’. The application of conventional concepts to AI makes it look as if there were only two fundamentally different possibilities to conceive of the relation of AI to the world: that of (1) an object and (2) a subject.
The first takes AI to be an object such as a tool or a machine and assesses its impact on the world we live in in the same categories as that of conventional technologies. It is certainly true that AI can be part of devices we can use for certain purposes, good or bad. Because tools enable and suggest certain uses, and disable or discourage others, they are not neutral objects. Tools are objects that are embedded in a use, which means that they mediate the relationship of humans to the world.Footnote 8 These are important aspects of AI technology. The use of technology cannot be ignored and there are attempts to focus on the interaction between material objects and their use, such as ‘material engagement theory.’Footnote 9 The chapter at hand affirms that such theories take a step in the right direction and yet shows that they do not go far enough to understand the nature of AI. It is not wrong to say that AI systems are material objects that are used in certain ways (if ‘material object’ includes data and software), but this does not suffice to account for this novel technology. While conceiving AI as a mere object used in particular ways is true in some respects, it does not tell the whole story.
AI exhibits features we do not know from any conventional technology. Devices that make use of AI can do things otherwise only known from the intelligent and autonomous behaviour of humans and sometimes animals. AI systems can process large amounts of meaningful data and use it to navigate the lifeworld in meaningful ways. They can perform functions so complex they are hard to fathom but need to be explained in ordinary language.Footnote 10 AI systems are not mere objects in the world, nor are they only objects that are used in particular ways, such as tools. Rather, they actively relate to the world in ways that often would require consciousness and understanding if humans were to do them.Footnote 11 AI here changes subjective aspects of the lifeworld, although it does not necessarily experience or understand, or simulate experience or understanding. The object concept of AI ignores the fact that AI can operate on meaningful aspects of the world and transform them in meaningful ways. No other technology in the history of humanity has done so. AI indeed entails enormous potential – both to do good and to inflict harm.
The subject concept of AI (2) attempts to account for the fact that AI can do things we otherwise only know from humans and animals. AI is imagined as a being that relates to the world analogously to a living subject: by subjectively experiencing and understanding the world by means of mental attitudes such as beliefs and desires. The most common form of the subject account of AI is the idea that AI is something more or less like a human, and that it will possibly develop into a super-human being. Anthropomorphic conceptions of AI are often based on an animistic view of AI, according to which software has a mind, together with the materialistic view that brains are computers.Footnote 12 Some proponents who hold this view continue the science-fiction narrative of aliens coming to earth.Footnote 13 Vocal authors claim that AI will at one point in time be intelligent in the sense that it will develop a mind of its own. They think that Artificial General Intelligence (AGI) will engage in high-level mental activities and claim that computers will literally attain consciousness and develop their own will. Some speculate that this may happen very soon, in 2045,Footnote 14 or at least well before the end of this century.Footnote 15 Estimates like these are used to justify either enthusiastic salvation phantasies,Footnote 16 or alarmistic warnings of the end of humanity.Footnote 17 In the excitement caused by such speculations, however, it is often overlooked that they promote a concept of AI that has more to do with science fiction than actual AI science.
Speculative science fiction phantasies are only one, extreme, expression of the subject conception of AI. The next section investigates the origin of the subject conception of AI in the claim that AI can simulate human intelligence. The comparison with natural intelligence is already suggested by the term AI, and the next sections investigate why the comparison of human and artificial intelligence has misled thinking on AI. There is a sense in which conceiving of AI as a subject is due to a lack rather than a hypertrophy of phantasy: the lack of imagination when it comes to alternative ways of understanding the relation of AI to the world. The basic problem with the object and subject conceptions of AI is that they apply old ways of thinking to a novel technology that calls into question old categories such as that of object and subject. Because these categories are deeply rooted in human thought, they are hard to overcome. In the next section, I argue that the attempt to directly compare them is misleading and the resulting confusion prone to hinder both the development and assessment of AI.
III. Why the Comparison of Human and Artificial Intelligence Is Misleading
Early AI researchers did not try to artificially recreate consciousness but rather to simulate human capabilities. Today’s literal ascriptions of behaviour, thinking, experience, understanding, or authorship to machines ignore a distinction that was already made by the founders of the study of ‘Artificial Intelligence.’ AI researchers such as John McCarthy, Marvin Minsky, Nathaniel Rochester, and Claude Shannon were aware of the difference between, on the one hand, thinking, experiencing, understanding and, on the other, their simulation.Footnote 18 They did not claim that a machine could be made that could literally understand. Rather, they thought that “every aspect of learning or any other feature of intelligence can in principle be so precisely described that a machine can be made to simulate it.”Footnote 19
If AI merely simulates some human capacities, it is an object with quite special capacities. For many human capacities, such as calculating, this prospect is relatively unexciting and does not contradict the object idea of AI. The idea that AI can simulate core or even all features of intelligence, however, gives the impression of some mixture of subject and object, an uncanny ‘subject-object.’Footnote 20 In the case of McCarthy et al.,Footnote 21 the belief in the powers of machine intelligence goes along with a belief that human intelligence is reducible to the workings of a machine. But this is a very strong assumption that can be questioned in many respects. Is it really true that all aspects of learning and all other features of intelligence can be precisely described? Are learning and intelligence free of ambiguity and vagueness?
Still today, nearly 70 years later, and despite persistent efforts to precisely describe learning and intelligence, there is no coherent computational account of all learning and intelligence. Newer accounts often question the assumption that learning and intelligence are reduceable to computation.Footnote 22 While more caution with regard to bold prophecies would seem advisable, the believers in AGI do not think that the fact that we have no general computational account of intelligence speaks against AGI. They believe that, even though we do not know how human intelligence works, we will somehow be able to artificially recreate general intelligence. Or, if not us, then the machines themselves will do it. This is not only deemed a possibility, but a necessity. The fact that such beliefs are based on speculation and not a clear concept of their alleged possibility is hidden behind seemingly scientific numerical calculations that produce precise results such as the number ‘2045’ for the year in which the supposedly certain and predictable event of ‘singularity’ will happen, which is when the development of ‘superhuman intelligence’ becomes uncontrollable and leads to the end of the ‘human era’.Footnote 23
In the 1950s and 1960s, the idea that AI should simulate human intelligence was not far off from the efforts of actual AI research. Today, however, most real existing AI does not even attempt to simulate human behaviour and thinking. Only a small part of AI research attempts to give the appearance of human intelligence, although that part is still disproportionally represented in the media. For the most widely used AI technologies, such as Machine Learning (ML), this is not the case. The reason is obvious: machines are most effective not when they attempt to simulate human behaviour but when they make full use of their own strengths, such as the capability to process vast amounts of data in little time. The idea that AI must simulate human intelligence has little to do with the actual development of AI. Even more disconnected from reality are the speculations around the future rise of AGI and its potential consequences.
Yet, even in serious AI research, such as on ML, the tendency to think of AI in comparison to humans persists. When ML is covered, then often by using comparisons to human intelligence that are easily misleading, such as “system X is better than humans in recognizing Y.” Such claims tend to conceal that there are very specific conditions under which the ML system is better than humans. ‘Recognition’ is defined with respect to input-output relations. The machine is made the measure of all things. It is conveniently overlooked that current ML capabilities break down already in apparently straightforward ‘recognition’ tasks when there are slight changes to the input. The reason is simple: the ML system is usually not doing the same thing humans do when they recognize something. Rather, it uses means such as data correlation to replace recognition tasks or other work that had before been done by humans – or to accomplish things that before had not been possible or economic. Clearly, none of this means that ML becomes human-like. Even in social robotics, it is not always conducive for social interaction to build robots that resemble humans as closely as possible. One disadvantage is expressed in the concept of ‘uncanny valley’ (or ‘uncanny cliff’Footnote 24), which refers to the foundering of acceptance of humanoid robots when their close resemblance evokes eerie feelings. Claiming that AI systems are becoming human-like makes for sensationalistic news but does not foster clear thought on AI.
While AI systems are sometimes claimed to be better than humans at certain tasks, they have obvious troubles when it comes to ‘meaning, reasoning, and common-sense knowledge’,Footnote 25 all of which are fundamental to human intelligence. On the other hand, ML in particular can process inhuman amounts of data in little time. If comparisons of AI systems with humans makes sense at all, then only with reservations and with regard to aspects of limited capabilities. Because of the vast differences between the capabilities, AI is not accurately comparable to a human, not even to an x-year-old child.
For the above reasons, the definitions of AI as something that simulates or replicates human intelligence are misleading. Such anthropomorphic concepts of AI are not apt to understand and assess AI. We need a radically different approach that better accounts for how AI takes part in the world we live in. A clear understanding of the unique ways in which AI is directed to the lifeworld does not only allow for a better assessment of the impact of AI on the lifeworld but is furthermore crucial for AI research itself. AI research suffers from simplistic comparisons of artificial and human intelligence, which make progress seem alternatively very close or unreachable. Periods in which it seems as if AI would soon be able to do anything a human can do alternate with disappointment and the drying out of funding (‘AI Winter’Footnote 26). Overcoming of the anthropomorphic concept of AI contributes to more steady progress in AI science.
How natural it is for humans to reduce complex developments to simplistic notions of agency is obvious in animistic conceptions of natural events and in conspiracy theories. Because AI systems show characteristics that appear like human agency, perception, thought, or autonomy, it is particularly tempting to frame AI in these seemingly well-known terms. This is not necessarily a problem as long as it is clear that AI cannot be understood in analogy to humans. Exactly this is frequently suggested, however, by the comparison of AI systems to humans with respect to some capacity such as ‘perception.’ Leaving behind the idea that AI needs to be seen in comparison to natural intelligence allows us to consider anew how different AI technologies such as ML can change, disrupt, and transform processes by integrating into the lifeworld. But this is easier said than done. The next section shows how deeply rooted the direct comparison of humans and AI is in the standard account of AI going back to Alan Turing.
IV. The Multiple Roles of the Evaluator in the Turing Test
The Turing Test is the best-known attempt to conceive of a quasi-experimental setting to find out whether a machine is intelligent or not.Footnote 27 Despite its age – Turing published the thought experiment, later called the ‘Turing Test’, in 1950 – it is still widely discussed today. It can serve as an illustrative example for the direct comparison of AI to humans and how this overlooks their specific relations to the world. In this section I argue that by making the ‘interrogator’ part of the experiment, the Turing Test only seemingly avoids difficult philosophical questions.
Figure 5.1 shows a simple diagram of the Turing Test. A human and the AI machinery are put in separate rooms and, via distinct channels, exchange text messages with an ‘interrogator’ who has, apart from the content of the messages, no clues as to which texts stem from the human and which from the machine. The machine is built in such a way that the answers it gives to the questions of the ‘interrogator’ appear as if they were from a human. It competes with the human in the other room, who is supposed to convince the evaluator that their exchange is between humans. The ‘interrogator’ is not only asking questions but is furthermore tasked to judge which of the entities behind the respective texts is a human. If the human ‘interrogator’ cannot correctly distinguish between the human and the machine, the machine has passed the Turing Test.
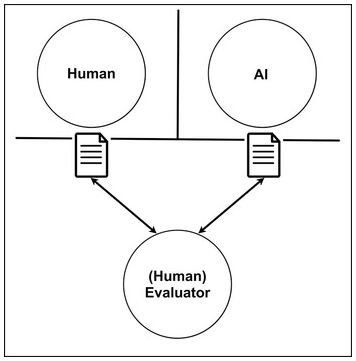
Figure 5.1 The Turing Test
The Turing Test is designed to reveal, in a straightforward way, whether a machine can be said to exhibit intelligence. By limiting the exchanges to texts, the design puts the human and the AI on the same level. This allows for a direct comparison of their respective outputs. As pointed out by Turing himself, the direct comparison with humans may be unfair to the machine because it excludes the possibility that the machine develops other kinds of intelligence that may not be recognized by the human ‘interrogator’.Footnote 28 It furthermore does not take into consideration potential intelligent capabilities of the AI that do not express in exchanges of written text. On the side of the human, the restriction to text exchanges excludes human intelligence that cannot be measured in text exchanges. Textual exchanges are just one of many forms in which intelligent behaviour and interaction of humans may express itself. While the limitation to textual exchanges enables a somewhat ‘fair’ evaluation, at the same time it distorts the comparison.
At first sight, the Turing Test seems to offer only a few possibilities of interaction by means of texts. Turing’s description of the test suggests that the ‘interrogator’ merely ask questions and the human or the AI give answers. Already interrogation can consist of extreme vetting and involve a profound psychological examination as well as probing of the consistence of the story unveiled in the interrogation.Footnote 29 Furthermore, there is nothing in the setup that limits the possible interchanges to questions and answers. The text exchanges may go back and forth in myriad ways. The ‘interrogator’ is as well a conversation partner who engages in the text-driven conversations. He or she takes on, at the same time, the multiple roles of interrogator, reader, interpreter, interlocuter, conversation partner, evaluator, and judge. This chapter uses the wider term ‘evaluator’, which is less restrictive than ‘interrogator’ and is meant to comprise all mentioned roles.
Like other open-ended text exchanges, the Turing Test can develop in intricate ways. Turing was surely aware of the possible intricacies of the exchanges because the declared origin of his test is the ‘Imitation Game.’Footnote 30 The Imitation Game involves pretending to be of a different gender, a topic Turing may have been confronted with in his own biography. If we conceive the exchanges in terms of Wittgenstein’s concept of language-games, it is clear that the rules of the language game are usually not rigid but malleable and sometimes can be changed in the course of the language-game itself.Footnote 31 In free exchanges that involve ‘creative rule-following’,Footnote 32 the interchange may seem to develop on its own due to the interplay of possibly changing motivations, interests, and emotions, as well as numerous natural and cultural factors. While the intricate course of a conversation often seems logical in hindsight, it can be hard to predict even for humans, and exceedingly so for those who do not share the same background and form of life.
Mere prediction of probable words can result in texts that make sense up to a certain degree. Without human editing, they may appear intelligent in the way a person can appear intelligent who rambles on about any trigger word provided by the ‘conversation’ ‘partner’. It is likely to leave the impression of somebody or something that did not understand or listen to the other. Text prediction is not sufficient to engage in a genuine conversation. The claim that today’s advanced AI prediction systems such as GPT-3 are close to passing the Turing TestFootnote 33 are much exaggerated as long as the test is not overly limited by external factors such as a narrow time frame, or a lack of intelligence, understanding, and judgement on the part of the human evaluator.
The Turing Test is thus as much a test of the ‘intelligence’ of an AI system as it is a test of how easy (or hard) it is to trick a human into believing that some machine-generated output constitutes a text written by a human. That was probably the very idea behind the Turing test: tricking a human into believing that one is a human is a capability that surely requires intelligence. The fact that outside of the Turing Test it is often astonishingly easy to trick a human into believing there was an intelligent being behind some action calls into question the idea that humans always show an impressive ‘intelligence.’ The limitations of human intelligence can hence make it easier for a machine to pass a Turing Test. The machine could also simply attempt to pretend to be a human with limited language capabilities. On the other hand, however, faking human flaws can be very difficult for machines. Human mistakes and characteristics such as emotional reactions or tiredness are natural to humans but not to machines and may prove difficult to simulate.Footnote 34 If the human evaluator is empathetic, he or she is likely to have a feeling for emotional states expressed in the texts. Thus, not only the intellectual capabilities of the evaluator but also their, in today’s expression, ‘emotional intelligence’ plays a role. All of this may seem self-evident for humans, which is why it may be easy to overlook how much the Turing Test asks of the evaluator.
Considering the intricate exchanges possible in the Turing Test, the simplicity of its setup is deceptive. Turing set up the test in a way that circumvents complicated conceptual issues involved in the question ‘can machines think?’ It only does so, however, because it puts the burden of evaluation on the evaluator, who needs to figure out whether the respective texts are due to intelligence or not. If, however, we attempt to unravel the exact relations between the evaluator, the other human, the machine, the texts, and the world, we are back to the complicated conceptual, philosophical, and psychological questions Turing attempted to circumvent with his test.
The evaluator may not know that such questions are implicitly involved in her or his evaluation and instead may find the decision obvious or decide by gut feeling. But the better the machine simulates a human and the more difficult it becomes to distinguish it from a human, the more relevant for the evaluation becomes a differentiated consideration of the conditions of intelligence. Putting the burden of decision on the evaluator or anybody else does not solve the complicated conceptual issues that are brought up by machines that appear intelligent. For the evaluator, the process of decision is only in so far simplified that the setup of the Turing Test prevents her or him from inspecting the outward appearance or the internal workings of the machine. The setup frames the evaluation, which also means that it may mislead the evaluation by hiding in plain sight the contribution of the evaluator.
V. Hidden in Plain Sight: The Contribution of the Evaluator
While the setup of the Turing Test puts the burden of assessing whether a machine is intelligent on the evaluator, it also withholds important information from the evaluator. Because it prevents the evaluator from knowing anything about the processes behind the outputs, one can always imagine that some output was produced by means other than understanding. We need to distinguish two meanings of ‘intelligent’ and avoid the assumption that the one leads to the other. ‘Intelligent’ in the first sense concerns the action, which involves understanding of the meaning of the task. Task-solving without understanding the task, for example, by looking up the solutions in the teacher’s manual, is usually not called an ‘intelligent’ solution of the task, at least not in the same sense.
The other sense of ‘intelligent’ refers to the solution itself. In this sense, the solution can be intelligent even when it was produced by non-intelligent means. Because the result is the same as that achieved by understanding, and the evaluator in the Turing Test only gets to see the results, he or she is prevented from distinguishing between the two kinds of intelligence. At the same time, however, the design suggests that intelligence in the second sense amounts to intelligence in the first sense. The Turing Test replaces the question ‘Can machines think?’ with the ‘closely related’Footnote 35 question whether a machine can react to input with output that makes a human believe it thinks. In effect, Turing demands that if the output is deemed intelligent (in the second sense), then the machine should be called intelligent (in the first sense). Due to the setup of the Turing Test, this can only be a pragmatic criterion and not a proof. It is no wonder the Turing Test has led to persistent confusions. The confusion of the two kinds of ‘intelligent’ and confusions with regard to the interpretation of the Turing Test are pre-programmed in its setup.
Especially confusing is the source of the meaning of the texts the evaluator receives. On the one hand, the texts may appear to be produced in an understanding manner, on the other hand, the evaluator is withheld any knowledge of how they were produced. In general, to understand texts, their constituting words and symbols must not only be recognized as such but also be understood.Footnote 36 In the Turing Test, it is the human evaluator who reads the texts and understands their meaning. Assumedly, the human in one room, too, understands what the texts mean, but the setup renders irrelevant whether this really is the case. Both the human and the machine may not have understood the texts they produced. The only thing that matters is whether the evaluator believes that the respective texts were produced by a human. The evaluator will only believe that the texts were produced by a human, of course, when they appear to express an understanding of their semantic meaning. The fact that the texts written by the human and produced by the machine need to be interpreted is easily overlooked because the interpretation is an implicit part of the setup of the Turing Test. By interpreting the texts, the evaluator adds the meaning that is often ascribed to the AI output.
The texts exchanged in the Turing Test have very different relevance for humans and for computers. For digital computation, the texts are relevant only with respect to their syntax. They constitute mere sets of data, and data only in its syntactic form, regardless of what it refers to in the world, or, indeed, whether it refers to anything. For humans, data means more than syntax. Like information, data is a concept that is used in fundamentally different ways. Elsewhere I distinguished different senses of information,Footnote 37 but for reasons of simplicity this chapter speaks only of data, and only two fundamentally different concepts of data will be distinguished.
On the one hand, the concept of data is often used syntactically to signify symbols stored at specific memory locations that can be computationally processed. On the other hand, the concept of data is used semantically to signify meaningful information about something. Semantic meaning of data paradigmatically refers to the world we live in, such as the datum ‘8,849’ for the approximate height of Mount Everest. Data can represent things, relations, and temporal developments in the world, including human bodies, and they may also be used to simulate aspects of the real or a potentially existing world. Furthermore, data can represent language that is not limited to representative data. Humans only sometimes use data to engage in a communication and talk about something in the shared world. They are even less often concerned with the syntactic structure of data. Quite often, texts can convey all kinds of semantic content: besides information, they can convey moods, inspire fantasy, cause insight, produce feelings, and challenge the prejudices of their readers.
To highlight that data can be used to represent complex structures of all kinds, I here also speak of ‘digital knowledge.’ Like data, there is a syntactic and a semantic meaning of digital knowledge. Computers operate on syntactic relations of what constitutes semantic knowledge once it appropriately represents the world. The computer receives syntactic data as an input and then processes the data according to syntactic rules to deliver a syntactically structured output. Syntactic data processing can be done in different ways, for example, by means of logical gates, neuronal layers, or quantum computing. Despite the important differences between these methods of data processing, they are still syntactic methods of data processing, of course. Data processing is at the core of computational AI.
If we want to consider whether a computer has intelligence by itself, the fundamental question is whether certain transformations of data can constitute intelligence. Making the machine look like a human does not fundamentally change the question. In this regard, Turing is justified when he claims that there ‘was little point in trying to make a “thinking machine” more human by dressing it up in such artificial flesh.’Footnote 38 Most likely, doing so would only lead to complications and confusions. Because the core work of computing is syntactic symbol-manipulation, the restriction to texts is appropriate with regard to the core workings of computational AI.
The intelligence to be found here, however, can only concern the second sense of ‘intelligent’ that does not involve semantic understanding. The fact that mere syntactic operations are not sufficient for semantic understanding has been pointed out by numerous philosophers in the context of different arguments. Gottfried Wilhelm Leibniz holds ‘that perception and that which depends upon it are inexplicable on mechanical grounds.’Footnote 39 John Searle claims that computers ‘have syntax but no semantics,’Footnote 40 which is the source of the ‘symbol grounding problem.’Footnote 41 Hubert Dreyfus contends that there are certain things a certain kind of AI, such as ‘symbolic AI,’ can never do.Footnote 42 Recent researchers contend that ‘form […] cannot in principle lead to learning of meaning.’Footnote 43 All these arguments do not show that there is no way to syntactically model understanding, but rather that no amount of syntactic symbol-manipulation by itself amounts to semantic understanding. There is no semantic understanding in computation alone. The search for semantic understanding in the computational core of AI looks at the wrong place.
The point of this chapter is not to contribute another argument for the negative claim that there is something computation cannot do. The fundamental difference between syntactic data and semantic meaning does not mean that syntactic data cannot map structures of semantic meaning or that it could not be used to simulate understanding behaviour. According to Husserl, data can ‘dress’ the lifeworld like a ‘garb of ideas,’Footnote 44 which fits the lifeworld so well that the garb is easily mistaken for reality in itself. Because humans are also part of reality, it easily seems like the same must be possible for humans. Vice versa, data can cause behaviour (e.g., of robots) that sometimes resembles human behaviour in such a perfect manner that it looks like conscious behaviour. At least with regard to certain behaviours it is possible that an AI will appear like a human in the Turing Test or even in reality, even though this is much harder than usually thought.Footnote 45
The point of differentiating between syntactic computation and semantic meaning in this chapter is to build bridges rather than to dig trenches. To understand how the two can cooperate, we need to understand how they are embedded in a wider context. Although it is futile to look for meaning and understanding in the computational core of AI, this is not the end of the story. Even when AI systems by themselves do not experience and understand, they may take part in a wider context that also comprises other parts. To make progress on the question of how AI can meaningfully integrate into the lifeworld, it is crucial to shift the perspective away from the computational AI devices and applications alone toward the AI in its wider context.
The Turing Test can again serve as an example of the embeddedness of the AI in a wider context. By withholding from the evaluator any knowledge of how the texts are processed, the Turing Test stands in the then-prevailing tradition of behaviourism. The Turing Test sets up a ‘black box’ in so far as it hides from the evaluator all potentially relevant information and interaction apart from what is conveyed in the texts. By making the evaluator part of the test, however, Turing goes beyond classical behaviourism. The content of the texts may enable inferences to the mental processes of the author such as motivations and reasoning processes, inferences which the evaluator is likely to use to decide whether there is a human behind the respective channel. By allowing such inferences, the Turing Test is closer to cognitivism than behaviourism. Yet, making the evaluator part of the setup is not a pure form of cognitivism either. To come to a decision, the (human) evaluator needs to understand the meaning of the texts and reasonably evaluate them. By making the evaluator part of the test, understanding of semantic meaning becomes an implicit part of the test. The setup of the Turing Test as a whole constitutes a bigger system, of which the AI is only one part. The point here is not that the system as a whole would understand or be intelligent, but that only because the texts are embedded in the wider system, they are meaningful texts rather than mere objects.
Data is another important part of that bigger system. For the AI in the Turing Test, the input and output texts constitute syntactic data, whereas for the evaluator they have semantic meaning. The semantic meaning of data goes beyond language and refers to things in the world we live in. The lifeworld is hence another core part of the bigger system and needs to be considered in more detail.
VI. The Overlooked Lifeworld
The direct comparison of AI with humans overlooks the fact that AI and humans relate to the lifeworld in very different ways, which is the topic of this section. As mentioned in the introduction, AI systems such as autonomous cars need not only navigate the physical world but also the lifeworld. They need to recognize a stop sign as well as the intentions of other road users such as pedestrians who want to cross the road, and act or react accordingly. In more abstract terms, they need to be able to recognize and use the rules they encounter in their environment, together with regulations, expectations, demands, logic, laws, dispositions, interconnections, and so on.
Turing had recognized that the development and use of intelligence is dependent on things that shape how humans are embedded in, and conceive of, the world, such as culture, community, emotion, and education.Footnote 46 Nevertheless, and despite the incompatibilities discussed in the last section, behaviourists and cognitivists assume that in the Turing Test all of these can be ignored when probing whether a machine is intelligent or not. They overlook that the texts exchanged often refer to the world, and that their meaning needs to be understood in the context of what they say about the world. Because the texts consist outwardly only of data, they need to be interpreted by somebody to mean something.Footnote 47 By interpreting the texts to mean something, the evaluator adds meaning to the texts, which would otherwise be mere collections of letters and symbols. Here, the embeddedness of the evaluator into the lifeworld – including culture, community, emotion, and education – as well as inferences to the lifeworld of the human behind the channel come into play.
The realization that the limitation to textual exchanges captures only part of human intelligence has led to alternative test suggestions. Steve Wozniak, the co-founder of Apple Inc., proposed the AI should enter the house of a stranger and make a cup of coffee.Footnote 48 The coffee test is an improvement over the Turing Test in certain respects. The setup of the coffee test does not hide the relation of the output of the AI to the lifeworld; to the contrary, it explicitly chooses a task that seems to require orientation in the lifeworld. It involves an activity that is relatively easy for humans (but may be quite intricate), who can make use of their common-sense knowledge and reason, to find their way in a stranger’s home. While the Turing Test may involve common sense, for instance to understand or to answer certain questions, this involvement is not as obvious as it is in the coffee test. There are several questions about the coffee test, however. The action of making coffee is much simpler than engaging in open-ended exchanges of meaningful text and may be solved in ways that in fact do only require limited orientation in the physical world rather than general orientation in the lifeworld. Most important in our context is, however, that, like the Turing Test, Wozniak’s test still attempts to directly compare AI with human capabilities. As argued above, this is not apt to adequately capture the strengths of AI and is likely to lead to misrepresentations of the relation of the AI system to the lifeworld.
The relation of the AI system to the lifeworld is mediated through input and output consisting of data, regardless of whether the data corresponds to written texts or is provided by and transmitted to interfaces. Putting a robotic body around a computational system does make a difference in that it enables the system to retrieve data from sensors and interfaces in relation to movements initiated by computational processing. But it doesn’t change the fact that, like any computational system, ultimately the robot continues to relate to the lifeworld by means of data. The robotic sensors provide it with data, and data is used to steer the body of the robot, but data alone is not sufficient for experience and understanding. Like any machine, the robot is a part of the world, but it does not have the same intentional relation to the world. Humans literally experience the lifeworld and understand meaning, whereas computational AI does not literally do so – not even when the AI is put into a humanoid robot. The outward appearance that the robot relates to the world like a living being is misleading. Computational AI thus can never be integrated in the lifeworld in the same way humans are. Yet, it would plainly be wrong to claim that they do not relate to the lifeworld at all.
Figure 5.2 shows the fundamental relations between humans and AI together with their respective relations to data and the lifeworld that are described in this chapter. Humans literally experience the lifeworld and understand meaning, whereas computational AI receives physical sensor input from the lifeworld and may modify physical aspects of the lifeworld by means of connected physical devices. AI can (1) represent and (2) simulate the lifeworld, by computing (syntactic) data and digital knowledge that corresponds to things and relations in the lifeworld. The dotted lines indicate that data and digital knowledge do not represent and simulate by themselves. Rather, they do so by virtue of being appropriately embedded in the overall system delineated in Figure 5.2. The AI either receives sensor or interface input that is stored in digital data and which can be computationally processed and used to produce output. The output can be used to modify aspects of the lifeworld, for example, to control motors or interfaces that are accessible to other computing systems or to humans.
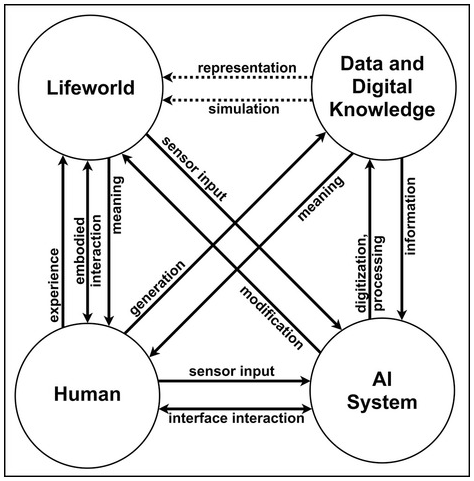
Figure 5.2 The fundamental relations between humans, AI, data, and the lifeworld
In contrast to mere computation, which only operates with data, AI in addition needs to intelligently interact in the lifeworld. Even in the Turing Test, in spite of the restriction of the interactions to textual interchange rather than embodied interactions, the lifeworld plays a crucial role. From the discussion of the test, we can extract two main reasons: (1) the textual output needs to make sense in the context of the lifeworld of the evaluator, and (2) even though exchanges by means of written texts are rather limited, the exchange is carried out via a channel in the lifeworld of the evaluator. The interchange itself happens in the lifeworld, and the AI needs to give the impression of engaging in the interchange.
In other applications of AI technology, AI devices are made to intelligently navigate and modify the lifeworld, as well as interact in it. As pointed out in Section VI, autonomous cars need to take into account the behaviour of human road users such as human drivers and pedestrians. The possibly relevant behaviour of human road users is generally neither the result of strict rule-following nor is it just random. Humans often behave according to their understanding of the situation, their aims, perspectives, experiences, habits, conventions, etc. Through these, humans direct themselves to the lifeworld. Humans experience the lifeworld as meaningful, and their behaviour (mostly) makes sense to other members of the same culture.
Relating to the lifeworld in intelligent ways is an exceptionally difficult undertaking for computational AI because it needs to do so by means of data processing. As acknowledged above, there is a radical difference between syntactic data processing and experience and understanding, a difference that cannot be eliminated by more syntactic data processing. This radical difference comes through in the difference of, on the one hand, data and digital knowledge, and, on the other, the lifeworld. As discussed above, syntactic data and digital knowledge need to be interpreted to say something about the lifeworld, but such interpretation cannot be done by data processing. The difference between syntactic data processing and experience and understanding must be bridged, however, if AI is supposed to intelligently interact in the lifeworld. The combination of the need to bridge the difference and the impossibility of doing so by data processing alone looks like an impasse if we limit the view to the AI and the processing of data and digital knowledge.
We are not stuck in the apparent impasse, however, if we take into account the wider system. The wider system bridges the gap between, on the one hand, data and digital knowledge, and, on the other, the lifeworld. Bridging a gap is different from eliminating a gap, as the difference between both sides remains. All bridges are reminders of the obstacle bridged. Bridges are pragmatic solutions that involve compromises and impose restrictions. In our case, data and digital knowledge do not fully capture the world as it is experienced and understood but rather represent or simulate it. The representation and simulation are made possible by the interplay of the four parts delineated in the diagram.
Biomimetics can certainly inspire new engineering solutions in numerous fields, and AI research especially is well-advised to take a more careful look at how human cognition really operates. I argued above that a naïve understanding of human cognition has led to misguided assessments of the possibilities of AI. The current section has given reasons for why a better understanding of human cognition needs to take into account how humans relate to the lifeworld.
It would be futile, however, to exactly rebuild human cognition by computational means. As argued in Section III, the comparison of human and artificial intelligence has led to profound misconceptions about AI, such as those discussed in Sections IV and V. The relation of humans to their lifeworld matters for AI research, not because AI can fully replace humans but because AI relates to the lifeworld in particular ways. To better understand how AI can meaningfully integrate into the lifeworld, the role of data and digital knowledge needs to be taken into account, and the interrelations need to be distinguished in the way delineated in Figure 5.2. This is the precondition for a prudent assessment of both the possibilities and dangers of AI and to envision responsible uses of AI in which technology and humans do not work against but with each other.