Breast cancer is one of the leading causes of death among women in developed countries. The association between deregulated lipid metabolism and the risk of CVD is well established( Reference Huxley, Lewington and Clarke 1 ). In contrast, regarding breast cancer, the role of biomarkers of lipid metabolism has been less investigated and remains unclear. This is notably the case for blood cholesterol levels, which represent easily measurable markers that are often assessed in clinical practice.
Experimental studies have provided evidence about the possible mechanisms by which cholesterol (especially HDL-cholesterol (HDL-C)) could influence carcinogenesis( Reference Soran, Hama and Yadav 2 – Reference Silvente-Poirot and Poirot 4 ). Since the 1980s and until very recently( Reference Kitahara, Berrington de and Freedman 5 – Reference Strohmaier, Edlinger and Manjer 10 ), many studies have investigated the relationship between total cholesterol (TC) and the risk of breast cancer( Reference Kitahara, Berrington de and Freedman 5 , Reference Melvin, Seth and Holmberg 7 , Reference His, Zelek and Deschasaux 9 – Reference Fagherazzi, Fabre and Boutron-Ruault 21 ), some providing specific results regarding HDL-C( Reference Bosco, Palmer and Boggs 6 – Reference His, Zelek and Deschasaux 9 , Reference Hoyer and Engholm 15 , Reference Moorman, Hulka and Hiatt 22 – Reference Agnoli, Berrino and Abagnato 27 ) and fewer investigating LDL-cholesterol (LDL-C)( Reference Melvin, Seth and Holmberg 7 , Reference His, Zelek and Deschasaux 9 , Reference Hoyer and Engholm 15 , Reference Gaard, Tretli and Urdal 16 ), apoA1 and apoB( Reference Melvin, Seth and Holmberg 7 , Reference His, Zelek and Deschasaux 9 ). These studies have shown contrasting results that need to be synthesised and put into perspective( Reference Silvente-Poirot and Poirot 4 ). However, to our knowledge, no systematic review and meta-analysis of prospective studies investigating these relationships has been published so far.
Caution is needed regarding potential preclinical bias in epidemiological studies investigating the association between blood cholesterol level and the risk of breast cancer( Reference Jacobs and Gapstur 28 ). Indeed, cancer itself (even at the subclinical stage) is likely to influence blood cholesterol levels by several possible mechanisms, such as increased cholesterol catabolism due to improved LDL receptor activity in malignant cells( Reference Vitols, Gahrton and Bjorkholm 29 – Reference Henriksson, Eriksson and Ericsson 31 ), greater utilisation of cholesterol for new membrane biogenesis, or accumulation of esterified cholesterol in tumour tissues( Reference Dessi, Batetta and Pulisci 32 ). Thus, the most relevant epidemiological studies are those with a prospective design, and, even better, those that have tested the associations between blood cholesterol parameters and the risk of breast cancer after exclusion of cancer cases diagnosed during the first years of follow-up( Reference Kitahara, Berrington de and Freedman 5 , Reference His, Zelek and Deschasaux 9 – Reference Vatten and Foss 14 , Reference Gaard, Tretli and Urdal 16 , Reference Eliassen, Colditz and Rosner 19 , Reference Moorman, Hulka and Hiatt 22 , Reference Kucharska-Newton, Rosamond and Mink 24 , Reference Kabat, Kim and Chlebowski 26 , Reference Bjorge, Lukanova and Jonsson 33 ). A recent publication( Reference Melvin, Holmberg and Rohrmann 34 ) meta-analysed the association between TC (>6·5 mmol/l) and the risk of breast cancer, and found a hazard ratio (HR) of 1·08 (95 % CI 0·89, 1·31). However, both prospective and non-prospective studies were included in that meta-analysis, which limited the interpretation. A recent meta-analysis on the metabolic syndrome and the risk of postmenopausal breast cancer observed an inverse association of these risk factors with HDL-C levels( Reference Esposito, Chiodini and Capuano 35 ); however, only three of the available studies were included.
The objective of the present study was to perform a systematic review and meta-analysis of prospective studies investigating the association between TC, HDL-C, LDL-C, ApoA1, ApoB and the risk of breast cancer. The meta-analysis was performed overall and separately for studies that excluded cancer cases diagnosed during the first years of follow-up (thereby eliminating potential preclinical bias) and for pre- and postmenopausal breast cancers. A highest v. lowest as well as dose–response meta-analysis was conducted.
Materials and methods
The PRISMA protocol/checklist was followed for the present systematic review and meta-analysis( Reference Moher, Liberati and Tetzlaff 36 ).
Literature search
We conducted a search in MEDLINE (up to January 2014), without publication date or language restrictions, by combining the medical subject and corresponding entry terms for TC, HDL-C, LDL-C, apolipoproteins, primary breast neoplasms and observational studies (see online Supplementary material 1). We also hand-searched reference lists of retrieved articles, reviews and meta-analysis papers on the related topic.
Study selections or full-text manuscripts were identified and reviewed independently by two investigators, with all discrepancies resolved through discussion or arbitration by a third reviewer.
Studies were included if they met the following inclusion criteria: original research article; cohort or nested case–control study design with female primary breast cancer as the outcome; assessment of serum or plasma cholesterol levels; report of the HR or OR and 95 % CI for blood cholesterol level. Only published peer-reviewed studies were included. No age restriction or minimum length of follow-up was required. For a given meta-analysis, only one publication per cohort study was retained. In the case of duplicate publications, selection was based on longer follow-up, more cases recruited, and completeness of information required to perform the meta-analysis. A total of twenty-six potentially relevant full-text publications were identified( Reference Kitahara, Berrington de and Freedman 5 – Reference Agnoli, Berrino and Abagnato 27 , Reference Bjorge, Lukanova and Jonsson 33 , Reference Hiatt, Friedman and Bawol 37 , Reference Manjer, Kaaks and Riboli 38 ). We excluded two duplicate publications( Reference Hiatt, Friedman and Bawol 37 , Reference Manjer, Kaaks and Riboli 38 ).
Data extraction
Using a standardised data collection form, the following information was extracted for each study: first author's last name; publication year; study characteristics (country and region, design, recruitment, and mean follow-up periods); participant characteristics (sample size, number of cases, ethnic origin of participants, mean age, and percentages of pre- and postmenopausal women); blood cholesterol assessment (parameter assessed and serum or plasma); cholesterol level comparisons and corresponding HR or OR and 95 % CI: adjustment for covariates; results of stratified analyses (e.g. by menopausal status). Data regarding the association between cholesterol and the risk of breast cancer according to different breast cancer types (by histological type and hormonal receptor status) were also recorded.
We assessed the quality of each individual study according to the Newcastle–Ottawa Scale( Reference Wells, Shea and O'Connell 39 ), designed for assessing the quality of non-randomised studies in meta-analyses.
Statistical analyses
Summary HR and 95 % CI for highest v. lowest and dose–response meta-analyses were computed using random- and fixed-effects models when at least two studies were available. Random-effects models were retained as the main analysis as there was evidence of heterogeneity in most models( Reference DerSimonian and Laird 40 ). The weight of each individual study in the random-effects meta-analysis was calculated by the Stata ‘Metan’ procedure based on within- and between-study variations in the DerSimonian and Laird method( Reference Harris, Bradburn and Deeks 41 ). We used the most fully adjusted relative risk in each article, provided that they were not adjusted for factors potentially in the causal pathway. If studies reported results separately by menopausal status, we combined two specific estimates using fixed-effects models to generate a global estimation. Dose–response meta-analysis was performed using either directly the HR per 1 mmol/l increment when it was provided, or the method described by Greenland & Longnecker( Reference Greenland and Longnecker 42 ) to compute study-specific slopes (linear trends) and 95 % CI from the natural logarithms of HR and CI across blood cholesterol categories (at least three) reported in the studies. The method requires the report of the distribution of cases and person-years or non-cases, and the relative risk with the variance estimates for at least three quantitative exposure categories. Using standard methods( Reference Bekkering, Harris and Thomas 43 ), we estimated the distribution of cases or person-years in studies that did not report these parameters but reported the total number of cases and person-years. The median level of exposure in each category was assigned to the corresponding HR or OR when reported. If not reported, the value assigned was the midpoint of the lower and upper bounds in each category. For extreme open-ended categories, half the width of the adjacent exposure category was subtracted (for the lowest category) or added (for the uppermost category) to obtain the midpoint. Dose–response slopes for an increment of 1 mmol/l of blood cholesterol parameters were estimated using the midpoint of each cholesterol category. The slopes and CI were back-transformed using the exponential function.
The thresholds for the ‘highest v. lowest’ meta-analysis were those determined by the authors of each original publication (i.e. based on either the distributions within their study population or predefined cut-offs). All cut-offs used in each study are provided in online Supplementary Table S2 and in forest plots (Figs. 2(b) and 3(b)).
The parameters studied were TC, HDL-C, LDL-C, ApoA1, ApoB, TC:HDL-C ratio and LDL-C:HDL-C ratio. The meta-analysis was also conducted separately in pre- and postmenopausal women, and in studies that did and did not exclude cancer cases diagnosed during the first years of follow-up (1–5 years excluded, depending on the studies).
The influence of each individual study with low HR( Reference His, Zelek and Deschasaux 9 , Reference Tornberg, Holm and Carstensen 12 , Reference Vatten and Foss 14 , Reference Hoyer and Engholm 15 ) on the summary relative risk was examined by excluding each in turn and pooling the rest.
Heterogeneity between the studies was assessed by the Cochran Q test and the I 2 statistic, which is the amount of total variation explained by the between-study variation( Reference Higgins, Thompson and Deeks 44 ). To investigate the potential sources of heterogeneity, we also conducted bivariate linear meta-regression analyses (procedure ‘Metareg’ in Stata)( Reference Harbord and Higgins 45 ) for each of the following parameters in turn: length of follow-up (short or long median follow-up durations); range of exposure (low or high median of ranges between midpoints of extreme categories in mmol/l across all studies); geographical location; year of publication ( < 2000 or ≥ 2000); adjustment for confounding factors (such as physical activity, alcohol intake, smoking status, parity, height, glycaemia and TAG levels, BMI, and simultaneous adjustment for all components of the metabolic syndrome except HDL-C, one meta-regression per factor). Among studies that excluded preclinical bias, a meta-regression analysis was also performed to test the influence of the number of years of follow-up excluded ( < 3 v. ≥ 3 years). Since the number of years of follow-up excluded varied between 1 and 5 across the studies, we used the median value (i.e. 3 years) as the cut-off for this sensitivity analysis. When the results of the meta-regression were statistically significant, stratified analyses were performed. Publication bias was assessed with the following complementary indicators: funnel plots; Egger's test( Reference Egger, Davey and Schneider 46 ); Begg's test( Reference Begg and Mazumdar 47 ).
The non-linear association between total blood cholesterol and the risk of breast cancer was explored by using restricted cubic splines with three knots (two spline transformations) at fixed percentiles (10, 50 and 90 %) of the cholesterol distribution. Only studies showing data for four or more categories of blood cholesterol were included in the analysis. The P value for non-linearity was obtained by testing whether the regression coefficient of the second spline was null( Reference Orsini, Li and Wolk 48 ).
A two-sided P< 0·05 value was considered statistically significant. All statistical analyses were performed with Stata version 12 (Stata Corporation).
Results
Figure 1 shows the flow chart of the study selection process. The results from twenty-four articles were included in the main or stratified meta-analysis (twenty-two cohort and two nested case–control studies), corresponding to twenty different studies. Of the included studies, thirteen were from Europe, seven from America and four from Asia. The characteristics of the included studies are provided in online Supplementary Tables S1 and S2. All the included studies were performed in adults, with a long follow-up (minimum 8 years). The Newcastle–Ottawa Scale( Reference Wells, Shea and O'Connell 39 ) score range was narrow (number of points out of 9: 6( Reference Bosco, Palmer and Boggs 6 , Reference Tornberg, Holm and Carstensen 12 , Reference Knekt, Reunanen and Aromaa 13 , Reference Steenland, Nowlin and Palu 17 , Reference Eliassen, Colditz and Rosner 19 , Reference Fagherazzi, Fabre and Boutron-Ruault 21 , Reference Moorman, Hulka and Hiatt 22 ), 7( Reference Kitahara, Berrington de and Freedman 5 , Reference Melvin, Seth and Holmberg 7 – Reference Hiatt and Fireman 11 , Reference Vatten and Foss 14 – Reference Gaard, Tretli and Urdal 16 , Reference Iso, Ikeda and Inoue 20 , Reference Furberg, Veierod and Wilsgaard 23 – Reference Agnoli, Berrino and Abagnato 27 , Reference Bjorge, Lukanova and Tretli 49 ) and 9( Reference Tulinius, Sigfusson and Sigvaldason 18 )). All the twenty-four selected studies met sufficient quality criteria to be included in the analysis. There was no indication of publication bias with Egger's and Begg's tests in all the meta-analyses tested. Most of these studies reported only on TC( Reference Kitahara, Berrington de and Freedman 5 , Reference Strohmaier, Edlinger and Manjer 10 – Reference Vatten and Foss 14 , Reference Steenland, Nowlin and Palu 17 – Reference Fagherazzi, Fabre and Boutron-Ruault 21 , Reference Bjorge, Lukanova and Jonsson 33 ). Unless otherwise mentioned below, excluding each study with a low HR in turn and pooling the rest did not affect the summary estimates.
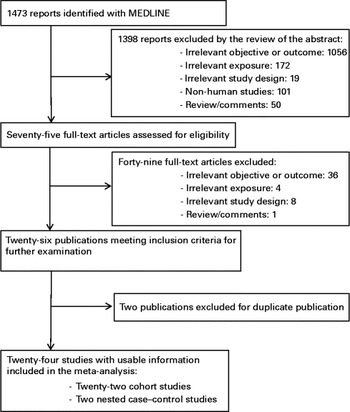
Fig. 1 Flow chart of the study selection process for the prospective association between total cholesterol, HDL-cholesterol, LDL-cholesterol, ApoA1, ApoB and the risk of breast cancer (up to January 2014).
Total cholesterol
For TC and the risk of breast cancer, thirteen cohort studies (21 436 breast cancer cases/1 192 565 participants) were included in the dose–response meta-analysis( Reference Kitahara, Berrington de and Freedman 5 , Reference Melvin, Seth and Holmberg 7 , Reference His, Zelek and Deschasaux 9 – Reference Vatten and Foss 14 , Reference Gaard, Tretli and Urdal 16 – Reference Iso, Ikeda and Inoue 20 ) and fourteen (22 831 cases/1 208 300 participants) in the highest v. lowest meta-analysis( Reference Kitahara, Berrington de and Freedman 5 , Reference Melvin, Seth and Holmberg 7 , Reference His, Zelek and Deschasaux 9 – Reference Steenland, Nowlin and Palu 17 , Reference Eliassen, Colditz and Rosner 19 – Reference Fagherazzi, Fabre and Boutron-Ruault 21 ) (Table 1).
Table 1 Summary hazard ratios (HR) for the meta-analysis of prospective studies investigating total cholesterol (TC), HDL-cholesterol (HDL-C) and LDL-cholesterol (LDL-C) levels and the risk of breast cancer (Hazard ratios and 95 % confidence intervals)
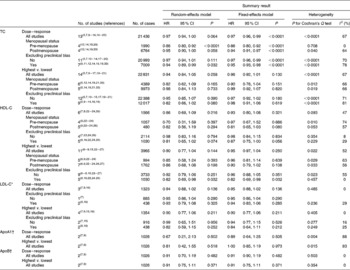
* For LDL-C, no study provided risk estimates for the risk of breast cancer separately in pre- and postmenopausal women.
† For ApoA1 and ApoB, information was only available for the overall meta-analysis.
Overall, the summary HR for the prospective association between TC and the risk of breast cancer was 0·97 (95 % CI 0·94, 1·00) in the dose–response meta-analysis (per 1 mmol/l increment) (Fig. 2(a) and Table 1) and 0·94 (95 % CI 0·84, 1·05) in the highest v. lowest meta-analysis (Fig. 2(b) and Table 1). In both cases, heterogeneity was high (I 2= 67 % for both).
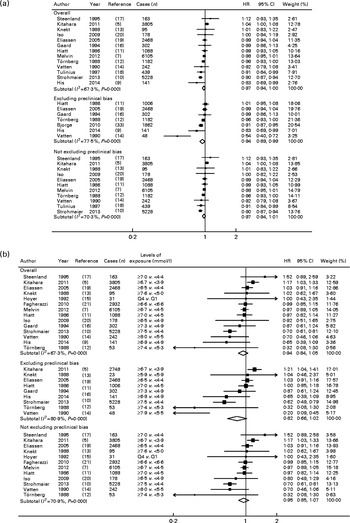
Fig. 2 Meta-analysis of total cholesterol and the risk of breast cancer, overall and separately in studies that did and did not exclude cancer cases diagnosed during the first years of follow-up. (a) Dose–response (for an increment of 1 mmol/l) and (b) highest v. lowest meta-analyses. P values were obtained using the χ2 test for heterogeneity. Q, quartile.
For studies that eliminated preclinical bias, a modest but statistically significant inverse association was observed between TC and the risk of breast cancer in the dose–response meta-analysis (HR 0·94 (95 % CI 0·89, 0·99), seven studies, I 2= 78 %; Fig. 2(a) and Table 1). When testing the influence of each individual study with low HR( Reference His, Zelek and Deschasaux 9 , Reference Tornberg, Holm and Carstensen 12 , Reference Vatten and Foss 14 , Reference Hoyer and Engholm 15 ), this result became statistically non-significant when the study of His et al. ( Reference His, Zelek and Deschasaux 9 ) was excluded (HR 0·95 (95 % CI 0·90, 1·01), I 2= 79 %), while the exclusion of other studies did not modify the findings. The HR for the highest v. lowest analysis was 0·82 (95 % CI 0·66, 1·02, nine studies, I 2= 81 %; Fig. 2(b) and Table 1). This inverse association tended to be slightly stronger among pre-menopausal women (dose–response: all studies – HR 0·86 (95 % CI 0·80, 0·92), I 2= 0 %, four studies (Table 1); in studies without preclinical bias – HR 0·79 (95 % CI 0·66, 0·94), I 2= 71 %, four studies (data not shown)), whereas the corresponding results were not statistically significant among postmenopausal women (dose–response: all studies – HR 0·95 (95 % CI 0·90, 1·00), I 2= 64 %, four studies (Table 1); in studies without preclinical bias – HR 0·94 (95 % CI 0·85, 1·04), I 2= 87 %, two studies (data not shown)) (P for meta-regression by menopausal status = 0·023).
Within the studies that excluded preclinical bias, no heterogeneity was detected according to the number of years of follow-up excluded (P= 0·7). Similarly, other factors tested by the meta-regression as potential sources of heterogeneity were not statistically significant (all P>0·05), except for geographical location (P meta-regression= 0·012 for dose–response analyses). In stratified analyses, an inverse association between TC and the risk of breast cancer was observed for the dose–response meta-analysis of European studies (HR 0·94 (95 % CI 0·91, 0·98), I 2= 55 %, eight studies), but not for American studies (P= 0·800, three studies) and Asian studies (P= 0·051, two studies).
There was a suggestion of potential non-linearity (P non-linearity= 0·03) in the analysis including all studies (ten studies with the required data; see online Supplementary Fig. S1(a)). However, the non-linearity appeared to be restricted to the studies with the risk of preclinical bias as there was no evidence of non-linearity when the analysis was restricted to the six studies that controlled for preclinical bias (P non-linearity= 0·30; see online Supplementary Fig. S1(b)). Also, the curve for the studies that did not account for preclinical bias (eight studies, P non-linearity= 0·06) was similar to that observed for all studies (see online Supplementary Fig. S1(c)).
HDL-cholesterol
For HDL-C and the risk of breast cancer, five cohort studies( Reference Melvin, Seth and Holmberg 7 , Reference His, Zelek and Deschasaux 9 , Reference Furberg, Veierod and Wilsgaard 23 , Reference Kucharska-Newton, Rosamond and Mink 24 , Reference Kabat, Kim and Chlebowski 26 ) and one nested case–control study( Reference Moorman, Hulka and Hiatt 22 ) (1566 cases/56 042 participants) were included in the dose–response meta-analysis, and nine cohort( Reference Bosco, Palmer and Boggs 6 – Reference His, Zelek and Deschasaux 9 , Reference Hoyer and Engholm 15 , Reference Furberg, Veierod and Wilsgaard 23 – Reference Kabat, Kim and Chlebowski 26 ) and two nested case–control( Reference Moorman, Hulka and Hiatt 22 , Reference Agnoli, Berrino and Abagnato 27 ) studies (3965 cases/178 564 participants) were included in the highest v. lowest meta-analysis.
Overall, the summary HR for the prospective association between HDL-C and the risk of breast cancer was 0·86 (95 % CI 0·69, 1·09) in the dose–response meta-analysis (per 1 mmol/l increment) (Fig. 3(a) and Table 1) and 0·90 (95 % CI 0·77, 1·04) in the highest v. lowest meta-analysis (Fig. 3(b) and Table 1). In both cases, heterogeneity was moderate (I 2= 47 and 52 %, respectively). Regarding studies that eliminated preclinical bias, a modest inverse association was observed between HDL-C and the risk of breast cancer, which was statistically significant in the highest v. lowest meta-analysis (HR 0·82 (95 % CI 0·69, 0·98), five studies, I 2= 0 % (no heterogeneity); Fig. 3(b) and Table 1). This result became statistically non-significant when the study of His et al. ( Reference His, Zelek and Deschasaux 9 ) was excluded (HR 0·86 (95 % CI 0·71, 1·04), I 2= 0 %). The HR for the dose–response analysis was 0·81 (95 % CI 0·65, 1·02, five studies, I 2= 30 %; Fig. 3(a) and Table 1).
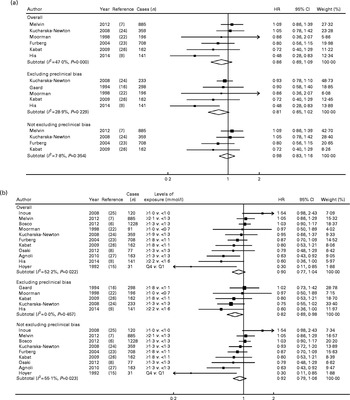
Fig. 3 Meta-analysis of HDL-cholesterol and the risk of breast cancer, overall and separately in studies that did and did not exclude cancer cases diagnosed during the first years of follow-up. (a) Dose–response (for an increment of 1 mmol/l) and (b) highest v. lowest meta-analyses. P values were obtained using the χ2 test for heterogeneity. Q, quartile.
The results stratified by menopausal status were all non-statistically significant for HDL-C, except for an inverse association observed in the highest v. lowest meta-analysis of studies that excluded preclinical bias, in pre-menopausal women (HR 0·45 (95 % CI 0·31, 0·67), I 2= 0 %, three studies; P for meta-regression by menopausal status = 0·043; data not shown).
Other factors tested by the meta-regression as potential sources of heterogeneity were not statistically significant (all P>0·05), including the number of years of follow-up excluded in studies that accounted for preclinical bias (P= 0·6).
Since the non-linear analysis requires a sufficient number of studies (n≥ 5) that provided complete data for at least four categories of exposure, it could not be performed for other relationships studied than TC. However, visual inspection of data across the categories of exposure in individual studies did not suggest a non-linear association between HDL-C and the risk of breast cancer.
LDL-cholesterol
For LDL-C and the risk of breast cancer, only three cohort studies( Reference Melvin, Seth and Holmberg 7 , Reference His, Zelek and Deschasaux 9 , Reference Gaard, Tretli and Urdal 16 ) (1323 cases/68 373 participants) provided the risk estimates for the dose–response meta-analysis, and four cohort studies (1354 cases/73 580 participants) were included in the highest v. lowest meta-analysis( Reference Melvin, Seth and Holmberg 7 , Reference His, Zelek and Deschasaux 9 , Reference Hoyer and Engholm 15 , Reference Gaard, Tretli and Urdal 16 ).
No statistically significant association was observed between LDL-C and the risk of breast cancer in the dose–response (P= 0·136) and highest v. lowest (P= 0·211) meta-analyses, with no heterogeneity (I 2= 0 %) (Table 1).
ApoA1 and ApoB
Only two prospective studies investigated the association between ApoA1 and ApoB and the risk of breast cancer( Reference Melvin, Seth and Holmberg 7 , Reference His, Zelek and Deschasaux 9 ) (1026 cases/38 510 participants). Regarding ApoA1, one study observed an inverse association with the risk of breast cancer (HR 0·36 (95 % CI 0·18, 0·73))( Reference His, Zelek and Deschasaux 9 ) and one did not observe any association( Reference Melvin, Seth and Holmberg 7 ). Regarding ApoB, both studies observed no association with the risk of breast cancer( Reference Melvin, Seth and Holmberg 7 , Reference His, Zelek and Deschasaux 9 ). In both cases, summary estimates were not significant (Table 1).
Ratios
We performed the meta-analysis of two studies that provided the results on the association between the risk of breast cancer and the TC:HDL-C and LDL-C:HDL-C ratios( Reference Melvin, Seth and Holmberg 7 , Reference His, Zelek and Deschasaux 9 ). The results were not significant (HR 0·99 (95 % CI 0·81, 1·20), I 2= 0 % for the LDL-C:HDL-C ratio and HR 1·01 (95 % CI 0·75, 1·36), I 2= 28 % for the TC:HDL-C ratio.
Specific results according to breast cancer types
Only one study investigated the association between cholesterol level and the risk of breast cancer by taking the histological type into account( Reference His, Zelek and Deschasaux 9 ). That study found an inverse association between the risk of ductal breast cancer and HDL-C (HR 0·39 (95 % CI 0·20, 0·76)) and ApoA1 (HR 0·33 (95 % CI 0·14, 0·77)) but not TC and ApoB. However, lobular and other histological types could not be tested in that study due to an insufficient number of cases.
Only three studies provided information regarding hormonal receptor status( Reference His, Zelek and Deschasaux 9 , Reference Eliassen, Colditz and Rosner 19 , Reference Fagherazzi, Fabre and Boutron-Ruault 21 ). All the results from these studies were not statistically significant with regard to blood TC level and oestrogen receptor (ER)+/progesterone receptor (PR)+( Reference Soran, Hama and Yadav 2 , Reference His, Zelek and Deschasaux 9 , Reference Eliassen, Colditz and Rosner 19 ), ER − /PR − ( Reference Eliassen, Colditz and Rosner 19 , Reference Fagherazzi, Fabre and Boutron-Ruault 21 ), ER+/PR − (21) and ER − /PR+( Reference Soran, Hama and Yadav 2 ) breast cancer types. Of these studies, one investigated the relationship between HDL-C and the risk of ER+/PR+ breast cancer, and found a significant inverse association (HR 0·41 (95 % CI 0·21, 0·97))( Reference His, Zelek and Deschasaux 9 ). However, that study did not investigate other receptor types due to insufficient statistical power.
All the results of the meta-analysis were similar or slightly improved in terms of statistical significance when fixed-effects models were used instead of random-effects models (Table 1).
Discussion
To our knowledge, the present study is the first systematic review and meta-analysis of all prospective studies that investigated the associations between several parameters of blood cholesterol levels and the risk of breast cancer. Since preclinical bias may largely influence these associations, the stratified meta-analysis separating studies that did or did not exclude cancer cases diagnosed during the first years of follow-up represents an important strength. Indeed, the presence of a tumour is likely to influence blood cholesterol concentrations by several mechanisms( Reference Jacobs and Gapstur 28 – Reference Dessi, Batetta and Pulisci 32 ). The meta-analysis conducted in studies that eliminated potential preclinical bias suggested a modest but statistically significant inverse association between pre-diagnostic blood levels of TC and HDL-C and the risk of breast cancer. While heterogeneity was low for HDL-C, it remained high for TC. The latter result should thus be interpreted with caution. These complementary investigations allowed us to draw an overview of the prospective relationships between the different blood cholesterol parameters and the risk of breast cancer.
Several elements suggest that the association with TC could in fact be driven by HDL-C, although further studies are needed to confirm this interpretation. First, only HDL-C, but not LDL-C (the other important component of TC) was associated with the risk of breast cancer. In line with this finding, a recent meta-analysis has shown no association between statins, which mainly influence LDL-C concentrations, and the overall risk of cancer( Reference Mihaylova, Emberson and Blackwell 50 ).
Second, mechanistic data also support the plausibility of the observed inverse associations between cholesterol, more specifically HDL-C, and the risk of breast cancer. Indeed, experimental studies have shown that HDL presents antioxidant and anti-inflammatory properties( Reference Soran, Hama and Yadav 2 , Reference von Eckardstein, Hersberger and Rohrer 3 , Reference Esteve, Ricart and Fernandez-Real 51 ). HDL plays a role in the inhibition of the LDL oxidation cascade( Reference Soran, Hama and Yadav 2 , Reference von Eckardstein, Hersberger and Rohrer 3 ), and thus it could reduce oxidative stress. The ability of apoA1 (the major protein component of HDL-C in serum( Reference Huang, Silva and Jerome 52 )) to inhibit cell proliferation and cell-cycle progression, shown in vascular smooth muscle cells, is also a possible involved mechanism( Reference von Eckardstein, Hersberger and Rohrer 3 ). A recent study( Reference de Medina, Paillasse and Segala 53 ) has revealed a cholesterol metabolite (dendrogenin A) that triggers breast cancer cell redifferentiation and improves survival in animal models.
Third, regarding studies that excluded cancer cases in the early follow-up, HDL-C was found to be inversely associated with the risk of breast cancer (five studies), and all heterogeneity disappeared. In contrast, TC was also inversely associated with the risk of breast cancer (seven studies included in the dose–response meta-analysis), but heterogeneity remained high (I 2= 78 %) even when considering the studies that eliminated preclinical bias. Thus, the result on TC should be interpreted with caution. This point is in favour of a more robust relationship between the risk of breast cancer and HDL-C than TC, and consistently suggests that the result of TC may in fact be driven by the HDL-C result.
Apart from the potential influence of preclinical bias, several other possible sources of heterogeneity were investigated by the meta-regression and stratified analysis where appropriate. Notably, stratification by menopausal status was performed. While no statistically significant result was observed in the postmenopause, several inverse associations were statistically significant in the pre-menopause between the risk of breast cancer and TC and HDL-C levels, and the P value of the meta-regression by menopausal status was statistically significant. Although this may be a false positive result due to first-kind error, menopause influences both sex hormone levels and cholesterol metabolism( Reference Kolovou and Bilianou 54 ). In addition, pre- and postmenopausal breast cancers are differentially associated with body adiposity and are heterogeneous in terms of receptor status( 55 ). Thus, a different association of cholesterol parameters with pre- and postmenopausal breast cancers might be possible. The present results need to be confirmed and research on mechanisms is warranted to elucidate this specific aspect.
All other sources of heterogeneity were not statistically significant in meta-regression analyses, except for broad geographical region in the dose–response meta-analysis for TC, for which a statistically significant inverse association with the risk of breast cancer was observed only in European but not in American and Asian studies. However, even in European studies, this stratification did not substantially decrease heterogeneity, which remained high.
There was no influence of the number of years of follow-up excluded within studies that accounted for preclinical bias, suggesting that 1 year may already be sufficient to avoid main bias effects.
As it is usually the case in the highest v. lowest meta-analysis, compared categories of blood cholesterol levels varied across the studies. However, this variation was limited since the range of blood cholesterol concentrations is relatively similar across human populations (unlike other more fluctuant parameters such as dietary intakes). According to the National Cholesterol Education Program (NCEP) Adult Treatment Panel III( 56 ), TC levels < 5·2 mmol/l and >6·2 mmol/l are considered as ‘desirable’ and ‘high’, respectively, and HDL-C level < 1·3 mmol/l is considered as ‘low’ in women and is one of the criteria for the metabolic syndrome. Higher and lower cut-offs used in most studies were consistent with these reference values. Although the highest v. lowest meta-analysis is less ‘precise’, it requires less information than the dose–response meta-analysis, and thus it can include more available studies. Both types of meta-analysis are thus complementary. In addition, the present results were overall consistent between the two types of meta-analysis (same direction and same order of magnitude), which strengthen the consistency of our findings. However, for TC, a significant result was observed for the dose–response analysis, while for HDL-C, a significant result was found for the highest v. lowest analysis.
The limitations of the present study should be considered. First, it is possible that the observed relationships could be partly due to unmeasured or residual confounding. For instance, relatively few studies adjusted for lifetime oral contraceptive use (see online Supplementary Table S2), which is potentially related to both cholesterol levels and the risk of breast cancer. Similarly, only three studies( Reference Bosco, Palmer and Boggs 6 , Reference Iso, Ikeda and Inoue 20 , Reference Fagherazzi, Fabre and Boutron-Ruault 21 ) controlled for diabetic condition and none controlled for insulin levels (see online Supplementary Table S2). Only one study( Reference Fagherazzi, Fabre and Boutron-Ruault 21 ) excluded subjects who were on lipid-lowering medications as an initial exclusion criterion (but two studies( Reference His, Zelek and Deschasaux 9 , Reference Iso, Ikeda and Inoue 20 ) adjusted for this factor). However, most of the studies included in the meta-analysis adjusted for major known risk factors of breast cancer, and when it was not the case, the influence of the adjustment for these factors was tested by the meta-regression in the present study (and did not influence the findings). Thus, residual confounding is not likely to entirely explain our findings. Second, the imperfections associated with published information may constitute limitations of the meta-analyses. Notably, lack of information about therapeutic strategies potentially influencing lipid biomarkers during the follow-up (such as treatments for metabolic disorders), as well as a single measurement of cholesterol biomarkers at baseline represent limitation of most individual studies included in these meta-analyses. However, it has been shown that lipids and lipoprotein biomarkers could be considered reliable enough to be used in cohort studies, based on measurements made at two different time points several years apart( Reference Al-Delaimy, Jansen and Peeters 57 ). Third, only the MEDLINE database was searched. However, this approach is often used in meta-analysis on health-related topics( Reference Aune, Chan and Greenwood 58 , Reference Aune, Norat and Romundstad 59 ), and in this field, the use of other databases such as Embase usually does not retrieve additional relevant articles compared with those already retrieved by the MEDLINE search. In addition, we systematically screened the references of the relevant articles, reviews and meta-analyses retrieved by our search, and we could not identify any missing study. Next, available information did not allow us to meta-analyse the associations between blood cholesterol parameters and the risk of breast cancer according to histological and receptor types. This would be interesting information since oestrogens may have hypolipidaemic properties that potentially influence cholesterol metabolism( Reference De Marinis, Martini and Trentalance 60 ). Thus, the hypothesis of a differential association between cholesterol and different breast cancer types is plausible and should be further investigated. Finally, in some analyses, our statistical power was limited and did not allow us to cumulate several levels of stratification and thus several potential sources of heterogeneity that could exert cumulative or opposite effects on the summary result.
In conclusion, the quantitative summary of the existing evidence from prospective studies supports a modest yet significant inverse association between cholesterol (high heterogeneity), more specifically HDL-C (low heterogeneity), and the risk of breast cancer. These associations are supported by mechanistic plausibility from experimental studies( Reference Soran, Hama and Yadav 2 , Reference von Eckardstein, Hersberger and Rohrer 3 , Reference de Medina, Paillasse and Segala 53 ). From an aetiological standpoint, it appears highly interesting to more thoroughly elucidate the implication of cholesterol and its components in carcinogenesis. Before being able to formulate official recommendations for health professionals and the general population, these results need to be confirmed by further large prospective observational studies and randomised clinical trials that adequately control for preclinical bias and for all major potential confounders. Also, these further studies should be able to elucidate whether TC has an effect per se or only via HDL-C, as hypothesised here. If the latter is confirmed, efforts would be made to develop lifestyle and pharmacological interventions that would have a positive impact on blood HDL-C levels to contribute to primary breast cancer prevention, in line with recommendations for cardiovascular health.
Supplementary material
To view supplementary material for this article, please visit http://dx.doi.org/10.1017/S000711451500183X
Acknowledgements
The present study was supported by the French National Institute for Health and Medical Research (INSERM U1153), the French National Institute of Agronomy Research (INRA U1125), and Paris 13 University.
The authors' responsibilities were as follows: M. T. and P. L.-M. designed the research; M. T., P. F., M. H., N. D.-P. and P. L.-M. conducted the research; P. F. analysed the data; M. T. and P. F. wrote paper; M. T., P. F., M. H., T. N., D. S. M. C., J. B., S. H., P. G., N. D.-P. and P. L.-M. contributed to the data interpretation and revised each draft of the manuscript for important intellectual content; M. T. had primary responsibility for the final content. All authors read and approved the final manuscript.
The authors declare that they have no conflict of interest.