Introduction
The use of mobile technology for mental health service delivery offers the potential to extend the reach and ultimately the public health impact of care, reducing the burdens associated with psychological disorders and symptoms (Kazdin and Rabbitt, Reference Kazdin and Rabbitt2013). This potential has sparked much excitement in the mental health space with the development, dissemination and implementation of numerous technologies, including a variety of mobile mental health applications (‘MH apps’). For the individual practitioner, the immediate appeal of MH apps probably relates to their offering novel ways to deliver existing interventions which can be integrated into existing treatment plans, rendering them more efficient, effective, convenient and client-friendly (Erhardt and Dorian, Reference Erhardt and Dorian2013; Lui et al., Reference Lui, Marcus and Barry2017; Schueller et al., Reference Schueller, Washburn and Price2016). Indeed, many MH apps aim to facilitate the implementation of core components of established and evidence-based treatments (Bakker et al., Reference Bakker, Kazantzis, Rickwood and Rickard2016; Bush et al., Reference Bush, Armstrong and Hoyt2019; Karcher and Presser, Reference Karcher and Presser2018).
The adjunctive use of digital mental health tools, including MH apps, to enhance the delivery of interventions in the context of traditional, face-to-face psychotherapy has been referred to as blended care (Neary and Schueller, Reference Neary and Schueller2018; Wentzel et al., Reference Wentzel, van der Vaart, Bohlmeijer and van Gemert-Pijnen2016). Although some MH apps (e.g. CPT Coach; PE Coach) are designed specifically to be used as therapy adjuncts, in practice, a wide variety of MH apps including many designed to be stand-alone, self-help resources, can be appropriated for blended care regardless of whether they were initially designed for that purpose. The potential advantages of a blended care approach include increases in client engagement and adherence, and extending care into ‘real-world’ contexts (Bush et al., Reference Bush, Armstrong and Hoyt2019; Erhardt and Dorian, Reference Erhardt and Dorian2017; Lui et al., Reference Lui, Marcus and Barry2017; National Institute of Mental Health, 2016). MH apps can also help make specific clinical skills more concrete for the client through interactive digital tools, in addition to providing helpful prompts such as notifications or reminders (Muroff and Robinson, Reference Muroff and Robinson2020). A recent meta-analysis supports the use of MH apps in a blended care model, with a number of trials finding a small advantage for the use of MH apps as adjuncts to traditional interventions over traditional interventions alone with respect to improvements in depressive symptoms (Linardon et al., Reference Linardon, Cuijpers, Carlbring, Messer and Fuller-Tyszkiewicz2019). Thus, it is not surprising that many rigorous evaluations of MH apps in clinical settings have used human-supported delivery models (e.g. Graham et al., Reference Graham, Greene, Kwasny, Kaiser, Lieponis, Powell and Mohr2020).
Although most practitioners report themselves to be receptive to the idea of using MH apps to support their interventions, their actual adoption rates appear to be quite low (Ben-Zeev, Reference Ben-Zeev2016). In separate surveys, Schueller and colleagues found adoption rates of 0.9% and zero, respectively, among providers who were and were not affiliated with healthcare systems (Schueller et al., Reference Schueller, Washburn and Price2016). Challenges facing practitioners who are interested in using MH apps in their practice include: (1) identifying specific MH apps to consider from more than 10,000 available (Carlo et al., Reference Carlo, Hosseini Ghomi, Renn and Areán2019), (2) evaluating apps of interest along dimensions relevant to clinical practice including alignment with therapeutic goals, faithful representation of target constructs and skills, evidence of usefulness, ease of use, and attention to data security and privacy issues (Edwards-Stewart et al., Reference Edwards-Stewart, Alexander, Armstrong, Hoyt and O’Donohue2019; Gagnon et al., Reference Gagnon, Ngangue, Payne-Gagnon and Desmartis2016), (3) knowing which app to recommend to a client and how, and (4) integrating an app into practice in a way consistent with one’s treatment conceptualization. Psihogios et al. (Reference Psihogios, Stiles-Shields and Neary2020) propose a framework to support providers facing these challenges. The process includes four stages: (1) narrow in on the target problem, end user, and contender apps; (2) explore contender apps in terms of scientific and theoretical support, user experience, and data security and privacy, (3) contextualize if a technology is a good fit for a particular patient in terms of their age, race/ethnicity, ability, gender, sexual orientation, technology access, etc., and (4) recommend/re-evaluate on a continuous basis, checking in with the client regularly to discuss app use and benefits. More detailed guidance on each of these stages is provided by Psihogios et al. (Reference Psihogios, Stiles-Shields and Neary2020). Given that these steps largely conform to the challenges clinicians face in identifying MH apps to use in their practice, empowering clinicians to complete these steps could help support MH app adoption. Important for the purposes of this paper, clinicians need information useful for each of these stages and synthesized in ways that reduces the time required to narrow, explore, contextualize and recommend.
Efforts to help clinicians identify and appraise apps include app rating platforms (e.g. One Mind PsyberGuide, MindTools) that describe, review and evaluate publicly available apps (Neary and Schueller, Reference Neary and Schueller2018) and proposed guidelines for evaluating MH apps (e.g. the Mobile App Rating Scale, Stoyanov et al., Reference Stoyanov, Hides, Kavanagh, Zelenko, Tjondronegoro and Mani2015; the Enlight Rating Guidelines, Baumel et al., Reference Baumel, Faber, Mathur, Kane and Muench2017; the American Psychiatric Association’s Evaluation Framework, Torous et al., Reference Torous, Chan, Gipson, Kim, Nguyen, Luo and Wang2018). However, as useful as platforms and guidelines may be, they often lack the detailed information that would be informative for practitioners. Specifically, rating platforms rarely drill down into the details of how faithfully specific clinical interventions are represented (Neary and Schueller, Reference Neary and Schueller2018). Rating platforms might also have challenges keeping reviews up to date, which will necessitate practitioner reviews (Carlo et al., Reference Carlo, Hosseini Ghomi, Renn and Areán2019). However, it is unlikely that practitioners themselves will have the time to follow guidelines and evaluate a multitude of apps in order to help them determine which to use. The current review aims to address the lack of detailed information about fidelity to evidence-based content, at least with respect to apps designed to replicate a specific widely used technique that represents a staple of cognitive behavioural therapy (CBT) – cognitive restructuring. In doing so, this work also aims to provide a model for future evaluation of other types of MH apps that can provide a helpful adjunct to existing resources to inform adoption and use of clinical tools.
CBT apps are worth considering because of their popularity and the consistent evidence demonstrating their efficacy. A meta-analytic review found that CBT apps produced larger effects on multiple outcomes than non-CBT apps (Linardon et al., Reference Linardon, Cuijpers, Carlbring, Messer and Fuller-Tyszkiewicz2019). At the same time, however, many publicly available MH apps do not include CBT content (Wasil et al., Reference Wasil, Venturo-Conerly, Shingleton and Weisz2019), even among those that claim they do (Huguet et al., Reference Huguet, Rao, McGrath, Wozney, Wheaton, Conrod and Rozario2016). As such, evaluating at a granular level how specific MH apps implement particular CBT skills could be useful in helping clinicians decide which ones to adopt, and developers and researchers to better understand the gaps and opportunities that exist in this space.
A core component of CBT is cognitive restructuring (CR). Integral to the cognitive aspect of CBT, CR is defined as ‘intervention strategies that focus on the exploration, evaluation, and substitution of the maladaptive thoughts, appraisals, and beliefs that maintain psychological disturbance’ (Clark, Reference Clark2014; p. 2). In practice, CR involves teaching individuals how to identify, evaluate, and modify cognitions that appear to be contributing to their distress and/or dysfunction (Beck, Reference Beck2020). CR has traditionally been implemented in clinical practice through the use of the pen-and-paper thought record (Wright et al., Reference Wright, Basco and Thase2006). Thought records provide clients with a structured, multi-column form to help them navigate and document the CR process. This traditional thought record provides a well-established structure, including a sequenced series of guiding prompts, which can be translated to a digital version with relative ease. This, along with the widespread use of thought records, their nearly universal representation in the training of cognitive behavioural therapists, and their 40+ year history of being intertwined with CR, has led to an increased number of digitized thought records available in the MH app marketplace.
The confluence of CBT’s status as the most widely practised, empirically supported form of psychotherapy (Beck, Reference Beck2020; David et al., Reference David, Cristea and Hofmann2018; Gaudiano, Reference Gaudiano2008; Kennerley et al., Reference Kennerley, Kirk and Westbrook2016; Shafran et al., Reference Shafran, Clark, Fairburn, Arntz, Barlow, Ehlers, Freeston, Garety, Hollon, Ost, Salkovskis, Williams and Wilson2009), the centrality of thought record-assisted CR to cognitive therapy, and the rising expectations for practitioners to adopt MH apps suggests an acute need for practitioners to familiarize themselves with currently available CR apps so as to make informed choices as to which might add value to their practices. Currently, resources are lacking to guide practitioners specifically with respect to CR-oriented apps that include digitized versions of thought records. Thus, the current study aimed to inform practitioners about publicly available MH apps amenable for use within blended models of treatment to promote CR skills through the use of digitized thought records. We pursued this by conducting a systematic review of publicly available apps that include elements of CR based on thought records, and characterized the features available within these apps. Specific goals included providing sufficient descriptive and comparative information to empower practitioners to make optimal choices regarding which MH apps implement CR with fidelity and therefore which MH apps might be able to be integrated into their practice. Moreover, in an effort to provide as much useful and actionable guidance as possible, the information obtained through the review was used to provide illustrative examples of the strongest representations of thought record-based CR among these apps.
Method
Overview
We identified and reviewed available CR apps for their fidelity to CR, general app features, and user experience. The process of collecting and reviewing apps for CR elements was conducted by a team of five reviewers: two PhD-level clinical psychologists, both with expertise in digital mental health, two masters-level researchers, one with experience in providing clinical services, and one bachelors-level reviewer. Each member of the team had experience in digital mental health and training and expertise in development or evaluation of MH apps. User experience reviews were completed by a separate team of four raters, who were not the same team who conducted the app selection and coded the CR elements, but were trained in reviewing mHealth apps. This training consisted of watching a video tutorial on using the user experience questionnaire. As part of the training process, each rater rates two apps and then meets with the developer of the scale to justify the score on each item, discuss the process, and ask questions. This training typically takes 3–4 hours plus time spent scoring apps outside of training sessions. Two of these raters were PhD-level reviewers, each with expertise in user experience and mental health app reviews, and two were undergraduate psychology students with lived experience of mental health issues and familiarity with psychological treatments commensurate with their level of education. Each app was rated by three individuals, the two PhD-level reviewers and one of the two individuals with lived experience.
App selection process
We used a structured review process that spanned from May 2020 to January 2021 to identify and evaluate the CR apps, mirroring processes used in previous app reviews (e.g. O’Loughlin et al., Reference O’Loughlin, Neary, Adkins and Schueller2019). This structured process follows what has been described as a systematic search of mobile app platforms which helps synthesize user-centred metrics such as content and user experience. This approach supplements traditional reviews using searches of academic databases (e.g. PsychInfo, SCOPUS), which tend to under-represent the types of publicly accessible consumer apps that were the focus of this study (Lau et al., Reference Lau, O’Daffer, Yi-Frazier and Rosenberg2021), by examining apps that are publicly available on consumer app marketplaces. During May 2020, we first identified potentially relevant apps by searching the Apple App Store and the Android Google Play Store using a combination of the following search terms, resulting in 35 relevant permutations: anxiety, depression, mood, worry, cognitive behavior/al (CBT), therapy, cognitive restructuring, thinking, thought, calendar, diary, journal, log, notes, record, tracker, feeling, negative, icbt, cognitive psychology and mental health. This search was conducted using a Python code, rather than searching these stores directly on team members’ personal devices, in order to overcome differences in searches that might occur due to search histories. We paralleled previous studies (e.g. O’Loughlin et al., Reference O’Loughlin, Neary, Adkins and Schueller2019; Huckvale et al., Reference Huckvale, Torous and Larsen2019) by retaining up to the first 10 available results from each keyword search. This approach is supported by research suggesting that most users do not look past the top 10 search results or download apps beyond the top five search results (Dogruel et al., Reference Dogruel, Joeckel and Bowman2015). We elected to limit our search to the Android Google Play Store and Apple App Store because these are by far the largest app marketplaces with 3.48 million and 2.22 million apps, respectively, as of 2021 (Statista, 2021). The dominance of these app stores is clear when one considers that the number of apps offered by the two next largest app marketplaces in 2021 was 669,000 for the Windows Store and 460,000 for the Amazon App Store. We collected up to the first 10 results for all 35 keywords on both iOS and Android. This resulted in an initial total of 572 apps (as some combinations produced less than 10 apps). This was then reduced to a total of 246 apps following the removal of 326 duplicates.
Inclusion/exclusion criteria
Figure 1 provides an overview of the app identification process, including the inclusion and exclusion criteria applied at each stage. Apps met inclusion criteria if they (1) were available in English for the U.S. market; (2) were updated within two years prior to the keyword search, (3) had an average user rating of ≥ 3 stars (out of 5); and (4) had an app description that suggested the presence of a cognitive-restructuring (CR) element based on a digitized version of a thought record. Presence of a CR element was operationalized based on its consistent representation across four expert sources describing the process of thought record-based CR (viz., Beck, Reference Beck2020; Greenberger and Padesky, Reference Greenberger and Padesky2016; Leahy, Reference Leahy2003; Wright et al., Reference Wright, Basco and Thase2006) and key terms in the app descriptions including thoughts records and diaries; cognitive restructuring and reframing; and identifying, tracking, and reflecting on thoughts and emotions. At this stage, which commenced in June 2020, apps were excluded if they were (1) not available or accessible to the reviewers (for example, if they were not functioning or had been removed from the app stores), (2) required payment or subscription to access any of the thought-record based CR features, or (3) did not have a CR element present within the app. Of the 246 non-duplicate apps selected for review, 210 were excluded during review of their app descriptions, resulting in 36 remaining eligible apps.
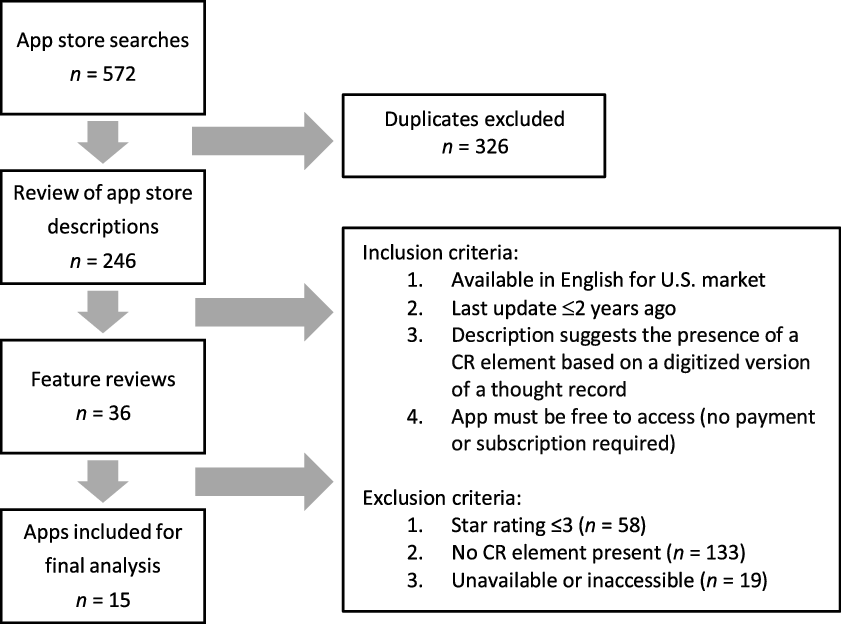
Figure 1. Overview of app selection and review process.
Review of CR elements
Each of the 36 eligible apps was downloaded and reviewed by two of the five reviewers, each of whom completed a feature review. Feature reviews began in September 2020 and continued until January 2021.This in-depth feature review revealed that 21 apps initially identified as CR apps only involved identifying situations, emotions or thoughts, but did not include developing an alternative thought, which is essential to the process of CR (i.e. these apps contained no restructuring component). Thus, only 15 apps were included in the final analysis.
Apps were coded by two members of the five-member reviewing team to identify the presence or absence of each CR element (shown in Table 1). Every app was double-rated for CR elements (82.7% agreement, kappa = .64), and, when necessary, coded by a third reviewer to resolve any discrepancies. Two of the five members coded the majority of apps (28 and 26 apps, respectively), with the remaining raters coding fewer apps or resolving discrepancies (8 and 7 initial apps coded and 5, 6, and 2 discrepancies resolved). App assignments were done randomly but preferenced the two reviewers available to complete the majority of ratings. Discrepancies were resolved by the PhD-level psychologists unless they were involved in the discrepancies that defaulted to the masters-level review. Discrepancies identified were discussed at the end of the reviewing process in January 2021. For apps that included in-app subscriptions, only free elements were evaluated, as research on consumer attitudes towards mental health apps show that people are more likely to choose free apps and commonly express reservations about committing to in-app subscriptions (Schueller et al., Reference Schueller, Neary, O’Loughlin and Adkins2018). App reviewers spent between 30 and 60 minutes exploring each app and its different features to complete the coding. This time was sometimes spread over multiple days when a specific app’s features required multiple days to use or unlock certain features (e.g. tracking features, sequentially released didactic modules). Features were tested on iOS and/or Android platforms, depending on availability of the app on each platform. In total, of the 36 initially eligible apps, 25 were reviewed on iOS and nine were reviewed on Android.
Table 1. Selected mental health apps sorted by the number of CR elements present

Each app’s thought record-based CR tool was coded to determine whether users were prompted to engage in the following five distinct ‘core’ components of CR (as determined by the aforementioned review of expert sources): (1) identify the situation in which distress occurred, (2) label emotions, (3) identify automatic thoughts, (4) generate adaptive alternative thoughts, and (5) evaluate the outcome with respect to changes to emotions and/or overall distress. Within each of these categories, reviewers provided detailed notes and screenshots to support their ratings regarding the presence or absence of these features. Given the variability in how each of the five ‘core’ CR components was represented across apps, each app was also evaluated with respect to the presence or absence of ‘sub-elements’ of CR. In order to identify these sub-elements, the review of expert sources included delineation of what specific components comprised each of the five core elements. For instance, in some cases, the ‘Label Emotions’ component included an intensity rating (sub-element) of the identified emotion whereas others omitted such ratings. The presence or absence of examples to guide the user through each CR component represented another varying sub-element.
Reviewers also coded additional features reflective of various general elements of the app including app content, interoperability/data sharing, professional involvement, disclosures, privacy policies, and data safeguards. These features were selected from various app evaluation frameworks that identify key elements that might guide decision-making when considering adoption of an app (e.g. Neary and Schueller, Reference Neary and Schueller2018; Torous et al., Reference Torous, Chan, Gipson, Kim, Nguyen, Luo and Wang2018).
Review of user experience
We reviewed the user experience for each CR app using the Mobile App Rating Scale (MARS). These reviews were conducted during the same period as the feature reviews, beginning in September 2020. The MARS is an objective and reliable tool for classifying and assessing the quality of mobile health apps (Stoyanov et al., Reference Stoyanov, Hides, Kavanagh, Zelenko, Tjondronegoro and Mani2015). All apps were reviewed on four app quality subscales (Engagement, Functionality, Aesthetics and Information Quality), comprising 19 items. Each item uses a 5-point response scale (1 = inadequate to 5 = excellent). MARS total scores are obtained by averaging the mean of the four subscales. We report a weighted average of total scores, balancing the two reviews completed by PhD-level reviewers and the one review completed by a person with lived experience. The average variance of MARS scores between the raters was 0.32 (SD = 0.23). To monitor inter-rater reliability, the PhD-level raters discussed any scores with a difference greater than 1. The rationale behind each rating was discussed, and reviewers were allowed to adjust their scores. Reliability of the raters with lived experience scores was not judged against the PhD-level reviewers scores as these scores are intended to be a reflection of consumer use and perspectives. The MARS rating scale has been used in numerous studies to evaluate mHealth tools including apps for mindfulness (Mani et al., Reference Mani, Kavanagh, Hides and Stoyanov2015), self-care (Anderson et al., Reference Anderson, Burford and Emmerton2016), well-being (Antezana et al., Reference Antezana, Bidargaddi, Blake, Schrader, Kaambwa, Quinn, Orlowski, Winsall and Battersby2015), and psychoeducation for traumatic brain injuries (Jones et al., Reference Jones, O’Toole, Jones and Brémault-Phillips2020). The MARS has demonstrated good psychometric properties and suitability for quality assessment, including high reliability, objectivity and convergent validity (Terhorst et al., Reference Terhorst, Philippi, Sander, Schultchen, Paganini, Bardus and Messner2020).
Results
App store information
Of the 15 apps reviewed, 11 (73%) were available on both the iOS App Store and Android Google Play Store. Two apps (13%) were available exclusively on iOS and two apps (13%) were available exclusively on Android. The average app store user rating for the iOS apps was 4.5, and the average rating for Android apps was 4.2, both out of 5.
CR elements
Of the 15 apps, seven apps (47%) included all five core elements of CR, six apps (40%) included four elements, and two apps (13%) included two elements. Of the five core elements, identifying automatic thoughts and generating adaptive alternative thoughts were present in all 15 apps (100%), whereas the least frequent element was evaluating the outcome which was present in six apps (40%). Table 1 displays each app reviewed sorted by number of CR elements present, and the frequency of each CR element across apps.
Each CR element consisted of additional sub-elements, which identify more granular aspects of CR that may or may not be contained within each app. Table 2 displays the frequency of each sub-element.
Table 2. Frequency of cognitive restructuring (CR) sub-elements in CR apps

In addition to evaluating the presence of CR elements, reviewers examined the apps for the inclusion of general app elements based on features identified by various app evaluation frameworks including psychoeducation and other specific types of app content, interoperability/data sharing, professional involvement, disclosures, privacy policies, and data safeguards. The frequency of these elements in the reviewed apps is presented in Table 3. The most common of these elements present were privacy policies, summaries of user responses (n = 15), involvement of mental health professionals in app development (n = 14), and psychoeducational content (n = 13). Less common were disclaimers restricting third party data sharing (n = 4), and the ability for clinicians to access user’s data (n = 3). Only two apps claimed to be HIPPAA compliant and only one app claimed to provide data encryption.
Table 3. General app elements present in reviewed CR apps

User experience
Table 4 shows the total MARS scores for each app, as well as the scores for the four subscales. The average MARS score for the 15 reviewed apps is 3.53 out of 5, with a standard deviation of 0.60, which falls between acceptable (3.00/5.00) and good (4.00/5.00). The highest average score by subscale was Functionality (3.90), and the lowest was Engagement (3.23).
Table 4. MARS total scores and subscores of selected apps

Discussion
We conducted a practitioner-focused review of CR apps, breaking down how faithfully they implemented various components of the CR process, the presence of various general app features, and ratings of different aspects of user experience, all of which might be important considerations for practitioners weighing which app to use. Despite the multitude of mental health apps available, we found only a modest number of apps that in their free version offered some version of CR. We note two important aspects of these findings before tying them to the extant literature. First, to be included in our review, apps needed to include functionality that supported identifying adaptive alternative thoughts. Thus, we excluded apps that were merely thought trackers or journals or apps that allowed one to add thoughts onto other types of trackers, like mood tracking apps. Second, our keyword search might be quite similar to the process a practitioner might use to find potential CR apps, and although our initial keyword search identified 246 unique apps, only 15 of those or 6% demonstrated enough adherence to the CR process to be included in our analyses. This discrepancy between the large number of initial apps identified and those included in our analyses supports the need for such a review – to provide practitioners assistance in identifying and differentiating different apps that might be considered for use in clinical practice. Implications of our findings for practitioners embarking upon or engaged in this process are discussed below.
Despite relative similarity in the features present in the apps – 87% contained at least four of the five general CR elements, and 12 of 15 (80%) included steps of identifying the situation, emotion, negative automatic thought, and adaptive alternative thought – we found considerable variance in the user experience of the apps and in the more granular sub-elements of CR. Figure 2 displays the four apps with the highest number of CR elements defined by the total number of sub-elements within each app. Apps varied little in the ability to support users identifying situations and identifying automatic thoughts, with most apps including these elements to some degree. There was more variability in the degree to which apps supported users with labelling emotions, generating adaptive alternative thoughts, and evaluating outcomes. Furthermore, various features that might be quite important for clinical practice – privacy, data security, data exportability by the user, and data access by the practitioner – also varied considerably across the apps. As such, this review shows that not all CR apps are created equal and, as we discuss further below, practitioners would benefit from understanding the differences that exist in such products.
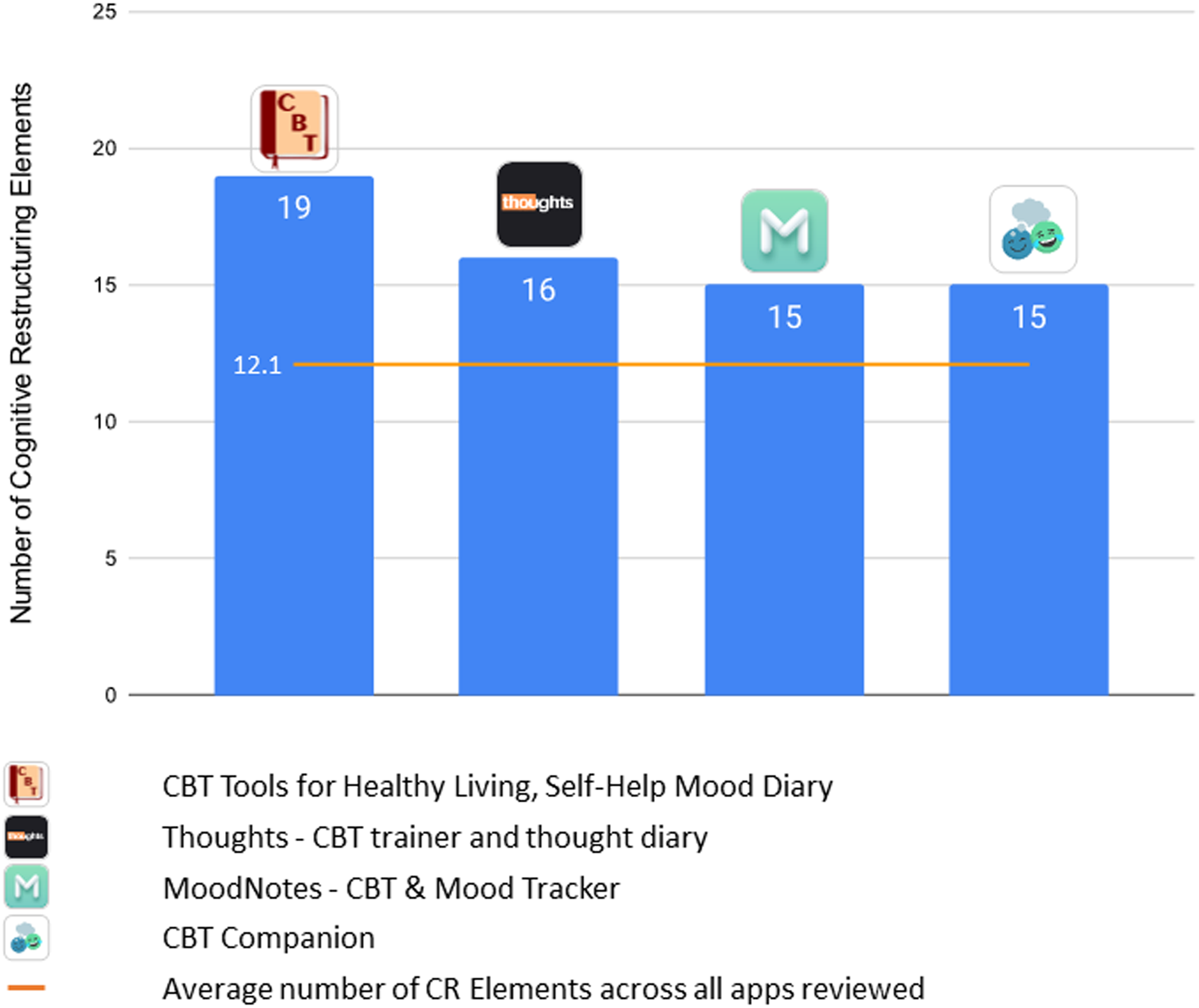
Figure 2. Highest performing apps based on number of CR sub-elements.
As noted, we found considerable variability in user experience (MARS) scores, ranging from a high of 4.58 of 5.00 for Sanvello to a low of 2.30 of 5.00 for iPromptU. Figure 3 displays the four top-performing apps based on these scores. The average MARS total score, however, was still in what is considered the acceptable to good range, demonstrating that overall CR apps, at least those that actually include CR elements in the app and not just suggest so in their description, are generally providing a fairly good user experience. In fact, the average MARS total score of 3.53 was higher than that found for mindfulness apps (Mani et al., Reference Mani, Kavanagh, Hides and Stoyanov2015) – although we note here that our inclusion and exclusion criteria may have resulted in a group of apps with a higher user experience to begin with (e.g. app store star rating of 3+; presence of a CR element). Within the MARS scores, the highest scoring subscale was Functionality and the lowest was Engagement. This suggests that while these apps generally perform well technically, and are easy to use and navigate, they may lack features that help engage users and encourage repeated use. While ease of use is an important feature in mental health apps, interactive features and customization are also valued by those with mental health needs (Schueller et al., Reference Schueller, Neary, O’Loughlin and Adkins2018).

Figure 3. Highest performing apps based on user experiences (MARS) scores.
Even if different apps provide the same features or have similar user experience scores, this does not mean a user’s experience of the app will be the same. What seem like subtle differences between apps in things like colours, images and word choice, might result in meaningful differences in acceptability, adoption and usage among different practitioners and clients. Indeed, other studies that have used human-centred design methods or usability testing to develop or evaluate MH apps have identified these aspects in people’s feedback (Ben-Zeev et al., Reference Ben-Zeev, Kaiser, Brenner, Begale, Duffecy and Mohr2013; Rotondi et al., Reference Rotondi, Sinkule, Haas, Spring, Litschge, Newhill and Anderson2007; Vilardaga et al., Reference Vilardaga, Rizo, Zeng, Kientz, Ries, Otis and Hernandez2018). Figure 4 displays screenshots of the element related to generating adaptive thoughts from each of the four highest performing apps based on user experience. One can see that, unlike traditional paper-based thought records that are fairly general and plain, CR apps are visually stylized. Therefore, although reviews, such as this one, can provide high-level guidance, individual practitioners will still need to explore apps to make decisions and aid in their process of contextualizing an app if it is recommended to a client (Psihogios et al., Reference Psihogios, Stiles-Shields and Neary2020).

Figure 4. Sample CR app approaches to generating adaptive alternative thoughts.
Limitations
Despite conducting a structured review and multi-faceted evaluation of CR apps, various limitations should be noted. Our search was restricted to apps available in English and the United States versions of two app stores; exploration of CR apps in other regions, other languages, or other app stores might yield different findings. These apps might be higher quality, or it might be that apps available in other languages or regions are more limited. We also restricted our evaluation to features available in free apps or the free versions of apps with paid offerings, because many consumers and practitioners are likely to prefer apps that are totally free (Schueller et al., Reference Schueller, Neary, O’Loughlin and Adkins2018). However, paid apps are likely to have more in-depth features that we are unable to comment upon based on our review. MH apps change frequently, with regular updates within apps and fluctuation within the marketplace (Lagan et al., Reference Lagan, D’Mello, Vaidyam, Bilden and Torous2021; Larsen et al., Reference Larsen, Nicholas and Christensen2016). As such, given that our results were based on apps and app versions available in January 2021, the landscape of CR apps may have changed even since the time of our review.
Beyond features, we used only the MARS and no other available rating scales or systems, such as the APA model (Lagan et al., Reference Lagan, Aquino, Emerson, Fortuna, Walker and Torous2020) or Enlight (Baumel et al., Reference Baumel, Faber, Mathur, Kane and Muench2017), to rate app quality. The MARS has shown good convergent validity with Enlight (Terhorst et al., Reference Terhorst, Philippi, Sander, Schultchen, Paganini, Bardus and Messner2020). We also did not fully assess inter-rater reliability of the MARS ratings; however, our methods did include strategies to mitigate large differences between the PhD-level reviewers such as discussion and opportunities to adjust scores. Our MARS ratings were based on scores from three raters, including both professionals and those with lived experience with mental health issues, all of whom had training and experience reviewing apps. Obtaining reviews from a larger sample, including more practitioners and end-users, might provide a more generalizable assessment of how practitioners and their clients would view the user experience of these apps. Different consumers may also have different assessments of user experience based on their own characteristics such as preferences or digital literacy. We also did not have any assessment of the effectiveness of these apps, although we could not find any study directly evaluating the benefit of the reviewed CR apps either on their own or within clinical practice. Thus, our findings cannot speak to the presumed clinical benefits of using CR apps as adjunctive tools in traditional face-to-face therapy.
Clinical implications
Given the increasing use of technology in our daily lives, including health care and service delivery, many CBT-oriented practitioners will likely see traditional paper thought records replaced by digital tools to facilitate CR. Like other providers seeking to identify quality apps for a particular purpose from an often-overwhelming abundance of choices, these practitioners face a daunting task. App store descriptions intended to drive use and appeal to the broadest possible audience often contain exaggerated or misleading claims (Larsen et al., Reference Larsen, Huckvale, Nicholas, Torous, Birrell, Li and Reda2019). Indeed, as noted, only a fraction of the apps we identified through a keyword search actually included CR and a keyword search is likely to be an approach many practitioners would adopt to find an app. Highly accessible ‘go-to’ metrics such as average star ratings and total number of reviews have generally been found not to be predictive of an app’s actual features or quality (Lagan et al., Reference Lagan, D’Mello, Vaidyam, Bilden and Torous2021). Independent app-rating and evaluation platforms (e.g. One Mind PsyberGuide, mHealth Index and Navigation Database ‘MIND’) can be invaluable starting points but do not address how faithfully and effectively specific interventions (e.g. CR) are represented in the high volume of apps they review. At the time of this writing, 12 of the 15 apps included in this review are available on One Mind PsyberGuide and nine of the 15 are available on MIND; so while they might be useful resources, they are not exhaustive in their coverage of such products. Moreover, despite being a common component of evidence-based protocols for depression and anxiety, CR is absent from the majority of mental health apps for these conditions (Wasil et al., Reference Wasil, Venturo-Conerly, Shingleton and Weisz2019), and discerning which apps include it (not to mention which components of CR they include or how well they are represented) is difficult without a time-consuming ‘deep-dive’ examination of specific apps. Unfortunately, findings from this review expose the inadequacy of commonly employed cursory search strategies with respect to yielding high-quality apps that align with practitioners’ needs and preferences.
Given the need for practitioners to consider specific apps in some detail in order to make an optimal selection, we hope this review’s multifaceted examination of CR apps proves to be a useful substitute for their having to comprehensively explore these apps one by one. Noteworthy in this regard is the fact that, unlike other reviews that found a surprising amount of uniformity across a large number of mental health apps (e.g. Lagan et al., Reference Lagan, D’Mello, Vaidyam, Bilden and Torous2021; Wasil et al., Reference Wasil, Venturo-Conerly, Shingleton and Weisz2019), the current review found considerable heterogeneity with respect to inclusion of specific CR sub-elements and the number and type of non-CR features. Thus, although these apps might seem quite similar at a high level, at a more granular feature level there are differences that may be important to practitioners. Although it might complicate the app selection process, we regard this variability as potentially advantageous, as it increases the likelihood that an individual practitioner can identify apps that align with their preferred style of conducting CR, their desired additional features, and their clients’ needs and capacities. For example, a practitioner who prefers to have all the core elements of CR represented in the app or whose client particularly struggles with the evaluating outcomes element of the CR process, might select Moodnotes, whereas one who judges their clients as more likely to engage in and persist with a stripped-down approach (e.g. identify a thought contributing to distress and an alternative thought likely to reduce it) might opt for a more streamlined app like Quirk CBT or IntelliCare – Thought Challenger. A practitioner who values adopting an app judged to be visually and functionally engaging by objective raters might favour Sanvello, based on its total and subscale MARS scores. If the ability for users to share data with a therapist (or others) is valued, then apps with such export functionality, like Mindshift CBT and Moodnotes, would merit consideration. Clearly, one size does not fit all with respect to choosing apps to use in therapy and this ability to tailor app selections to better approximate practitioners’ preferred methods of teaching and using CR and to meet the needs of their particular clients might lead to increased use and benefits. On the other hand, some features, like data safeguards, which should be regarded as universally desirable, if not required, are surprisingly rare among the reviewed CR apps. For instance, only Quirk CBT offers both a form of login protection (e.g. password, face ID) and data encryption.
Recommendations
A number of recommendations for both researchers and app developers emerge from the current review. Researchers can augment the current findings by reviewing apps that require subscriptions or in-app purchases to access CR content. Additionally, the field and practitioners seeking to use apps in their practice would benefit greatly from additional or updated reviews focused on how and how well apps incorporate other widely used techniques common to evidence-based practice (e.g. behavioural activation, problem-solving, exposure, relaxation, mindfulness, behavioural experiments). Moreover, echoing a recommendation that is ubiquitous across reviews of mental health apps, there is a critical need for controlled trials focused on evaluating the efficacy of CR apps.
Our review exposed a number of gaps and shortcomings that future mental health app developers could readily address. Given the relative under-representation of CR among apps that purport to feature evidence-based content (Wasil et al., Reference Wasil, Venturo-Conerly, Shingleton and Weisz2019), developers should seek to produce more apps that include high-quality CR components, as either a primary or supplementary tools. In many cases, this could be accomplished by modestly expanding existing journalling content (e.g. prompts to identify situations, thoughts, and/or emotions) to include thoughtfully sequenced additional prompts that would create a CR component (e.g. to generate adaptive alternative thoughts and evaluate their impact). Although there are benefits to maintaining heterogeneity across CR apps with respect to which elements are included, certain elements are sufficiently infrequent (e.g. evaluating the outcomes of the CR process by re-rating the intensity of the distressing emotion; rating the degree to which a target thought is believed before and after the CR process) that developers should be encouraged to include them more often. Given the concerns that practitioners often have around data security and privacy issues with MH apps (Bush et al., Reference Bush, Armstrong and Hoyt2019; Schueller et al., Reference Schueller, Washburn and Price2016), developers should pay far more attention to privacy protections and data security safeguards. HIPAA compliance and data encryption were woefully under-represented among the CR apps included in this review.
Conclusion
Practitioners should be aware that cursory efforts to identify high-quality CR apps are likely to be inadequate as only a small percentage of apps that appear to provide CR functionality based on their app store descriptions actually do. However, the apps identified as containing CR elements through our systematic search tended to include most core CR elements and, on average, provide an acceptable to good user experience. But these CR apps varied considerably with respect to their inclusion of sub-elements of CR, their user experience ratings, and other variables likely to be of importance to practitioners deciding which app to adopt. Clinicians should consider these differences in order to make optimal choices of apps that are well aligned with their style, preferences and clientele. App developers need to invest more effort in producing apps that adequately protect users’ privacy and that are sufficiently engaging to promote regular use and consequent skill acquisition. Ultimately, no one-size-fits-all solution for selecting mental health apps exists and efforts like this that provide more systematic and nuanced guidance may help those who hope to use such tools in their practice.
Key practice points
-
(1) Few of the apps identified through keyword searches related to CBT and cognitive restructuring (CR) actually offer the defining components of CR.
-
(2) Identifying automatic thoughts and generating adaptive alternatives were the most commonly represented features in CR apps.
-
(3) CR apps generally scored in the acceptable to good range on user experience ratings, but still demonstrate considerable differences in their graphics and inclusion of various features.
-
(4) Given the differences among apps, it is critical to pilot an app to determine if it might help support your practice and use of CR.
Data availability statement
The data collected for this review are available at an OSF repository that can be accessed at: https://osf.io/ds7r8/ (doi: 1.0.17605.OSF.IO/DS7R8).
Acknowledgements
None.
Author contributions
Drew Erhardt: Conceptualization (lead), Investigation (equal), Methodology (equal), Writing – original draft (lead), Writing – review & editing (equal); John Bunyi: Data curation (equal), Formal analysis (equal), Investigation (equal), Software (equal); Zoe Dodge-Rice: Data curation (equal), Investigation (equal), Project administration (lead), Writing – review & editing (supporting); Martha Neary: Conceptualization (supporting), Data curation (equal), Methodology (equal), Project administration (supporting), Writing – review & editing (supporting); Stephen Schueller: Conceptualization (equal), Formal analysis (equal), Funding acquisition (lead), Methodology (equal), Project administration (supporting), Resources (lead), Supervision (lead), Writing – original draft (supporting), Writing – review & editing (equal).
Financial support
A portion of this work was supported by funding that Stephen Schueller receives from the mental health non-profit organization One Mind.
Conflicts of interest
Drew Erhardt co-developed one of the apps included in the present review (viz., moodnotes) but relinquished any ownership of or financial interest in it prior to participating in the research associated with this submission. Stephen Schueller also co-developed one of the apps included in the present review (viz., IntelliCare – Thought Challenger) which has since been licensed by Adaptive Health, but he does not have any ownership or financial interest in Adaptive Health. Stephen Schueller has received consulting payments from Otsuka Pharmaceuticals for work unrelated to this manuscript and compensation for his service on the Scientific Advisory Board for Headspace. He also receives research funding from One Mind for the operation and management of One Mind PsyberGuide.
Ethical standards
The authors have abided by the Ethical Principles of Psychologists and Code of Conduct as set out by the BABCP and BPS. Approval to conduct this non-human subject research project was granted by Pepperdine University’s Graduate & Professional Schools Institutional Review Board (reference no. 52621).
Comments
No Comments have been published for this article.