INTRODUCTION
Syndromic surveillance is the collection, analysis, interpretation and dissemination of health-related data in near real time [1]. Public Health England (PHE) coordinates a programme of syndromic surveillance which routinely monitors for the emergence and spread of common infectious diseases (e.g. influenza and norovirus), and the public health impact of non-infectious events (e.g. fires and floods), in the community [2]. These systems utilize data from a range of different sources including remote health advice services (telephone/internet based), general practitioner networks, and a network of sentinel emergency departments (EDs).
The increased morbidity, mortality and subsequent burden on healthcare services caused by respiratory infections make comprehensive surveillance of respiratory infections an important part of any national public health programme, both for the annually expected rise in activity during winter, and any out-of-season outbreaks (including influenza pandemics). Traditional monitoring of community-based respiratory disease relies on sentinel primary-care-based systems; swabbing of influenza-like illness (ILI) cases, with laboratory confirmation and reporting, and physician notifications [Reference Brammer3–Reference Zambon6]. Syndromic surveillance has become an additional tool in the surveillance of respiratory illness, providing valuable intelligence on the levels and any trends (both increasing and decreasing levels) of respiratory disease, particularly those in the community requiring self-care, rather than medical intervention or treatment. Although individuals do not have a laboratory-confirmed diagnosis, syndromic surveillance of respiratory illness has been shown to be closely linked to levels of laboratory confirmations of influenza and respiratory syncytial virus (RSV) in particular [Reference van den Wijngaard7–Reference Cooper10].
The Emergency Department Syndromic Surveillance System (EDSSS) is the most recent addition to the PHE syndromic surveillance service, providing insights at the secondary-care level. This is the first system of its kind in the UK, securely extracting anonymized daily data from a sentinel network of EDs across England and Northern Ireland [Reference Elliot11]. The EDSSS automation requires no input from ED staff, while making existing data from individual EDs available for use as a single source of ‘big data’ for near real-time public health surveillance purposes. The EDSSS was developed in 2010 to support preparations for, and to meet enhanced surveillance needs during the London 2012 Olympic and Paralympic Games and continues as a long-term national public health legacy [Reference Elliot11–Reference Elliot13].
Within the UK, previous syndromic surveillance studies have attributed the underlying respiratory pathogens that contribute to the winter pressures exerted on healthcare systems through statistical estimation [Reference Cooper9, Reference Cooper10]. The aim of this investigation was to understand the factors that might be driving respiratory attendances at EDs, in terms of the potential causal organisms and the temporal trends. We also aimed to describe any potential for ED syndromic surveillance to provide early warning of public health problems and winter pressures by identifying and reporting on increased seasonal activity of specific syndromic indicators related to respiratory pathogens, ahead of traditional laboratory surveillance.
METHODS
EDSSS
For each patient attending an EDSSS-participating department, an anonymized record is received detailing simple (non-identifiable) demographic information, including patient's sex and age, in addition to a clinical diagnosis code [Reference Elliot11]. The information reported to EDSSS uses a standardized coding system [14]; however, the clinical diagnosis information remains in the format used within each individual ED. Different diagnostic coding systems reveal different levels of clinical detail and thus different complexities of EDSSS syndromic indicators are used [Reference Elliot11]. For the purposes of this investigation, a generic ‘all respiratory disease’ (ARD) attendances indicator captured data from all participating EDs, while more specific ‘acute respiratory infections’ (ARI), ‘acute bronchitis/ bronchiolitis’, ‘influenza-like illness’ and ‘pneumonia’ indicators were reported from those EDs using sufficiently detailed diagnostic coding systems (full details of the diagnosis codes included in each syndromic indicator, using both ICD-10 [15] and SNOMED CT [16] coding systems are available upon request).
EDSSS reporting
Daily reporting to EDSSS began at the first two EDs during July 2010. As the number of participating departments has increased, so, inevitably, has the number of attendances recorded, making it necessary for this investigation to be separated into annual periods. Absolute numbers were used for all analyses as the overall attendances (denominator for any percentage calculation) can be affected by weather, national holidays and school holiday periods which were not considered in this investigation. Only those EDs which had reported during the full winter period for each year were included. The winter period was based around the normal increase in respiratory illness peak during weeks 40 to 19 of 2011–2012 (3 October 2011 to 13 May 2012; three English EDs) and 2012–2013 (1 October 2012 to 12 May 2013; 24 English EDs).
Laboratory data
Anonymized laboratory reports for a number of respiratory organisms (Table 1) were obtained from LabBase [17]. Each laboratory report included the specimen date, patient's age, organism identified and specimen type. Analyses were restricted to respiratory specimens (Table 1) to exclude any instances of invasive disease. Following initial examination of the full range of respiratory pathogens, additional laboratory data for RSV were obtained from the Respiratory DataMart System, which collects laboratory data on selected respiratory viruses on a daily basis [Reference Zhao18].
Table 1. Laboratory-confirmed organisms and specimen types included in this study

Statistical analysis
All analyses were performed on weekly data (Monday–Sunday), based on date of sample/ED attendance. This was to ensure compatibility between the daily ED and weekly laboratory datasets, as ED respiratory attendances peak over the weekend, when very few samples are taken for laboratory testing.
Multiple linear regression models were constructed to estimate the proportion of attendances recorded for each of the ED syndromic indicators (all ages and by each age group individually) which were attributable to the respiratory pathogens, using the laboratory data as an indicator of the circulating pathogens. Each model was restricted to the same age groupings for each data source (i.e. ED attendances within a single age group, laboratory detections from the same age group). Using backward stepwise regression all pathogens were initially included in the model; those not statistically significant (P > 0·05) were removed, as were those with negative coefficients, resulting in a final model including only those pathogens with a positive, statistically significant coefficient.
The relationship between the trend in ED attendances and laboratory detections was investigated using regression analysis based on the dependent variable of the change in the number of weekly attendances for each ED indicator (and each ED indicator by age group), against the change in number of detections per week (by date of sample) for each organism separately, to lessen the autocorrelation effects in the time-series data. The statistical models were re-run for selected age groups and the all-ages total group against the RSV laboratory data with lags of ±2 weeks built in to the laboratory results.
All analyses were performed using Stata v. 12.1 [19].
RESULTS
ED attendances: descriptive analysis
For each ED respiratory syndromic indicator the pattern of attendances showed an increase during the winter period in both years studied (Fig. 1). During 2011–2012 there were two peaks in both the ARD and ARI attendances. The main peak occurred during weeks 48/49 of 2011, which was consistent with the peak also seen in acute bronchitis/bronchiolitis attendances. The second, smaller peak at weeks 7/8 of 2012 was in line with the peaks for pneumonia and ILI attendances (Fig. 1). A single winter peak was more apparent for ED respiratory attendances during 2012–2013 (Fig. 1). Similar to 2011–2012, the peak in acute bronchitis/bronchiolitis attendances occurred before those observed for ILI and pneumonia.

Fig. 1. Weekly Emergency Department Syndromic Surveillance System attendances, by respiratory syndromic indicator, 2011–2012 [three emergency departments (EDs)] and 2012–2013 [total and all respiratory disease (ARD): 24 EDs, more detailed indicators: 13 EDs]. ARI, Acute respiratory infection; ILI, influenza-like illness.
The largest number of total ED attendances was for the 15–44 years age group; however, this age group was not the largest group attending for ARD. This was observed in the 1–4 years age group (Fig. 2).

Fig. 2. Total weekly Emergency Department Syndromic Surveillance System attendances and by syndromic indicator, by age group, winter 2011–2012 [three emergency departments (EDs)] and 2012–2013 [total and all respiratory disease (ARD): 24 EDs, more detailed indicators: 13 EDs]. ARI, Acute respiratory infection; ILI, influenza-like illness.
In addition to different overall levels of attendances in each age group, distinct and separate peaks were observed for the ARD and ARI indicators in particular. ARD and ARI attendances in the 1–4 years age group showed both the highest and earliest peak in attendances, several weeks before the levels increased in other age groups. Bronchitis/bronchiolitis also showed a high, and early, peak in attendances in this age group; however, the number of bronchitis/bronchiolitis attendances was highest for those aged <1 year, despite this group accounting for the lowest number of ED attendances overall (Fig. 2).
ARD and ARI attendances in the 5–14 years age group peaked after those aged ≤4 years but ahead of the older age groups each year. By contrast, pneumonia attendances were most commonly recorded in those aged ⩾75 years, several weeks after peaks seen for other respiratory attendances (Fig. 2).
During both years ILI attendances were very low, up to eight per week during 2011–2012 and 26 per week in 2012–2013 (Fig. 1). ILI attendances were the only syndromic indicator found to be most common in the 15–44 years age group (Fig. 2).
Comparison with laboratory data
Over 130 000 LabBase detections (62 951 during 2011–2012 and 67 438 during 2012–2013) were eligible for inclusion. The most common respiratory organisms showed a seasonal trend, with influenza A, influenza B and RSV showing marked increases during the winter periods (Fig. 3).

Fig. 3. Laboratory detections of respiratory pathogens, from respiratory specimens, by week of specimen collection, week 40 to week 19, 2011–2012 and 2012–2013. RSV, Respiratory syncytial virus; HMPV, human metapneumovirus.
RSV was the first respiratory pathogen to display a rise and subsequent peak in activity during both years. Unlike seasonal influenza, which can peak at any time during the winter, the rise in RSV activity is predictable each year in the UK [Reference Goddard20], usually occurring before influenza activity rises. The RSV peak was observed before the influenza peak in both years; however, the RSV peak during 2012–2013 (2012, week 48) occurred 4 weeks before that observed during 2011–2012 (2011, week 52).
Few influenza B detections were reported during 2011–2012; however, 2012–2013 was initially dominated by influenza B, which peaked during week 1 in 2013. Influenza A activity peaked at similar levels each year (200–250 detections per week), which was considered to be relatively low-level seasonal activity. The highest levels during 2011–2012 (2012, weeks 7–9) were earlier than during 2012–2013 (2013, week 12), when influenza A activity peaked after that for influenza B.
Model results
The final multiple linear regression models constructed for the respiratory and ARI indicators, for all ages, included RSV for each year studied and influenza B during 2012–2013 (Table 2). The 2012–2013 dataset included a larger number of ED attendances, resulting in better fit for most of the models reported. During 2012–2013 an estimated 25% of the variation in ARD attendances was found to be attributable to respiratory pathogens. For those attendances with a diagnosis of acute bronchitis/bronchiolitis a higher percentage (around 40%) was attributable to respiratory pathogens (Table 2). Statistical analyses were not undertaken using the ILI attendance data, due to the low numbers involved.
Table 2. Multiple linear regression model results for respiratory, acute respiratory infection, acute bronchitis/bronchiolitis and pneumonia syndromic indicators, by age group, against laboratory reports within the same age group, by organism
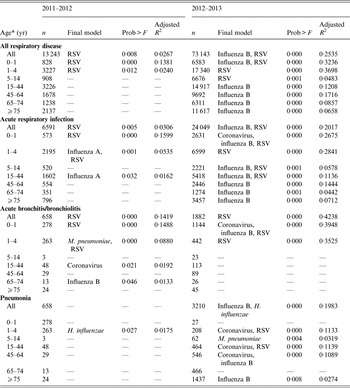
RSV, Respiratory syncytial virus.
* ‘All’ is the total attendances including those reported with no age information.
– No organisms with a positive, statistically significant, coefficient remaining.
The pathogens remaining in the final model often varied according to age group. The association with RSV in the youngest age groups remained significant in each model, for ARD, ARI and bronchitis/bronchiolitis attendances.
ED attendances for pneumonia were found here to have a statistical association with laboratory detections of seasonal coronavirus across the whole winter period, although there was no evidence of association in the temporal trends. The numbers of both ED pneumonia attendances and coronavirus detections were, however, much lower than those for the other indicators and organisms studied. Influenza A was notably absent from all of the final models during 2012–2013 and only found to have a statistical association with ARI in two age groups (1–4 and 15–44 years) during 2011–2012.
Regression analysis was carried out on the weekly change in number of attendances for each of the ED variables against the number of laboratory detections of influenza A, influenza B and RSV. As was expected following the results of the multiple regression models (Table 2) there were clear differences in the age-specific attendances and how these were associated with the laboratory data (Table 3).
Table 3. Regression model results for each syndromic indicator, by age group, against laboratory reports of influenza A, influenza B and respiratory syncytial virus

Bold values are statistically significant; —, no result.
The initial regression models for 2012–2013 indicated an association between several ED indicators and influenza B laboratory detections (Table 2). These were also found to be statistically significantly associated with ARD and ARI attendances for those aged <1 year, 45–64 years and the all-ages in total (Table 2). There was a notable absence of any statistically significant association with influenza A.
Respiratory, ARI and bronchitis/bronchiolitis attendances in those aged ≤4 years showed an increase in attendances in line with the increase seen in laboratory detections of RSV. These associations were found to be statistically significant in almost all cases with two exceptions, the indicators with the fewest attendances (ARI and bronchitis/bronchiolitis attendances, <1 year, 2011–2012; Table 3).
Although the statistical models showed an association, the time series of attendances for those aged 1–4 years in particular appeared to precede the peak in laboratory RSV detections (by specimen date) by several weeks during both years (Figs 2 and 3). The statistical models were re-run for the youngest age groups and the all-ages total group against the RSV laboratory data with lags of ±2 weeks built into the laboratory results. These results showed an improved fit with the RSV –1 week model, implying that the ED attendances increased 1 week before the rise in RSV laboratory confirmations (data not shown). This investigation was repeated using data from the Respiratory DataMart system, with similar results (Table 4). During 2011–2012 the EDSSS showed improved fit with the RSV –1 week model for almost all indicators and age groups tested. While the –1 week and 0 week models both showed good fit for 2012–2013.
Table 4. Regression model results of selected age groups and syndromic indicators against respiratory syncytial virus positive results in the DataMart system ±2 weeks

Bold values are statistically significant.
DISCUSSION
Main findings
Routine ED respiratory attendance data demonstrated clear winter peaks across a range of syndromic respiratory indicators and a close association with the incidence of selected laboratory pathogens. Interestingly, the most valuable information derived from these respiratory indicators was from age-specific data: here, we demonstrate that RSV, which is known to affect young children in particular [Reference Fleming and Cross21, Reference Hall22], is an important factor driving the levels of ED respiratory attendances in children aged ≤4 years. ARI and bronchitis/bronchiolitis attendances in the 1–4 years age group are a particularly sensitive indicator for increasing RSV activity, which may account for over 30% of the variation of these attendances. The ARD and ARI attendances in this age group may give up to 1 week early warning of an increase in laboratory detections of RSV (based on date of specimen collection). There is a potentially even earlier warning compared to the issue and eventual receipt of final laboratory results.
Although ARD and ARI attendances in the 5–14 years age group were observed to peak ahead of the older age groups (and after those aged ⩽4 years), this was not shown to have a statistically significant temporal link with any one organism.
Strengths and weakness of this study
This work illustrates the importance of analysing age-specific ED data. Our age-specific analysis revealed temporal differences which can be attributed to specific pathogens. One limitation of using ED attendance data is the diagnosis coding of attendances: due to the nature of emergency medicine, detailed coding can be limited by the high acuity of the attendance and the urgency to admit patients. Therefore, the use of specific diagnostic codes, e.g. ‘influenza’ in the ED is far less frequent than more generic codes, e.g. ‘respiratory disease’. There are also large differences in the UK with regards to the quality and variability of coding within individual EDs, although initiatives to drive standardization of coding fields and diagnostic codes are improving the national situation [14].
This study was not able to find a positive association between influenza A activity and ED attendances. The low recording of ILI attendances may have been due to a combination of the low number of EDs reporting (especially during 2011–2012), the relatively low influenza activity as reported from laboratory surveillance or possibly the limited availability of diagnosis codes specific to ‘influenza’ within individual EDs. It is possible that the use of EDSSS, as it stands, is not sensitive to ILI in the adult age groups during low-intensity influenza A seasons (as occurred in the years of this work), whereas community activity of RSV and influenza B, which are particularly associated with children and infants, can be detected by EDSSS.
As a near real-time system, the EDSSS has an advantage over laboratory-based surveillance in providing information for action in the timeliest manner possible. Our results demonstrate that monitoring respiratory ED attendances in real time can provide early warning of increasing activity of pathogens such as RSV and influenza B, which can be triangulated with other respiratory surveillance systems including laboratory surveillance to understand the underlying aetiology of the increase. The findings here that ED attendances may precede an increase in RSV laboratory confirmations may be due to the 24 h a day, 7 days a week availability of emergency care through EDs. Although virological results for surveillance purposes are now available on a daily basis using DataMart, the numbers attending EDs for respiratory conditions is usually at the highest levels during the weekend, when fewer specimens are collected, so ED attendances would be recorded and recognized through syndromic surveillance systems before there has been opportunity for a specimen to be taken and even further in advance of a result.
As the EDSSS continues to grow, so the number of hospitals, total attendances and geographical coverage increases. The first year examined here only included data from three English EDs, compared to national laboratory data, although it still showed comparable results to the 24 English EDs included in 2012–2013 in terms of the youngest age groups and RSV.
How does this study compare to others work?
A key feature of syndromic surveillance is the ability to provide early warning of infectious disease outbreaks or other public health incidents [Reference van den Wijngaard7, Reference Cooper10, Reference Heffernan23]. The results presented here show that increased ED attendances may occur at least 1 week in advance of the increase seen by laboratory testing, by date of sample collection, for RSV in particular. The comparison of syndromic surveillance of respiratory illness through NHS Direct telephone calls and the numbers of laboratory-confirmed respiratory pathogens in England has been shown to provide an early warning of seasonal increases in influenza, up to 2 weeks before laboratory surveillance in some seasons [Reference Cooper10]. The seasonal variation in the numbers of NHS Direct calls recorded as due to respiratory illness has also been previously estimated to be mainly due to influenza and RSV [Reference Cooper9].
There is less evidence presented here to support previous studies that have shown ED syndromic surveillance of respiratory attendances to be associated with community influenza activity [Reference Olson8, Reference Heffernan23], which may be due to the relatively low intensity influenza seasons experienced during the winters studied. Although influenza B often remained in the final models for 2012–2013 during the preliminary multiple regression, a temporal relationship was not identified. The apparent lack of early warning of, or even association with, influenza A provided by monitoring English ED attendance data may not be unexpected. The EDSSS aims to capture more severe presentation of illness than other sources of syndromic surveillance, e.g. telephone helplines; early presentation of community-based mild self-limiting disease will therefore not be captured by English ED data. Although influenza A results in a more severe disease presentation than influenza B, it is also more generally associated with older age groups. It is, however, the youngest age groups, those aged ≤4 years in particular, which present to EDs with respiratory conditions in the highest numbers. Adults with suspected ILI in England are actively encouraged to stay at home and self-treat, which may account in part for the low numbers of ILI attendances reported, as well as the lack of statistical association with influenza A reported here.
Our study is unique in that we investigated and showed the benefit of using a range of ED respiratory syndromic indicators, by age group (rather than a single all-encompassing grouping) compared to laboratory surveillance. Our previous work illustrated the value of using age-specific indicators for influenza surveillance from a telephone helpline syndromic surveillance system [Reference Cooper9, Reference Cooper10].
Implications for clinicians or policy makers
The findings of this study are potentially of use to emergency medicine clinicians, as the indicators developed suggest that changes in attendance patterns for a particular respiratory syndromic indicator, in a specific age group, may provide warning of increased community activity of particular respiratory pathogens. The evidence presented here, using a retrospective analysis, shows that the rise and subsequent peak in ED respiratory attendances in young children may occur up to 1 week prior to RSV detections by specimen collection date (there is a subsequent time delay for microbiological analyses and results, which is likely to differ between pathogens). EDSSS data are collected and monitored on a daily basis. Changes in trends can therefore be identified and potentially actioned, an estimated minimum of 1 week before increases in specimen collection and subsequent laboratory confirmations. This period of early warning may aid in the instigation of any preparations required to respond to RSV effectively in terms of the availability of paediatric resources such as equipment, drugs and bed availability.
There is also the additional possibility of using ED syndromic surveillance to predict the occurrence of the winter peak in pneumonia attendances in those aged ⩾75 years. This peak itself was not seen to occur at the same time as activity of any one pathogen, although it may follow several weeks after other peaks in respiratory attendances. ED attendances for pneumonia in the elderly will have greater resource implications for hospitals as a greater proportion of these attendances will result in admissions requiring longer spells of care, thereby reducing numbers of available beds resulting in ‘winter pressures’. The EDSSS data may, in future, therefore be able to provide advanced warning and thus aid in planning within hospitals. Although the EDSSS cannot directly reduce attendances, it may aid the timing of the implementation of existing plans to deal with increases in elderly admissions which can cause huge pressures in secondary care [Reference Elliot, Cross and Fleming24]. Information from EDSSS may also aid in the identification of any particular sources of pressure, such as types of attendances or specific age groups, both retrospectively to aid the development of plans, and prospectively to respond to acute changes in real time.
The EDSSS is now established and has been monitoring data over several years and winter periods, establishing expected levels of attendances for each indicator. The ability of EDSSS to identify changes in ED presentations, in terms of overall numbers, types of presentations or age groups and the severity of presentation, make it a valuable tool in the preparation for identification and monitoring of any emerging novel pathogens in near real time.
Future work
Most ED attendances used in this investigation were those associated with the two most broad indicators investigated here (all respiratory disease and acute respiratory infections). However, as the number of departments reporting to EDSSS continues to increase as the system continues to improve coverage across England in particular, so the numbers of attendances associated with the more specific indicators, e.g. bronchitis/bronchiolitis, ILI and pneumonia will also increase. The potential for further investigation of these indicators alongside the results of laboratory surveillance will therefore improve.
Due to the nature of emergency medicine, microbiological investigation and final confirmation is unlikely while the patient is still in the ED, due to the time scales involved with testing. Therefore, it is rare for there to be laboratory confirmation of the causative organism for respiratory ED attendances. Future research work within the EDSSS could employ a series of near-patient tests or self-sampling coupled with laboratory confirmation on a selected number of patients attending with respiratory illness. Results would be able to provide some indication of sensitivity and specificity of coding, provide validation of the syndromic indicators and potentially valuable near real-time clinical information for improving patient care.
This paper confirms the potential of the EDSSS to monitor, and provide early warning of seasonal respiratory pathogens, revealing that the EDSSS is particularly valuable in monitoring RSV activity. Further work will involve investigating the potential for RSV surveillance, including the development of early warning thresholds to indicate increasing activity, and intensity thresholds to indicate levels of seasonal activity to provide context for determining the potential impact on healthcare services, similar to those that have been used in other surveillance systems such as in general practice [Reference Fleming4, Reference Goddard, Kyncl and Watson25]. These may include the use of age-specific EDSSS indicators sensitive to RSV and potentially influenza, which will be further developed for use within the national PHE syndromic surveillance service, for both seasonal and new, emerging pathogens such as pandemic influenza.
ACKNOWLEDGEMENTS
We acknowledge the contribution and support from the ED clinicians and Trust staff; the ongoing support of the Royal College of Emergency Medicine; the technical support provided by Ascribe Ltd and L2S2 Ltd in developing the EDSSS system and all laboratories providing data to LabBase and DataMart. We also thank the members of the PHE Real-time Syndromic Surveillance Team for excellent scientific support in maintaining the EDSSS.
This research received no specific grant from any funding agency, commercial or not-for-profit sectors. This surveillance is undertaken as part of the national surveillance function of Public Health England.
DECLARATION OF INTEREST
None.