Ethnic inequalities in a wide range of chronic diseases have been documented( Reference Becker, Boreham and Chaudhury 1 ), with risk factors evident early in life( Reference Donin, Nightingale and Owen 2 ). Children and youth from ethnic minority groups are more likely to be overweight or obese than White European populations( Reference Ogden, Flegal and Carroll 3 , Reference Nelson, Gortmaker and Subramanian 4 ). The cardiometabolic risks associated with obesity are well known; therefore, it is not surprising that some ethnic minority populations suffer disproportionately high rates of chronic diseases such as type 2 diabetes (T2D), hypertension and CHD( Reference Becker, Boreham and Chaudhury 1 , Reference Zhang, Wang and Huang 5 ).
Ethnic inequalities in obesity and chronic disease risk may be explained by differences in diet and lifestyle. Differences in the nutritional composition of the diet are apparent; a recent cohort study of children in the UK reported significantly higher energy, fat and sugar intake among South Asians, while Black Africans demonstrated lower intake of fat and saturated fat but higher intake of carbohydrate, compared with White British( Reference Donin, Nightingale and Owen 6 ). Unfavourable dietary habits, such as regularly skipping breakfast and consumption of sugar-sweetened fizzy drinks and fast foods, are also more common among children and adolescents from some ethnic minority groups( Reference Harding, Teyhan and Maynard 7 – Reference Donin, Nightingale and Owen 10 ). The detrimental impact of skipping breakfast and consumption of fast food and fizzy drink on childhood and adolescent health has been documented through a number of cohort studies showing that those who regularly skip breakfast have an increased risk of obesity and detrimental cardiometabolic risk profiles( Reference Donin, Nightingale and Owen 10 – Reference Berkey, Rockett and Gillman 12 ). In addition, studies have shown that children who skip breakfast regularly have poorer-quality diets, particularly higher energy density, saturated fat and lower micronutrient intake( Reference Szajewska and Ruszczynski 13 ). More recently longitudinal studies have investigated the prevalence of these behaviours in young adulthood and the detrimental impact of childhood behaviours on adult health( Reference Smith, Gall and McNaughton 11 , Reference Merten, Williams and Shriver 14 , Reference Watts, Mason and Loth 15 ). Young adulthood is recognised as an important transition period, with increasing responsibilities and the development of independent health behaviours. The Australian Childhood Determinants of Adult Health (CDAH) study found skipping breakfast in both childhood and young adulthood to be associated with larger waist circumferences, higher fasting insulin and higher total cholesterol and LDL-cholesterol concentrations( Reference Smith, Gall and McNaughton 11 ), while in the US-based project EAT (Eating and Activity in Teens) socio-economic status was found to be a principal determinant of increases in breakfast skipping between childhood and adulthood( Reference Watts, Mason and Loth 15 ). To date, no longitudinal cohort studies have described ethnic patterns in adolescent and young adult nutritional intake and dietary behaviours and determined their longitudinal effects on body weight and cardiometabolic risk factors. The ‘DASH 10 years on’ study is the first multi-ethnic cohort of young adults in the UK and provides longitudinal follow-up data for a subset of the Determinants of Adolescent Social well-being and Health (DASH) cohort, who are now young adults (21–23 years). We aimed to describe longitudinal patterns of dietary intake in the multi-ethnic Determinants of Adolescent, now young Adults, Social well-being and Health cohort, and investigate the impact of dietary intake on cardiometabolic risk in young adulthood.
Methods
Study design
Details of the DASH study can be found at http://dash.sphsu.mrc.ac.uk/ and in a published cohort profile( Reference Harding, Whitrow and Maynard 16 ). The original cohort of 6643 students, aged 11–13 years, was recruited between 2002 and 2003, from fifty-one secondary schools in ten London boroughs and sampled to represent the main ethnic groups of the UK population. A pilot follow-up study of 665 participants was completed in March 2014; dietary data were collected from 627 participants who took part in the follow-up.
The study was approved by the National Health Service South East Scotland Research Ethics Committee (Ref:11/SS/0051). Written informed consent was obtained from participants. The study is registered on clinicaltrials.gov ID: NCT03283332.
Participants
The subsample consisted of 107 White British, 102 Black Caribbean, 132 Black African, 98 Indian, 111 Bangladeshi or Pakistani and 115 other (mainly mixed) ethnicities and was purposively sampled to be representative of sex and socio-economic circumstances (SEC) across the ten London boroughs and fifty-one schools. Ethnicity was self-declared, measured by self-report using census categories, checked against reported parental ethnicity and grandparents’ country of birth. Bangladeshis and Pakistanis were approximately equally represented and combined because of the relatively small sample sizes; participants declaring their ethnicity as White European, White Irish, Chinese, mixed ethnicity were classified as ‘other ethnicity’.
Procedures
Measurement protocols and questionnaires can be found at http://dash.sphsu.mrc.ac.uk/. Nurses and research assistants were trained in the assessment methods by the research team for 1 week before the start of fieldwork and were re-certified at 6 monthly intervals. All equipment was checked and calibrated regularly by the field supervisors. Participants were given a choice of locations (local general practitioner’s surgeries, local community pharmacies, Clinical Research Centres and Kings College London) and assessments took about 2 h.
Dietary behaviours and intake
At age 11–13 and 21–23 years, participants completed a short questionnaire regarding their consumption of breakfast, fruits and vegetables. For breakfast consumption, participants were given a choice of four responses (every day, 3–4 d a week, 1–2 d a week and never/hardly ever). Fruit and vegetable consumption were separate questions with a choice of seven responses for each (five or more portions a day, four portions a day, three portions a day, two portions a day, one portion a day, I eat fruit/vegetables some days but not every day and I never eat fruit/vegetables). Examples of portions (e.g. handful of grapes, an apple, serving spoon of vegetables, bowl of salad) were given alongside the questions for more accurate estimation of portion size. In addition, at age 11–13 years, participants answered an additional question regarding consumption of fizzy drinks, for which a choice of five answers were available (every day, most days, weekly, less than weekly and never).
At 21–23 years, dietary intake was assessed using the standardised triple-pass 24 h recall methodology( Reference Nelson, Holmes and Roberts 17 ). The study investigator, trained in dietary assessment methods, interviewed the participant face-to-face to recall their food and beverage intake for the preceding 24 h, extracting details regarding food types, brands, recipes and cooking methods. Detailed information was collected for pre-packaged foods including brands and portion sizes, and full recipes and cooking methods were recorded for home-prepared foods and dishes. Portion sizes were confirmed using a photographic food atlas( Reference Nelson and Meyer 18 ) and household measures.
Dietary intake records were coded for analysis by a single coder (C. B.) and analysed for food and nutrient composition using WISP software, which is based on McCance and Widdowson’s The Composition of Foods, 6th edition. Where food items were not available within the WISP database, new entries were created, particularly for home-prepared recipes. Nutritional composition information was sought from food labels and manufacturer websites for branded items, and information for cultural foods were sought from our own database of African and Caribbean dishes( Reference Goff, Timbers and Style 19 ), the West African Food Table( Reference Stadlmayr, Charrondiere and Bayli 20 ) and recipe books. Food weights were assigned from the photo atlases that were used in the interviews. Where food weights were not indicated, average adult portion sizes from the National Diet and Nutrition Survey were used( 21 ).
The quality of reporting in the 24 h recall records was assessed using a scoring system developed by the researchers. Seven components were identified as important to assess the quality of dietary information (portion sizes, brand information, food description, cooking methods, recipe information, beverage information, intake timings), and these were scored on a scale of 0–2, in which 0 indicated no information provided, 1 indicated incomplete information provided and 2 indicated complete information provided. The scores assigned to each category were totalled and a percentage calculated, indicating the quality of dietary information obtained. For dietary recalls which did not include categories such as composite or home-made dishes, these categories were excluded when calculating the diet quality percentage. To test the sensitivity of the protocol to assess the quality of the dietary information obtained, two researchers trialled a sample of recalls deemed of poor (n 10) and high quality (n 10). Researchers compared quality scores to remain consistent. Overall, the mean quality score of the dietary records was 69 %, ranging from 21 to 100 %, with 85 % of the recalls scoring ≥50 % for the quality of the data recording. In preliminary analysis, we investigated the impact of reporting quality on the dietary data to determine whether recalls of poorer quality should be excluded from our final analyses; we found no statistical difference in reported energy intake when records scoring <50 % were excluded; therefore, the final analyses included data from the whole cohort.
Macronutrient intake was expressed as percentage of total energy intake. Dietary fibre (Association of Official Agricultural Chemists (AOAC) method) was expressed in g/4184 kJ and Na intake in mg/4184 kJ. Energy intake was compared with estimated energy requirements to assess potential under-reporting; age- and sex-specific standard equations were used to estimate BMR( Reference Henry 22 ). These were multiplied by a lower cut-off physical activity level of 1·2 to provide estimates of minimum total energy expenditure and recognise probable under-reporting( Reference Rennie, Coward and Jebb 23 ); 36 % of participants reported an energy intake below this cut-off and were considered probable under-reporters (rates did not vary by sex, ethnicity or educational attainment (data not shown)). Data from these participants were not excluded from further analyses.
Body size, biological and social measures
Height was measured without shoes, using portable stadiometers, to the nearest 0·1 cm. Weight was measured in light clothing using Salter electronic scales, to the nearest 0·1 kg. BMI was derived as weight (kg)/height (m)2; BMI >25 kg/m2 was defined as overweight/obese. Waist circumference (cm) was measured midway between the 10th rib and the top of the iliac crest, and 0·5 cm subtracted to correct for measurement over T-shirt or vest. The mean of two duplicate measures was derived, with waist:height ratio calculated. Systolic blood pressure was measured using validated OMRON M5-1 instruments and appropriately sized cuffs, after sitting quietly for a timed 5 min, with >1 min between three subsequent readings. The mean of the second and third readings was used in analysis. A 25 ml blood sample was taken by venepuncture for the assessment of total and HDL-cholesterol and glycated Hb (HbA1c); lipids were analysed using colour photometric assays on an automated clinical chemistry analyser (Siemens Advia 2400 analyser), HbA1c was analysed using HPLC (Premier 9210 analyser). Physical activity was measured using ActivPal™ monitors (PAL Technologies). The monitor, validated for identifying sitting, standing and walking( Reference Dowd, Harrington and Donnelly 24 ), was worn continuously on the front of the thigh for 5 d; the number of minutes spent sitting or in sedentary activity per d was derived and used as the measure of physical inactivity.
A self-complete questionnaire measured current smoking behaviour and SEC (i.e. educational attainment and employment status).
Statistical analysis
The dietary behaviours (skipping breakfast, fizzy drink, fruit and vegetable consumption) were recoded into dichotomous variables as follows: skipping breakfast was defined as not consuming breakfast every day; fizzy drink consumption was defined as consuming fizzy drinks every day or most days; low daily fruit consumption was defined as consuming <2 portions of fruit/d and low daily vegetable consumption was defined as consuming <3 portions of vegetables/d. Missing data in each categorical variable were recoded as ‘not stated’. Missing data among continuous variables were 0·001 % for sex, 0·15 % for BMI, 4·5 % for waist:height ratio, 25·0 % for total cholesterol, 27·2 % for HDL-cholesterol, 27·7 % for HbA1c, 0·15 % for systolic blood pressure and 5·6 % for all six nutritional composition variables.
Continuous variables were tested for normality using the Shapiro–Wilk test, and no transformation was necessary. Ethnic differences in anthropometry, cardiometabolic risks factors and dietary intake were performed using ANOVA with post hoc analysis. Longitudinal patterns in dietary behaviours (skipping breakfast, fruit and vegetable intake) from 11–13 to 21–23 years were examined with mixed-effects logistic regression, adjusted for age, sex and ethnicity. Multivariate linear regression was used to examine the association between dietary behaviours at 11–13 and 21–23 years and cardiometabolic risk factors at 21–23 years, adjusted for sex and ethnicity at 11–13 years, and additionally adjusted for educational attainment (attainment/non-attainment of higher education degree), physical activity (based on tertiles of sitting/sedentary time), smoking (current smoker/non-smoker), energy intake and saturated fat intake at 21–23 years. n 664, 635, 499, 484, 481 and 664, respectively, for linear regression models with BMI, waist:height ratio, total cholesterol, HDL-cholesterol, HbA1c and systolic blood pressure, as the outcome. Multivariate linear regression was used to examine the association between dietary behaviours at 11–13 and 21–23 years and nutritional composition of the diet at 21–23 years, adjusted for sex and ethnicity at 11–13 years, and additionally adjusted for educational attainment (attainment/non-attainment of higher education degree), physical activity (based on tertiles of sitting/sedentary time) and smoking (current smoker/non-smoker) at 21–23 years. n 628 for linear regression models for all six nutritional composition variables as the outcome. All analyses were performed using STATA (version 13; StataCorp LP), and statistical significance was considered at P<0·05.
Results
The cohort consisted of 329 men and 336 women. The representation by ethnicity was 107 White British, 102 Black Caribbean, 132 Black African, 98 Indian, 111 Bangladeshi or Pakistani and 115 other (mainly mixed ethnicity). The mean age of the cohort was 22·7 years, mean BMI 24·7 kg/m2, 39 % were overweight or obese (BMI >25 kg/m2), 24 % were smokers and 47 % were educated to degree level. Rates of smoking were highest among White British (47 %) and lowest among Black African (11 %) participants. Attainment of a university degree was highest among Black African (58 %) and lowest among Black Caribbean (34 %) participants.
Dietary data, collected for 627 participants, are shown in Table 1 alongside anthropometry and cardiometabolic risk factors for the whole cohort and for each ethnic group at 21–23 years. At 21–23 years, overall, 56 % of participants reported skipping breakfast on a regular basis, 57 % reported consuming <2 portions of fruit a day and 63 % reported consuming <3 portions of vegetables a day. Ethnic differences in diet, anthropometry and cardiometabolic risk were evident. Black Caribbean and Black African participants were significantly heavier than the Indian and Pakistani/Bangladeshi participants and had higher BMI compared with White British and Indian participants, but there were no differences in waist circumference or waist:height ratio. Total cholesterol was lowest in the Black African and highest in the Pakistani/Bangladeshi participants, while HDL-cholesterol was highest in the Black Caribbean and Black African participants and lowest in the Indian participants. The highest proportion of breakfast skipping occurred among the Black Caribbean (66 %) and Black African (64 %) groups and was lowest in the Indian group (46 %), although there were no statistical differences. Saturated fat intake was lowest among the Black African and Indian participants and highest among the Pakistani/Bangladeshi participants. Dietary fibre intake was highest in the White British and Indian groups and was significantly higher than the Black groups or the Pakistani/Bangladeshi participants. The Pakistani/Bangladeshi participants were significantly more likely to have a low daily consumption of vegetables, but there were no ethnic differences in fruit consumption.
Table 1 Dietary intake, anthropometry and cardiometabolic risk factors of participants, at 21–23 years, in the Determinants of Adolescent, now young Adults, Social well-being and Health study* (Mean values and 95 % confidence intervals)

HbA1c, glycated Hb.
a,b,c,d Values with unlike superscript letters within a row denote significant differences between those ethnic groups.
* Overweight/obese defined as BMI >25 kg/m2. Low fruit consumption defined as <2 portions/d; low vegetable consumption defined as <3 portions/d; skipping breakfast defined as not eating breakfast every day of the week. Continuous variables are shown as mean (95 % CI), categorical variables shown as n or %, as indicated in the variable label. Differences between ethnic groups in continuous variables were tested with one-way ANOVA with post hoc analysis.
† Ethnic differences in frequency variables were tested with χ 2 test with post hoc analysis.
We examined longitudinal patterns in the diet behaviours; there were significant increases in the rates of skipping breakfast (37 v. 56 %, P=0·035), low consumption of fruit (46 v. 57 %, P=0·024) and of vegetables (57 v. 63 %, P=0·021) from 11–13 to 21–23 years among the whole cohort (Fig. 1). Age was associated with risk of unhealthful diet behaviours, independent of sex and ethnicity. Individuals of 21–23 years old, were more likely to skip breakfast (OR: 2·65 (95 % CI 2·00, 3·50), P<0·001), consume low fruits (OR: 1·39 (95 % CI 1·08, 1·79), P=0·011) and vegetables (OR: 1·28 (95 % CI 0·99, 1·66), P=0·059) compared with when they were 11–13 years old, although the association for low vegetable consumption did not reach statistical significance. After adjusting for sex, ethnicity and educational attainment, skipping breakfast regularly at 11–13 years was a significant determinant of skipping breakfast in young adulthood (OR: 2·36 (95 % CI 1·62, 3·44), P<0·001). Low fruit and vegetable consumption at 21–23 years were also significant determinants of skipping breakfast at 21–23 years (fruit: OR: 2·21 (95 % CI 1·54, 3·18), P<0·001; vegetables: OR: 1·91 (95 % CI 1·32, 2·76), P=0·001) but low fruit and vegetable consumption at 11–13 years were not significant determinants of skipping breakfast in young adulthood (fruit: OR: 1·29 (95 % CI 0·88, 1·88), P=0·190; vegetables: OR: 1·32 (95 % CI 0·91, 1·91), P=0·150). Fig. 1 shows the rates of (a) skipping breakfast, (b) low fruit and (c) low vegetable consumption at 11–13 and 21–23 years by ethnic group. Small ethnic sample sizes precluded rigorous testing of ethnic-specific effects, nonetheless the descriptive data indicate ethnic variations. Increases in the rates of skipping breakfast were evident in all ethnic groups, with the greatest increase occurring in the White British group. Prevalence of low fruit consumption increased in all ethnic groups, with the Black African group experiencing the greatest increase. The rates of low vegetable consumption did not increase in either the White British or ‘other’ ethnic groups but increased in all other ethnicities, with the greatest increase occurring among the Black African group.

Fig. 1 Rates of (a) regularly skipping breakfast, (b) low daily fruit and (c) low daily vegetable consumption according to ethnicity at 11–13 (
) and 21–23 (
) years. The Determinants of Adolescent, now young Adults, Social well-being and Health study.
Longitudinal associations between dietary behaviours, anthropometric and cardiometabolic risk factors
The influence of the dietary behaviours during both adolescence and young adulthood on cardiometabolic risk factors during young adulthood was investigated using multivariate analysis, adjusting for sex, ethnicity, educational attainment, smoking, physical activity, energy and saturated fat intake (Table 2). Skipping breakfast at 11–13 years was a significant determinant of BMI at 21–23 years (1·41 (95 % CI 0·57, 2·26), P<0·001) as was skipping breakfast at 21–23 years, although the effect was slightly attenuated in this age group (1·05 (95 % CI 0·22, 1·89), P=0·014). Skipping breakfast at both 11–13 and 21–23 years was also important determinant of total cholesterol levels (11–13 years: 0·15 (95 % CI –0·01, 0·31), P=0·063; 21–23 years: 0·22 (95 % CI 0·06, 0·37), P=0·007). Low intake of fruit at 11–13 years was a significant determinant of waist:height ratio at 21–23 years (0·01 (95 % CI 0·00, 0·03), P=0·034), and regular fizzy drink consumption at 11–13 years was a significant determinant of systolic blood pressure at 21–23 years (1·77 (95 % CI 0·12, 3·43), P=0·036).
Table 2 Influence of dietary behaviours at 11–13 and 21–23 years on cardiometabolic risk factors at 21–23 years, in the Determinants of Adolescent, now young Adults, Social well-being and Health study* (Coefficients (Coef.) and 95 % confidence intervals)

HbA1c, glycated Hb; Ref., reference.
* Skipping breakfast defined as eating breakfast <5 d/week; low fruit consumption defined as <2 portions/d; low vegetable consumption defined as <3 portions/d; fizzy drink consumption defined as consuming fizzy drinks every day or most days. Multivariate linear regression models for BMI, waist:height ratio, total cholesterol, HDL-cholesterol, HbA1c and systolic blood pressure (separate models); coefficients were estimated with linear regression, adjusted for sex and ethnicity at 11–13 years, and additionally adjusted for educational attainment (attainment/non-attainment of higher education degree), physical activity (based on tertiles of sitting/sedentary time), smoking (current smoker/non-smoker), energy intake and saturated fat intake at 21–23 years. n 664, 635, 499, 484, 481 and 664, respectively, for linear regression models with BMI, waist:height ratio, total cholesterol, HDL-cholesterol, HbA1c, and systolic blood pressure, as the outcome.
Longitudinal associations between dietary behaviours and nutritional composition of the diet
The influence of the dietary behaviours during both adolescence and young adulthood on the nutritional composition of the diet during young adulthood was investigated using multivariate analysis, adjusting for sex, ethnicity, educational attainment, smoking and physical activity (Table 3). Skipping breakfast at both 11–13 and 21–23 years were significant determinants of lower dietary fibre intake (11–13 years: –0·81 (95 % CI –1·49, –0·12), P=0·022; 21–23 years: –1·33 (95 % CI –2·01, –0·65), P<0·001), as were regular fizzy drink consumption at 11–13 years (–0·72 (95 % CI –1·42, –0·02), P=0·045), low fruit consumption at 21–23 years (–1·22 (95 % CI –1·90, –0·54), P<0·001) and low vegetable consumption at 21–23 years (–1·30 (95 % CI –2·04, –0·56), P=0·001). Low fruit and vegetable consumption at 21–23 years were also important determinants of higher saturated fat intake (fruit: 0·89 (95 % CI –0·01, 1·78), P=0·052; vegetables: 1·06 (95 % CI 0·09, 2·02), P=0·032).
Table 3 Influence of dietary behaviours at 11–13 and 21–23 years on nutritional composition of the diet at 21–23 years, in the Determinants of Adolescent, now young Adults, Social well-being and Health study* (Coefficients (Coef.) and 95 % confidence intervals)
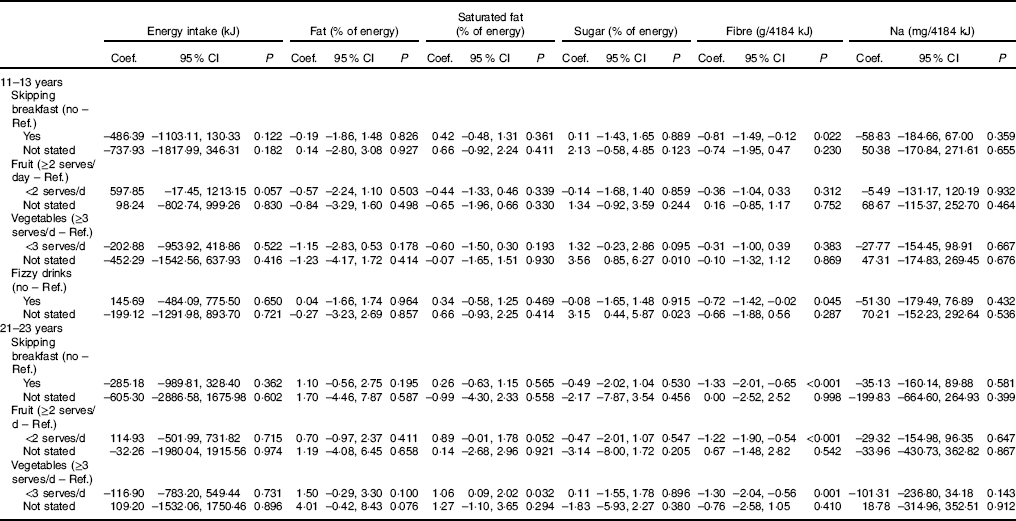
Ref., reference.
* Skipping breakfast defined as eating breakfast <5 d/week; low fruit consumption defined as <2 portions/d; low vegetable consumption defined as <3 portions/d; fizzy drink consumption defined as consuming fizzy drinks every day or most days. Multivariate linear regression models for energy intake, percentage energy from fat, percentage energy from saturated fat, percentage energy from sugar, fibre intake, Na intake (separate models); coefficients were estimated with linear regression, adjusted for sex and ethnicity at 11–13 years, and additionally adjusted for educational attainment (attainment/non-attainment of higher education degree), physical activity (based on tertiles of sitting/sedentary time) and smoking (current smoker/non-smoker) at 21–23 years. n 628 for linear regression models for all six nutritional composition variables as the outcome.
Discussion
We have undertaken a longitudinal assessment of nutritional intake and dietary behaviours and their association with cardiometabolic risk factors among an ethnically diverse cohort of young adults in the UK. In this work, we report the detrimental impact of childhood eating behaviours, such as regularly skipping breakfast, on cardiometabolic risk factors in young adulthood. We identified an increasing prevalence, from childhood to young adulthood, of less healthy dietary behaviours. Furthermore, we described distinct ethnic patterns, wherein skipping breakfast is most prevalent among Black African and Caribbean groups.
The DASH cohort was recruited primarily to investigate ethnic determinants and patterns of health; and through the collection of dietary data, we have been able to explore potential predictors of cardiometabolic risk in our young adults. In this study, we found the Black African and Caribbean groups to have the highest body weight and BMI; however, waist circumference and waist:height ratio were comparable with the other groups. In the Indian ethnic group, body weight and BMI were significantly lower compared with the White British and both Black ethnic groups. These findings are in line with the existing literature and suggest that higher body weight/BMI amongst African ancestry populations is not related to abdominal fat accumulation( Reference Gower, Nagy and Goran 25 ), although fat deposition in the abdominal region is usually more prevalent in South Asian populations( Reference Ramachandran, Snehalatha and Viswanathan 26 ). The Black Africans exhibited low total cholesterol and high HDL-cholesterol concentrations; these cardiometabolic risk differences may be attributed to dietary differences, namely, lower saturated fat, which was also apparent among the Black African participants. Among the Indian participants HDL-cholesterol was lower. Distinctions in nutritional intake were also evident for this group, namely, higher intake of fibre in the Indian group. Ethnic differences in cardiometabolic risk factors have been extensively reported; and in general, our data corroborate the literature. However, our categorisation of ethnicity, which is more detailed than that which is often employed, highlights some differences between our findings and the existing literature. Higher HbA1c levels amongst African Americans relative to White Americans have been previously reported( Reference Herman, Ma and Uwaifo 27 , Reference Ziemer, Kolm and Weintraub 28 ); in our study, the Black Caribbeans and Black Africans did not exhibit higher HbA1c levels. It is not clear the extent to which higher HbA1c among Black populations is a result of dietary intake v. biological factors. Higher sugar intake among Black Caribbean compared with Black West African adults has been previously described( Reference Goff, Timbers and Style 19 ); however, we did not find this in our participants, which is in agreement with Donin et al. ( Reference Donin, Nightingale and Owen 6 ) who reported no difference in sugar intake between Black Caribbean and Black African children in the Child Heart and Health Study in England (CHASE). Lower total cholesterol amongst Black Africans but not Black Caribbeans is in agreement with the findings from CHASE( Reference Donin, Nightingale and Owen 2 ) and may relate to lower saturated fat intake amongst Black Africans but not Black Caribbeans; a finding that is consistent with that of CHASE. Historically the African Caribbean diet has been recognised for its low intake of saturated fat, which has been proposed to contribute to the cardioprotective lipid profile that these ethnicities traditionally exhibited( Reference Vyas, Greenhalgh and Cade 29 – Reference Miller, Kotecha and Wilkinson 31 ). However, dietary acculturation and ‘Westernisation’ of the diet result in increasing fat intake, a change that is more apparent amongst Black Caribbean than Black African communities( Reference Goff, Timbers and Style 19 ), which may explain the lower total cholesterol concentration amongst the Black African group. Lower HDL-cholesterol amongst South Asian populations has been extensively reported in the literature( Reference Miller, Kotecha and Wilkinson 31 – Reference Knight, Smith and Whittles 33 ), and the nutritional profile that we identified is also consistent with previous reports( Reference Vyas, Greenhalgh and Cade 29 , Reference Miller, Kotecha and Wilkinson 31 , Reference McKeigue, Marmot and Adelstein 32 , Reference Sevak, McKeigue and Marmot 34 ).
Unhealthy dietary behaviours were highly prevalent among our young adults. In agreement with other longitudinal investigations, we saw that adolescent breakfast skipping was predictive of the behaviour in adulthood( Reference Smith, Gall and McNaughton 11 , Reference Merten, Williams and Shriver 14 ). In our study, breakfast skipping was particularly prevalent amongst certain ethnic groups. In agreement with other studies of children and young adults( Reference Donin, Nightingale and Owen 10 , Reference Merten, Williams and Shriver 14 , Reference Deshmukh-Taskar, Nicklas and Radcliffe 35 ), our Black African and Black Caribbean participants had the highest rates of breakfast skipping, although the White British group saw the greatest increase in skipping breakfast from childhood to young adulthood. Our longitudinal analysis indicated that skipping breakfast in childhood was an important determinant of BMI and total cholesterol concentration in young adulthood. Whilst there have been a number of longitudinal studies to demonstrate the detrimental effects of skipping breakfast on body weight and the development of obesity, there has been very little demonstration of the long-term effects of this behaviour on cardiometabolic risk factors, such as blood lipids. Our study is the first to focus on an ethnically diverse cohort that represents the main ethnic groups of the UK population. Before this, the long-term evidence was limited to the Australian CDAH study, which studied a predominantly White Australian population, and found skipping breakfast in adolescence has a unfavourable impact on waist circumference, fasting insulin and total cholesterol and LDL-cholesterol( Reference Smith, Gall and McNaughton 11 ). Furthermore, adolescent and young adult dietary behaviours were seen to be significant determinants of nutritional composition. Skipping breakfast and regular fizzy drink consumption during adolescence were associated with significantly lower intake of dietary fibre in young adulthood. In young adulthood, skipping breakfast and low consumption of fruits and vegetables were associated with significantly lower dietary fibre and significantly higher saturated fat intake. These findings corroborate with the literature, demonstrating the detrimental impact of skipping breakfast and other dietary behaviours on the quality of the diet( Reference Szajewska and Ruszczynski 13 ); these results are particularly alarming, given these unhealthy behaviours are most prevalent among some of our ethnic minority groups who are most at risk of developing obesity and cardiometabolic diseases such as T2D. Promoting high fibre intake and restriction of saturated fat are two of the most important public health nutrition campaigns in a bid to reduce chronic disease risk among the general population. Our findings provide a useful insight into dietary behaviours and specific ethnic groups that health promotion campaigns could target in aiming to improve the nutritional intake of the diets of young people.
The limitations of our study warrant consideration. The size of the cohort subsample is relatively small, and we did not have statistical power to investigate longitudinal patterns by ethnic group; however, it is the first cohort study of young adults to specifically focus on ethnic determinants of health and chronic disease risk. Furthermore, our study contributes to the very limited longitudinal data on the role of adolescent diet on cardiometabolic risk in young adulthood and is the first such study in the UK. We achieved a response rate of 97 % in our follow-up, which is remarkably high; this was achieved mainly through the use of social media, which allowed us to locate participants who had moved from their childhood residence. Our data collection focused on a relatively small number of cardiometabolic risk factors, which prevented us from assessing all aspects of risk, for example, the prevalence of metabolic syndrome, and making comparisons with the literature in this regard. Although our study is the first longitudinal study of adolescents and young adults to collect nutritional intake data, we were limited in our dietary data collection to assessing a single 24 h period and therefore we have not assessed micronutrient intake; this has limited our ability to investigate the role of, for example, vitamin D, in determining cardiometabolic risk. Under-reporting is a common issue in dietary data collection; we estimated under-reporting to affect 36 % of our recalls, which is comparable with other studies( Reference Poslusna, Ruprich and de Vries 36 ). Importantly, under-reporting was not associated with sex, ethnicity or educational attainment, suggesting there is no systematic bias in our dietary data. We did not exclude under-reporters from our analyses; our reporting period was relatively short, and we deemed low energy intake to be plausible over a 24 h period. In our dietary behaviour questionnaire, which asked about daily portions of fruit and vegetables, we provided portion size guidance to aid the participants in estimating their daily portions; however, our guidance was not exhaustive and did not focus on composite dishes such as soup, which may have led to inaccuracies in reporting. We did not collect data on fizzy drink consumption at 21–23 years due to time restrictions for our data collection visits; sugar-sweetened beverages are receiving significant attention at present due to their links with cardiometabolic risk; our data have not enabled us to look at longitudinal patterns of consumption. Our dietary analysis is also limited to focusing on single nutrients and food groups and does not consider interactions between these or reflect the complexity of dietary intake; dietary pattern analysis would enable a better understanding of dietary interactions.
In conclusion, findings from this cohort of young adults representing the major ethnic groups of the UK population demonstrate the detrimental effects of adolescent dietary behaviours, particularly skipping breakfast on cardiometabolic risk factors. The results also show these behaviours to be particularly prevalent amongst Black African and Caribbean ethnic groups. Our study highlights the importance of targeting health promotion interventions to improve dietary behaviours such as breakfast consumption at specific groups of young adults in the population.
Acknowledgements
The authors acknowledge the invaluable support of participants and their parents, the Participant Advisory Group, schools, civic leaders, local general practitioner surgeries and community pharmacies, the Clinical Research Centre at Queen Mary University of London, the Clinical Research Facility at University College Hospital, the survey assistants and nurses involved with data collection, Rachel Bonner, senior respiratory physiologist (UCL GOS ICH) for spirometry training and over-reading of data for quality control, the Primary Care Research Network, and Professor Sanders and Cruickshank at the Diabetes and Nutritional Sciences Division at Kings College London for hosting the feasibility study. S. H. is the Principal Investigator of DASH. All authors contributed to study design, analyses and writing of the article.
The study was funded by the MRC (MC_U130015185/MC_UU_12017/13), Chief Scientist Office (SPHSU13), North Central London Research Consortium and the Primary Care Research Network.
Data sharing: the DASH data are available to researchers via a data request to the MRC Social and Public Health Science Unit. Applications and the data sharing policy for DASH can be found at http://dash.sphsu.mrc.ac.uk/DASH_dsp_v1_November-2012_draft.pdf. It reflects the MRC guidance on data sharing with the aim of making the data as widely and freely available as possible while safeguarding the privacy of participants, protecting confidential data and maintaining the reputation of the study. All potential collaborators work with a link person, an experienced DASH researcher – to support their access to and analysis of the data. The variable-level metadata are available from the study team and also via the MRC Data Gateway.
MRC (MC_U130015185/MC_UU_12017/13), Chief Scientist Office (SPHSU13), North Central London Research Consortium and the Primary Care Research Network.
S. H., M. M., L. M. G. designed research; E. Z. E., A. C., C. B. conducted research; P. H., C. B., M. J. S., O. R. M. analysed data and performed statistical analyses; L. M. G. wrote manuscripts and had primary responsibility for final content.
The authors declare that there are no conflicts of interest.