Statistical learning (SL), the ability to use probabilistic co-occurrence to group elements present in the environment, has been argued to support development across multiple sensory and cognitive domains. One domain in which SL has been identified as playing a role is language development (Romberg & Saffran, Reference Romberg and Saffran2010; Saffran et al., Reference Saffran, Aslin and Newport1996), where it has been linked to learning across the subdomains of phonology (e.g., Kuhl, Reference Kuhl2004), segmentation and word learning (e.g., Hay et al., Reference Hay, Pelucchi, Graf Estes and Saffran2011; Lany & Saffran, Reference Lany and Saffran2010), and grammar (Kidd & Arciuli, Reference Kidd and Arciuli2016). A common assumption of research in this field is that SL results in detailed long-term memory representations for learned stimuli; however, how these representations endure and how they influence children’s performance in experiments is largely unknown. In the current paper, we explore this issue by asking how children’s knowledge of their native language influences their performance on an auditory SL task, and whether variation in performance is linked to their language proficiency.
There is significant evidence in favor of the argument that participants’ prior linguistic experience guides their SL of linguistic stimuli. Notably, many studies have shown that adult participants’ segmentation of words and grammatical patterns (e.g., non-adjacent dependencies) from running speech is constrained both by language-specific phonotactic constraints (i.e., rules) and, within the class of legal sequences, phonotactic probabilities within and across languages (e.g., Bonatti et al., Reference Bonatti, Peña, Nespor and Mehler2005; Dal Ben et al., Reference Dal Ben, Souza and Hay2021; Finn & Hudson Kam, Reference Finn and Hudson Kam2008; Mersad & Nazzi, Reference Mersad and Nazzi2011; Onnis et al., Reference Onnis, Monaghan, Richmond and Chater2005; Trecca et al., Reference Trecca, McCauley, Andersen, Bleses, Basbøll, Højen, Madsen, Ribu and Christiansen2019). Although infants and children have much less experience with language, their prior knowledge also influences SL. For instance, Lew-Williams and Saffran (Reference Lew-Williams and Saffran2012) found that nine- and ten-month-old infants’ statistical speech segmentation was guided by their expectations about word length, which were forged through pre-exposure to words that were either consistent or inconsistent in length with items in the to-be-segmented stream. These findings are echoed in work by Thiessen et al. (Reference Thiessen, Onnis, Hong and Lee2019), who demonstrated that 13-month-old, but not seven-month-old, native English-learning infants showed a preference for attending to backward versus forward transitional probabilities (TPs) in an ambiguous artificial language. That is, older infants attended to the direction which aligned with the structural properties of their native language (i.e., the head-initial nature of English, which favors backward processing; see Onnis & Thiessen, Reference Onnis and Thiessen2013, for related findings with adults). Taken together, these data provide converging evidence that learners’ expectations about linguistic input emerge over experience with language and can permeate into the laboratory to shape participants’ performance on SL tasks.
In the current paper, we ask a related though crucially different question: do children draw upon their distributional knowledge of syllable transitions when processing new statistically defined linguistic input? Specifically, does the presence of attested and therefore highly probable bigrams from participants’ native language boost SL? Attention to TPs has featured prominently in explanations of SL, since this is how higher units of linguistic organization (i.e., “words” in studies of segmentation) have been defined (Saffran et al., Reference Saffran, Aslin and Newport1996). Successful learning on these tasks indicates that participants either track TPs or chunk co-occurring syllables into bigger units (Aslin et al., Reference Aslin, Saffran and Newport1998; Perruchet, Reference Perruchet2019; Perruchet & Vinter, Reference Perruchet and Vinter1998). The underlying assumption that the output of SL – the acquisition of statistical structure as defined by TPs – leads to long-term representations that aid future learning is less frequently tested. Accordingly, we ask whether participants use these prior expectations about TPs in their ambient language, which they have presumably acquired across development, to process new input.
There is growing evidence from adults in support of this proposal. In a series of studies investigating the interrelationships between visual SL and auditory SL for linguistic and non-linguistic stimuli, Siegelman et al. (Reference Siegelman, Bogaerts, Elazar, Arciuli and Frost2018) reported that, while visual SL and auditory SL for non-linguistic stimuli were related (suggesting a common underlying capacity for performance across modalities), auditory SL for linguistic stimuli was not related to the other two. Indeed, they reported poor internal consistency for their linguistic auditory SL task, suggesting that participants showed little overlap in performance across individual test items within the task. However, Siegelman et al. found that performance on the linguistic SL task was predicted by independent ratings of “wordness” for the test items. That is, the more closely a test word resembled a real word in the participants’ native language (Hebrew), the more likely they would be to segment it from the speech stream and recognize it as a word at test, suggesting that participants entered the experiment with entrenched linguistic knowledge affecting their performance on the SL tasks based on linguistic items.
Two more recent studies with adult populations provide more direct evidence for this entrenchment effect. In a between-participants design, Elazar et al. (Reference Elazar, Alhama, Bogaerts, Siegelman, Baus and Frost2022) tested Spanish-speaking participants on an auditory SL task using one of two speech streams. In a “Spanish-like” condition, participants listened to a stream of continuous speech containing test words defined by TPs that were highly attested in Spanish corpora. In a “Spanish-unlike” condition, the test words were defined by TPs that were rarely attested. Overall, the authors reported a pattern of results that was suggestive of a learning advantage for Spanish-like stimuli, thus providing evidence in favor of the argument that participants build expectations of likely syllable transitions, which they use as priors when parsing new input.
In a study that we build upon in the current paper, Stärk et al. (Reference Stärk, Kidd and Frost2023) investigated whether native German-speaking adults drew on their prior knowledge of German syllable co-occurrences (i.e., syllable pairs with high TPs) to acquire new but distributionally consistent linguistic input. Unlike Elazar et al. (Reference Elazar, Alhama, Bogaerts, Siegelman, Baus and Frost2022), the authors tested the influence of attested TPs in a within-participants design, a more stringent test of the entrenchment hypothesis since any across-condition differences could not be attributed to differences across participants. Doing so required a different method of measuring SL. Accordingly, participants heard and repeated three different kinds of sequences in a serial recall task: two of which were structured, while the other sequences were unstructured foils, with the dependent measure being recall accuracy. The structured sequences contained bisyllabic experimental words that were either based on likely German syllable transitions (naturalistic sequences) or were devoid of attested transitions (non-naturalistic sequences). The unstructured sequences were scrambled combinations of syllables, serving as baseline. Findings indicated that adults drew on their knowledge of German syllable transitions during the task and did so from the early phases of the experiment onward, showing higher recall accuracy and faster improvement for the naturalistic sequences over the other two sequence types. The participants also performed significantly better on recall of the non-naturalistic sequences in comparison to foils, demonstrating that they were able to acquire knowledge of two sequences within the context of the task, despite the fact that there was likely a degree of interference across conditions because all sequences were constructed from the same syllable inventory.
Overall, the work of Elazar et al. (Reference Elazar, Alhama, Bogaerts, Siegelman, Baus and Frost2022) and Stärk et al. (Reference Stärk, Kidd and Frost2023) provides converging evidence in support of the hypothesis that adults draw on their prior linguistic knowledge to process and learn subsequent input, which raises the question of whether children differ from adults in their weighing of prior knowledge and the input statistics, given their ability to pick up on new statistics present in a SL task. This may or may not differ from adults, depending on the paradigm (see Raviv & Arnon, Reference Raviv and Arnon2018; Shufaniya & Arnon, Reference Shufaniya and Arnon2018). Notable differences between adults and children have been found in studies of verbal learning. For instance, Smalle et al. (Reference Smalle, Muylle, Szmalec and Duyck2017) showed that nine- to ten-year-old children require substantially less exposure to implicitly acquire phonotactic restrictions on novel words than adults do. Additionally, Smalle et al. (Reference Smalle, Page, Duyck, Edwards and Szmalec2018) have reported that eight- to nine-year-old children retain implicitly learned phonological sequences better than adults, as demonstrated through Hebbian Repetition Learning. This raises the possibility that, while still within the sensitive period for language acquisition, children differ from adults. Consequently, we investigate whether children draw on their prior distributional knowledge when processing subsequent linguistic input, as previously reported in adults (Elazar et al., Reference Elazar, Alhama, Bogaerts, Siegelman, Baus and Frost2022; Stärk et al., Reference Stärk, Kidd and Frost2023).
Furthermore, we address the issue concerning whether and how performance in laboratory-based SL tasks relates to children’s real-world language development. Typically developing children’s language proficiency (as measured with assessments of their expressive and receptive vocabulary) has been found to be correlated with their SL ability (e.g., Evans et al., Reference Evans, Saffran and Robe-Torres2009; Frost et al., Reference Frost, Jessop, Durrant, Peter, Bidgood, Pine, Rowland and Monaghan2020; Kidd & Arciuli, Reference Kidd and Arciuli2016; Lany, Reference Lany2014; though the relationship is not always observed and appears to be task-dependent, see West et al., Reference West, Melby-Lervåg and Hulme2021). Here we take a slightly different approach and ask: do individual differences in language proficiency result in different learning trajectories throughout the course of SL (e.g., as measured over the course of our experiment)? If SL constitutes an individual ability that supports natural language learning (Siegelman et al., Reference Siegelman, Bogaerts, Christiansen and Frost2017), we should expect children of different language abilities to be differentially sensitive to statistically defined sequences, under the assumption that superior SL abilities (and its component processes, Arciuli, Reference Arciuli2017) have supported acquisition throughout development.
Thus, in the present study, we examined the effect of prior distributional knowledge on the learning of statistically defined linguistic input in seven- to nine-year-old German-speaking children. We utilized the repetition paradigm from Stärk et al. (Reference Stärk, Kidd and Frost2023) as described above, adjusting it for children’s lower working memory capacity by shortening the sequence length. We hypothesized that children would recall naturalistic sequences better than non-naturalistic sequences and show faster improvement for the naturalistic than non-naturalistic sequences, similar to the findings with adults (Elazar et al., Reference Elazar, Alhama, Bogaerts, Siegelman, Baus and Frost2022; Stärk et al., Reference Stärk, Kidd and Frost2023). Additionally, we investigated whether children’s language proficiency was related to their SL. While past studies have reported correlational analyses between SL performance and language proficiency, including studies utilizing sequence repetition (e.g., Smalle et al., Reference Smalle, Page, Duyck, Edwards and Szmalec2018), we were interested in how differences in language proficiency influenced the dynamics of learning throughout the task. Since no similar study exists, this analysis was exploratory.
Method
This study was preregistered on AsPredicted: https://aspredicted.org/546hk.pdf. All of our materials, data, analyses, and results are openly available on OSF: https://osf.io/t5qf4/.
Participants
Forty-nine seven- to nine-year-old native German-speaking children (29 female, 20 male; mean age = 8;7 years; months, SD = 0;6, range: 7;6–9;11) without any known hearing, speech, or language disorders were included in the final sample (N = 49). We had planned to test 60 children, but recruitment and testing were hindered by the COVID-19 pandemic. Despite not being able to recruit our originally planned sample, our power analysis suggests that a sample of 49 is sufficient to detect an effect size of 0.2 syllables recall difference between naturalistic and non-naturalistic sequences as well as between non-naturalistic and foil sequences (i.e., an effect size half the size of the one found in adults, compensating for children’s cognitive development). The analysis was conducted in R 4.1.2 (R Core Team, 2022) using the package simr 1.0.6 (Green & MacLeod, Reference Green and MacLeod2016) and relied upon data from Stärk et al. (Reference Stärk, Kidd and Frost2023) whose design we adjusted for the current study (see the Analysis folder on OSF for a detailed description of this and two post hoc power analyses).
Participants were recruited from a German primary school in Leipzig, Germany. Invitations for participation were sent to the parents of all second- and third-graders (with German second-graders being 7–8 years old and third-graders being 8–9 years old). Of these, 55 consented to participating in our experiment. Data for six children were excluded from analyses: two did not fulfil the inclusion criteria regarding language proficiency (see Design section for more details), two found the serial recall task (i.e., our SL measure) too difficult to complete, and two were excluded due to technical failure. The study was approved by the Ethical Committee of the Faculty of Social Sciences, Radboud University, and was carried out in accordance with the World Medical Association Declaration of Helsinki. Children were free to withdraw at any time. They received a certificate and stickers for their participation.
Design
The experiment utilized a serial recall task, in which participants were presented with sequences of six syllables and repeated them out loud (see Figure 1). The task is based on studies of the Hebb repetition effect (Hebb, Reference Hebb and Delafresnaye1961; Page & Norris, Reference Page and Norris2009), and was modified from Stärk et al.'s (Reference Stärk, Kidd and Frost2023) related study with adults to be suitable for children. Specifically, we reduced the length of the sequences (sequences comprised 6 syllables, rather than 8), thereby making allowances for the fact that working memory (Cowan, Reference Cowan2016) and other cognitive processes supporting performance (e.g., processing speed, Kail & Salthouse, Reference Kail and Salthouse1994) improve across childhood. The study had a within-participants design, in which all participants received all three sequence types: naturalistic, non-naturalistic, and unstructured foils. The naturalistic and non-naturalistic sequences were structured, with each containing three bisyllabic experimental words, whereas unstructured sequences comprised the same syllables as the structured sequences but in a scrambled order (see the Materials section for further details). Participants’ repetitions of the sequences were scored for accuracy, and we examined performance across the task to gain insights into learning over the course of exposure.

Figure 1. Three example experimental sequences: one naturalistic sequence (1), one unstructured foil sequence (2), and one non-naturalistic sequence (3). On each trial, participants listened to a six-syllable sequence and then repeated it. Design adapted from Stärk et al. (Reference Stärk, Kidd and Frost2023).
Materials
Language proficiency assessments
We assessed the children’s language proficiency to ensure that both their lexical and morphosyntactic knowledge was representative of the average German-speaking seven- to nine-year-old. To measure their lexical knowledge, we used the German Peabody Picture Vocabulary Test version IV (PPVT; Lenhard et al., Reference Lenhard, Lenhard, Segerer and Suggate2015). This is a test of receptive vocabulary, in which children are shown four pictures per trial and have to identify the picture that best matches a word they were given. Their correct responses are counted and converted into an age-dependent score. Children whose performance was 1.5 SDs below the average of their age group were excluded from the main analyses.
To measure the children’s morphosyntactic knowledge, we used the German LITMUS sentence repetition task (SRT; Abed Ibrahim et al., Reference Abed Ibrahim, Hamann, Öwerdieck, Bertolini and Kaplan2018; Hamann et al., Reference Hamann, Chilla, Ruigendijk and Abed Ibrahim2013; Hamann & Abed Ibrahim, Reference Hamann and Abed Ibrahim2017). This test consists of 45 sentences with varying degrees of difficulty, which children listen to and repeat. Their correct responses are counted and form their score. If performance was 2.5 SDs below the average estimated from our own sample, children were excluded from the main analyses. A more lenient cut-off for the SRT was chosen because the test is not standardized.
Experimental stimuli
For the serial recall task (i.e., our SL measure), participants repeated sequences of six syllables. There were three different sequence types presented pseudo-randomly throughout the exposure: (i) naturalistic sequences, (ii) non-naturalistic sequences, and (iii) unstructured foil sequences. The first two sequence types were structured, meaning that they contained experimental words which participants could segment, while the unstructured foil sequences did not contain any learnable patterns. Importantly, the words contained in the naturalistic sequences differed from the words contained in the non-naturalistic sequences, in that the naturalistic words comprised frequently co-occurring German syllable pairs while the non-naturalistic words comprised unattested syllable pairs. That is, neither sequence type contained natural German words but the naturalistic words might appear familiar to German speakers because of their high TPs in natural German. They represent the outcome of participants’ SL in natural German. In the current study, we investigate whether seven- to nine-year-old children draw on this prior knowledge (i.e., the outcome of previous SL in natural language) to process a new experimental language (as modeled by the six naturalistic words). This is compared to participants’ SL in a language without prior knowledge (as modeled by the six non-naturalistic words).
The experimental words were created via a corpus analysis, which analyzed the TPs of the 1000 most frequent words of German child-directed speech on the CHILDES database (MacWhinney, Reference MacWhinney2000) Footnote 1 . Six syllable pairs (i.e., bigrams) occurring with high within-word backward TPs were selected for use as the naturalistic words (naturalistic words: gefa, minu, moti, pagei, versu, zusa; see Stärk et al., Reference Stärk, Kidd and Frost2022, for evidence that backward TPs are more reliable cues to ‘wordness’ than forward TPs in German speech). These were in turn used to create the naturalistic sequences. Importantly, the experimental naturalistic words did not comprise existing German words, but the high TPs (TP > 0.20, with an average TP = 0.69 and a range of 0.21–1) between the syllables within each pair make them potentially familiar to German speakers. There was no repetition of syllables across the words. The non-naturalistic words were created by switching the first and second syllables of the naturalistic words and combining each final syllable with a different first syllable, such that the syllable pairs neither form German words nor have high TPs (TP = 0) in natural German (non-naturalistic words: fazu, geimi, nuver, samo, suge, tipa). Unstructured foil sequences consisted of the same 12 syllables presented in a scrambled order, such that these sequences contained neither learnable patterns nor German or experimental words.
Within the context of the experiment, both types of structured sequences had perfect within-word TPs (structured sequences: within-word TPs = 1, between-word TPs ≤ 0.25), while the TPs between syllables in the unstructured foil sequences were generally low (unstructured sequences: TPs ≤ 0.17). Since participants repeated all three sequence types, with syllables being repeated across types, within-word TPs for both structured sequences were TP = 0.33, and TPs for all other syllable pairs were TPs ≤ 0.14, calculated over the entire exposure. Forward and backward TPs were equal within the experimental sequences (see Stimuli file on OSF for the precise numbers and the details of the stimuli creation).
Each syllable was recorded in isolation by a female native speaker of German. The recordings were adjusted using Audacity (Audacity Team, 2018) to ensure uniformity of length, resulting in an average syllable duration of 377ms (range: 352ms–416ms). The experiment comprised 72 sequences in total, 24 of each sequence type. Each sequence consisted of six syllables (i.e., three experimental words in the structured sequences), separated by 500ms of silence. Thus, while we refer to our structured sequences as containing “words,” it is important to remember that to the participant, the stimuli were lists of syllables; thus, any grouping of the syllables based on TPs, be it from existing naturalistic knowledge or knowledge gained through the course of the task, is evidence of SL, which in this case can be interpreted as the chunking of co-occurring syllables. The final syllable of each sequence was followed by a beep, which indicated that participants could start their repetition.
The experiment was divided into 12 blocks of six sequences, which contained two items of each sequence type. Within each block, sequences were presented pseudo-randomly, with no direct repetition of a particular sequence type (e.g., there was no adjacent presentation of naturalistic sequences). There were four experimental lists, which differed in the order of blocks (and with which sequence type the experiment started). Participants were randomly assigned to one list. Across the entire experiment, each word occurred 12 times in total, appearing equally often in each position within a sequence (for more information on the stimuli and their creation see the Materials folder of the project on OSF).
Procedure
Children whose parents signed the consent form were invited for two experimental sessions at their after-school club. The children were tested over two sessions of approximately 30 minutes. In the first session, children completed the German PPVT (Lenhard et al., Reference Lenhard, Lenhard, Segerer and Suggate2015) and the SRT (Abed Ibrahim et al., Reference Abed Ibrahim, Hamann, Öwerdieck, Bertolini and Kaplan2018; Hamann et al., Reference Hamann, Chilla, Ruigendijk and Abed Ibrahim2013; Hamann & Abed Ibrahim, Reference Hamann and Abed Ibrahim2017). In the second session, they completed the serial recall task (i.e., our main task, measuring children’s SL). Both sessions took place in a quiet, private room at the school. Stimuli were played over closed-cup headphones via a laptop, and the children repeated the sequences into a microphone, with repetitions being recorded for subsequent offline coding. The serial recall task was conducted using the software Presentation (Neurobehavioral Systems, Inc, 2014).
If the children fulfilled the language proficiency inclusion criteria in the first session, they were invited for the second session on the following day. The serial recall task was introduced as a game, which had the aim of helping an alien to repair their broken spaceship and fly back to their home planet. The children were told that they could help the alien by repeating what it says. First, they received six unstructured practice trials: three four-syllable sequences and three six-syllable sequences, comprising a different set of syllables than the experimental sequences (ba, fun, gi, re, se, to), before receiving the 72 experimental sequences. The children received a sticker and were told more components of the alien story after the practice trials, during the two breaks (after Sequences 24 and 48), and after completing the experiment. At the end of the session, the children were debriefed and received a certificate.
Data preparation
Language proficiency assessments
The PPVT was coded online by the experimenter, while the SRT was transcribed and coded offline upon completion by the experimenter and a trained assistant. The SRT data were coded for identical repetition following Hamann and Abed Ibrahim (Reference Hamann and Abed Ibrahim2017). That is, children received a point for each sentence repeated entirely verbatim. Five participants’ SRT recordings (10%) were transcribed by both coders for inter-transcriber reliability analyses, which revealed a strong reliability between the two transcribers (observed agreement (OA) = 94.7%; κ = 0.83 with 95% CIs of [0.74, 0.93]; following the more conservative interpretation of the kappa statistic suggested by McHugh, Reference McHugh2012). Furthermore, Cronbach’s alpha (α) was calculated for the SRT to determine task-internal reliability, which revealed good internal consistency (α = .81 [.74, .88]). This justifies its use as individual differences measure in our exploratory analysis (see OSF for almost identical values of McDonald’s omega).
Serial recall task
Sequence repetitions were transcribed by the experimenter and a trained assistant. Participants’ repetitions were scored at both the syllable and the bigram level, with a bigram being an experimental word in the two structured conditions and random syllable pairs in the unstructured condition (therefore referred to as “bigram level” rather than “word level” here). At the syllable level, participants received one point for each syllable repeated correctly in the correct position (max. 6 points per sequence). At the bigram level, participants received one point for each bigram (i.e., “word” or syllable pair) repeated correctly in the correct position (max. 3 points per sequence), providing valuable information about whether participants recalled sequences better because of learning the experimental words (i.e., bigrams) in the structured sequence types. In the unstructured sequences, the three bigrams per sequence were the random syllable pairs in Syllable positions 1 and 2, 3 and 4, and 5 and 6 (with syllable combinations varying between sequences).
Recall scores at the syllable and bigram level were highly correlated for all three sequence types (naturalistic sequences: r(47) = .96, p < .001; non-naturalistic sequences: r(47) = .94, p < .001; unstructured foil sequences: r(47) = .88, p < .001). Data for five participants were transcribed by both coders for inter-transcriber reliability analyses, revealing a moderate to strong reliability between the two transcribers (syllable level: OA = 79.2%; κ = 0.74 [0.69, 0.79]; bigram level: OA = 88.3%; κ = 0.80 [0.74, 0.86]; again following the more conservative interpretation of kappa suggested by McHugh, Reference McHugh2012). Furthermore, Cronbach’s alpha (α) was calculated for the serial recall task as measure of task-internal reliability, revealing acceptable to excellent internal consistency (see Table 1, and see OSF for almost identical values of McDonald’s omega).
Table 1. Task-internal consistency measures for the serial recall task

Notes: Statistic: α = Cronbach’s alpha.
We preregistered two different coding schemes for the serial recall task, the one described above and a serial-order coding scheme, which grants participants points more generously for repeating a syllable or bigram in the correct serial order rather than in the exact position within the sequence (see Isbilen et al., Reference Isbilen, McCauley, Kidd and Christiansen2020, and Kidd et al., Reference Kidd, Arciuli, Christiansen, Isbilen, Revius and Smithson2020). We report the results of the former coding scheme only, since the strict scheme is more conservative. However, the results of all coding schemes can be found in the Analysis folder of our OSF project. The results do not differ substantially between the different coding schemes.
Results
All of the analyses presented in this paper were performed in R 4.1.3 (R Core Team, 2022) using RStudio (RStudio Team, 2022). Data preprocessing and visualization were performed using the package tidyverse 1.3.1 (Wickham, Reference Wickham2017; Wickham et al., Reference Wickham, Averick, Bryan, Chang, McGowan, François, Grolemund, Hayes, Henry, Hester, Kuhn, Pedersen, Miller, Bache, Müller, Ooms, Robinson, Seidel, Spinu and Yutani2019).
Language proficiency assessments
On the PPVT, the children scored on average 54.63 (SD = 9.07, range: 38–73), which is slightly higher than the normed average of 50. One child performed below our inclusion threshold of 1.5 SDs below the norming average (PPVT = 35) and was thus excluded from the analyses. On the SRT, the children scored on average 34.89 (SD = 5.36, range: 23–44). One child scored 2.2 SDs below the group average on the standard scoring criteria, but below the inclusion threshold of 2.5 SDs on more sensitive coding schemes (see preregistration, coding scheme, and analysis files on OSF). This participant was excluded from the analyses.
Serial recall task
Analysis by experimental block
We analyzed the data using generalized linear mixed-effects models (package lmerTest 3.1-3; Kuznetsova et al., Reference Kuznetsova, Brockhoff and Christensen2017; based on lme4 1.1-28; Bates et al., Reference Bates, Mächler, Bolker and Walker2015), with syllable recall and bigram recall as the dependent variables to test overall recall and recall of the experimental words, respectively. We specified a Poisson distribution with a log-link, since the dependent measures were count variables. Models were fitted with a fixed effect of sequence type using sliding contrasts (naturalistic: 0.5 vs. non-naturalistic: −0.5, and non-naturalistic: 0.5 vs. foils: −0.5) to examine whether recall differed across the experimental conditions, and a fixed effect of block was entered as a centered continuous variable to examine learning over the course of the experiment. Additionally, the interaction between the two factors was included as a fixed effect. We fitted the maximal model supported by the data (Barr et al., Reference Barr, Levy, Scheepers and Tily2013), controlling for participants and items as random intercepts, with sequence type and block (as well as their interaction) as random slopes for participants, but not items as those differed between sequence types and blocks. The marginal and conditional R 2 effect sizes are also reported as goodness-of-fit estimates. These denote the proportion of the variance explained by the model both with (conditional R 2) and without (marginal R 2) controls for sources of random variance (Johnson, Reference Johnson2014; Nakagawa & Schielzeth, Reference Nakagawa and Schielzeth2013).
There was a significant main effect of sequence type at both the syllable level and the bigram level, with participants displaying better recall for naturalistic than non-naturalistic sequences (see Table 2). At the bigram level, participants also showed better recall for the non-naturalistic than the unstructured foil sequences, indicating that the non-naturalistic words were also learnt, even though this did not facilitate the overall syllable recall for those sequences (for a visualization of participants’ syllable and bigram recall accuracy, see Figures 2 and 3, respectively). There was no significant main effect of block, and no significant interaction between block and sequence type, suggesting that the conditional differences across sequence types reflected the overall performance of children across the experiment.
Table 2. Summary of the linear mixed-effects models investigating the influence of sequence type and block on the children’s syllable and bigram recall, respectively

Notes: Sequence type: Nat. = naturalistic sequences, Non-nat. = non-naturalistic sequences, Foils = unstructured foil sequences. Model fit syllable level: AIC = 10,961; BIC = 11,023; R 2 m = 0.027; R 2 c = 0.088; ICC = 0.063; RMSE = 1.248; σ = 1; model fit bigram level: AIC = 5661; BIC = 5742; R 2 m = 0.046; R 2 c = 0.415; ICC = 0.387; RMSE = 0.599; σ = 1.

Figure 2. Mean syllable recall per sequence for the three sequence types (naturalistic, non-naturalistic, and unstructured foils), given by experimental block (1-12). Error bars indicate standard error.

Figure 3. Mean bigram recall per sequence for the three sequence types (naturalistic, non-naturalistic, and unstructured foils), given by experimental block (1-12). Error bars indicate standard error.
Analysis by exposure phase
While there were no effects of block in the previous analyses, performance across the experiment was not totally even. Stärk et al. (Reference Stärk, Kidd and Frost2023) found that adult performance in the current task varied across phases of the experiment (early, intermediate, and late). We conducted the same analysis, dividing the exposure into three phases, separated by breaks during the data acquisition (early exposure: Blocks 1–4; intermediate exposure: Blocks 5–8; late exposure: Blocks 9–12). Accordingly, we added exposure phase instead of block as a fixed effect in the models examining children’s syllable and bigram recall. We fitted the maximal model supported by the data (Barr et al., Reference Barr, Levy, Scheepers and Tily2013) with sequence type (sliding contrast: naturalistic: 0.5 vs. non-naturalistic: −0.5, and non-naturalistic: 0.5 vs. foils: −0.5), exposure phase (sliding contrast: early exposure: −0.5 vs. intermediate exposure: 0.5, and intermediate exposure: −0.5 vs. late exposure: 0.5), and their interaction as fixed effects, and random intercepts and slopes for participants and items, where appropriate.
Comparable to the results of the previous analysis, we found significant main effects of sequence type but neither a main effect of exposure phase nor an interaction between the two factors (see Table 3). For an illustration of syllable and bigram recall accuracy over the three phases see Figures 4 and 5.
Table 3. Summary of the linear mixed-effects models investigating the influence of sequence type and exposure phase on the children’s syllable and bigram recall, respectively

Notes: Sequence type: Nat. = naturalistic sequences, Non-nat. = non-naturalistic sequences, Foils = unstructured foil sequences. Exposure phase: Early, Intermediate, Late. Model fit syllable level: AIC = 10,962; BIC = 11,085; R 2 m = 0.024; R 2 c = 0.394; ICC = 0.379; RMSE = 1.246; σ = 1; model fit bigram level: AIC = 5674; BIC = 5761; R 2 m = 0.045; R 2 c = 0.084; ICC = 0.041; RMSE = 0.598; σ = 1.

Figure 4. Mean syllable recall per sequence for the three sequence types (naturalistic, non-naturalistic, and unstructured foils), given by exposure phase (early, intermediate, late). Error bars indicate standard error.

Figure 5. Mean bigram recall per sequence for the three sequence types (naturalistic, non-naturalistic, and unstructured foils), given by exposure phase (early, intermediate, late). Error bars indicate standard error.
Relationship between language proficiency and SL
We next report our exploratory analyses investigating whether differences in children’s performance on the SL task were related to their native-language proficiency. We obtained two measures of language proficiency – vocabulary size, as measured by the PPVT, and morphosyntactic processing, as measured by the SRT. Table 4 shows the bivariate correlations between all variables, in addition to partial correlations between repetition of structured sequences and language proficiency, controlling for foil repetition. All bivariate correlations between repetition of syllable sequences and the SRT and PPVT were positive and all but two were significant. The partial correlations testing the relationship between syllable recall and language proficiency controlling for foil repetition were lower. Only the partial correlation between naturalistic repetition of bigrams and SRT performance was significant.
Table 4. Pearson bivariate correlations between children’s recall in the three sequence types (naturalistic, non-naturalistic, and foil sequences) and their performance on the language proficiency assessments (SRT and PPVT) at the syllable (left) and bigram level (right). Partial correlations between language assessments and structured sequences, controlling for foil repetition, appear in brackets

Notes: Sequence type: Nat. = naturalistic sequences, Non-nat. = non-naturalistic sequences, Foils = unstructured foil sequences. N = 49; * p < .05, ** p < .01, *** p < .001
While the simple correlations between sequence recall and PPVT or SRT were significant, we chose the latter measure as our individual differences variable because the recall task and the SRT both involve sequencing linguistic units in the same modality, but with different units (syllables versus morphemes). Finding that the two are systematically related would provide evidence in support of the idea that sequencing of syllables and morphemes share an underlying common and statistically sensitive mechanism (Isbilen et al., Reference Isbilen, McCauley and Christiansen2022). Thus, we next analyzed whether SRT performance influenced children’s performance on the experiment. We fitted the maximal model supported by the data (Barr et al., Reference Barr, Levy, Scheepers and Tily2013) with sequence type (sliding contrast: naturalistic: 0.5 vs. non-naturalistic: −0.5, and non-naturalistic: 0.5 vs. foils: −0.5), block (centered continuous variable) and SRT (centered continuous variable), as well as the interactions between the three factors, as fixed effects, and random intercepts and slopes for participants and items, where appropriate. In addition to the main effects of sequence type described above, there was a significant main effect of SRT score (syllable level: β = 0.41 [0.32, 0.50], t = 8.34, p < .001; bigram level: β = 0.59 [0.41, 0.76], t = 6.55, p < .001), with children who scored higher on the SRT displaying better syllable and bigram recall on the experimental task. Furthermore, there was a significant interaction between sequence type and SRT performance (syllable level: β = −0.05 [−0.09, 0.00], t = −2.04, p = .04; bigram level: β = −0.11 [−0.20, −0.02], t = −2.36, p = .02), with a greater recall difference between naturalistic and non-naturalistic sequences for children with lower SRT scores compared to their higher SRT scoring peers. At the syllable level, there was an additional significant interaction between block and SRT performance (syllable level: β = 0.05 [0.00, 0.09], t = 2.25, p = .03), with children with higher SRT scores showing greater improvement in syllable recall throughout the experiment, although the effect was not significant at the bigram level (see analysis file on OSF for full details).
Given that linguistic proficiency was related to performance across the experiment, we scrutinized this further by replacing the variable of block with the more interpretable variable of phase (early, intermediate, late). We fitted the maximal model supported by the data (Barr et al., Reference Barr, Levy, Scheepers and Tily2013) with sequence type (sliding contrast: naturalistic: 0.5 vs. non-naturalistic: −0.5, and non-naturalistic: 0.5 vs. foils: −0.5), exposure phase (sliding contrast: early exposure: −0.5 vs. intermediate exposure: 0.5, and intermediate exposure: −0.5 vs. late exposure: 0.5), and SRT performance (centered continuous variable), as well as the interactions between the three factors, as fixed effects, and random intercepts and slopes for participants and items, where appropriate. The outcomes of the model analyzing the data at the syllable level are given in Table 5, and the outcomes of the model analyzing the data at the bigram level are given in Table 6. As in the previous models, we found significant main effects of sequence type (with syllable recall only differing between naturalistic and non-naturalistic sequences, and bigram recall additionally differing between non-naturalistic and foil sequences) and SRT performance. The main effect of exposure phase was not significant. At the syllable level, we found two significant interactions. The interaction between SRT performance and sequence type indicated that children with lower SRT scores showed a greater recall difference between naturalistic and non-naturalistic sequences than children with higher SRT scores. The interaction between SRT performance and exposure phase indicated that children with higher SRT scores showed stronger improvement between the early and intermediate exposure phase than children with lower SRT scores (see Figure 6, which plots performance for “High” and “Low” SRT performers, determined by median split).
Table 5. Summary of the linear mixed-effects model investigating the influence of sequence type, exposure phase, and SRT score on the children’s syllable recall

Notes: Sequence type: Nat. = naturalistic sequences, Non-nat. = non-naturalistic sequences, Foils = unstructured foil sequences. Exposure phase: Early, Intermediate, Late. Model fit: AIC = 10,924; BIC = 11,103; R 2 m = 0.225; R 2 c = 0.395; ICC = 0.219; RMSE = 1.246; σ = 1.
Table 6. Summary of the linear mixed-effects model investigating the influence of sequence type, exposure phase, and SRT score on the children’s bigram recall

Notes: Sequence type: Nat. = naturalistic sequences, Non-nat. = non-naturalistic sequences, Foils = unstructured foil sequences. Exposure phase: Early, Intermediate, Late. Model fit: AIC = 5647; BIC = 5789; R 2 m = 0.222; R 2 c 0.254; ICC = 0.041; RMSE = 0.599; σ = 1.

Figure 6. Mean syllable (top) and bigram (bottom) recall per sequence for the three sequence types (naturalistic, non-naturalistic, and unstructured foils) of the children with an SRT score higher than the median split (left) and lower than the median split (right), by exposure phase (early, intermediate, late). Error bars indicate standard error.
Children’s performance showed a dramatic decrease in the final block, most likely driven by fatigue, which could potentially obscure learning effects across the later part of the experiment. To rule out this possibility, we ran one final exploratory analysis without Block 12, reducing the late exposure phase to Blocks 9 to 11, and fitted the same four models predicting participants’ syllable or bigram recall by sequence type, block or exposure phase, and SRT performance (as well as their interactions). Importantly, all previously observed effects remained significant, with most effects becoming even slightly larger than in the original models including all 12 blocks. The two models including the factor block showed the same overall outcome whether Block 12 was included or not. In the model predicting bigram recall by sequence type, phase, and SRT performance, the two interactions already observed in the other models now also reached the traditional significance threshold. These were the interactions between sequence type and SRT performance, indicating that children with a lower SRT score showed a higher recall difference between naturalistic and non-naturalistic sequences than children with a higher SRT score (β = −0.14 [−0.23, −0.03], t = −2.83, p = .005), and the interaction between exposure phase and SRT performance, indicating that children with a higher SRT score improved more in the early stages of the experiment than children with a lower SRT score (β = 0.09 [0.00, 0.18], t = 1.97, p = .049). Finally, an interaction between sequence type, phase, and SRT performance became significant at the syllable level, indicating that the recall difference between naturalistic and non-naturalistic sequences decreased for children with higher SRT scores as compared to children with lower SRT scores toward the end of the experiment (β = −0.05 [−0.10, 0.00], t = −2.02, p = .04). Figure 7 in the Appendix illustrates high-SRT and low-SRT children’s recall across the three exposure phases when Block 12 is removed.
Discussion
There is a growing body of evidence to suggest that SL is influenced by related prior knowledge – including phonotactic knowledge (e.g., Dal Ben et al., Reference Dal Ben, Souza and Hay2021; Finn & Hudson Kam, Reference Finn and Hudson Kam2008; Mersad & Nazzi, Reference Mersad and Nazzi2011; Onnis et al., Reference Onnis, Monaghan, Richmond and Chater2005), expectations about word length (Lew-Williams & Saffran, Reference Lew-Williams and Saffran2012), and knowledge of language structure (Thiessen et al., Reference Thiessen, Onnis, Hong and Lee2019). Since learners have been shown to form enduring memory representations for statistically defined input (see e.g., Batterink & Paller, Reference Batterink and Paller2017), it is conceivable that the influence of prior knowledge on SL also extends to prior knowledge of linguistic TPs (e.g., in the form of chunked syllables), obtained via exposure to natural language. There is promising early evidence for this possibility in adults (Elazar et al., Reference Elazar, Alhama, Bogaerts, Siegelman, Baus and Frost2022; Siegelman et al., Reference Siegelman, Bogaerts, Elazar, Arciuli and Frost2018; Stärk et al., Reference Stärk, Kidd and Frost2023); however, little is known about the way in which knowledge of syllable co-occurrences might influence SL in children. Here, we investigated whether seven- to nine-year-old German-speaking children used existing distributional knowledge to recall sequences of syllables and whether this was related to their language proficiency.
Children’s recall was significantly better for naturalistic sequences (i.e., those which complemented the distributional properties of their native language) than non-naturalistic sequences, in line with our experimental hypothesis. This echoes the findings of related work with adults (Elazar et al., Reference Elazar, Alhama, Bogaerts, Siegelman, Baus and Frost2022; Stärk et al., Reference Stärk, Kidd and Frost2023) and indicates that children too (in this case, seven- to nine-year-old native speakers of German) have well-entrenched memory traces of frequently co-occurring syllables, forged through experience with their native language and which shape their subsequent learning of related material (Siegelman et al., Reference Siegelman, Bogaerts, Elazar, Arciuli and Frost2018). Past research has demonstrated that both infants and adults draw upon prior experience with language structure to guide their use of forward versus backward TPs when processing new input (Onnis & Thiessen, Reference Onnis and Thiessen2013; Thiessen et al., Reference Thiessen, Onnis, Hong and Lee2019). The present study goes further by demonstrating that children use the precise TPs previously encountered in their native language to both recall and hierarchically group (or chunk) syllable sequences. That is, we demonstrated that children had acquired the syllable co-occurrence patterns in natural German and implicitly brought these to bear to process new linguistic input.
Children also showed better recall for non-naturalistic sequences over unstructured foil sequences at the bigram level, demonstrating once more how children readily acquire new words from input regularities (Evans et al., Reference Evans, Saffran and Robe-Torres2009; Kidd et al., Reference Kidd, Arciuli, Christiansen, Isbilen, Revius and Smithson2020; Saffran et al., Reference Saffran, Newport, Aslin, Tunick and Barrueco1997). The absence of the effect at the syllable level suggests that, while the children were sensitive to the in-experiment bigrams in the non-naturalistic condition, this advantage did not extend to a greater window as measured by the syllable recall measure. We suspect that the effect was not visible at the syllable level because of the difficulty of learning simultaneous sequences constructed from the same syllable inventory. Thus, with participants receiving sequences of all three sequence types simultaneously, the immediate advantage of the naturalistic sequences might have drawn participants’ attention to these patterns first, leading to a disadvantage for any other learnable pattern in the input, such as in the non-naturalistic sequences (cf. Antovich & Graf Estes, Reference Antovich and Graf Estes2018; Bulgarelli & Weiss, Reference Bulgarelli and Weiss2016; Gebhart et al., Reference Gebhart, Aslin and Newport2009).
Contrary to our expectations, at the group level, children’s recall did not improve over the course of the task. Thus, we did not replicate the pattern of within-experiment learning observed in adults (cf. Stärk et al., Reference Stärk, Kidd and Frost2023). There are two potential explanations for this result. First, this could be explained by an implicit learning advantage for children relative to adults. That is, that the effect emerged early and did not change thereafter could be because children rapidly identified (parts of) the patterned sequences and did not learn anything more thereafter. There is some recent evidence in support of children’s more rapid acquisition of statistical patterns in comparison to adults. Smalle et al. (Reference Smalle, Muylle, Szmalec and Duyck2017) showed that nine- to ten-year-old children implicitly acquire phonotactic restrictions on novel words faster than adults do. Additional work by Smalle et al. (Reference Smalle, Page, Duyck, Edwards and Szmalec2018) using the Hebbian repetition paradigm suggests that eight- to nine-year-olds show greater retention of implicitly learnt syllable sequences than adults four hours and one week after they were first tested. Thus, it appears that, in repetition learning tasks at least, children can learn faster and retain verbal information better than adults, general differences in cognition like working-memory span notwithstanding. This finding is consistent with the generally held belief that there is a critical period for language learning (Hartshorne et al., Reference Hartshorne, Tenenbaum and Pinker2018; Newport, Reference Newport1990). One tentative possibility is that the difference between our data and that of Stärk et al. (Reference Stärk, Kidd and Frost2023), where children seize upon the naturalistic sequences earlier, reflects children’s greater ability to identify distributional patterns.
While possible, we think this explanation might be less likely than a second explanation that appeals both to the complex nature of the task and to individual differences in children’s performance on the task. As outlined above, the fact that all three sequences came from the same syllable inventory introduced a complexity to the task that is not seen, for instance, in studies of Hebbian learning, which typically have patterned and foil sequences without syllable overlap, and which typically observe improvement in the repetition of the patterned sequence relative to foil repetition across time (e.g., Smalle et al., Reference Smalle, Page, Duyck, Edwards and Szmalec2018). This interference across sequence conditions may have prevented us from observing any interaction with block or phase, with the end result being that we only observed overall differences across the conditions.
Individual differences seem to have also impacted on learning across the experiment, with the children’s language proficiency, as measured by the SRT task, interacting with learning over the course of the experiment. We observed a significant correlation between the SRT and performance in the naturalistic condition, even after partialling out variance attributable to foil repetition, suggesting that higher language proficiency was associated with better performance in that condition. Intriguingly, in our statistical models, we found that language proficiency interacted with the overall magnitude of the difference between the naturalistic and non-naturalistic conditions: children with lower SRT scores had a greater difference in sequence recall than children with higher SRT scores. One possible explanation for this effect is that the magnitude of the difference was smaller in the more proficient speakers because, in addition to learning the transitions in the naturalistic condition, they were also building more robust knowledge of the non-naturalistic sequences than were the children with lower proficiency. This interpretation is consistent with the fact that the high-SRT children showed a decreasing trend in their recall of the naturalistic sequences relative to the non-naturalistic sequences in the latter part of the experiment, which emerged as an effect when we removed Block 12 from the analyses. If the children were also acquiring more robust knowledge of the non-naturalistic sequences during the latter half of the experiment, this newly acquired knowledge may have interfered with their performance in the naturalistic condition, given that the sequences contained the same syllables but different transitions. That is, the simultaneous learning of naturalistic and non-naturalistic sequences may have come at a price, with the acquisition of non-naturalistic sequences interfering with the naturalistic ones as their exposure to both sequences increased across time.
Our individual differences analyses revealed some suggestive patterns, but we stress that they should be interpreted with caution and treated as preliminary. Although our measures had good internal consistency (cf. Arnon, Reference Arnon2020), our sample size was not large and was further restricted due to circumstances outside of our control. Future studies could improve upon ours by recruiting a larger sample and by recruiting a larger age range. Language proficiency is correlated imperfectly with age, which may be due to the fact that the mechanisms underlying language acquisition vary across individuals in ways that are not completely age-dependent (Kidd & Donnelly, Reference Kidd and Donnelly2020; Kidd et al., Reference Kidd, Donnelly and Christiansen2018). Thus, more high-powered studies with wider age ranges may better elucidate the role of language proficiency in auditory SL of syllable sequences. At the moment, our data suggest that these individual differences exist and may be related to proficiency in nontrivial ways.
One final discussion point concerns the mechanism by which children are learning the syllable transitions in the task (and presumably, how they are learning the knowledge of syllable transitions they bring to the task). While the statistical structure of our sequences was defined in terms of TPs, we suspect that the process underlying learning is more likely to be the chunking of frequently co-occurring syllables into higher units – in our case, bisyllabic words – following models of SL and language that identify chunking as a basic learning mechanism (Christiansen & Chater, Reference Christiansen and Chater2016; Frank et al., Reference Frank, Goldwater, Griffiths and Tenenbaum2010; Perruchet, Reference Perruchet2019; Perruchet & Vinter, Reference Perruchet and Vinter1998). One benefit of conceiving of SL as chunking is that it builds natural bridges to related areas of the literature on verbal learning (e.g., Isbilen et al., Reference Isbilen, McCauley, Kidd and Christiansen2020; Jones, Reference Jones2012; Jones et al., Reference Jones, Gobet and Pine2007), thus grounding the field within the broader cognitive domain of learning and memory. Tasks like serial recall are well-suited to exploring the overlap in these literatures since recall is sensitive to long-term knowledge and learning effects (e.g., Kidd et al., Reference Kidd, Arciuli, Christiansen, Isbilen, Revius and Smithson2020; Smalle et al., Reference Smalle, Page, Duyck, Edwards and Szmalec2018; Szewczyk et al., Reference Szewczyk, Marecka, Chiat and Wodniecka2018).
Limitations and future directions
In the current study, we showed that seven- to nine-year-old children draw on their prior knowledge of syllable co-occurrences in their native language when processing new linguistic input, which has previously been found in adults (Elazar et al., Reference Elazar, Alhama, Bogaerts, Siegelman, Baus and Frost2022; Stärk et al., Reference Stärk, Kidd and Frost2023). We also found that children’s learning of attested and unattested syllable transitions was related to their language proficiency, as measured by sentence recall. The general conclusion from these data is that children form long-term representations for distributional information acquired over language development and use this knowledge to process new input. This skill may vary across individuals. We see these results as consistent with the general observation that language acquisition involves attending to and inducing abstract knowledge from regularly occurring sequences of linguistic units (e.g., Arnon, Reference Arnon2021; Bannard & Matthews, Reference Bannard and Matthews2008; Saffran et al., Reference Saffran, Aslin and Newport1996). However, we stress that we are not reducing acquisition purely to SL. From a very young age, children begin to build abstract knowledge at multiple levels of description. Thus, our demonstration that seven- to nine-year-olds are sensitive to distributional information does not entail that only this information is represented, but rather shows that this is one source of information that likely matters for learning. We note that almost every theory of language acquisition incorporates SL to some degree (e.g., Chang et al., Reference Chang, Dell and Bock2006; Lidz & Gagliardi, Reference Lidz and Gagliardi2015; Pearl, Reference Pearl2021; Tomasello, Reference Tomasello2003), but exactly how SL contributes in these theories differs substantially. An important future direction is accurately placing SL within the broader enterprise of language acquisition.
Conclusion
To conclude, we investigated the influence of children’s prior distributional knowledge on their auditory SL performance and examined whether this effect was related to the children’s language proficiency. Children drew upon their prior knowledge of TPs of their native language (i.e., highly frequent syllable co-occurrences) to process and learn new linguistic input, demonstrating that, like adults (Stärk et al., Reference Stärk, Kidd and Frost2023), German-speaking children had indeed developed entrenched knowledge of German syllable co-occurrences, which permeated through into the experiment to shape subsequent learning. In exploratory analyses, we found that children’s performance on the SL task interacted with their language proficiency, with the results suggesting that children with higher proficiency were more sensitive to both the naturalistic and non-naturalistic patterned sequences. This is consistent with the idea that there are meaningful individual differences in SL that are related to language acquisition (Kidd et al., Reference Kidd, Donnelly and Christiansen2018; Siegelman et al., Reference Siegelman, Bogaerts, Christiansen and Frost2017), although significant additional work in this space is required to determine the exact nature of the effect.
Appendix
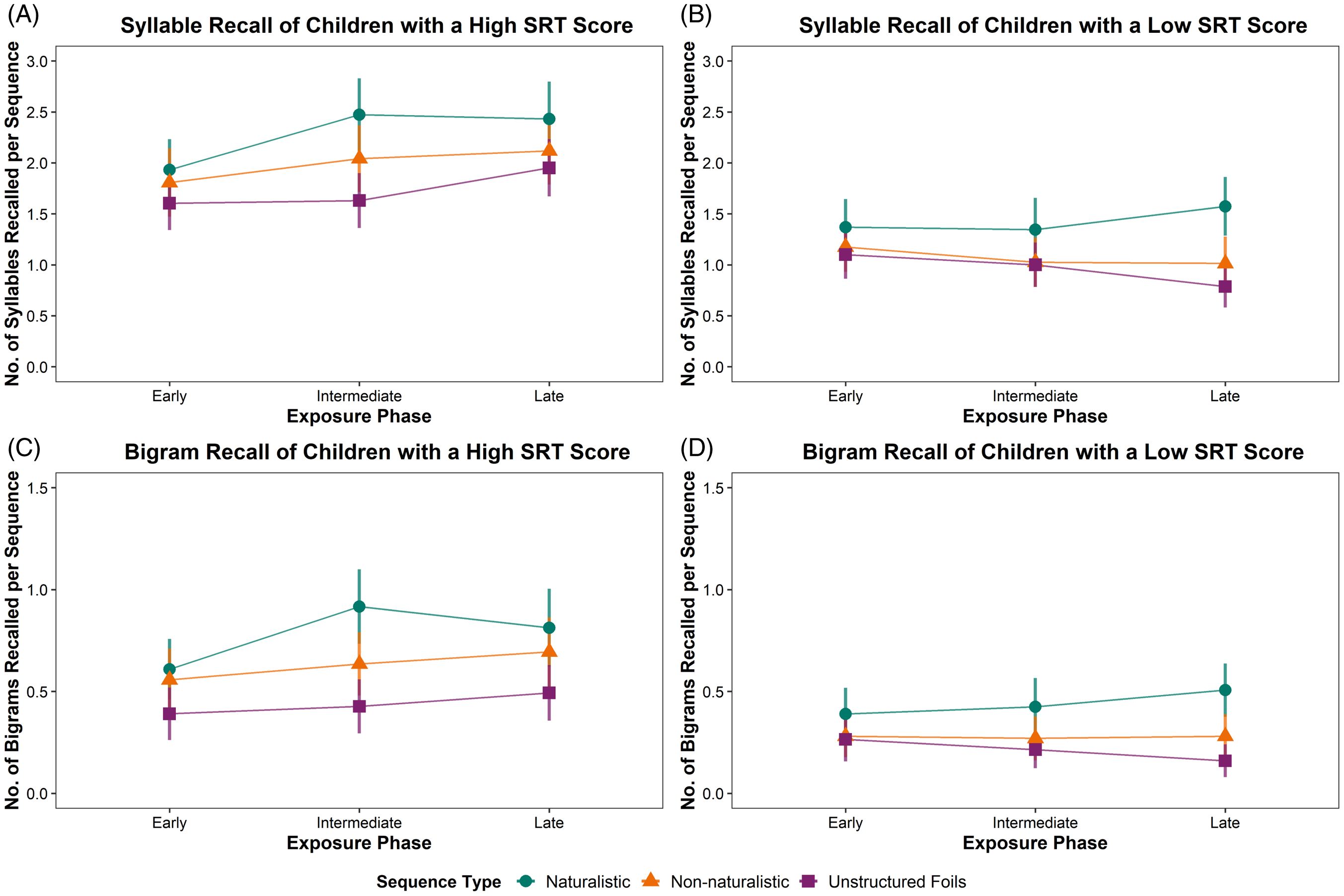
Figure 7. Mean syllable (top) and bigram (bottom) recall per sequence for the three sequence types (naturalistic, non-naturalistic, and unstructured foils) of the children with an SRT score higher than the median split (left) and lower than the median split (right), by exposure phase (early, intermediate, late) when removing the final block (late exposure phase = Blocks 9-11). Error bars indicate standard error.