1. Introduction
This paper considers progress in access to health care in South Africa after more than two decades of democracy. With the world’s highest level of income inequality, the country makes an important and informative case study.
The end of apartheid in 1994 put health care reform high on the country’s development agenda. Progressive policies promoting accessible and affordable primary health care, and substantial fiscal shifts over the past 20 years, are reforming the centralised, curative and hospital-based public health care system (Gilson and McIntyre, Reference Gilson and McIntyre2007; Black et al., Reference Black, Siebrits and Van der Merwe2011; Van Rensburg and Engelbrecht, Reference Van Rensburg and Engelbrecht2012). The government aimed to improve access to health care for the poorest and most marginalised by expanding the health care facility network and abolishing user fees for primary health care.
However, despite these efforts, health outcomes remain polarised, unequal and unfair (Heaton and Acheampong, Reference Heaton and Acheampong2007; Coovadia et al., Reference Coovadia, Jewkes, Barron, Sanders and McIntyre2009; Ataguba et al., Reference Ataguba, Akazili and McIntyre2011; Sahn, Reference Sahn2012; Ataguba et al., Reference Ataguba, Day and McIntyre2014; Marten et al., Reference Marten, McIntyre, Travassos, Shishkin, Longde, Reddy and Vega2014). Child and maternal mortality have decreased, but the improvements remain disappointing compared to those of peer countries.Footnote 1 The country is shouldering a heavy burden of disease,Footnote 2 with a disproportionate share borne by poor black households (Coovadia, Reference Coovadia2014).
In pursuit of universal health coverage, the recently released White Paper on National Health Insurance (NHI)Footnote 3 aims to build on these reforms to improve quality, coverage and equity, as a response to the poor performance of South Africa’s health care system, the persistent inequities and the vulnerability of subgroups. Considering the ongoing health reforms, quantifying access to health care is imperative. In lieu of a standardised monitoring and evaluation framework for measuring progress towards universal health coverage (Ataguba et al., Reference Ataguba, Day and McIntyre2014; Marten et al., Reference Marten, McIntyre, Travassos, Shishkin, Longde, Reddy and Vega2014), our study adds to the meagre quantitative literature describing access to health care in South Africa.
We investigate three interlinked but distinct dimensions of access: availability, affordability and acceptability, a framework based on Thiede et al.’s (Reference Thiede, Akweongo and McIntyre2007) proposal to collapse Penchansky and Thomas’s (Reference Penchansky and Thomas1981) five-dimension taxonomy (availability, accessibility, accommodation, affordability and acceptability) into three. We use data from the large 2009 and 2010 nationally representative General Household Surveys (GHSs) (n=190,164), which included a health care module with questions about reasons for bypassing the closest health facilities. We also address concerns about perception bias in the dimension of acceptability.
2. Context
The South African health care system is starkly divided between the public and private sectors. This reflects the country’s social divide: most beneficiaries of private health care services are affluent, skilled, educated and members of medical schemes (Marten et al., Reference Marten, McIntyre, Travassos, Shishkin, Longde, Reddy and Vega2014).
By 2001 the average spending on members of medical schemes was six times higher than that on uninsured individuals reliant on public health care services (McIntyre et al., Reference McIntyre, Doherty and Ataguba2012).Footnote 4 As would be expected, higher expenditure buys more medical expertise, specialised facilities, sophisticated technology, and advanced and expensive medication. It is therefore not surprising that Monitor Group’s (2008) international comparison of the quality of health care systems of 48 developing and developed countries found that the South African public health care sector ranked eighth from the bottom, just above Turkey and Columbia, while the private health care sector ranked sixth overall, on par with Switzerland and Sweden.
While the health care system remains inequitable and polarised, the post-apartheid government has made commendable progress towards reforming and improving the public sector and improving and consolidating access to it (Van Rensburg, Reference Van Rensburg2014), mainly by expanding the physical availability of public health care (Marten et al., Reference Marten, McIntyre, Travassos, Shishkin, Longde, Reddy and Vega2014). More than 1600 health care facilities have been built or upgraded since 1994, a significant investment affecting 40% of existing health facilities (South African Government News Agency, 2014). The availability of health care services has improved since the post-apartheid period, with Burger et al. (Reference Burger, Bredenkamp, Grobler and Van der Berg2012) finding that the poor report dramatically shorter travelling times to health care facilities (clinics).
Affordability-focussed policies in South Africa have eliminated user fees and expanded priority programmes with the aim of making health care more accessible for the most vulnerable groups (Van der Berg, Reference Van der Berg2002; Van Rensburg, Reference Van Rensburg2014). Nevertheless, research suggests that an exclusive focus on affordability will not necessarily improve health care access. Recent empirical studies by Brink and Koch (Reference Brink and Koch2015) and Koch (Reference Koch2017) found no evidence that post-1994 user-fee abolition increased the likelihood of vulnerable groups accessing public health care facilities. Likewise, Goudge et al. (Reference Goudge, Gilson, Russell, Gumede and Mills2009) found that fee abolition alone does not guarantee improved access to public health care, and that broader interventions are needed. Even if public health care services are ‘free’ or affordable, perceptions of poor quality may dissuade clients from using them. Honda et al. (Reference Honda, Ryan, Van Niekerk and McIntyre2015) argue that improvements in the availability and affordability of public health care in South Africa are unlikely to improve health outcomes if clients do not find the quality of the services acceptable. Policymakers need to understand clients’ experiences of accessing public health care services. Despite the importance of the acceptability dimension of access, the literature remains limited.
3. Methods
3.1 Study design
We took an empirical approach to measuring access, using Thiede et al.’s (Reference Thiede, Akweongo and McIntyre2007) access dimensions of availability, affordability and acceptability as our conceptual and interpretive framework. Within the parameters of the selected data set, we created the most suitable and comprehensive quantitative indicators of these access dimensions for use in the empirical analysis. We used univariate and bivariate analyses to describe the levels of access. Beyond the analysis of each dimension of access – availability, affordability and acceptability – we also considered overlaps in these dimensions, AA being an overlap between availability and affordability, and AAA an overlap between availability, affordability and acceptability. We used multivariate analysis to estimate the correlates of the three dimensions of access.
3.2 Data
We used nationally representative data from South Africa’s annual GHS, conducted by Statistics South Africa and publicly available. We chose to use the 2009 and 2010 GHSs because they included detailed questions about bypassing the closest health care facilities and asked patients about their health care provider, enabling us to capture the dimensions of access more accurately. Each of these surveys includes ~100,000 individuals, providing considerable statistical power for the analysis (Statistics South Africa, 2009, 2010).
For our analyses only respondents 18 or older were included. We chose this minimum age because certain survey questions were not applicable to individuals younger than 18 and responses from minors might be less reliable and could add noise. We used sampling weights to adjust the survey estimates for informal primary sampling units (PSUs), fast-growing PSUs, sample stabilisation and non-response.
3.3 Constructing access indicators
We constructed access indicators on the basis of Thiede et al.’s (Reference Thiede, Akweongo and McIntyre2007) three dimensions.
The availability indicator captures whether suitable health care facilities are provided in the right place at the right time to meet the principal needs of the population. This definition goes beyond issues of spatial or temporal access (distance or time to the nearest health care facility) to include the quantity and qualityFootnote 5 of services and the facilities’ opening hours. Affordability captures the cost of using health care facilities, the individual’s ability-to-pay, perception of value-for-money, understanding of health care prices, total costs (directFootnote 6 and indirectFootnote 7 ) and possible health care financing arrangements. The acceptability indicator is more normative. It captures clients’ attitudes to and expectations of the personal and professional characteristics of health care providers, such as attitudes and behaviour and client responsiveness, compared with the de facto characteristics. Together, these three access indicators capture the alignment between the health care system and the needs of individuals (McIntyre et al., Reference McIntyre, Thiede and Birch2009).
Thiede et al.’s (Reference Thiede, Akweongo and McIntyre2007) access dimensions provide an easily understandable framework for the empirical approximation of access. When translating a rich conceptual framework to an empirical estimate using survey data, a certain amount of reduction and simplification is unavoidable, but we contend nevertheless that the access indicators developed in this paper provide an informative and useful multi-dimensional overview of access.
We restricted our variables to those that are reliably captured in household surveys. Contrary to the traditional approach that often confounds ex ante and prospective opportunities with ex post and retrospective realised choices, and, similarly, access with utilisation, our approach considers opportunities available and not choices made. This is particularly important for a developing country like South Africa where comparisons with other countries’ utilisation rates may not be appropriate and could mask access problems.
Access broadly, but in particular acceptability, may be difficult to approximate empirically, especially if one is reliant on reported responses in a household survey with no opportunity to validate respondent perceptions. We are concerned about what Demery (Reference Demery2003) refers to as ‘perception bias’: the distortion of responses due to subjective factors such as perceptions, references and expectations. For instance, subjective views of illness and injury across income groups could bias reporting of illness and injury. The poor tend generally to have higher pain thresholds and higher opportunity costs for seeking health care (such as longer travel and waiting times), which can cause distortions in how individuals report illness or injury across the socioeconomic distribution (Rossouw et al., Reference Rossouw, Bago d’Uva and Van Doorslaer2017). Rossouw et al. (Reference Rossouw, Bago d’Uva and Van Doorslaer2017) suggest that poor South Africans may underestimate their health needs. There is thus a risk that their health care needs may be under-captured, since questions about access require comparisons across the income distribution. Similarly, when people report on the acceptability of health care facilities, their reference points and expectations matter. Burger et al. (Reference Burger, Ranchod, Rossouw and Smith2016) show that it is problematic to assume shared or comparable reference points and expectations for individuals across South Africa’s highly polarised society. Comparing self-reported satisfaction across the socioeconomic spectrum can lead us to underestimate the socioeconomic gradient. Consequently, in our analysis we took cognisance of perception bias when choosing data from which to construct the access indicators, and opted to use data derived from questions that were more likely to result in precise or, ideally, verifiable answers.
Our availability indicator was approximated using the travel time to the closest health care facility and information about the convenience of opening hours. The National Department of Health (2009) considers a distance of 5 km to primary health care facilities acceptable. On average, a slow 5-km walk (the most cost-effective mode of transport) would take 60 minutes. We set the bar at 30 minutes, ~2.5 km, as a more stringent test of availability. Respondents who reported travelling 30 minutes or more to their closest facility or who had experienced problems with opening hours at their most recent health care visit were classified as having barriers to the availability of health care. The resulting outcome variable was constructed as an availability dummy, with ‘1’ capturing availability and ‘0’ not.
The affordability indicator was designed to capture unavoidable out-of-pocket payments that added to the indirect cost of health care. This included bus, train and taxi fares to the closest public health care facilities, the three modes of transport most likely to be used by the lowest three quintiles (77% of quintiles 1–3 use these modes to get to health care facilities). It also included any payments for health care visits by the uninsured in rural areas. As we considered opportunities and not realised choices, we excluded payments for private health care providers in urban areas because we viewed these as a voluntary upgrade to access better quality care and services even though cost-free alternatives were available. Similarly, we considered only transport to the closest public health care facility and not to hospitals or private health care facilities. While bypassing a public health care facility is a worrying sign that people may consider the public health care system unacceptable, it is important to distinguish it from affordability constraints to avoid conceptual murkiness and double counting. We therefore captured any required and unavoidable payment as a restriction on affordability for the uninsured because it had the potential to exclude severely impoverished households or cause them suffering.
As our aim was to provide a general sense of affordability and because our concern was more with the poor than the affluent, we assumed that the provider payments incurred by a medical scheme member would be refunded largely or in full by the scheme. When viewed on an aggregate level, this indicator would provide accurate and valid information on fees and prices as a constraint to accessing health care. An alternative indicator could have been individuals who required care but did not consult a health care provider because they could not afford to. However, cases of this kind were very few (2% in the 2009 and 2010 GHSs). Questions about the prevalence of payments for basic public health care facilities appeared to produce more reliable and accurate data.Footnote 8 The resulting outcome variable was constructed as an affordability dummy, with affordable captured as 1, and unaffordable as 0.
To capture acceptability, we constructed an indicator based on bypassing the closest health care facility, which would be implicit evidence of preference. The GHSs asked respondents whether and why they bypassed their closest health care facility. If they said they bypassed it because it was dirty or staff were rude or a diagnosis was incorrect, or if they said they were dissatisfied at their most recent visit, these responses were interpreted as signs that the service was unacceptable.
Perception bias broadly means distortions in reporting because of subjectivity. Health studies frequently reveal differences in perception owing to differences in socioeconomic status (SES), and particularly underreporting of health needs and dissatisfaction with health services in responses by the poor. We used the concept of intersubjectivity to minimise the influence of perception bias by using the PSUs in the data set, which are small enough to make it plausible to assume that all respondents in a PSU would refer to the same facility when asked about their closest facility. Responses about bypassing were thus aggregated to create an acceptability score for each PSU. A health care facility was considered acceptable if more than 75% of individuals living in the PSU area found their last visit satisfactory or did not bypass the closest facility. Any aggregated score above 75% was then converted to 1 and those below 75% to 0. PSU acceptability scores were then assigned to all survey respondents within the PSU.
To test whether our acceptability measure was less sensitive to distortions correlated to per capita expenditure quintiles, we compared our measure (labelled ‘community-level acceptability scores’ in Figure 1) to two alternative measures: the raw individual satisfaction scores and the proportion of individuals who did not complain about staff being rude or uncaring. To ensure comparability and avoid confounding the influence of provider types with differences attributable to SES or the rural–urban divide, we concentrated on differences in acceptability scores by total household expenditure quintile and limited the sample to those who visited urban public health facilities.

Figure 1 Socioeconomic status slopes of three acceptability indicators, 2009–2010. Source: Own calculations using General Household Survey 2009/2010 data. Weighted by population.
Figure 1 shows a steep negative slope for complaints about staff attitudes, suggesting that the poorest respondents (quintile 1) are less likely to experience rude and uncaring staff than the middle-class respondents (quintile 4). Similarly, although not as marked, the slope for individual satisfaction shows the poorest quintile are more likely than quintile 4 to report having left an urban public health care facility satisfied. Although this does not conclusively prove that these counterintuitive results are attributable to perception bias, it is a plausible explanation and aligned to work by Burger et al. (Reference Burger, Ranchod, Rossouw and Smith2016) which used analysis with anchoring vignettes to confirm that there is perception bias amongst poorer households in South Africa and that it leads to underreporting of health care facility problems. The almost flat slope of the adjusted community-averaged acceptability scores for quintiles 1–4 supports our view that our measure reduces the influence of such bias.
3.4 Analysis
A linear probability model (LPM) was used for the multivariate analyses. An LPM regresses a dichotomised dependent variable on relevant explanatory variables by using the standard ordinary least squares method (Gujarati, Reference Gujarati2003). The LPM can be expressed as:

where Y is the dichotomised dependent variable and Xi represents a set of explanatory variables. Model 1 looks like a typical linear regression model except for the fact that the dependent variable is binary. It is called a LPM because the conditional expectation of Yi , given Xi, E (Yi |Xi), can be understood as the conditional probability that the event will occur, given Xi, i.e. Pr (Yi =1|Xi). While the LPM has its weaknesses, its simplicity and ease of interpretation outweighed its disadvantages in the context of our study, and the results obtained from LPM regressions did not differ significantly from those of Probit models, which we also ran. In the multivariate analyses, GHS data related to access were dichotomised and used as dependent variables. LPMs were estimated to show how proxies of the availability, affordability and acceptability dimensions of access are associated with sociodemographic, education, employment and household characteristics.
A SES index was created using principal component analysis (PCA) in lieu of adequate income and expenditure data in the GHS data set. PCA is used to combine highly correlated variables into a single factor, thereby expressing two or more variables in one index (Thurstone, Reference Thurstone1931). The SES index was constructed from a range of asset and expenditure variables including dwelling type, the household head’s level of education, access to water and type of sanitation system, and real household expenditure per capita (in August 2012 prices, using the mid-point method to derive household expenditure in each interval). The SES index was used to estimate population SES quintiles, a poverty indicator representing the bottom 40% of the SES distribution, and a dichotomous affluence indicator variable representing the top 20%.
In our regression model we include educational attainment and employment at both individual and household level. A dummy for the male gender was included, as well as continuous variables for age in years and age squared in years. The categorical variables for the four race groups (black, coloured, Indian and white) were included, with black being the reference (omitted) category. The averages for these covariates are reported in Table A1 in the Appendix. The regressions also included year and provincial dummies.
3.5 Limitations
One limitation is that the empirical analysis is cross-sectional and therefore coefficients are interpreted as partial correlations and not as causal relationships. Another is that our data are not the most recent but, as explained earlier, we chose them because the 2009 and 2010 GHSs included health care questions that enabled us to capture the dimensions of access more accurately.
4. Results
The largest deficiency in access was in the availability dimension, with 27% of individuals categorised as facing constraints (Figure 2). There was a large and statistically significant discrepancy in availability between the non-poor (83%) and the poor (59%). Approximately 85% of urban residents reported availability as opposed to 60% of rural residents. Availability by province ranged from 87% in the Western Cape and 81% in Gauteng to 65% in the Eastern Cape and 63% in Limpopo (Figure 3).

Figure 2 Available, affordable and acceptable access for poor and non-poor, 2009–2010. Source: Own calculations using General Household Survey 2009/2010 data. Weighted by population.
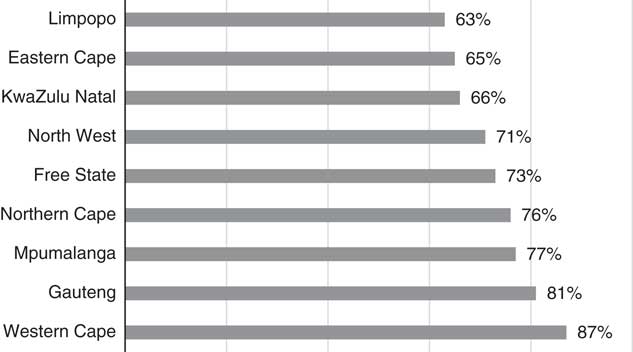
Figure 3 Availability by province, 2009–2010. Source: Own calculations using General Household Survey 2009/2010 data. Weighted by population.
Affordability was a constraint to health care for 23% of respondents (Figure 2). However, 73% of affordability constraints are due to travel costs which is aligned with findings of the availability constraints dimension. Again, there was a large and statistically significant discrepancy in affordability between the non-poor (84%) and the poor (65%), suggesting an anti-poor bias.
Acceptability levels were high at 90% for both poor and non-poor, thus showing no anti-poor bias (Figure 2). Given these high levels, we also analysed satisfaction scores. These were also high, with 80% of the population saying they were ‘very satisfied’ and ‘somewhat satisfied’ with the health care facilities. Table 1 shows high satisfaction scores despite some complaints about long waiting times, uncivil staff and medication not being available. For instance, almost 20% of the ‘very satisfied’ and 65% of the ‘somewhat satisfied’ individuals reported that they experienced long waiting times. This counterintuitive result may be explained by social desirability bias or the influence of low expectations and suggests that further work will be necessary to obtain consistent and comparable indicators of acceptability on both the conceptual and the survey side.
Table 1 Many health care users report being satisfied despite serious complaints, 2009–2010

Source: Own calculations using General Household Survey 2009/2010 data. Weighted by population.
An indicator was created to show the combined effect of the availability, affordability and acceptability variables, i.e., the percentage who had adequate access in all three dimensions. Defined this way, only 53% overall had adequate access to health care. Only 37% of the poor had access in all three dimensions (AAA), compared with 64% of the non-poor (Figure 4).

Figure 4 Availability compared with overlaps in access dimensions: available and affordable (AA) and available, affordable and acceptable (AAA), 2009–2010. Source: Own calculations using General Household Survey 2009/2010 data. Weighted by population.
Our LPM models (Table 2) considered equity concerns in more depth, allowing for heterogeneity in access. This further analysis was motivated by the concern that aggregated analysis may mask the subtler inequities that affect vulnerable subgroups. The results were robust to alternative variable definitions and other model specifications.
Table 2 Access models: availability, affordability, acceptability, available and affordable (AA); and available, affordable and acceptable (AAA), 2009–2010

Source: Own calculations using General Household Survey (GHS) 2009/2010 data. Weighted by population.
Note: Reference groups: Gender: Female, Race: Black.
GHS year dummies included but not shown in table.
Controlled for provinces.
***, **, *Significant at 1%, 5%, 10% level, respectively.
For availability, our LPM showed that being the most affluent, as well as being more educated and employed, were positively and statistically significantly related to the availability of health care. Age showed a convex relationship with availability. We found that black South Africans were statistically significantly less likely to report health services as available. The rural dummy showed a large, negative and statistically significant correlation with availability, meaning that a significant proportion of rural inhabitants continue to struggle with issues of long travel times to the nearest health care facilities or inconvenient operating hours.
The LPM for affordability showed that vulnerable subgroups of the population – black and rural South Africans – were statistically significantly less likely to report affordability of health care facilities. The most affluent and, to a lesser degree, more educated, were statistically significantly more likely to have affordable access to health care. Employment did not feature as a statistically significant correlate while age showed a concave and statistically significant relationship with affordability.
The LPM for acceptability showed that the most affluent were positively and statistically significantly related to acceptability. Education levels, employment status and age did not feature as statistically significant correlates. Again, black South Africans were statistically significantly less likely to report health services as acceptable than other race groups. Individuals living in rural areas were significantly more likely to report health services as acceptable. However, the most affluent were also statistically significantly more likely to report health services as acceptable.
The results for the combined indicator for access, AA and AAA, showed the same race and rural trends, with black South Africans and rural residents being least likely to report access (p<0.01). The anti-rural bias is supported by the provincial map of South Africa (Figure 5) which shows lower access rates in provinces with more rural inhabitants. More years of education, being employed and the most affluent were positively and statistically significantly correlated with access. Gender dynamics emerged in these models, showing an anti-female bias (p<0.05).

Figure 5 Provincial map of mean access rates (available, affordable and acceptable), 2009–2010. Source: Own calculations using General Household Survey 2009/2010 data. Weighted by population.
5. Discussion
Our findings suggest that, despite reforms intended to promote equal access to health care, many pockets of inequity remain. Acceptability levels are high, but availability and affordability remain prevalent constraints. The lower levels of availability and affordability imply that only 53% of the South Africans in our study had full access to health care, i.e., acceptable health care that is also available and affordable.
Our multivariate analysis shows that despite government efforts to make health care more accessible by expanding the facility network and abolishing user fees, rural households and vulnerable subgroups such as black South Africans, the less educated, the unemployed and the poor tend to be less likely to have adequate access to health care.Footnote 9
It is, however, imperative to understand the reasons for the affordability and availability constraints. Whereas there is clearly room for more reforms and greater vigilance regarding access constraints, our analysis shows that, in many cases, problems with affordability and availability are due to remoteness – the vulnerable groups have to travel further to the health facilities and pay more to do so. These problems are rooted in South Africa’s apartheid legacy of underdeveloped homelands and are therefore not simple to solve. The homelands system constrained migration to urban areas and promoted and legislated settlement in underdeveloped rural areas thus preventing more organic migration patterns coinciding with regional development dynamics. The high coefficient on the rural indicator in our study’s LPM provides further confirmation of the strong role of geography in constraining health care access. Adding to the geographical constraint is South Africa’s underdeveloped public transport network, whose deficiencies and shortcomings increase travel times and costs. Constraints of this kind fall outside the realm of the Department of Health.
A few qualifications should be borne in mind. We stress that the GHS question about health visitsFootnote 10 refers to a health care facility visit and thus access to services such as mobile health facilities could have been underreported, causing rural access to health care to be underestimated.
We also caution against taking the high acceptability levels at face value. Our proposed measure helped to reduce perception bias through small area aggregation and basing acceptability more on observed behaviour, i.e., bypassing of unsatisfactory facilities, than on perceptions. The positive coefficient on income provided some support for our belief that a fair share of the perception bias had been addressed with this proposed measure. However, this coefficient may represent a lower-bound estimate of the inequity in the acceptability of health care facilities.
Although we believe that we have eliminated some perception bias, our findings must be interpreted in conjunction with a critical awareness of the difficulties in measuring perceptions of acceptability on a coherent and comparable scale across South Africa’s very deep socioeconomic divide. Specifically, we are concerned about the positive and significant association we found between acceptability and rural communities and fear this may show that this acceptability indicator works well only in urban locations where we could reasonably assume that dissatisfaction would be reliably signalled through bypassing, because dissatisfied individuals would have more choice of health care facilities in their vicinity, and the cost of accessing a more distant facility would rarely be prohibitive. There is a pressing need to develop reliable and robust measures of acceptability that are less prone to perception bias.
6. Conclusion
As South Africa embarks on major health reforms under the umbrella of the NHI plan, there will be an increased need for empirical evidence to guide and inform policy. The paper is a contribution to this literature.
The evidence presented shows high levels of acceptability of health services, but lower levels of availability and affordability, especially for vulnerable subgroups such as the poor, the rural population and black South Africans. Further pro-poor health reforms are expected to contribute significantly to improving equity. However, as the problems are embedded in South Africa’s homelands legacy and exacerbated by deficient transport networks, innovative cross-sectoral solutions may be needed.
The acceptability of health care services was found to be high, but we are concerned about the shortcomings of using bypassing of facilities as a reliable indicator of acceptability when assessing rural households, which have fewer choices.
Further research is required to find a way to measure acceptability consistently and robustly. Despite the increased prominence of people-centred and client-responsive approaches on the global health agenda, the acceptability dimension of access and the associated client perspectives on health services remain inadequately understood. More research is needed to explain the dynamics underlying acceptability, especially given the difficulty of dealing with perception biases, caused by varying levels of expectations that could skew the estimates. Attempts should be made to anchor perceptions by incorporating a short set of vignettes in household surveys that researchers can use to stabilise reported perceptions across the socioeconomic spectrum.
The shortage of local research on user acceptability is part of a larger problem: concerns with supply-side constraints and affordability have long dominated policy debates in South Africa and overshadowed other equally pertinent issues linked to the demand side of health access, i.e., whether consumers find public health services acceptable or not (McIntyre et al., Reference McIntyre, Thiede and Birch2009). For instance, while the NHI White Paper published on 10 December 2015 talks about ensuring ‘a more responsive healthcare system … likely to improve use satisfaction, lead[ing] to a better quality of life … and improved health outcomes’, the bulk of the document is devoted to supply-side and affordability constraints. Although these deserve attention, it is myopic to neglect the acceptability dimension and we argue that such bias will limit the contribution and impact of the planned health reform over the long term.
Acknowledgements
This work is based on research supported in part by the National Research Foundation of South Africa (grant numbers: RCA13102556861 and CPRR150722129596. Academic support from ReSEP (Research on Socio-Economic Policy) is acknowledged. Comments from academics who attended presentations of this work at iHEA's (International Health Economics Association's) 11th World Congress 2015 and ESSA's (Economics Society of South Africa's) 2015 Biennial Conference are also appreciated.
Appendix
Table A1 Summary statistics, 2009–2010
