Social media summary: Hot-spot for learning ecological knowledge in middle childhood offers insights into childhood evolution.
1. Introduction
Humans have a distinct pattern to their life history, even compared with other apes. We require adult provisioning for a long period of time, which constitutes childhood (Bock & Sellen, Reference Bock and Sellen2002; Bogin, Reference Bogin1997; Leigh, Reference Leigh2002). The emergence of this phase in our lineage has been connected to other specifically human traits, including early weaning, short inter birth intervals, cooperative breeding and the presence of multiple dependent offspring in a family (Bogin, Reference Bogin1997; Kaplan, Reference Kaplan1996; Kramer, Reference Kramer2005, Reference Kramer2010, Reference Kramer2011; Kramer & Ellison, Reference Kramer and Ellison2010). It is theorised that this period is important for acquiring skills and knowledge, which are necessary to exploit our species’ complex niche (Kaplan, Bock, & Hooper, Reference Kaplan, Bock and Hooper2015; Kaplan, Hill, Lancaster, & Hurtado, Reference Kaplan, Hill, Lancaster and Hurtado2000; Robson & Kaplan, Reference Robson and Kaplan2003; Schuppli, Isler, & Van Schaik, Reference Schuppli, Isler and Van Schaik2012). The connection between life history, knowledge and the prevalence in our niche of nutrient-dense, difficult-to-acquire food resources has been extensively explored in Kaplan et al. (Reference Kaplan, Hill, Lancaster and Hurtado2000). Individuals who postponed reproduction in order to improve their understanding of the environment and increase their ability to extract its resources were selected through the benefits reaped in adulthood, when overproduction could support dependent offspring (Hill & Kaplan, Reference Hill and Kaplan1999).
Schuppli, Graber, Isler and van Schaik (Reference Schuppli, Graber, Isler and van Schaik2016) present a comparative analysis supporting this hypothesis, as they show that niche complexity correlates with adult provisioning and lengthy development in carnivora and primates, respectively. However, testing this hypothesis in our species is difficult. Previous research focused on describing age-specific patterns of foraging efficiency (Bird & Bliege Bird, Reference Bird, Bliege Bird, Hewlett and Lamb2005; Bliege Bird & Bird, Reference Bliege Bird and Bird2002; Blurton Jones & Marlowe, Reference Blurton Jones and Marlowe2002; Bock, Reference Bock2002; Crittenden, Conklin-Brittain, Zes, Schoeninger, & Marlowe, Reference Crittenden, Conklin-Brittain, Zes, Schoeninger and Marlowe2013; Gurven, Kaplan, & Gutierrez, Reference Gurven, Kaplan and Gutierrez2006; Koster et al., Reference Koster, McElreath, Hill, Yu, Shepard, Van Vliet and Ross2020) or knowledge (Koster, Bruno, & Burns, Reference Koster, Bruno and Burns2016; Schniter et al., Reference Schniter, Macfarlan, Garcia, Ruiz-Campos, Beltran, Bowen and Lerback2021). However, the detailed modelling approaches used in studies of somatic and cerebral growth (González-Forero, Faulwasser, & Lehmann, Reference González-Forero, Faulwasser and Lehmann2017; Kuzawa et al., Reference Kuzawa, Chugani, Grossman, Lipovich, Muzik, Hof and Lange2014) have not yet been extended to knowledge acquisition.
Human bodies grow slowly, but our brains grow quickly. A 10 year old child's brain has already reached its adult size, before the reproductive tissues start to develop and long before somatic growth is completed (see dashed lines in Figure 1, adapted from Bogin, Reference Bogin1997). Children's bodies grow slowest around the time when the brain is adult in size, but not yet completely adult in structure. What are the evolutionary origins of this pattern, and how does it relate to what the brain is doing at each age?

Figure 1. Growth curves for different body tissues in dashed grey lines, adapted from Bogin (Reference Bogin1997), with overlaying possible growth curves for knowledge, continuous lines. We could expect knowledge to follow the trajectory of the green curve, if learning rates depended exclusively on brain development, for example if learning was to begin only after the brain reached a certain volume, but could then proceed quite fast, in a ‘brain-threshold model’. On the contrary, if knowledge was a pre-requisite for reproduction, and could be acquired in a short period of time, it could simply increase before sexual maturity, as described by the purple curve, a ‘learn-to-reproduce’ hypothesis. Finally, the blue line shows a slow increase in knowledge with age, potentially continuing even after sexual maturity, which is consistent with the hypothesis that filling one's brain requires time.
Unfortunately, we have little precision over how knowledge and skill maps onto changes in brain and body structure. Does knowledge increase track cerebral growth (e.g. the green curve in Figure 1), as would be expected if the only condition necessary for the acquisition of knowledge was brain development, for example in a ‘brain-threshold’ model? Or is knowledge simply a prerequisite to reproduce (and acquirable in a short period), such that it increases just before individuals reach sexual maturity (purple curve, Figure 1), what we might dub the ‘learn-to-reproduce’ hypothesis? Previous studies report a slow, long period of knowledge acquisition, inconsistent with both aforementioned explanations, but as yet we do not have a clear understanding of how learning is patterned. Fast or innate behaviour acquisition is unlikely to be adaptive for a species like ours, which relies on highly specialised cultural adaptations to cope with environment-specific challenges. On the contrary, the slow development observed in previous studies could be the result of a combination of cognitive development and exposure to context-specific experiences as well as other factors, in some sort of ‘brain-filling’ hypothesis. Yet how knowledge acquisition correlates with age is not fully understood, and ascertaining its shape can give insights into its role for the evolution of childhood.
In this paper, we focus on the acquisition of ecological knowledge because of its potential association with fitness, for example through increased returns when foraging (Koster et al., Reference Koster, Bruno and Burns2016; Reyes-Garcia et al., Reference Reyes-Garcia, Guze, Diaz-Reviriego, Duda, Fernandez-Llamazares, Gallois and Pyhälä2016) or health benefits (McDade et al., Reference McDade, Reyes-García, Blackinton, Tanner, Huanca and Leonard2007; Reyes-García et al., Reference Reyes-García, McDade, Vadez, Huanca, Leonard, Tanner and Godoy2008). We make three specific contributions to our understanding of how knowledge is acquired.
First, we develop a novel analysis of ecological knowledge using item response theory (IRT) (Osteen, Reference Osteen2010). We model knowledge as a latent variable measured with several independent survey tools. Item response theory refers to a family of statistical models whose structure allows us to measure individual differences in ability and gender-specific patterns of learning, and assess the reliability of survey questions across subjects.
Second, we describe in detail how ecological knowledge varies with age and sex. Concerning age, we focus on a sample ranging from 5 to 26 years, and we allow the relational function between age and knowledge to emerge from the data. This is in contrast to previous studies, which impose linear or logistic functions and focus on adults (Bortolotto, de Mello Amorozo, Neto, Oldeland, & Damasceno-Junior (Reference Bortolotto, de Mello Amorozo, Neto, Oldeland and Damasceno-Junior2015), 21–86 year olds, Geng, Zhang, Ranjitkar, Huai, & Wang (Reference Geng, Zhang, Ranjitkar, Huai and Wang2016), 21–91 year olds; Koster et al. (Reference Koster, Bruno and Burns2016), 10–80 year olds, with few individuals below 15; Schniter et al. (Reference Schniter, Macfarlan, Garcia, Ruiz-Campos, Beltran, Bowen and Lerback2021), 5–86 years, only seven individuals below 30), or divide children into large age categories (Cruz-garcia et al. (Reference Cruz-Garcia, Caffi, Elena, Zans and Sanchez-choy2018), children 7–14 years old divided in two categories; Quinlan, Quinlan, Council, & Roulette (Reference Quinlan, Quinlan, Council and Roulette2016), children from 4 to 17 years divided into three categories; Gallois, Duda, and Reyes-García (Reference Gallois, Duda and Reyes-García2017), divides only between children and adults). This allows us to explore with more precision the relation between age and ecological knowledge in the most important phases of our development, in an attempt to understand how knowledge acquisition maps onto other life history traits. Following the assumption that labour division along gender lines entails knowledge specialisations, we also investigate sex differences in ecological knowledge. Previous studies provide contrasting results on whether boys and girls differ in their levels of ecological knowledge (Blacutt-Rivero, Moraes R., Gruca, & Balslev, Reference Blacutt-Rivero, Moraes, Gruca and Balslev2016; Cruz-garcia et al., Reference Cruz-Garcia, Caffi, Elena, Zans and Sanchez-choy2018; Gallois et al., Reference Gallois, Duda and Reyes-García2017; Geng et al., Reference Geng, Zhang, Ranjitkar, Huai and Wang2016; Schniter et al., Reference Schniter, Macfarlan, Garcia, Ruiz-Campos, Beltran, Bowen and Lerback2021; Setalaphruk & Price, Reference Setalaphruk and Price2007). Here we find evidence of gender-specific dimensions of ecological knowledge, rather than differences in overall levels of knowledge.
Third, we supplement our description of variation in the pattern of ecological knowledge acquisition with a causal analysis of the factors influencing this variation. We focus on three specific factors: first, exposure and practice (gendered-activities effects); second, access to adult experts or peers (household and family composition effects); and third, opportunities for learning (formal education effects). These were chosen because they were expected to represent opportunities for learning through practice or exposure to models of behaviour in the particular ethnographic context. We show how gendered differences in ecological knowledge are related to sex-specific engagement with harvesting in different ecological niches. This is consistent with expectations that such differences are not necessarily innate. Household composition is leveraged to identify how adult experts or peers (Boyette, Reference Boyette2013; Lew-Levy, Reckin, Lavi, Cristóbal-Azkarate, & Ellis-Davies, Reference Lew-Levy, Reckin, Lavi, Cristóbal-Azkarate and Ellis-Davies2017; Ohmagari & Berkes, Reference Ohmagari and Berkes1997; Page et al., Reference Page, Emmott, Dyble, Smith, Chaudhary, Viguier and Migliano2021; Ruddle, Reference Ruddle and Inglis1993), in addition to parents (Hewlett & Cavalli-Sforza, Reference Hewlett and Cavalli-Sforza1986; Lozada, Ladio, & Weigandt, Reference Lozada, Ladio and Weigandt2006), might influence the acquisition of knowledge about the local environment. Similarly, we examine the effects on knowledge acquisition of birth order (Quinlan et al., Reference Quinlan, Quinlan, Council and Roulette2016), to determine whether late-borns gain more access to older sibling role models and/or early-borns enjoy preferential access to parental and grandparental wisdom. Finally, participation in formal education can lower exposure to locally relevant ecological knowledge, as time invested in school attendance could reduce opportunities for acquiring information about the environment (Quinlan et al., Reference Quinlan, Quinlan, Council and Roulette2016; Reyes-García et al., Reference Reyes-García, Kightley, Ruiz-Mallén, Fuentes-Peláez, Demps, Huanca and Martínez-Rodríguez2010).
2. Materials and methods
2.1. Research location
The data used for this study were collected in Pemba, the northern island of the Zanzibar archipelago, a semi-autonomous zone of Tanzania. The study was performed in and around a village located in proximity to the forest of Ngezi, in the shehia (administrative unit) of Kifundi (see Figure 2). Pemba is part of the Swahili world, with multiple historical and cultural influences: first the Bantu expansion from the mainland, then 700 years of trade in the Indian ocean, and later Portuguese, Omani and British colonial power (Fleisher et al., Reference Fleisher, Lane, Laviolette, Horton, Pollard, Quintana Morales and Wynne-Jones2015; LaViolette & Fleisher, Reference LaViolette and Fleisher2009). Especially important has been the influence of the Arab world and Islam, since at least the eleventh century and then during the rule of the Omani Sultanate, which was weakened by the British at the end of the nineteenth century, but formally deposed only in 1963 (Fleisher & La Violette, Reference Fleisher and La Violette2013).
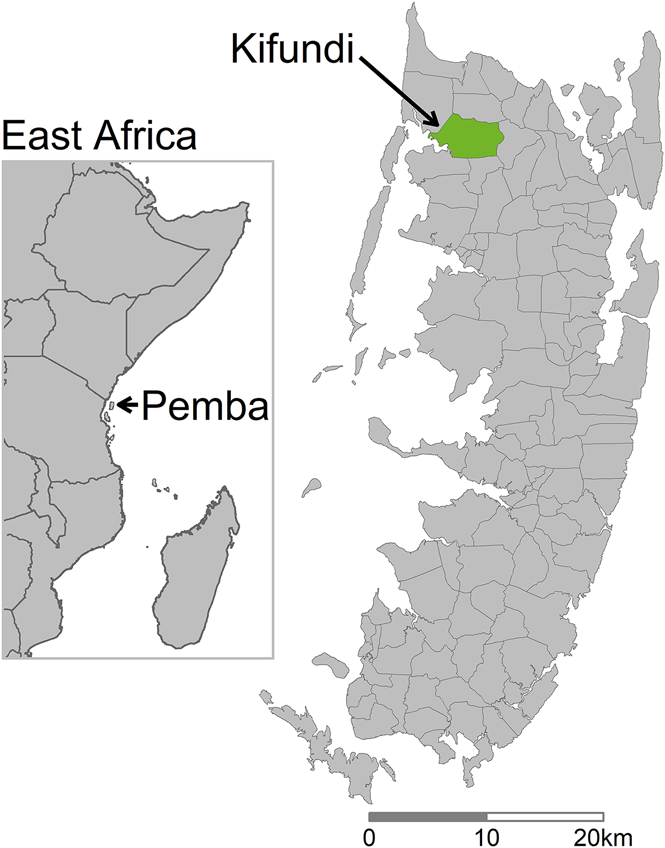
Figure 2. Pemba lies offshore Tanzania. Data were collected in the shehia of Kifundi, coloured in green, which is still partially covered by the forest of Ngezi.
The island, located just below the equator (5°S), enjoys a tropical climate and mild seasonality, with an alternation between drier and rainier seasons following the monsoon cycle. Its densely distributed population (428/km2, about twice that of Switzerland) mostly lives in rural areas (around 80%, according to the National Bureau of Statistics Ministry of Finance, Statistician, Ministry of State President's Office, & Good Governance, 2014) and depends largely on agriculture, fishing and other forest-based activities (Andrews & Borgerhoff Mulder, Reference Andrews and Borgerhoff Mulder2022; Zahor Zahor, Reference Zahor2021).
Located on the northwest of the island, the forest of Ngezi is the largest remaining patch of rainforest in Pemba, and the people living around it depend on terrestrial and maritime habitats for more than 80% of their livelihoods, with the remaining proportion derived from commercial activities and paid labour. Most of the income of the typical household in the area around Ngezi comes either from fishing (9.7%), agriculture (farming 20.6%, livestock 3.9%, agroforestry 4.2%, 11.9% clove production, the main cash crop on the island) or the forest itself (forest products, mainly firewood, for 33% of total income for the average family in the area around Ngezi forest, according to Andrews and Borgerhoff Mulder, Reference Andrews and Borgerhoff Mulder2022). Hunting is relatively rare among the adult population, in large part because of the scarce wildlife of reasonable size, but still present in many areas of the island (Walsh, Reference Walsh2007).
Children comprise a large part of the growing population of Pemba, where 48% of inhabitants are below 15 years of age (National Bureau of Statistics Ministry of Finance et al., 2014). Young people divide their time between domestic and farming chores, education at both the state and Koranic schools and play. There is variability in the extent to which children and teenagers interact with the natural environment, mainly when they forage on the shore or in the forest, depending on personal inclination and their familial or environmental conditions.
2.2. Data collection
Village census
With government approval for research (IMMZ/07/17/25, ethical approval provided also by Max Planck Institute Ethics Council, application number 2019_05), IP visited all households in a village in the area surrounding the forest of Ngezi between June and August 2019. The objective of this survey was to record a full village census with relevant household-level characteristics, so that background variables on subjects (opportunistically sampled, see below) were known. The survey also provided the opportunity to introduce the project to the full community, and to obtain approval for future interviews with the children.
Knowledge interviews
Using an opportunistic sampling procedure – effectively children and young adults volunteering for interview – IP conducted a survey instrument measuring ecological knowledge of the natural environment (as defined in the Supplementary Information, section 9.1). Opportunistic sampling might have introduced some bias in our results, but these are qualitatively comparable with those originating from a smaller, unbiased sample (see the Supplementary Information, section 9.2). In sum, 93 individuals between 5 and 26 years of age participated in the knowledge interviews. These consisted of three different types of questions, namely: (a) freelisting questions (Quinlan, Reference Quinlan2005), in which children were asked to name all the living creatures (viumbe, which include plants, different kinds of animals and also spirits that are thought to inhabit nature) found in the village/cultivated fields, in the forest or in the sea (the named items were then checked for misspelling, and then again with local and international collaborators to ensure appropriate identification of the answered items); (b) a list of 50 multiple choice quiz questions probing different aspects of ecological knowledge, each with two options (developed with the help of both the Department of Forestry and Non Renewable Natural Resources and focus groups with adults in the village); and (c) a task of image recognition using pictures collected around the chosen village or elsewhere in Pemba, in which children were asked to name all the 71 living beings appearing in a sequence of 27 photographs. Answers given to each picture were rated as acceptable or non-acceptable during a focus group with adults in the village following data collection. Table 1 reports sample sizes by age groups and a summary of answers. More details on the interviews and data can be found in the Supplementary Information, section 9.4.
Table 1. Sample size by decade of age, as well as best result by decade and type of question

2.3. Causal framework
Data collection and analysis were informed by preliminary work aimed at describing the causal and non-causal factors influencing the association between age and ecological knowledge. Specifically, we described all of the aspects of individuals that are expected to influence ecological knowledge in a direct acyclic graph (DAG) and later in a full structural causal model that we used to validate the analysis code (see Figure 3 and the Supplementary Information, section 9.5). A DAG is a heuristic generative model that allows the derivation of a causal estimand (Pearl, Reference Pearl1995). Each causal query may require a different statistical procedure that includes different variables.

Figure 3. Direct acyclic graph describing relationships between analysed variables. Note that age does not ‘cause’ knowledge directly. Rather, the effect of age is mediated by other variables, including activities practised and school attendance, but also other unmeasured events and developmental changes. In the models, as we introduce activities or schooling, age remains as a proxy for these unmeasured factors, represented by the circled U in the graph. Moreover, we do not assume a direct effect of sex on knowledge, as we do not expect innate differences between sexes in knowledge acquisition. On the contrary, the sexes differ in the frequency with which they engage in activities, although we do not discuss whether this has an innate or cultural origin.
We relied on the DAG described in Figure 3 to plan data collection, so that all of the variables would be recorded, as well as to define the analyses described below. The analysis can be divided in a descriptive and a causal section. The first illustrates how ecological knowledge changes with age; the causal section, instead, uses different models to address causal queries concerning the effect of sex, activities, family and schooling on the development of knowledge.
Of course it is possible that unobserved confounds exist along any edge in our DAG. We encourage the perspective that any estimated effects are possibly partially due to such confounding. This is true in all observational, anthropological investigations. The additional transparency of our approach, and its ability to logically derive statistical procedures from causal assumptions, is its strength.
2.4. Analysis
We developed multiple Bayesian models targeting our different inference goals, each composed of two different parts. The first part of the models is an IRT model. IRT models, initially developed as a mean to grade tests in pedagogy (Osteen, Reference Osteen2010), aim at estimating one or multiple latent traits, such as individual ecological knowledge (K i), from answers to questions while simultaneously allowing these questions to vary in difficulty (b j) and discrimination (a j, i.e. how helpful they are at discriminating between high- and low-knowledge individuals). For each question j, an individual i has a probability p i,j of answering correctly (Y i,j = 1, see equations 1 and 2); that is, naming a specific item in the freelist, answering correctly an item in the quiz or recognising an item in the image recognition task. The quiz questions differ from the other two types because the correct answer can be given simply by guessing, and thus requires a 3PL IRT model, which includes a pseudo-guessing parameter c j (Osteen, Reference Osteen2010). All three types of data contribute to the estimation of one single measure of ecological knowledge for each individual (see the Supplementary Information, section 9.6 for an extended description of the model's functioning and implications).


The ecological knowledge K i in the IRT part of the models is simultaneously used as a dependent variable in the second part of the models, which estimates the effect of various factors on individual ecological knowledge. This second section varies according to the inference goal of each model (see below equations 3–5, and equations 1 and 2 in the Supporting Information section 9.6). All models include a global intercept ω and non-centred individual random effects α i, with σ 1 being the standard deviation parameter. Age is also included in all model variants as an ordered categorical variable, so that there is a sex-specific maximum effect for age β s (for sex s) and each year adds a proportion δ y of the total effect. This means that, while we impose monotonicity on the function relating age to ecological knowledge (knowledge never decreases with age, at least in the observed range), the curve of the relation between knowledge and age emerges from the data themselves, rather than being imposed to be a specific function in the model design. Model 1, which provides a description of age-related changes in ecological knowledge and for which the second section is described by equation (3), produces sex- and age-specific estimates, and can hence tell us the total effect of age and sex combined.

Note that this model has been extended to allow different dimensions of ecological knowledge to emerge from the data (becoming a compensatory Multidimensional IRT model (Reckase, Reference Reckase2009), see the Supplementary Information, equations 3 and 4, and the Supplementary Information, section 9.8). This is aimed at determining whether the individuals in our sample are experts in certain aspects of the environment, while having a lower knowledge level in others; in other words if there is knowledge specialisation. The probability individual i has of answering a single question j correctly is the sum of multiple probabilities for each dimension d, and individuals are assigned a measure of ecological knowledge in each dimension K i,d. This approach allows us to partition the variation among knowledge of individuals in any number of dimensions.
The results of Model 1, both in the unidimensional and multidimensional versions, are descriptive. The relationship between age and ecological knowledge is not really a causal relationship, because any association between age and knowledge is presumably not result of mere calendar age, but instead of accumulated experience and instruction, which cannot be measured and for which age is a proxy.
In contrast, the models described below aim instead at estimating the causal effect of the other factors included in the DAG in Figure 3. In order, Model 2 (for which equation 4 shows the list of estimated parameters) also includes household-level varying random effects η h (σ 2 is the standard deviation parameter for household level random effects) and the effects of each of the 10 possible activities γ act on individual ecological knowledge (see the caption of Figure 6 and the Supplementary Information, section 9.3.2 for a description of these activities). Model 3 (equation 5) estimates the effect of co-residing with either parent for individuals of both sexes (ψ s is the sex specific effect of being in the same household with mothers, ϕ s with fathers). Further models allow estimation of the effect of other factors such as schooling and birth order, described in the Supplementary Information, section 9.6.


We determined priors for all models through prior predictive simulation. The resulting priors are weakly informative, specifying low probability for impossible outcomes and inducing regularised estimates, but allowing a very wide range of results (see the Supplementary Information, Figures S8 and S10). The priors used for the model are:

The prior for a, a normal distribution limited to positive values, ensures that more knowledgeable individuals have higher values than less knowledgeable ones. The slightly less constrained prior for b allows the axis of the latent knowledge to expand to accommodate all the values for each question (to see what happens when changing the priors for b, see Figure S9). Note that the individual ecological knowledge measures K i do not require a prior distribution, but are informed by the combination of the priors for a and b. For the parameters α i, η h, $\sigma _\alpha$, $\sigma _\eta$
, δ y, γ act, ψ s, and ϕ s weakly informative regularising priors have been used. The priors for the parameters ω and β s, instead, have been chosen to allow the function to expand in the negative space where the values of ecological knowledge are placed by the IRT model.
We estimated the posterior distribution for each model using the Hamiltonian Monte Carlo engine Stan (Stan Development Team, 2021b), specifically CmdStan (Stan Development Team, 2021a). Posterior distributions were processed in R 4.1.0 (R Core Team, 2021), with the help of the Statistical Rethinking R package, version 2.13 (McElreath, Reference McElreath2020). For all analyses, visual inspection of traceplots, Gelman-Rubin diagnostic and effective number of samples indicate model convergence.
Parameter estimation in the Bayesian framework does not return point estimates, but rather a posterior distribution of possible values for a parameter. The figures in the result section reflect this feature, e.g. each line in Figure 4 is draw by a set of values sampled from the posterior distribution of parameters. Finally, prior to fitting to real data, all models have been tested with simulated data, as described in the Supplementary Information, section 9.7. This allowed to ensure that the models could recover ‘real’ parameter values. Simulation code as well as all the other code necessary to reproduce these analyses is available on GitHub (https://github.com/lalla-ilaria/Children_eco_knowledge).

Figure 4. The points in the figure represent individual ecological knowledge K i as measured by the item response theory model, colour coded for sex. The average knowledge for an individual of a certain age and sex, as predicted by model 1 in equation (3), is represented by the continuous lines, where the darker one is the mean of the distribution and the lighter lines represent 150 draws from the posterior.
2.5. Results
2.5.1. Descriptive results
In agreement with previous studies, ecological knowledge in our model increases along all sampled ages. The lines in Figure 4 show the estimated increase in ecological knowledge per each year of age, plotted over the dots representing individual measures of ecological knowledge resulting from the IRT part of the model. Ecological knowledge increases quite steeply during early and middle childhood, keeps increasing during most of adolescence and then appears to start leveling off as individuals approach maturity. Age-specific effects, shown in Figure S7, are largest for ages 7 and 11, suggesting that the time around these years has higher importance for ecological knowledge than, for example, ages 20-25 (see the Supplementary Information, section 9.3.1 for age estimation methods).
Sex differences in ecological knowledge, as identified from the one dimensional model, are clearly visible in Figure 4 (see Table S6 for calculated contrasts between sexes, i.e. differences between posterior estimates of sex-specific age-effects). This difference appears during the juvenile period, between 7 and 12 years, right when a division of labour begins to emerge, and results in higher undifferentiated ecological knowledge among the oldest boys in our sample when compared with the oldest girls. But this one dimensional description of knowledge does not tell the whole story. When we partition ecological knowledge using the method described in section 2.4, three main dimensions emerge (Figure 5). In the first, both sexes acquire ecological knowledge at a similar rate, with girls achieving higher final values, plausibly representing general or slightly female-biased dimension of ecological knowledge. In the second, there is a sharp increase in ecological knowledge among boys, and almost no age effect among girls, which probably reflects a male-specific dimension of knowledge. Finally a third dimension captures remaining variation, which contains no strong sex differences (again, see Table S6 for contrasts). Although our analysis was not developed with the scope of analysing differences between question items, an inspection of the question-specific parameters can help interpret this dimensionality. In particular, a sex-specific pattern emerges when looking at difficulty parameters b j for the freelist items. For example, the ten easier items (i.e. with lower b j values) in the first, general dimension include mainly farmyard animals such as chicken and goats. The most salient items in the dimension where only males learn are mainly fish and wild birds, which are more relevant for the pursuits of boys. the Supplementary Information, section 9.8 explains why we think three dimensions are the best description of the data.

Figure 5. Individual ecological knowledge K i and predicted values by age and sex in three dimensions.
2.5.2. Causal results
We attempted to estimate the causal influence of activities, household family structure, and school attendance on ecological knowledge. In each case, we try to control for unmeasured household-level variables, using random effects. This is because any correlation caused by some social characteristic associated to specific households can induce spurious correlations between the outcome variable, Knowledge, and the other variables that stay in the path of Family (see DAG in Figure 3). The posterior estimates for these random effects suggest that not much knowledge variation is due to differences between the families (see the Supplementary Information, section 9.9). However, the models are not designed to estimate the causal influence of unmeasured household variables, so these estimates cannot be interpreted causally, as is the norm with control variables (Westreich & Greenland, Reference Westreich and Greenland2013).
The first variable we considered is practice, specifically the effect of the activities practised by children (defined combining reports by parents and the children themselves, see the Supplementary Information, section 9.3.2 for details). We find a substantial effect of activity on ecological knowledge. Figure 6 plots how much advantage, in terms of time, an individual can gain (positive effect) or lose (negative effect) from practising a specific activity. All else being equal, a person who hunts birds can reach the same ecological knowledge of a non-hunting 20 year old up to 10 years earlier. Similarly positive effects are given by collecting shellfish, fishing and by tending to the family's livestock. On the contrary, working in the fields can hinder ecological knowledge acquisition by more than a decade, as can attending to household chores. Activities practised account for a majority of the measured difference between boys and girls, so that, once their effect is controlled for, the difference in ecological knowledge between sexes seems to disappear (see Figure S16 and refer to last row of Table S6 for a measure of the contrasts between the sexes). Some portion of the measured association between activities and ecological knowledge is plausibly causal, but note that there may be unmeasured common causes of both activity and knowledge, as well as some reverse causation, if ecological knowledge is a necessary precondition for some activities. Therefore we suspect these activity effects are upper bound estimates.

Figure 6. The plot describes how much advantage, in terms of time, an individual can gain from practising an activity with respect to undifferentiated ecological knowledge. The x-axis represents the years gained, i.e. how much earlier (positive values) or later (negative ones) an individual practising each activity would reach the same ecological knowledge of a 20-year-old individual not practising that activity, all else kept constant. Activities such as shellfish collection and bird hunting could give up to 10 years’ advantage in terms of ecological knowledge to practising individuals – although most people would gain two to five years. On the contrary, doing agricultural work (bottom row) seems to slow down learning. Notice that the tail of the distributions for the bottom five activities extends outside of the limit of the plot, with the fifth or smaller percentile below −20. Activities description: seashells – extracting seashells from a sandy bottom; livestock – herding and watering cattle or goats; birds – hunting birds with various traps or slingshots; fishing – fishing using small-to-medium-sized boats, often in small groups; game – hunting larger game with the help of dogs, often targeting crop predators such as monkeys; algae – farming seaweed to sell; diving – advanced fishing, with masks and sometimes air tanks; cloves – paid labour in picking clove buds from trees; household – chores such as water collecting or cooking; agriculture – participating on work in family fields.
We present the results of household family structure and school attendance in the Supplementary Information, section 9.9. Co-residence of parents (Figure S18) appears to have an effect on individual's ecological knowledge. Mothers seem to boost their children's knowledge, helping to reach earlier, on average, the same ecological knowledge of a 20 years old with no parents. The presence of fathers, on the contrary, has different effect on his sons and daughters, improving the knowledge of the former, but impeding learning about the environment for the latter. Schooling seems to at least partially slow down learning for girls, but has no effect on boys (Figure S20). Birth order has no appreciable effect on ecological knowledge (see Figure S19 and the Supplementary Information, section 9.9 for more information).
3. Discussion
The observation that people in this sample learn during the whole pre-reproductive period complements previous work showing increases in ecological knowledge during adolescence and adulthood (e.g. Koster et al., Reference Koster, Bruno and Burns2016; Schniter et al., Reference Schniter, Macfarlan, Garcia, Ruiz-Campos, Beltran, Bowen and Lerback2021). Additionally, we show that the rate of acquisition is not constant, and that there are age ‘hot-spots’ for learning. Knowledge acquisition appears in our data to be fastest during middle childhood, between 7 and 12 years, after which its speed decreases. As expected, this pattern is inconsistent with the fictionalised ‘brain-threshold’ or ‘learn-to-reproduce’ models, and consistent with the slow ‘brain-filling’ hypothesis. More novel is our use of causal modelling to explore the role of activities in shaping sex differences in ecological knowledge. We find that higher ecological knowledge is associated with activities like foraging, hunting and fishing, consistent with the general view that practice is important to the acquisition of ecological information. Moreover, we provide general support for the idea that early life history is shaped by the need to learn.
Most hypotheses describing the importance of knowledge for life history evolution propose that childhood emerges in the Homo lineage as a time to complete full growth of the brain during early childhood, after which the brain has to be filled with information during middle childhood (Thompson & Nelson, Reference Thompson and Nelson2011). Our results are congruent with this view: after the brain reaches its full size, ecological knowledge acquisition speeds up, maintaining a fast rate during the middle childhood years. Perhaps not incidentally, this period is marked by a slowdown in somatic growth, which reduces the energetic requirements of children while they start to engage in productive activities and acquire the required ecological knowledge. Adult provisioning and protection are needed up to at least the beginning of adolescence, but the first attempts at performing most activities happen well before 12 years, and allow children to have a buffer zone in their life history in which to try out various tasks with the back-up offered by parents and other caretakers (Lew-Levy & Boyette, Reference Lew-Levy and Boyette2018). During adolescence, learning is slower, although it keeps going until after sexual maturity, potentially providing opportunities for specialisation.
Differences in ecological knowledge between the sexes appear by age 7 and become more prominent during adolescence. This is consistent with studies showing the emergence, around this age, of inter-sex differences in foraging behaviours, such as mobility (Wood et al., Reference Wood, Harris, Raichlen, Pontzer, Sayre, Sancilio and Jones2021), and division of labour (Lew-Levy, Lavi, Reckin, Cristóbal-Azkarate, & Ellis-Davies, Reference Lew-Levy, Lavi, Reckin, Cristóbal-Azkarate and Ellis-Davies2017; Lew-Levy et al., Reference Lew-Levy, Reckin, Kissler, Pretelli, Boyette, Crittenden and Davis2022). Although Pemban children of both sexes live in close contact with the natural and cultivated landscape, boys begin to participate in more gender-specific activities in the forest and sea, such as hunting and fishing (see Lew-Levy, Boyette, Crittenden, Hewlett, & Lamb (Reference Lew-Levy, Boyette, Crittenden, Hewlett and Lamb2020) for further examples of cross-cultural variation in gender segregation during play). Shellfish collection is the main activity in the wild practised preferentially by girls, but young boys often follow their sisters in these expeditions, and are therefore exposed to this kind of ecological knowledge. Accordingly, the two sexes will typically differ to some extent in their amount of exposure to information about the environment, with boys having access to more girl-specific knowledge than the contrary. Indeed, while both sexes learn at similar rates ecological knowledge that is shared, see left panel in Figure 5, boys have access to a whole area of information that is precluded to girls, which is represented in the central panel of the same figure. Our result could help explain why previous studies showed inconsistencies in the amount of knowledge differences found between the sexes (Blacutt-Rivero et al., Reference Blacutt-Rivero, Moraes, Gruca and Balslev2016; Cruz-garcia et al., Reference Cruz-Garcia, Caffi, Elena, Zans and Sanchez-choy2018; Gallois et al., Reference Gallois, Duda and Reyes-García2017; Geng et al., Reference Geng, Zhang, Ranjitkar, Huai and Wang2016; Schniter et al., Reference Schniter, Macfarlan, Garcia, Ruiz-Campos, Beltran, Bowen and Lerback2021; Setalaphruk & Price, Reference Setalaphruk and Price2007). Indeed, the multidimensional nature of ecological knowledge means that the survey design impacts the ability to measure knowledge evenly across dimensions, potentially overestimating the importance of one dimension above the others (e.g. a survey focusing on wild birds will show that males have much higher environmental knowledge, see the Supplementary Information, section 9.4.4 for observations on how the types of data we collected vary in reflecting ecological knowledge differences between sexes).
The importance of activities in promoting the differences between the sexes is supported by the causal analysis of their effect. Once we control for activities, we can see that the differences almost completely disappear (see Figure S16 and last row in Table S6). Hunting, fishing and shellfish collection all have positive effect on ecological knowledge, and they are practised at different rates by boys and girls, so that knowledge differences between the sexes most likely emerge through differential participation to gendered activities, rather than because of any presumed innate attitudes to environmental knowledge.
The differential participation in activities that involve the natural environment can also explain why residing with mothers appears to have a positive effect on ecological knowledge for both girls and boys, but only the latter benefit from the presence of fathers. At least, this would be the case if both sons and daughters learn from their mothers when collecting shellfish on the reef, but only sons learn to fish with their fathers, or if, as found by Hassan, Schaffnit, Sear, Urassa, and Lawson (Reference Hassan, Schaffnit, Sear, Urassa and Lawson2019), mothers do not discriminate, while fathers invest more in their male offspring. The fact that this is the only clear finding from our analysis of the effects of household composition on environmental knowledge may reflect the fact that children are immersed in a large and diverse social environment, which makes it difficult to investigate its effect on knowledge. As in so many contexts (Boyette & Hewlett, Reference Boyette and Hewlett2017; Lew-Levy et al., Reference Lew-Levy, Crittenden, Boyette, Mabulla, Hewlett and Lamb2019; Lew-Levy, Reckin, et al., Reference Lew-Levy, Lavi, Reckin, Cristóbal-Azkarate and Ellis-Davies2017), children are almost always surrounded by other adults, by older siblings or cousins, and by a varied set of peers and neighbours in play groups. Knowledge transmission is likely to happen through all of these ties, probably weakening any direct influences of household structure, particularly if children with few siblings are more likely to seek out neighbours and more distant relatives as playmates. Future investigations into the effects of the social environment on knowledge should probe friendship networks and household composition at a higher resolution.
Our analysis of the effects of participation in formal education on ecological knowledge does not yield a clear answer on whether formal schooling impacts ecological knowledge, consistent with previous work (Quinlan et al., Reference Quinlan, Quinlan, Council and Roulette2016; Reyes-García et al., Reference Reyes-García, Kightley, Ruiz-Mallén, Fuentes-Peláez, Demps, Huanca and Martínez-Rodríguez2010). It is possible that the specific settings of the village where the data were collected (both forest and seashore are close-by) allow children to acquire enough experience of the natural environment irrespective of the amount of time they spend in formal education, but also, schooling might affect what children know about the environment, rather than only how much they know. It is even possible that information on other biomes learned at school substitutes for or even enhances locally relevant environmental knowledge. As such, no effect of schooling would be detected, as it might be substituted by information on other biomes learned at school and result in no variation in total ecological knowledge, as in our sample.
In summary, our results support the idea that the pre-reproductive phase serves as a period for knowledge acquisition. First, we demonstrate that, among children and teenagers in Pemba, ecological knowledge increases with age in a non-linear fashion and that middle childhood is a hot-spot for learning. Second, we show how knowledge differences among the sexes can be ascribed to different dimensions of ecological knowledge, which reflect specialisation. These differences appear not to be innate, but seem to be a product of labour division, given the role played by activities in driving differentiation of ecological knowledge. Third, we show the importance of practising activities for knowledge acquisition and, fourth, we find some evidence that the social environment influences the ecological knowledge of children, but more specific analyses are needed in this sense. All of these results have significant implications for the hypotheses on the evolution of human early life history. Knowledge cannot be acquired very early nor in a short time because of the crucial role that activities play, exposing individuals to relevant information. There are physical constraints to achieving adult rates of success in most foraging activities (Bliege Bird & Bird, Reference Bliege Bird and Bird2002; Bock, Reference Bock2002), but children start trying them out much before they are actually physically apt and proficient (Lew-Levy et al., Reference Lew-Levy, Reckin, Kissler, Pretelli, Boyette, Crittenden and Davis2022), and during this time they seem to be acquiring the necessary ecological knowledge (Lew-Levy & Boyette, Reference Lew-Levy and Boyette2018). Faster learning, boosted for example by innate behaviours acquired through natural selection, such as proposed in the ‘brain-threshold’ or ‘learn-to-reproduce’ models, is probably not adaptive in humans because of the high behavioural flexibility we exhibit. Children have to learn in many different dimensions to complete different tasks, with differences between sexes and individual specialisation.
These insights into the evolution of childhood could be strengthened by applying comparable methods to samples from other populations, thereby providing a more nuanced understanding of the relationship between age and knowledge (Deffner, Rohrer, & McElreath, Reference Deffner, Rohrer and McElreath2021). With such data we can account for cultural variation, while at the same time produce comparable, non-site-specific data to make adequate inferences on generalisable human learning patterns. For example, it might be interesting to examine whether the hot-spot age range identified in Pemba is a human universal, consistent with the ‘brain-filling’ hypothesis, or variable across sites, more consistent with the idea that learning schedules vary according to the ecological affordances offered by different environments and cultures.
Moreover, we advocate for the realisation of longitudinal studies, tracking the variation of ecological knowledge over time in the same child. This would allow us to draw individual learning curves, and thus estimate general trajectories of knowledge acquisition. Longitudinal studies would also allow us to better understand the direction of causation between participation in activities and knowledge about these activities, as well as the effect of social environments.
Finally, we believe that understanding how and when ecological knowledge is acquired is an important goal in today's changing world. Local environmental knowledge is important to cope with climate change (Berkes & Jolly, Reference Berkes and Jolly2002), and can boost the perceived value of plant foods, often considered weeds, which provide important ecosystem services (Turner et al., Reference Turner, Luczaj, Migliorini, Pieroni, Dreon, Sacchetti and Paoletti2011). In fact we know that agricultural societies rely on the natural (uncultivated) environment for multiple services: archaeological evidence proves that the transition to agriculture did not exclude foraged food from neolithic diets (Ottoni et al., Reference Ottoni, Boric, Cheronet, Sparacello, Dori, Coppa and Cristiani2021), modern populations fall back on wild foods in case of famine (Walsh, Reference Walsh1995) and children are observed foraging in many different social settings (Lee & Brewis, Reference Lee and Brewis2009). Hence we believe that our study on ecological knowledge of children in Pemba can add a relevant contribution to the literature on how societies can adapt to social and environmental transitions.
Acknowledgements
This work would not have been possible without the kindness and hospitality of people in Pemba. In particular, the staff at the Department of Forestry and Non Renewable Natural Resources in Wete, Asha Yusuf Khamis and her family, our neighbours and the rest of the people in the village. To Dr Martin Walsh, Massoud Bakar Massoud, Bakar Makame Khamis and Haji Masoud Hamadi a special thanks is due for helping review the list of items on the freelist.
Author contributions
IP, MBM and RM designed the study. IP collected the data. IP and RM performed statistical analysis. IP drafted the MS with inputs from RM and MBM.
Financial support
This research is funded by the Department of Human Behavior, Ecology, and Culture at the Max Planck Institute for Evolutionary Anthropology.
Conflicts of Interest declarations in manuscripts
The authors declare they have no conflict of interest.
Research transparency and reproducibility
The data that support the findings of this study are openly available in https://github.com/lalla-ilaria/Children_eco_knowledge and https://osf.io/wjmgq.
Supplementary material
To view supplementary material for this article, please visit https://doi.org/10.1017/ehs.2022.31.