1. Introduction
In 2017, 203 of the estimated 248 biogas generators operating in the United States were located on dairy farms (U.S. Environmental Protection Agency [EPA], 2017). The other 45 anaerobic digester (AD) systems consist of installations on hog farms. Dairies are more likely candidates than hog farms for AD systems for two major reasons: (1) The majority of U.S. hog farms are in areas with low electricity prices, and (2) low-fiber diets in hog production do not provide enough digested fibers to be marketed as a coproduct for bedding (EPA, 2015). Further, dairies are located across a wider geographic range and produce digested fiber that can be marketed as bedding, thus providing an additional income stream.
Digester installation costs vary widely and are often cited as a barrier to adoption (Cowley and Brorsen, Reference Cowley and Brorsen2018b), but the economic benefits of adoption for producers can include the following: electricity generated for on-farm use; electricity sales in local markets; heat; and on-farm fertilizer, animal bedding, and compost production (EPA, 2018). Biogas generation also affords environmental benefits such as reducing odor from manure, lowering greenhouse gas emissions, decreasing pathogens, and decreasing weed seed germination (Yiridoe, Gordon, and Brown, Reference Yiridoe, Gordon and Brown2009). By reducing the negative externalities of livestock production, adoption of biogas generation could generate positive externalities that extend beyond the farm.
Given the on- and off-farm benefits of AD systems, installation of biogas generation systems in the United States is supported by the EPA and U.S. Department of Agriculture (USDA) through the outreach program AgSTAR. AgSTAR provides information, guidance, and research to aid producers’ adoption of AD systems. Grant support for initial capital investment is provided through the Renewable Energy for America Program (REAP) (USDA, 2015). However, nationwide AD adoption may be suboptimal. In a study estimating market potential of biogas systems on dairy and swine farms, AgSTAR indicated that dairy and swine manure is an underutilized resource with the potential to generate 13,144,441 MWh of electricity per year in the United States (EPA, 2018). In total, this is enough electricity to power more than 1,200,000 average homes, or less than 1.0% of all homes (U.S. Census Bureau, 2018; U.S. Energy Information Administration, 2018b). In a recent report, the EPA (2018) identifies 2,704 dairies in the United States as potential AD system candidates; however, herd size was the sole criterion for determining farm suitability. States with the greatest potential include California (799 farms), Idaho (179 farms), Wisconsin (358 farms), and Texas (126 farms). AgSTAR’s analysis claims that if AD systems were installed on those 126 Texas dairies, a total of 429,000 MWh/year could be generated (EPA, 2018), enough to power 40,000 average homes, just over half of the homes in Amarillo, Texas, or 0.5% of all of the homes in Texas (U.S. Census Bureau, 2018; U.S. Energy Information Administration, 2018b). To support adoption, REAP allocated $600 million in grant funding and loans for 2018 (Lips, Reference Lips2018), which suggests that financial resources exist to support adoption of this production practice.
Despite financial support and outreach for producers, compared with the previous 5- and 10-year averages, the rate of AD installation has decreased since 2013. AgSTAR’s Livestock Anaerobic Digester Database shows a construction rate of 2.2 AD systems/year since 2013 (EPA, 2019). Previously, from 2008 to 2012 and 2003 to 2012, AD systems were constructed at a rate of 8.8 AD systems/year and 7.4 AD systems/year, respectively. Based on market potential reports from AgSTAR, the rate of construction has slowed in an unsaturated market, even as the average size of dairies continues to grow (Mahnken and Hadrich, Reference Mahnken and Hadrich2018). One explanation for the observed decreased installation rate could be that the AD systems may not be profitable or that necessary support for installation and profitable operation might not exist.
Case studies and more in-depth research suggest that although the size of the dairy is an important factor to determine suitable AD candidates (Klavon et al., Reference Klavon, Lansing, Mulbry, Moss and Felton2013), success and profitability of a biogas generation system is also based on the availability of subsidies and support for investment (Key and Sneeringer, Reference Key and Sneeringer2012) and access to electricity markets (Camarillo et al., Reference Camarillo, Stringfellow, Jue and Hanlon2012; Cowley and Brorsen, Reference Cowley and Brorsen2018a). Specifically, AD system adoption has been successful in states with favorable electricity pricing schemes such as feed-in-tariffs or additional price premiums (Wang et al., Reference Wang, Thompson, Parsons, Rogers and Dunn2011) or where additional environmental regulations and grant support exist (Anders, Reference Anders2007; Ellerby, Reference Ellerby2010).
An overview of case studies showed that although the specific factors that influenced profitability varied from farm to farm, important variables were efficiency rate of biogas conversion and herd size (Giesy et al., Reference Giesy, Wilkie, de Vries and Nordstedt2005); ability of a manager to maintain records and sell back electricity to the grid at high prices (Lazarus and Rudstrom, Reference Lazarus and Rudstrom2007); governmental support and digester sizing (Skovsgaard and Jacobsen, Reference Skovsgaard and Jacobsen2017); and herd size and coproduct marketing (Leuer, Hyde, and Richard, Reference Leuer, Hyde and Richard2008). Additional variables used in the evaluation of profitability included, but were not limited to, the change in milk cost, payback period, the internal rate of return on the digester, and the electricity price required to achieve breakeven (Lazarus and Rudstrom, Reference Lazarus and Rudstrom2007).
Conversely, European agricultural producers have overwhelmingly adopted biogas systems, and studies show systems are profitable, benefit from governmental support, and are broadly distributed geographically (Jacobsen, Laugesen, and Dubgaard, Reference Jacobsen, Laugesen and Dubgaard2014; Nielsen and Hjort-Gregersen, Reference Nielsen and Hjort-Gregersen2002). In Europe, strict environmental targets requiring reductions of fossil fuel reliance have further motivated adoption of AD systems for a variety of waste streams and encouraged diversification in coproducts and use of generated by-products (Grando et al., Reference Grando, de Souza Antune, Da Fonseca, Sánchez, Barrena and Font2017). Contrary to the U.S. experience, AD systems in Europe are producing electricity, heat, and methane for transportation, which further increases the available income streams (Scarlat, Dallemand, and Fahl, Reference Scarlat, Dallemand and Fahl2018). Although overall the European energy markets are utilizing and incorporating more products of biogas generation than any other region, variation can be seen across countries as to the source of feedstocks, electricity generation, and utilization of other coproducts (Scarlat, Dallemand, and Fahl, Reference Scarlat, Dallemand and Fahl2018). This suggests that perhaps region-specific analysis could be pursued in the United States to encourage adoption of profitable AD systems; an inability to use or generate income from the methane and heat remains a limiting factor.
Studies of potential AD projects that rely solely on meeting minimum total head requirements and ignore regional market conditions do not accurately predict or identify sites for profitable installation, and continued operation, nor do they accurately represent risk to producers. Substantial changes are occurring in the dairy industry as farm consolidation, price changes, and changes in dairy locations are leading to alterations in historic dairy norms (Mahnken and Hadrich, Reference Mahnken and Hadrich2018). Given the interest in biogas generation and federally funded information suggesting the benefits of AD adoption, accurate regional analysis of on-farm profitability is needed. The Texas dairy industry is expanding, and there is interest in biogas generation (Modglin, Reference Modglin2010). In Texas, there is a need to inform government initiatives and producers when considering biogas generation systems. Thus, the objective of this study is to incorporate risk into an enterprise analysis of AD adoption on Texas dairies and to suggest conditions that might lead to a profitable environment for AD installation. The analysis uses simulated enterprise budgets of AD (Enahoro and Gloy, Reference Enahoro and Gloy2008) and industry assumptions of AD to test the profitability of digester installation and maintenance under risk using data from representative farms in Texas. Sensitivity analysis is included to show profitability under varying governmental support and electricity market conditions that exist in other states. This research specified to Texas farms is the first to (1) simulate the financial viability of AD installation and consider risk or other potential sources of input variability in the assessment of AD enterprise profitability and (2) demonstrate alternative market and governmental conditions that might lead to a profitable environment for AD installation.
2. Methodology and data
This study uses a simulated enterprise analysis to evaluate the 20-year profitability of farm-level investment in a lagoon AD system on dairy farms in Texas. The enterprise analysis is a regionally tailored model of dairy biogas generation system adapted from Enahoro and Gloy (Reference Enahoro and Gloy2008). Uncertainty and risk are included with methodology based on Richardson and Mapp (Reference Richardson and Mapp1976) and Richardson and Condra (Reference Richardson and Condra1981). The output variable is net present value of profit (NPV) as simulated using an enterprise analysis framework (Richardson, Reference Richardson2008).
2.1. Model
NPV at the end of a 20-year period was the key output variable taken from simulated model results. NPV is a measure of the profitability of the investment and captures whether the investment was profitable over 20 years. The basic model can be represented as follows:

Where

TCij = total investment costs
Grantt = grant support, which varied based on assumed scenario.
r is the discount rate and is assumed to be 10%
i is the subscript representing which Texas farm
j is the subscript representing which investment cost is assumed
k is the subscript representing which scenario is represented
The basic model is built for two different assumed farms in Texas that are subject to three different initial capital investment levels ($/cow), under five different assumed scenarios and a sensitivity analysis for electricity pricing and grant support. Each model uses the static and stochastic inputs described in the following sections to simulate 1,000 possible outcomes of each model under each scenario. An average NPV is then obtained using the 1,000 outcomes generated.
2.2. Representative farms
Model inputs to accurately depict farms were based on two representative dairies in Texas provided by the Agricultural and Food Policy Center (AFPC) at Texas A&M University. One set of models represented a dairy farm in central Texas with 1,500 head of milking-age cows, and a second set represented a farm in northern Texas with 3,800 milking-age cows. Data from the 2012 Census of Agriculture (USDA, 2014) indicate the count of dairies in Texas with more than 500 head of milking-age cows and their corresponding herd size. These characteristics demonstrate that representative farm herd sizes are illustrative of most farms in the state.
The representative farm data provided by AFPC represent two of the three inventory levels surveyed by the 2012 Census of Agriculture (Table 1), which account for approximately 75.0% of the cows milked in Texas and thus are representative of Texas dairies based on herd size and geographic distribution within the state. The three levels reported in Table 1 account for all dairies that AgSTAR cites as candidates for biogas generation through installation of ADs based on herd size.
This analysis primarily uses the herd size for each dairy to calculate electricity demand and manure generation. Dairy size provides an estimate of total kilowatt-hours of electricity used as well as manure generated. The AFPC representative farm information included estimates of the number of lactating and dry cows. Lactating cattle and dry cattle produce different amounts of manure. No other biological information was gathered on the herd.
In addition to using the number of animals from each of the AFPC representative dairies, scenarios use cost information to form the basis for cost savings from coproduct generation (CoProductOffsetski ) and the capital contribution for construction. The simulation used the representative farm’s reported cost of bedding (the larger dairy does not purchase bedding) and their annual cash receipts to establish a capital contribution amount toward constructing a new biogas generation facility.
2.3. Total revenue
The biogas generation system produces revenue through the sale of electricity, cost savings from eliminating off-farm electricity purchases, and coproduct offsets in the form of bedding. Revenue varies by farm as electricity consumption and production are dependent on herd size. Coproduct offsets are only possible on the small dairy and under certain scenarios.
2.3.1. Electricity production, electricity sales, and offset electricity costs
The model generates a stochastic amount of manure produced by individual cows. Steele (Reference Steele1995) provides a mean and standard deviation of the amount of manure produced by different livestock species and the components of each species’ manure. A normal distribution of manure produced per head per day constructed using data from Steele (1995; x− = 190 lb., s = 37 lb. for lactating animals, x−= 104 lb., s = 20 lb. for dry) is multiplied by the number of cows in each of the lactating and dry cow categories to obtain the total daily pounds of manure produced on each dairy.
In order to introduce variability into the biogas system’s output amount, a stochastic element was added to the amount of solids converted to biogas as a percent, and the cubic feet of biogas per pound of volatile solids converted. The model assumes a uniform distribution from 10.0% above and 10.0% below the expected values of both figures that are important in determining the amount of electricity the AD generates and reflect real-life variability in system output based on fluctuation in management decisions by operating staff. Lazarus and Rudstrom (Reference Lazarus and Rudstrom2007) suggest that daily management decisions are key in the overall efficiency and amount of biogas produced by a biogas generation system.
In order to value the amount of electricity that the biogas generation system generated in kilowatt-hours/year, the model calculates millions of British thermal units (MMBtu) per year provided by the manure production and converts them into kilowatt-hours. Kilowatt-hours are multiplied by the stochastic thermal conversion efficiency and daily online percentage of the system to obtain the final value of kilowatt-hours/year generated. The daily online percentage decreases by a percent each year to account for increasing risk of breakdowns leading to lower use not accounted for by depreciation of the asset.
Data from the University of Vermont Extension indicate that dairy cows in the United States require between 800 and 1,200 kWh of electricity annually (Spring Hill Solutions, 2009). A survey of dairy management systems in the southwestern United States by Capareda and Mukhtar (Reference Capareda and Mukhtar2010) showed lower kilowatt-hours/cow use in Texas dairies. Their survey of two north Texas dairies found usage of 299 kWh/cow and 268 kWh/cow. The two dairies surveyed were a 7,000-head, open-lot/free stall combination dairy and a 6,000-head, open-lot dairy. The mean (x−= 283.5 kWh/cow) and standard deviation (s = 15.5 kWh/cow) of the survey results formed a normal distribution of northern Texas electricity usage/cow. Capareda and Mukhtar’s (Reference Capareda and Mukhtar2010) survey of central Texas dairies found usage of 723 kWh/cow, 566 kWh/cow, 406 kWh/cow, and 692 kWh/cow. The four central Texas dairies surveyed were a 2,200 open-lot/free stall combination dairy, a 2,100 open-lot dairy, a 550 open-lot/free stall combination dairy, and a 990 open-lot/free stall combination dairy. The mean (x−= 652.8 kWh/cow) and standard deviation (s = 56.5 kWh/cow) of the survey results formed a normal distribution of central Texas electricity usage/cow.
Total farm energy requirements (kilowatt-hours/year) are the sum of the power use of the biogas generation system and the pre-system-installation farm requirements. The amount of electricity that the biogas generation enterprise sold back to the dairy is equal to the total farm energy requirements (kilowatt-hours/year) net of the requirements of the biogas generation system. The value of the electricity sold back to the farm is equal to the benefit of the defrayed electricity cost from installation of the biogas system. Depending on the scenario, excess electricity can be sold back to the grid. The value of on-farm electricity generated is equal to the stochastic purchase price from the grid (cents/kilowatt-hour) multiplied by the stochastic real electricity savings in kilowatt-hours per year. The value of excess electricity is generated by multiplying the excess generation in kilowatt-hours by the stochastic sale price to the grid (cents/kilowatt-hour).
A normal distribution was also used to obtain stochastic values of the cost of power purchased by each dairy and the wholesale price paid for power that the dairy generated and sold back to the grid. Using annual average energy price from the U.S. Energy Information Administration from 1990 to 2016, the model generates a stochastic purchase price from the grid with x−= 7.84 cents and s = 1.22 cents (U.S. Energy Information Administration, 2018a). The model assumes a normally distributed stochastic wholesale price (cents/kilowatt-hour) of excess electricity generated and sold to the grid, with x−= 3.4 cents and s = 1 cent using annual price from the 2016 State of the Market Report for the ERCOT Electricity Markets (Potomac Economics, 2017).
2.3.2. Coproduct benefits
There are substantial direct and social benefits from coproducts of AD systems. Jacobsen, Laugesen, and Dubgaard (Reference Jacobsen, Laugesen and Dubgaard2014), Nielsen and Hjort-Gregersen (Reference Nielsen and Hjort-Gregersen2002), and Holm-Nielsen, Seadi, and Oleskowicz-Popiel (Reference Holm-Nielsen, Seadi and Oleskowicz-Popiel2009) point to profits available from using coproducts of biogas generation systems such as fertilizer sales, sale of excess electricity, and the use of dried effluent as bedding. In addition to explicit profits, socioeconomic benefits from AD systems include reduced odor, storage savings for liquid manure, and greenhouse gas reduction (Nielsen and Hjort-Gregersen, Reference Nielsen and Hjort-Gregersen2002).
The only coproduct evaluated in this study is defrayed costs of bedding by using dried effluent as bedding material. The majority of Texas dairies incorporate effluent from their cattle in crop fertilization on-site or in sales for that use on other farms. As that practice is well established and the value of nitrogen, phosphorus, and potassium already accrue to the dairy, gains from the sale of those nutrients would be double counted if included as a use in the enterprise analysis of an AD.
The returns from defrayed bedding costs (CoProductOffsetski ) where k ∈ (ES+BG, ES+BG+G, BG+G) can be represented as

The value of CoProductOffsetski is then added as revenue to the AD system total revenue.
2.4. Total costs
2.4.1. Initial investment costs
Based on analysis of reported projects in the AgSTAR project database, there is no commonality in biogas generation system capital cost based on location, number of cows, type of system, or year of installation. In order to determine appropriate capital costs for the enterprise analysis, data on real capital costs per cow ($/cow, 2017 dollars) were gathered from AgSTAR’s Livestock Anaerobic Digester Database for 87 dairy projects listed from 1979 to 2017 (see Table A1 in the Appendix). An empirical distribution of potential capital costs ($/cow) was calculated using the information provided by the database and publicly available information found in newspapers, promotional material, and other self-reported values of capital costs available on individual dairy farm websites. The empirical distribution was chosen to address the sparse data set (89 observations), with the bulk of observations covering a period of almost 20 years under different dairy policies and market conditions. An additional option would be to conduct the simulation assuming a GRKS (Gray, Richardson, Klose, and Schumann) distribution of potential capital costs ($/cow), which would allow for 2.2% of draws to be above the maximum reported value and 2.2% below the minimum reported value.
Based on the AgSTAR Livestock Anaerobic Digester Database, the cost of AD construction per head did not necessarily decrease as the number of head increased. The lower quantile value, average, and upper quantile capital expenditure under a 95.0% confidence level using an empirical distribution, were low = $342.74/cow, average = $1,790.40/cow, and high = $5,547.15/cow, respectively. These values formed three separate capital cost levels ($/cow) that were simulated for each size dairy.
Based on the enterprise budget used (Enahoro and Gloy, Reference Enahoro and Gloy2008), the generator, boiler, pumps, and controls have a depreciable life of 7 years. The remainder of the cost of a building, site work, power wiring, manure piping, and the digester tank itself have a depreciable life of 20 years. Each category of capital expenditures was depreciated using the straight-line method (Enahoro and Gloy, Reference Enahoro and Gloy2008).
2.4.2. Grant support
The body of literature on biogas generation from cow manure on dairies overwhelmingly indicates the necessity of grant support. The average construction cost of the AD included in the AgSTAR Livestock Anaerobic Digester Database (shown in the Appendix) is $3.875 million. REAP provides loan assistance and grants for producers seeking to install a biogas collection and electricity generation system. REAP is the only grant support widely available to Texas dairy producers. Grant assistance is capped at the lesser of 25.0% of the total capital costs of the project or $500,000 (USDA, 2015). Certain scenarios evaluated in the model incorporate grant assistance. In 49 of the 54 cases of installation on 1,500-head dairies in the AgSTAR livestock Anaerobic Digester Database, AD construction costs exceeded $1 million. For those 49 dairies, the available grant support does not even cover half of the construction cost.
2.5. Scenarios
The model evaluates AD installation on each dairy under a total of 15 different scenarios. Each scenario introduces an additional mix of “coproduct” or financial support in an attempt to make the investment in biogas energy generation systems more profitable and reflect realistic projects in operation. For each of the three levels of capital cost, five scenarios were simulated. Table 2 describes the scenarios evaluated by the model.
Table 2. Description of scenarios evaluated in study

Note: Low (L) = $342.74, average (A) = $1,790.40, and high (H) = $5,547.15.
The first scenario is the base scenario, which is evaluated under each capital cost level under the assumption that the dairy does not sell energy back to the grid, has no gains from deferred bedding costs, and does not obtain any grants.
The second scenario under each capital cost model assumes that the dairy does possess the capability to sell excess power generated back to the grid and can secure a contract to sell excess electricity. The costs of equipment required to sell excess power is not included in the estimate and is considered negligible or already encompassed in the construction costs. The second scenario is called the electricity sale (ES) scenario.
The third scenario evaluated under each capital cost assumes the dairy possesses the ability to sell excess power back to the grid and can use dried refuse from the AD system as bedding. The use of dried refuse from the AD system as bedding defers a standard cost of certain types of dairies. The large dairy included in the analysis does not have an initial bedding cost, a common occurrence in “feedlot” style dairies. This is called the electricity sales and bedding gains (ES+BG) scenario.
The fourth scenario evaluated under each capital cost model assumes that the dairy possesses the ability to sell excess power back to the grid, can use dried refuse as bedding, and qualifies for grant support. This is called the electricity sales, bedding gains, and grant support (ES+BG+G) scenario.
The final scenario evaluated under each capital cost model evaluates a case deemed “the Texas Case,” which allows for grant support and bedding gains where applicable, but not energy sales. The cost of electricity in Texas is low enough that sales to the grid are not common, and so the inclusion of those gains is not realistic. This scenario was created to model market conditions that Texas producers would face when deciding to install an AD. This scenario is called the bedding gains and grant support (BG+G) scenario.
2.6. Sensitivity analysis
Sensitivity of AD profitability to changes in the price for electricity sold back to the grid and available grant support were included. Values for the price of electricity as well as the percentage of capital costs covered by grant support were modeled after programs in other U.S. states. Motivated by Vermont’s feed-in-tariff and incentive rate of $0.20/kWh (Wang et al., Reference Wang, Thompson, Parsons, Rogers and Dunn2011), sensitivity analysis was conducted assuming excess energy sales to the grid priced at $0.05/kWh, $0.10/kWh, $.15/kWh, and $0.20/kWh. Further, as shown in Cowley and Brorsen (Reference Cowley and Brorsen2018a), based on responses from producers grant support up to 75% of initial capital costs greatly increases NPV of projects. Thus, for this sensitivity analysis, grant support of 25%, 50%, and 75% of initial capital costs was included. Under the most favorable conditions, AD installation would occur with high electricity prices and considerable grant support. Thus, one analysis was conducted assuming grant support of 75% of initial capital costs and excess electricity sales priced at $0.20/kWh.
2.7. Distributions and risk
Deterministic investment feasibility analyses that do not incorporate a component of risk only yield a point estimate for the variables being estimated, instead of a distribution of outcomes (Hardaker et al., Reference Hardaker, Richardson, Lien and Schumann2004; Reutlinger, Reference Reutlinger1970; Richardson et al., Reference Richardson, Herbst, Outlaw and Gill2007). The increasingly uncertain environment in which farmers and ranchers operate necessitates the inclusion of risk into any choice (Richardson and Mapp, Reference Richardson and Mapp1976; Richardson, Klose, and Gray, Reference Richardson, Klose and Gray2000), and a key component of incorporating risk into any simulation is the inclusion of stochastic components modeled under a suitable distribution. In order to incorporate risk, each model is simulated using the Latin hypercube procedure, which segments each distribution into N intervals and ensures that, at minimum, one value is randomly chosen from each interval (Richardson, Reference Richardson2008).
The models utilize three types of distributions to create stochastic values for certain variables in order to incorporate risk into those variables. Table 3 describes the distributions used in the model and the source of the data used to construct each distribution’s parameters.
Table 3. Descriptions of variables and distributions used in enterprise analysis of anaerobic digester
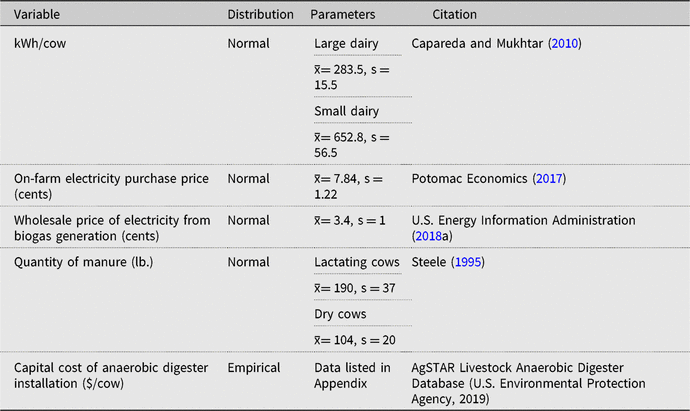
The normal distribution is utilized to calculate the kilowatt-hours/cow of electricity needed on each dairy, the stochastic wholesale price of electricity from the dairy, the stochastic price of electricity purchased by each dairy, and the amount of manure produced by each farm’s cows. The amount of manure produced is an important component when determining the amount of energy generated to offset electricity costs and generate revenue from electricity sales, and, ultimately, whether or not the biogas generation project is profitable. The normal distribution was appropriate for each of the variables discussed as the necessary parameters, mean and standard deviation, were available from accessible data.
The second type of distribution used in the biogas generation project enterprise analysis was the empirical (EMP) distribution, which estimates parameters using historical price data for the cost/cow of a biogas generator. Assuming an EMP distribution of these data avoids enforcing a specific distribution on the variable in question. The EMP distribution was applied to the initial capital outlay required to construct a biogas generator. Few observations were readily available and varied widely, making the EMP the ideal distribution (Richardson, Reference Richardson2008). The parameters produced by the EMP distribution provided the low, medium, and high level of capital costs, which served as the basis for the individual models under each dairy size.
The third type of distribution is the uniform distribution, used to characterize uniformly varying efficiency of capture in the biogas generation system. The uniform distribution draws evenly from values between 0 and 1, and the uniformly drawn value is multiplied by the efficiency level. The stochastic efficiency level represents fluctuation in management decisions by operating staff.
3. Results and discussion
We find that for both dairies, under all five scenarios, NPV is expected to be negative for an AD if investment costs per cow are either the average or upper cost/cow estimated (Tables 4 and 5). This analysis calculated simulated NPV of investment averaged across all scenarios for the small (central) dairy of $166,236 for the low-cost model, −$1,124,972 for the average-cost model, and −$4,476,218 for the high-cost model. Similarly, we obtained an average NPV across scenarios for the large (northern) dairy of $192,406 for the low-cost model, −$3,012,839 for the average-cost model, and −$11,502,664 for the high-cost model.
Table 4. Summary statistics for simulation for net present value of enterprise analysis for installation of biogas generator on a 1,500-cow dairy in central Texas

a Scenario abbreviations defined previously as ES (energy sales), ES+BG (energy sales and bedding offsets), ES+BG+G (energy sales, bedding offsets, and grant support), and BG+G (bedding offsets and grant support).
Table 5. Summary statistics for simulation for net present value of enterprise analysis for installation of biogas generator on 3,800-cow dairy in North Texas
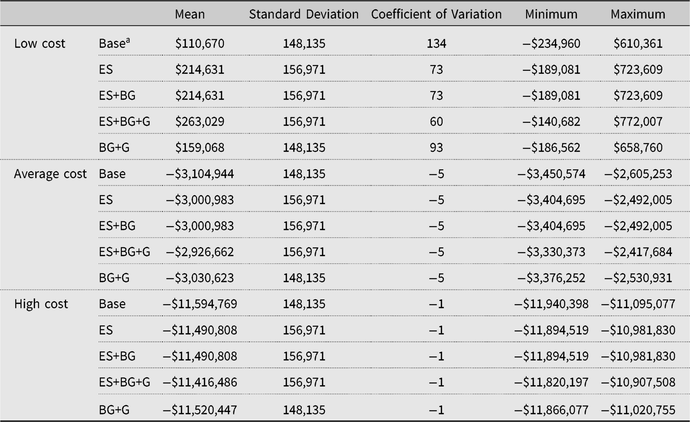
a Scenario abbreviations defined previously as ES (energy sales), ES+BG (energy sales and bedding offsets), ES+BG+G (energy sales, bedding offsets, and grant support), and BG+G (bedding offsets and grant support).
These results suggest that profitability of AD investment is highly dependent on investment costs and that investment should not be made unless total investment costs per cow are near the lowest capital cost documented by AgSTAR, similar to our low-cost model. In fact, the average NPVs for all scenarios in the average- and high-cost models are negative. When we compare results between representative farms, we find that although the larger farm creates more manure—and potential electricity generation—the smaller farm has a higher average NPV of installation and might be better suited to install an AD system. The more positive financial outcome is because under the current grant availability, the initial grant investment covers a larger percentage of the initial capital cost outlay for the smaller farm compared with the larger farm.
Under the low-cost/cow model, we obtained a mean NPV across simulations for the small dairy ranging from $16,591 (base scenario) to $266,000 (ES+BG+G and ES+BG scenarios) demonstrating that profitability of the system also depends heavily on available support and how the farm uses system outputs (Table 4). We found that the large dairy also showed a similar relationship between profitability and financial support and system outputs. In the large dairy simulations under the low-cost/cow model, mean NPVs ranged from $110,670 (base scenario) to $263,029 (ES+BG+G). The base and BG+G scenarios had lower standard deviations compared with the scenarios that included electricity sales. We also obtained smaller standard deviations on the small farm, $58,745 (base and BG+G scenarios) and $62,879 (ES, ES+BG, and ES+BG+G scenarios), compared with the large farm, $148,135 (base and BG+G scenarios) and $156,971 (ES, ES+BG, and ES+BG+G scenarios). For all three cost levels modeled on both dairies, the base case was always the least profitable. When we compare the dairies, we find that, despite increasing returns to scale often present in agriculture, the large dairies in Texas are at a disadvantage because of caps on grant support and because typically bedding is not used.
For both dairies, under a low-cost/cow investment, some of the simulated outcomes of NPV of AD investment fall above $0.00. For the large dairy, approximately 20% of the simulated outcomes in the base scenario exhibit an NPV of investment below $0.00 compared with 40% of the simulated outcomes in the small dairy. In the large dairy, ES and ES+BG scenarios exhibit the same cumulative distribution function (CDF) (Figure 1). The overlap occurs because the larger dairies in Texas typically do not have bedding that can be offset by AD coproducts. The distributions of simulated average NPV of investment are highly sensitive to the inclusion of grant support, and with the inclusion of grant support and electricity sales, the most profitable scenario for a larger dairy exhibits a positive NPV of investment more than 95.0% of the time under the low-cost/cow scenario.
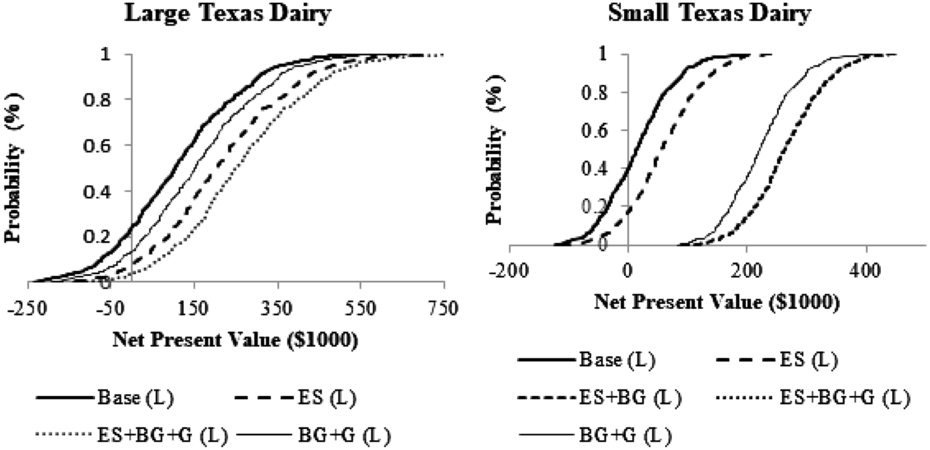
Figure 1. Cumulative distribution functions (CDFs) of net present value for an anaerobic digester installation on two representative dairy farms in Texas comparing five alternative scenarios under low capital cost investment: base, ES (energy sales), ES+BG (energy sales and bedding offsets), ES+BG+G (energy sales, bedding offsets, and grant support), and BG+G (bedding offsets and grant support).
When we observe the CDF for the smaller dairy, we find the positive impact of bedding gain offsets and grant support on NPV of investment. In the simulation of the small dairy, the ES+ BG, ES+BG+G, and the BG+G scenarios lie entirely above zero meaning that, given our assumptions, if small farms can invest in low-cost/cow systems, receive adequate grant support, and use dried effluent to offset bedding costs, then the NPV of the investment will be greater than zero, or profitable, nearly 100.0% of the time (Figure 1). Given that there is an upper bound on available grant support, installing systems that cost less per cow and therefore keep overall investment low allows the farm to recover a greater percentage of the total investment costs. We chose not to display the average- and high-cost models for both farms’ simulated NPV of AD investment because their outcomes were entirely negative.
The simulated breakeven construction cost of installing AD in Texas is approximately $342/cow, and installing an AD is potentially more profitable for a smaller dairy compared with a larger dairy. These results support our hypothesis that original estimates of biogas generation potential in Texas were overestimated because candidate farms were selected solely based on herd size. Further, although government support makes the ending NPV of any capital expenditure more feasible, the amount of support necessary for a profitable investment in Texas under high capital costs does not exist based on current programs. Low electricity prices in Texas make it very unlikely, under most models, that a dairy will recoup its investment in the first 20 years after installation. Additionally, we assumed that the initial capital cost included the price of equipment required to connect to the grid. That cost could vary widely based on distance to a viable collection point for excess power generation, and an increased cost would decrease the final NPV. We have also assumed that farms of all sizes employ a manure scraping system that allows for inexpensive manure collection and deposit into an AD system. In the event that a dairy does not possess this technology—a common occurrence on smaller dairies—its installation would increase the initial capital cost and decrease the final NPV.
When we consider additional components such as offsetting bedding costs, selling electricity back to the grid, and finding grants to support installing AD, some of the initial costs may be offset, but it may not be enough to make the project financially feasible. Further, limits on grant support or caps on government incentives favor smaller dairies, which have a lower grant to capital investment ratio. For this reason, it may be more profitable for a smaller dairy to invest in an AD but not profitable for a larger dairy given that larger farms do not benefit from economies of scale.
Based on these constraints, we extended the analysis to vary the price for electricity sold back to the grid and available grant support to determine if alternative market pricing schemes or governmental support would lead to a positive NPV of investment for projects with average or high costs of investment. Given that investments with a low cost/cow already exhibit a positive NPV of investment on average and the NPVs for all simulated observations for some scenarios are positive, only results for the average-cost/cow simulations will be discussed.
Despite increasing the price of electricity sold back to the grid more than 500% from the initial analysis, the NPV of installation assuming an average cost/cow remains negative for both the large and small dairies (see Figure 2). This suggests that even substantial increases of electricity pricing or additional price incentives cannot overcome the burden of initial capital outlay.
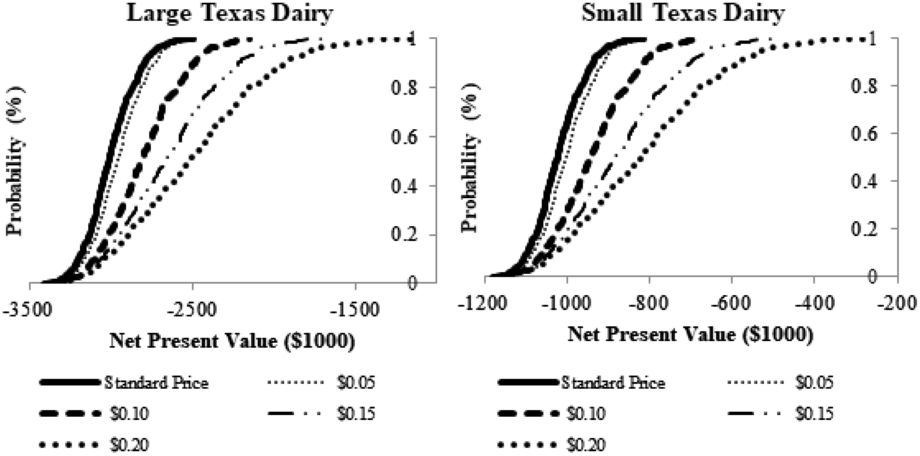
Figure 2. Cumulative distribution functions (CDFs) of net present value for an anaerobic digester installation on two representative dairy farms in Texas comparing five alternative electricity price scenarios under average capital cost investment: standard price (~$0.03/kWh), $0.05/kWh, $0.10/kWh, $0.15/kWh, and $0.20/kWh.
Other governmental support in the form of grants to cover the initial investment costs has been shown in installed digesters to increase the profitability of investment. However, even moderate grant support (50% of initial capital costs) was insufficient with the average simulated NPV of investment negative for both the small and large dairies (see Figure 3). In fact, only in the small dairy, with 75% grant support for initial capital investment, did we find that the average-cost/cow systems could achieve a positive NPV. Given these findings, it is not likely that providing additional state-level grant support will lead to profitable AD systems.
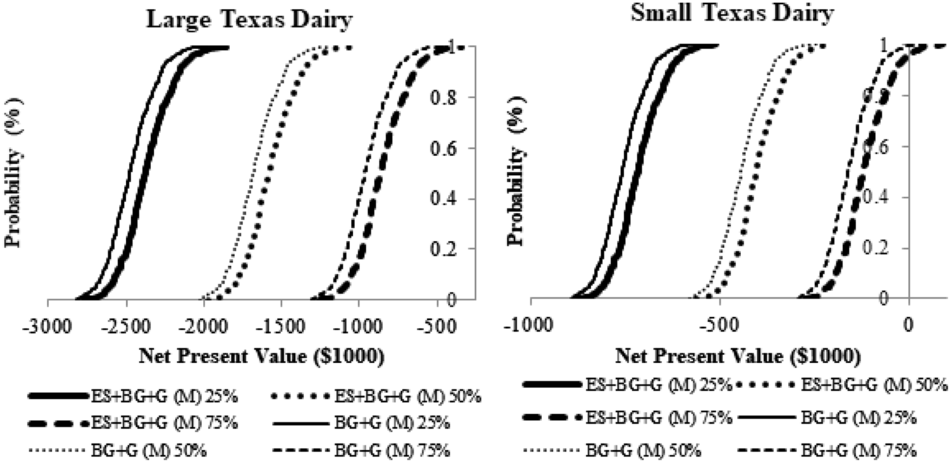
Figure 3. Cumulative distribution functions (CDFs) of net present value for an anaerobic digester installation on two representative dairy farms in Texas comparing three alternative grant support percentages for total capital costs under two alternative scenarios assuming an average capital cost investment: ES+BG+G 25% (energy sales, bedding offsets, and grant support of 25%), ES+BG+G 50% (energy sales, bedding offsets, and grant support of 50%), ES+BG+G 75% (energy sales, bedding offsets, and grant support of 75%), BG+G 25% (bedding offsets and grant support of 25%), BG+G 50% (bedding offsets and grant support of 50%), and BG+G 75% (bedding offsets and grant support of 75%).
The sensitivity analysis demonstrates that even with additional governmental support in the form of grants for initial capital costs and higher electricity prices to increase yearly income from the AD system, simulated NPV of investment is likely negative for digesters with average- or high-cost/cow systems. This finding is likely because of high yearly maintenance and operating costs, as well as asset depreciation. With additional risk added into yearly operations, these costs become even greater. Comparing the results for the additional grant support and higher electricity costs suggests that if faced with either funds to support grants of 75% or electricity prices of $0.20/kWh, producers would benefit more from grant support; however, neither option individually or jointly is enough to lead to positive average simulated NPV of AD adoption for either the average- or high-cost/cow systems.
4. Conclusion
Under the assumptions in our study, digesters are likely not profitable in Texas for the following reasons: (1) low price received when selling electricity to the grid (2) highly variable costs of investment based on available budgets of similar projects, and (3) few government incentives or grant support. Other similar studies nationwide have noted the sensitivity of profitability to electricity prices and ability to reuse bedding or take advantage of other coproducts (Lazarus and Rudstrom, Reference Lazarus and Rudstrom2007; Leuer, Hyde, and Richard, Reference Leuer, Hyde and Richard2008). However, Leuer, Hyde, and Richard (Reference Leuer, Hyde and Richard2008) found that larger dairy farms are more likely to profit from AD systems. Unlike other regions of the United States, the lack of bedding on large Texas dairies and a cap on available grant support that is not proportional to total capital costs remove any increasing returns to scale that were present in other previous studies.
Our results show that simulated NPVs for AD installation are positive when the low cost/cow is assumed. Thus, this work demonstrates the importance of low initial investment costs to overall profitability. Further, in this analysis, we show that introducing financial and production information from farms in Texas combined with Texas’s low electricity prices decreases the financial viability of AD investment on dairy farms in Texas. However, there are nonmarket benefits that accrue to society as a result of AD of dairy waste such as odor reduction and greenhouse gas emission reduction (Nielsen and Hjort-Gregersen, Reference Nielsen and Hjort-Gregersen2002). These nonmarket benefits do not accrue to the owner of the installation and therefore do not contribute to profits from the enterprise. In response, efforts could be made to ensure that producers have support to find and install low-cost/cow systems. Further, sensitivity analysis for various levels of grant funding and higher electricity prices suggest that even substantial support for AD installation fails to generate positive simulated NPV of investment under average- and high-cost/cow systems. Thus, high levels of governmental support or policy initiatives would be required to reach a socially optimal market outcome.
Other work has shown that the structure of purchase agreements, the method by which dairies sell their excess power generated to the grid, can be significant in the profitability of the enterprise (Binkley et al., Reference Binkley, Harsh, Wolf, Safferman and Kirk2013). As previously stated, it is uncommon for dairies in Texas to sell excess energy as the price of electricity is low; however, if electricity as a coproduct does become feasible, the policy of sales will be important to the installation of AD technology. A large body of work (Holm-Nielsen, Seadi, and Oleskowicz-Popiel, Reference Holm-Nielsen, Seadi and Oleskowicz-Popiel2009; Jacobsen, Laugesen, and Dubgaard, Reference Jacobsen, Laugesen and Dubgaard2014; Nielsen and Hjort-Gregersen, Reference Nielsen and Hjort-Gregersen2002) evaluated centralized biogas plants and found economies of scale in operation and significant opportunity for profitability through decreased cost to individual dairies. This option could be a viable step in areas such as northern Texas where dairies are continuing to expand and grow in number.
Our study is the first to show the importance of including risk and variability in models specific to the decision of whether or not to install biogas collection and electricity generation equipment on dairies under low electricity prices. Extensions to this work could explore alternative coproducts and additional waste streams necessary for AD on dairies to be profitable in Texas. Additional research could also be devoted to determining if systems could be designed that support economies of scale. Others could also explore the social benefits of adopting AD or determining the cost and benefits to the state of Texas from providing grant assistance to install AD on dairies in lieu of other support to renewable energy projects.
Acknowledgements
The authors would like to thank Dr. James Richardson for his guidance and unrestricted access to his time as we learned new methodology to explore this topic. We would also like to thank the three anonymous reviewers who provided feedback that led to our final product.
Financial support
This research received no specific grant from any funding agency or commercial or not-for-profit sectors.
Conflicts of interest
None.
Appendix
Table A1. Estimates of number of head and total cost of anaerobic digester installation at AgSTAR-supported dairies
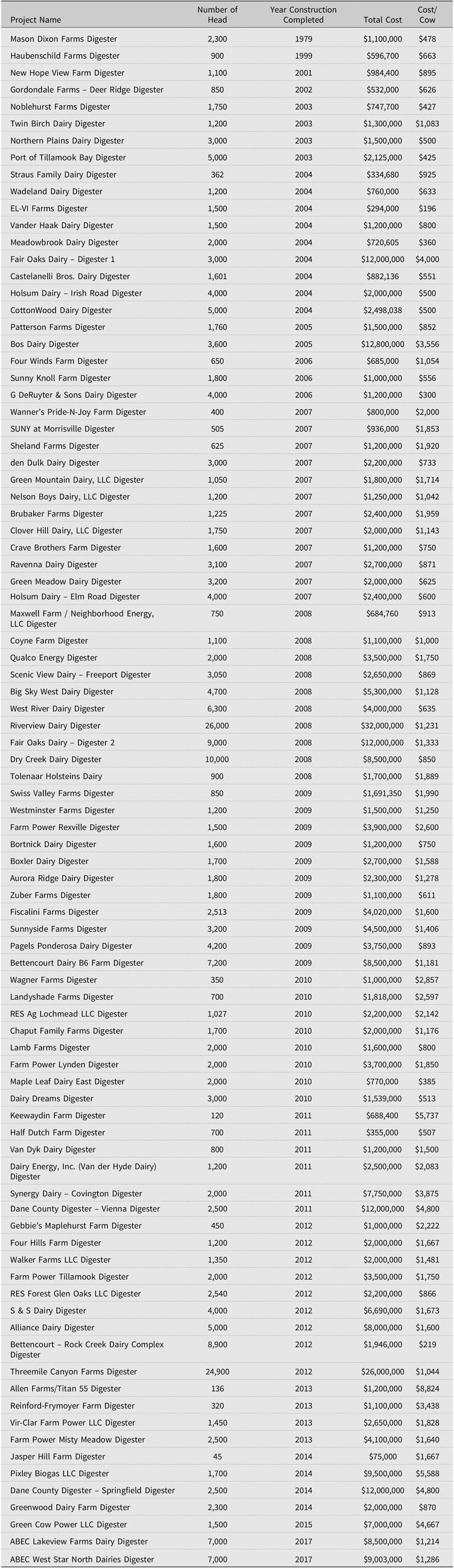