INTRODUCTION
Philosophers have argued that civility as a normative ideal is a requirement for democratic discourse. Apart from a type of behavior that displays good manners, character, courtesy, and self-control, civility in normative visions of theorists like Habermas and Rawls is deemed a virtue because it promotes respect and regard for others; it is “what enables the social order to exist, and therefore makes possible the very functioning of a city, state, or nation” (Herbst Reference Herbst2010, 33).Footnote 1 Yet others have argued that such accounts downplay the importance of fierce, unpredictable, and even uncivil disagreement as a core feature of democratic emancipation, especially for groups that have been historically marginalized and have used incivility to achieve freedom and equality (for a detailed discussion of this debate, see Bejan Reference Bejan2017, 9, but also Howard Reference Howard2019; Kennedy Reference Kennedy2001; Kramer Reference Kramer2022). These tensions about the role of civility in public discourse have become even more relevant in the context of social media. The presence—and, as of recently in some popular platforms, sharp increase (Miller Reference Miller2023)—of uncivil, intolerant, and violent content has led to fears that social media will prove detrimental not only to public discourse but also to democracy more generally. This leads to two critical questions: Should toxic content be moderated to maintain a civil public discourse? Or should such speech on social media be left unconstrained?
These questions acquire special significance in the context of the United States (US). Research by the Pew Research Center shows that there are growing levels of toxicity (of which incivility is but one dimension) across most social media platforms and roughly 4 in 10 Americans have experienced online harassment, including name calling, physical threats, and sexual abuse (Pew Research Center 2021a). Another study by Pew also shows that these and other types of harm befall disproportionately those more vulnerable (Pew Research Center 2017a). But while hate, harassment, and extremism motivate Americans to think that social media have negative effects on their country (Pew Research Center 2020b), their regulation is highly contested with multiple intertwined actors—tech companies, government, and non-governmental organizations—interacting within a very distinct legal and normative framework that renders many proposals to reform these platforms unconstitutional on First Amendment grounds (Caplan Reference Caplan2023; Chemerinsky and Chemerinsky Reference Chemerinsky, Chemerinsky, Bollinger and Stone2022; Gorwa Reference Gorwa2022).
The historical roots of that framework go as far back as 1927, and are encapsulated in Justice Brandeis’ much-quoted opinion in Whitney v. California that more speech is the antidote to harmful speech.Footnote 2 This view has been influential in contemporary rulings of the Supreme Court and has been supported by both liberal and conservative justices. Compared to countries where regulation aims to balance freedom of speech and protection from harm, the U.S. approach places significant emphasis on freedom of expression, thus limiting the extent to which the government can interfere (Adams et al. Reference Adams, Baron, Bollinger, Clinton, Cobb, Feingold, Paxson, Bollinger and Stone2022; Kohl Reference Kohl2022).Footnote 3 Nowhere is this better reflected than in Section 230 of the 1996 US Telecommunications Act, which shields platforms from liability for user-generated content while allowing them to moderate their spaces without becoming an official publisher (which would come with specific legal responsibilities; Gillespie Reference Gillespie2018, 30–1).
But what about the users, who—ultimately—are those who experience toxicity? Users still hold the power to report speech they consider inadmissible through different types of flagging mechanisms that can be used to alert platforms to objectionable content. As such, users can potentially play a critical role in the health of public discourse. If large numbers of users desire a healthy online environment, then platforms may be incentivized to moderate content. Understanding users’ voice on the matter, therefore, is critical for anticipating if there can ever be a critical mass to push—either through voice or exit (Hirschman Reference Hirschman1970)—for regulation. This motivates the fundamental question driving this study: how do users’ perceptions of toxic content align with their content moderation preferences for toxic speech?
Even if users’ perceptions of what constitutes harmful speech are in line with what some normative theorists have long praised as valuable for democracy, we know surprisingly little about how exposure to toxicity might translate into attitudinal and behavioral outcomes (for exceptions, see Druckman et al. Reference Druckman, Gubitz, Levendusky and Lloyd2019; Kim et al. Reference Kim, Guess, Nyhan and Reifler2021; Munger Reference Munger2017). In particular, while there is some research on user moderation preferences in the context of exposure to misinformation (Appel, Pan, and Roberts Reference Appel, Pan and Roberts2023; Kozyreva et al. Reference Kozyreva, Herzog, Lewandowsky, Hertwig, Lorenz-Spreen, Leiser and Reifler2023), to the best of our knowledge, there are no empirical insights on how toxic content translates into content moderation preferences for toxic speech. The epistemic community and social media companies are broadly aligned in their understanding of what constitutes inadmissible speechFootnote 4 and what should be done with it. But it is much harder to infer what users want; surveys conducted by Pew show that this is a divisive issue (Pew Research Center 2016; 2020a; 2021b; 2022c). Do users’ views align with the normative ideals of the epistemic elite, platforms’ community standards, and their policies against toxic speech?
Past work has noted that there is insufficient understanding of how variations in incivility affect individuals (Druckman et al. Reference Druckman, Gubitz, Levendusky and Lloyd2019). We contribute to filling this gap in empirical work by designing two studies (Study 1,
$ N=\mathrm{5,130} $
; Study 2,
$ N=\mathrm{3,734} $
) with a series of experiments exposing U.S. participants to different manifestations of toxic speech following the various ways in which it is theorized and empirically considered in the existing literature as well as taking into account a variety of groups being targeted with such speech. Building on research in political science and communication, we conceptualize toxic content in terms of incivility (Druckman et al. Reference Druckman, Gubitz, Levendusky and Lloyd2019; Gervais Reference Gervais2015; Jamieson et al. Reference Jamieson, Volinsky, Weitz, Kenski, Kenski and Jamieson2017; Kenski, Coe, and Rains Reference Kenski, Coe and Rains2020; Muddiman Reference Muddiman2017; Mutz Reference Mutz2007; Mutz and Reeves Reference Mutz and Reeves2005; Sydnor Reference Sydnor2018), intolerance (Rossini Reference Rossini2022; Siegel et al. Reference Siegel, Nagler, Bonneau and Tucker2021), and violent threats (Kalmoe and Mason Reference Kalmoe and Mason2022; Kim Reference Kim2023). To ensure that our findings are not situational and because theoretical arguments anticipate that toxicity offers a powerful way to differentiate ingroups from outgroups (Druckman et al. Reference Druckman, Gubitz, Levendusky and Lloyd2019; Gervais Reference Gervais2015; Mason Reference Mason2018), we exposed participants to different scenarios where they see toxic speech directed at either people of different socioeconomic, religious, sexual orientation or different partisan groups.
The picture we draw is one where there is limited demand for action against toxic speech in general. It requires violent threats toward minority groups to see a narrow majority of users demanding the removal of a toxic post or suspending the account of the aggressor while, across targets, the overwhelming majority of users exposed to incivility or intolerance express dramatically low support for moderating content.
MOTIVATION AND CONCEPTUAL FRAMEWORK
For many theorists, civility has been discussed as a set of social and cultural norms and a virtue of social life that is associated with etiquette or good manners, and which is in line with socially established rules of respect, tolerance, and considerateness (Calhoun Reference Calhoun2000). Within this school of thought, civility is important because, among other things, it implies a willingness to listen to others and try to see things from the point of view of their conception of the good (Habermas Reference Habermas1990; Rawls Reference Rawls1971, 337–8), thus driving well-behaved, respectful, and rational discussion. However, Aikin and Talisse (Reference Aikin and Talisse2020, 17) are careful to note that civility does not necessarily mean that one must always maintain etiquette, and thus a gentle, polite, and pacifying tone that pushes away what is essential for disagreement. Indeed, as both Herbst (Reference Herbst2010, 9) and Papacharissi (Reference Papacharissi2004, 266) outline in their reviews of debates on incivility, some philosophers have actually advanced forms of incivility as an essential element for democratic emancipation and the pursuit of justice. These accounts hint at the idea that certain normative understandings of what is civil and admissible to public discourse may be too connected to existing elite power structures, and thus to the more articulate and powerful. This means that some elite understandings of civility may be at odds with the individuality, uniqueness, antagonism, lack of restrain, and impoliteness that characterizes much of the speech citizens are normally used to in their everyday lives—especially on social media.Footnote 5
Modern approaches that are influenced by the affordances of the changing political information environment have led scholars to propose some red lines about when speech stops being merely impolite and crosses the border to incivility. According to Papacharissi (Reference Papacharissi2004, 267), for example, while rude, poor manners that do not abide by etiquette are not necessarily uncivil, speech involving attacks on specific social groups is what sets democratic society back. The fundamental normative idea behind this position is that civility must be linked to respect for the political equality of opponents which citizens must be committed to in any discussion (Aikin and Talisse Reference Aikin and Talisse2020, 17). An attack, thus, on someone based on their membership in a particular social group (race, ethnicity, national origin, etc.) violates political equality and crosses an important line.
This understanding of (in)civility provides, in our reading, the normative basis for the community standards and content moderation rules of the most popular social media sites. Facebook, for example, does not allow attacks against people on the basis of their “race, ethnicity, national origin, disability, religious affiliation, caste, sexual orientation, sex, gender identity, and serious disease,” including “violent or dehumanizing speech [..] and calls for exclusion or segregation” (Facebook Community Standards 2022). Twitter has almost the exact same rules in place (see their policy on hateful conduct). Attacking people based on their protected characteristics, to use Facebook’s terminology, is inadmissible to public discourse on these platforms.
Regardless of where one draws the line normatively, there is general agreement that toxic speech has become more quantitatively apparent in public discourse, to a large degree because of the (social) media (Coe, Kenski, and Rains Reference Coe, Kenski and Rains2014; Frimer et al. Reference Frimer, Aujla, Feinberg, Skitka, Aquino, Eichstaedt and Willer2022; Herbst Reference Herbst2010; Sobieraj and Berry Reference Sobieraj and Berry2011). So much is this the case that already in the early 2000s scholars were commenting on the daily lamentation on the “crisis of civility” (Boatright et al. Reference Boatright, Shaffer, Sobieraj and Goldthwaite Young2019; Calhoun Reference Calhoun2000), with Time magazine devoting a 2016 cover to “why we’re losing the internet to the culture of hate.”
Literature on the effects of incivility and other forms of toxic speech generally reports negative consequences but is not conclusive. Many studies have shown that incivility and intolerance (in various facets, such as ethnic and racial) are linked to a number of serious psychological and social outcomes, such as boosting aggressiveness, retaliation, reduction of cognitive processing, productivity, and creativity, inducing polarization, feelings of anger and aversion, dissatisfaction with political institutions, and negative attitudes toward politicians, damaging credible information, escalation of nasty talk, close-mindedness, and the marginalization of, and spreading fear to, minorities and marginalized groups (Anderson et al. Reference Anderson, Brossard, Scheufele, Xenos and Ladwig2014; Andersson and Pearson Reference Andersson and Pearson1999; Druckman et al. Reference Druckman, Gubitz, Levendusky and Lloyd2019; Gervais Reference Gervais2015; Massaro and Stryker Reference Massaro and Stryker2012; Mutz and Reeves Reference Mutz and Reeves2005; Searles, Spencer, and Duru Reference Searles, Spencer and Duru2020). Violent (political) rhetoric has also been found to induce polarization and can be a precursor of actual violence and stimulate hateful behavior (Kim Reference Kim2023). At the same time, incivility is emotionally arousing (Mutz Reference Mutz2007) and, when it comes to witnessing it, it has been shown to have positive political outcomes, such as sometimes being able to mobilize citizens and increase their interest in politics (Berry and Sobieraj Reference Berry and Sobieraj2013; Brooks and Geer Reference Brooks and Geer2007), induce enthusiasm, a politically mobilizing emotion (Kosmidis and Theocharis Reference Kosmidis and Theocharis2020), and even increasing perceptions of the credibility of certain information (Borah Reference Borah2013; Thorson, Vraga, and Ekdale Reference Thorson, Vraga and Ekdale2010). Others, finally, conclude that “a general ban of incivility on platforms may constrain socially beneficial uses of incivility and cede more power to the already powerful” (Chen et al. Reference Chen, Muddiman, Wilner, Pariser and Stroud2019, 3).
While incivility as a form of toxic speech has received significant and long-standing attention in existing literature, speech with the potential to harm is referred to in many different ways in academic scholarship, legal texts, and the press, including terms like “toxic,” “uncivil,” “harmful,” and “anti-normative.” In this study, we deal with the three types of speech whose negative consequences for public discourse we reviewed above, and which have been conceptualized and measured in the literature: incivility, intolerance, and violent threats. When we do not refer to one of those concepts explicitly but rather more broadly to speech with the potential to harm, we refer to “toxic speech.” As others have suggested (Tirrell Reference Tirrell2017), we use this medical conception of speech to highlight that all three types mentioned above engender a mechanism by which speech can inflict harm, but also to account for damage variation given that potential toxic effects might vary in the extent to which they cause harm depending on the target—or those who witness such speech. While in this article we use the term toxic language to describe social media posts that exhibit the above three dimensions, we do acknowledge that toxicity can still be perceived in a subjective manner and it is not exclusive to speech.Footnote 6
Political science and communication scholars have used many different conceptualizations and operationalizations of toxicity. We do not summarize those here as we are in agreement with Herbst who, arguing about the conceptual complexity around the term incivility, notes that scholars have chosen “to orient their work around definitions that make sense for the level and nature of their theoretical or empirical work” (Herbst Reference Herbst2010, 12). We do, however, discuss the three main manifestations of toxic speech around which much of existing scholarship has been oriented, as well as the ways in which they have been measured.
Defining and Measuring Toxic Speech
Incivility
As the above discussion makes clear, incivility is the prime manifestation of toxic speech studied by political scientists. Incivility has been considered as including anything from an unnecessarily disrespectful tone (Coe, Kenski, and Rains Reference Coe, Kenski and Rains2014, 660) and lack of respect (Mutz and Reeves Reference Mutz and Reeves2005, 5) to rudeness and inconsiderate language (Phillips and Smith Reference Phillips and Smith2003, 85). Past work has relied on different operationalizations, depending also on the medium under investigation. Analyzing newspaper discussion forums, for example, Rains et al. (Reference Rains, Kenski, Coe and Harwood2017) and Coe, Kenski, and Rains (Reference Coe, Kenski and Rains2014) used manual coding to classify five manifestations of incivility: name calling, aspersion, lying, vulgarity, and pejorative speech. Studying citizen incivility aimed at politicians, Theocharis et al. (Reference Theocharis, Barberá, Fazekas, Popa and Parnet2016) used human-annotated data to train a machine-learning classifier that labeled as uncivil speech ill mannered, disrespectful, or offensive language. Their list of top predictive n-grams almost exclusively consisted of profanity and swearwords (e.g., c**t, f**k, twat, stupid, shit, w****r, scumbag, and moron) and racist attacks including the n-word. Using a BERT-based neural classifier as well as a logistic-regression-based classifier trained on manually annotated and artificially labeled data from Reddit and Twitter, Davidson, Sun, and Wojcieszak (Reference Davidson, Sun and Wojcieszak2020, 97) created an incivility classifier relying on name-calling, mean-spirited, or disparaging words directed at (groups of) people, ideas, plans, policies, or behaviors, pejorative or disparaging remarks about the way in which a person communicates and vulgar or profane language. Finally, measuring the rise of incivility among American politicians on Twitter, Frimer et al. (Reference Frimer, Aujla, Feinberg, Skitka, Aquino, Eichstaedt and Willer2022) used Google’s Perspective API toxicity index, which scores text for the level of incivility on a continuous scale from 0 to 100. Other approaches have included calculating the average number of offensive words included in, for example, a tweet to come up with offensiveness scores for those using such speech (Munger Reference Munger2017).
What these operationalizations and measurement strategies make clear is that, besides the n-word which constitutes racist speech, most types of incivility captured by these studies consist predominantly of unkind words, profanity, insults, and swearwords and less so by clearly distinguishable attacks on specific social groups, or phrases that undermine them and disparage their political equality and protected characteristics. In this sense, normatively speaking, much of this language might be considered as not—or at least not always—necessarily crossing the border of what is admissible in public discourse and worth content moderation.
Intolerance
The second manifestation of toxicity, which undoubtedly crosses the line of civility, is intolerant speech. Intolerance differs from incivility in that it aims to derogate, silence, or undermine particular groups due to their protected characteristics, attack their rights, and incite violence and harm. According to Rossini (Reference Rossini2022), incivility and intolerance should be treated as distinct concepts because the second not only normatively violates political equality as per the accounts discussed above, but, empirically, it is found in different contexts than the first, such as in homogenous discussions about minorities. Intolerant behavior toward particular social groups is what harms democracy the most (Papacharissi Reference Papacharissi2004; Rossini Reference Rossini2022) and, as Rossini notes, does not necessarily have to even be uncivil. Intolerance is a multidimensional concept (Bianchi et al. Reference Bianchi, Hills, Rossini, Hovy, Tromble and Tintarev2022), and in past work has been measured as harassing and discriminatory speech intended toward people or groups based on personal characteristics, preferences, social status, and beliefs, as well as the denial of their individual liberties and participation in the public sphere (Rossini Reference Rossini2022, 411). Measurement strategies for racist intolerance have included looking at the presence of the n-word in tweets aimed at other users (Munger Reference Munger2017). Other work, focused on political intolerance, uses a two-step classification, first labeling content based on whether it is relevant to civil liberties and subsequently classifying relevant content as intolerant (e.g., supporting restricting civil liberties, limiting the right to free speech, protest, and assembly) or not (Siegel et al. Reference Siegel, Nagler, Bonneau and Tucker2021). Overall, manifestations of intolerance in this line of research, which also involves intolerant rhetoric by elites (Gervais Reference Gervais2021), include xenophobic, homophobic, racist, and religious intolerant remarks as well as violent threats.
Violent Threats
Violent threats have also been classified as a specimen of intolerant behavior (or as accompanying intolerant rhetoric) (Rossini Reference Rossini2022). However, the act of violently threatening another person lies, in our view, at a different level of toxic behavior because it explicitly announces the intention of physical harm. It is also a form of toxic speech that is widely and unambiguously perceived as constituting online harassment by the majority of Americans, according to a study by the Pew Research Center (2021). We thus distinguish violent threats as the third manifestation of toxic behavior. Our understanding of violent threats as a separate category is not only in line with the policies of some platforms which explicitly classify this behavior as out of bounds (see, e.g., Twitter’s Violent threats policyFootnote 7 or Violent speech policyFootnote 8). Threatening someone can also be a serious criminal offense (Howard Reference Howard2019, 101), falling in some U.S. states under the category of assault with penalties as severe as jail time. While this dimension of toxic behavior has received less attention in the literature (for exceptions on research in political violence, see Kalmoe and Mason Reference Kalmoe and Mason2022; Kim Reference Kim2023), there are indications that certain groups, such as female political candidates, tend to be disproportionately targeted online in this way (Guerin and Maharasingam-Shah Reference Guerin and Maharasingam-Shah2020).
Few studies have focused on individuals who threaten others on social media and even fewer on the effects of witnessing this type of behavior. In a study focusing on violent threats toward political opponents, Kim (Reference Kim2023, 8) defines political violence as “rhetoric expressing the intention of severe physical harm against political opponents” and violent threats are measured through a dictionary of violent political words along with additional manual labeling for difficult cases.
Empirical Expectations
While the above discussion makes clear that being at the receiving end of toxic behavior has a wide variety of negative consequences, as Jamieson et al. (Reference Jamieson, Volinsky, Weitz, Kenski, Kenski and Jamieson2017, 208) note in their review of psychological effects of incivility, the effects of witnessing this behavior are less settled. Yet it is precisely these effects on individuals that are of critical importance for understanding users’ content moderation preferences for toxic speech. First, studying these effects can reveal users’ willingness to demand regulation for online public spaces in ways that are meaningful to them and in line with their democratic values. Second, in line with the third-person effect theory which suggests that individuals will perceive media messages to have greater effects on other people than on themselves (Davison Reference Davison1983), exposure to toxic behavior may act for them as a heuristic for evaluating the health of the broader discourse, the magnitude of unpopular behavior by others around them, and their own position, pushing them to adjust their own behavior and preferences accordingly.Footnote 9
Establishing the effects of witnessing toxic behavior on content moderation preferences is a difficult task first and foremost because users hardly agree on what constitutes toxic speech that needs moderation (Jhaver et al. Reference Jhaver, Ghoshal, Bruckman and Gilbert2018) and because different types of toxic behavior may be perceived differently and elicit different responses (Gervais Reference Gervais2015; Kenski, Coe, and Rains Reference Kenski, Coe and Rains2020). Moreover, survey findings suggest that individuals may have very different attitudes toward toxic speech and platform regulation depending on their partisanship (Pew Research Center 2019a; 2020a; 2022b). As Rains et al. (Reference Rains, Kenski, Coe and Harwood2017, 166) put it, “When an outgroup is attacked, the norms for ingroup behavior shift to a mode of intergroup conflict and, as a result, partisans will be more likely to conform to the new norm and be uncivil.” Previous studies have found systematic variation in perceptions of incivility based on the identity of those targeted by uncivil speech. Gubitz (Reference Gubitz2022, 612), for example, using a conjoint survey experiment, showed “that White Americans are more likely to view statements directed at Black Americans as uncivil, and also more likely to perceive incivility when the target is a woman or a copartisan.” Further empirical evidence along this line suggests that people tend to perceive political figures from their own party as more civil than others (Muddiman Reference Muddiman2017) and that strong levels of incivility decrease perceptions of how rational one’s political outgroup is in a discussion (Popan et al. Reference Popan, Coursey, Acosta and Kenworthy2019).
Here, we are interested in how users respond when exposed to toxic speech, specifically in sanctioning others based on available types of content moderation. Content moderation strategies vary from “mild” tactics that algorithmically reduce the visibility of content in people’s feeds and give users the opportunity to flag content (hence still leaving the content online), to more severe tactics like deleting content and banning users. The three toxic behaviors we have outlined embed different levels of severity, with violent threats being obviously a far more serious offense than, for example, calling people names or using profanity. But whether these toxic behaviors are linked in a linear way with more severe types of content moderation for those exposed to them remains unclear.
Research has repeatedly shown that these behaviors have a string of negative effects on their victims but also on bystanders. As intolerant and threatening behavior are linked to particularly harmful outcomes, it is reasonable to assume from existing work that these two types of toxic speech would be linked to the strongest available types of content moderation. In other words, toxic speech with the gravest consequences will, theoretically, be linked to content moderation options that restrict such behavior most firmly.
Testing rival theories about how different types of toxic speech might be linked to content moderation is beyond the scope of this study. Nevertheless, it is important to note that there are at least two arguments that can be marshaled not just to dispute the expectation that starker forms of toxic speech would lead to more severe content moderation, but to even raise doubts that for some people moderation is a pressing issue to start with. The first argument, based on considerable research on groups affected by harmful speech, refers to the normalization of toxicity and suggests that groups often targeted by this behavior have learned to cope with it and do not bother to report it (Chadha et al. Reference Chadha, Steiner, Vitak and Ashktorab2020; Krook Reference Krook2020). Studies on online video game toxicity, for example, reveal that “players abstain from reporting toxic content because they view it as acceptable, typical of games, as banter, or as not their concern” (Beres et al. Reference Beres, Frommel, Reid, Mandryk and Klarkowski2021).
The second argument for leaving toxic content unmoderated is in some ways tied to the American free speech paradigm and the First Amendment. According to this argument, people must live with the occasional harmful speech because the cost of regulating speech would be too high for democracy. This idea supports that people need to be exposed to a wide range of views, including especially speech that might be unpopular (for a discussion of a similar argument, see Kramer Reference Kramer2022). A preference to not have toxic content moderated, thus, may stem from the belief that such content needs to be there to help users understand the types of views others have or engage in counter-speech to eliminate harm.
RESEARCH DESIGN AND METHODOLOGY
To measure the causal effects of toxic speech on users’ content moderation preferences for toxic speech, we randomly exposed people to social media posts. We opted for a design that contains incivility, intolerance, or violent threats as separate treatments (see Tables S1 (Study I) and S14 (Study II) in the SM for the exact wording). While the strategy of differentiating toxicity is similar to past work on incivility by Druckman et al. (Reference Druckman, Gubitz, Levendusky and Lloyd2019), where they introduced variations of (un)civil speech in treatments to distinguish in-/out-party (in)civility, our approach actually differentiates the type of toxic speech and examines in- and out-partisan dynamics with dedicated experiments based on partisan targets (see Study II below). These treatments were compared to a control group that expresses opposition to a particular group, but without including any kind of toxic speech. Moreover, and to make sure that we could also compare to a clear baseline, we also exposed a portion of our participants to a placebo group that contained no information about the targets. Finally, as toxicity is both context-dependent and in the eye of the beholder, we examined these effects across different targets representing three social groups in Study I and opposition partisans in Study II.
The three different operationalizations of toxicity make our approach more granular compared to approaches used in past work and which cluster “violations of politeness that include slurs, threats of harm, and disrespect” under the concept of incivility (Druckman et al. Reference Druckman, Gubitz, Levendusky and Lloyd2019; Muddiman Reference Muddiman2017). We note here that attacking a minority group—especially using violent threats—constitutes a violation of the terms of service for platforms like Facebook and Twitter. According to Twitter’s rules, for example, “you can’t state an intention to inflict violence on a specific person or group of people. We define intent to include statements like I will, I’m going to, […]; violations of this policy include, but are not limited to: […] threatening to seriously hurt someone.” Based on this, our threatening condition (“next time I see one of them I will punch them in the face”) would fully violate popular platforms’ community standards. Our treatments were inspired by real social media posts and we presented them as such to our respondents. Figure 1 visualizes the experimental setup using one of the targets as an example.

Figure 1. Experimental Treatment Groups
Note: Possible target groups are LGBTQ people, billionaires, and truck drivers with Christian bumper stickers (Study I). See the Supplementary Material for the exact wording.
Finally, our outcome variable measured users’ preferences over content moderation for toxic speech. After exposing our subjects to the treatment, we invited them to respond to the following prompt: “In your view, how should social media companies like Facebook and Twitter handle the post above?” Participants could choose between the following actions: “Leave it, do nothing” (1), “Place a warning label on the post” (2), “Reduce how many people can see the post” (3), “Permanently remove the post” (4), and “Suspend the person’s account” (5) (for details on question-wording, variables, and measurement, see Table S2 in the SM). As we have noted, we only focus on platform self-governance and we do not include options that would require government intervention.
Study I: Targeting Social Groups
Study I looks into three different social groups in three separate experiments. In the first experiment, a member of the LGBTQ community is targeted. While attitudes toward LGBTQ people became more positive in recent years and there is a cultural shift toward more acceptance of this group, there is still a considerable portion of Americans who harbor negative views (Fetner Reference Fetner2016). Several studies suggest a partisan divide in public opinion toward LGBTQ people, with Democrats taking a more positive stance. For example, according to several studies by the Pew Research Center, Democrats are far more likely than Republicans (75% vs. 44%) to favor same-sex marriage and say that greater acceptance of transgender people is good for society (Pew Research Center 2019d; 2022a). In the second experiment, the target is a driver of a pickup truck with visibly religious bumper stickers. The target in this experiment may evoke the image of a highly religious Christian, a white American who demographically typically belongs to, is favored by, and identifies with, the Republican Party. As indicated by another study by the Pew Research Center, Democrats and Republicans are sharply divided on the role of religion in society, with Republicans being far more likely to view churches and Christian organizations as a force for good and the decline of religion as a bad thing for society (Pew Research Center 2019c).
The target in the final experiment is billionaires. Billionaires as a target of toxicity in our study play a threefold role. First, it is not a social identity category and thus not likely to provoke as emotionally intense a response as the other two categories. Second, as the odds of anyone being close enough to an ultra-rich person are extremely low, billionaires act as a sort of control category that allows us to observe whether moderation preferences (especially in response to violent threats) are comparatively milder when directed at a target that would be impossible to engage with offline. Third, using billionaires as a target allows us to address dynamics pertaining to status divides and intergroup competition from the perspective of “envy up, scorn down” (Fiske Reference Fiske2010). Billionaires, clearly an upper-class target, can provoke the ire of those who perceive themselves as lower status and keen on “punching up.” The pickup truck driver, by contrast, allows us to observe effects among those keen on “punching down,” given that owners of such pickup trucks may be stereotypically viewed as belonging to the working class (Fischer and Mattson Reference Fischer and Mattson2009).
The experiments were fielded in July (LGBTQ and Billionaires) and October 2022 (Christians). We recruited between 1,300 and 2,000 U.S. adults for each study via the participant pool of the crowdsourcing platform Prolific (for details regarding the recruitment procedure, please see the relevant document in the APSR Dataverse; Pradel et al. Reference Pradel, Zilinsky, Kosmidis and Theocharis2024). We excluded, in line with our pre-registered exclusion criteria, participants who failed the attention check, and those who opted in the end for exclusion from the study, or who responded to the survey in less than 50 seconds. This leaves us with a final sample of 1,936 in the study focusing on LGBTQ, 1,860 in the study about billionaires, and 1,334 in the study focusing on highly religious Christians.Footnote 10 Our total sample size for Study I is 5,130. We obtained ethics approval from the Central University Research Ethics Committee of the University of Oxford and, finally, the study design was pre-registered before data collection.Footnote 11
Results
Before the presentation of our core results pertaining to content moderation for toxic speech, it is key to discuss how our respondents evaluated the treatments with respect to the three types of toxic speech. This serves two purposes; it is an important test for our design (as a form of a manipulation check), but it also shows how respondents evaluate content designed to convey the different types of toxic speech. After exposure to the treatment, we asked respondents to select which type of language they thought best described the language featured in the tweet. Respondents were given four concepts to rank order: civil, uncivil, intolerant, and threatening language. We used a randomized presentation of the concepts to avoid response ordering effects. We calculated the percentages of participants ranking one of the concepts first as their best description of the social media posts (versus those concepts that were not ranked first) for all experiments in Study I. In line with our expectations, our analysis in Table 1 shows that incivility was the concept ranked most often as first given an uncivil intervention,Footnote 12 and that intolerance and threatening language were those that were most often ranked first given an intolerant and threatening intervention respectively. In the placebo group, most respondents ranked first the item as civil; in the civil but anti-target group, the most frequently selected items for best fit were intolerant and civil.
Table 1. Participants’ Perceptions of the Underlying Toxic Language Dimension (Study I—Targeting Social Groups)

Note: The cells report percentages of participants who ranked as first one of the following features describing the post they were exposed to: (i) civil, (ii) uncivil, (iii) intolerant, and (iv) threatening. Ninety-five percent confidence intervals are reported in brackets (
$ N=\mathrm{5,130} $
). See row 3 of Table S2 in the SM for the exact wording.
Respondents’ evaluations show that they are able to associate features of uncivil, intolerant, and threatening speech well enough with each one of the concepts of toxic speech we are analyzing. They can also distinguish them from one another in ways similar to that in which they are conceptualized in academic literature. While we do not know whether especially incivility and intolerance hold for respondents the same normative currency they hold in broader epistemic debates (as discussed, intolerant speech is widely considered as normatively deplorable by the epistemic community), we consider this to be an important finding because regardless of their normative perceptions about the role of these types of speech in public discourse, respondents could clearly recognize their key features.
To estimate the effect of the experimental stimuli, that is, assignment to either an uncivil, intolerant, threatening social media post—or one of the two controls—on the preferences for taking action against a post, we estimate a logistic regression, pooling the data from the three studies. We use a binarized version of our outcome variable because our main quantity of interest is whether users support any form of action against the post (in Figure 3 of the next section, we also present the full distribution of respondents’ preferences, broken down by treatment and study). To estimate the pooled average treatment affect (ATE), we add indicator variables for the targets (studies) and condition on the respondent’s partisanship (the ATE is not affected by the inclusion of the partisan dummies). ATEs from this model are reported in the top-left panel of Figure 2. The baseline category for the treatment assignment is the anti-target post without any kind of toxic speech (e.g., in the experiment where LGBTQ people were targeted, it read “maybe i am old school but i think Hollywood should stick to making movies and stop pushing stories about gay couples”), and the coefficients displayed in black indicate effects relative to assignment to such a post. The omitted category for “target of the attack” is “Christian target,” and the coefficients displayed in green show the effects of viewing an attack against either a billionaire or a member of the LGBTQ community, relative to seeing a post about the highly religious Christian pickup truck driver.

Figure 2. Effects of Treatments on Support for Any Form of Content Moderation (Study I, Pooled Results and Estimates Broken Down by Target Group)
Note: The point estimates and the 95% confidence intervals represent average marginal effects calculated from a binary logit model. The dependent variable is set to 1 if the respondent selected any of “Permanently remove the post,” “Place a warning label on the post,” “Reduce how many people can see the post,” or “Suspend the person’s account” as their preferred action against the offending post.
$ N=\mathrm{5,130} $
(pooled data). The logit results can be found in the SM and are presented in Table S9 in the SM.

Figure 3. Preferences for Content Moderation by Treatment and by Experiment in Study I
Note: The bars show support for each type of action for the pooled model (upper left), the LGBTQ study (upper right), the Christian study (bottom left), and the Billionaires study (bottom right). The actual percentages are reported in Tables S7 and S8 in the SM.
Moving to our core results, we find that relative to the control group, we observe a 19.3 percentage point increase (95% CI: 16.0–22.6 p.p.) in the probability of support for taking action against the post when uncivil speech is introduced. Exposing respondents to intolerant speech, similarly, causes an approximately 19.2 percent point increase (95% CI: 15.9–22.5 p.p.) in support for content moderation.
The strongest effect for induced moderation preferences is observed when subjects view a post that includes threatening speech. When subjects are randomly assigned to read content that included a variation of “punch them in the face,” support for some (any) form of content moderation increases by 41.9 percentage points (95% CI: 38.5–45.3 p.p.). The effect is smaller when billionaires are targeted with violent threats (30 percentage points; 95% CI: 19.3–41.4 p.p.), slightly larger when the Christians are attacked (36 percentage points; 95% CI: 29.3–42.8 p.p.) and largest when LGBTQ people are targeted (nearly 56.7 percentage points; 95% CI: 56.3–57.1 p.p.), illustrating that the identity of the target matters for respondents’ evaluations of whether social media posts merit moderation.
Another way to quantify differences in the wishes to protect different targets is as follows: when a billionaire is attacked (in any way), average support for moderation stands at 21.4%. When a Christian is attacked (in any way), moderation rates were 36%. Demand for content moderation was highest when LGBTQ people were targeted (in any way): 58.2% of respondents stated that some action should be taken against the toxic post (or against the user).
Figure 3 offers an overview of which type of content moderation is chosen conditional upon treatment assignment and target. We find that about 11% of respondents want to remove both uncivil and intolerant social media posts targeting LGBTQ (see also Tables S7 and S8 in the SM for details on percentages). When the post is threatening, nearly 25% of respondents say that the post should be removed and an additional 27% say that the person’s account should be suspended. Overall, respondents in the threatening social media post condition opt for more stringent forms of moderation than those in the uncivil and intolerant group. In the experiment where billionaires were targeted, we see that across all treatment groups much fewer individuals opt for any form of moderation, and even fewer favor severe forms of moderation such as suspending an account—even when the post contains threats of physical violence (see Tables S7 and S8 in the SM for exact percentages).
We found that when the highly religious Christian pickup truck driver was targeted with threatening language, support for removing the post stood at approximately 8%. About 25% of those assigned to the threat experimental group supported placing a warning label on the post, 6% supported downranking the post, and approximately 6% indicated that the offender’s account merited a suspension.
A closer look at both Figures 2 and 3 reveals that while intolerance compared to incivility induces stronger demands for moderation in the LGBTQ study, we observe the opposite in the Billionaires experiment and no differences for the religious target. Recall from our literature review that this has been the theoretical prediction conceptualizing differences between intolerance and incivility (Papacharissi Reference Papacharissi2004; Rossini Reference Rossini2022): intolerance is perceived as more harmful because it attacks a person based on their protected characteristics. A similar argument can be made about the effect of violent threats that tend to trigger stricter content moderation preferences across all targets, but predominantly in the case of the LGBTQ target.
However, how strong are these demands for moderation? Figure 3 reveals that with the exception of the case of the LGBTQ target, across all studies large majorities do not support any kind of moderation—not even for the threatening condition—with banning a person’s account being the least preferred option across the board (again, excluding the LGBTQ case). This finding has important implications because Democrats, who are almost twice as likely to demand moderation than Republicans (Morning Consult 2023; see also our findings below), are overrepresented and thus a more balanced sample by partisanship would probably show narrower support for content moderation. Importantly, we analyzed the effect of age on moderation preferences to examine if the variation in our outcome variable is due to our relatively younger participant pool. We do not observe large differences between younger and older age groups (see Figure S1 in the SM) when demanding moderation, while older subjects seem to be fairly frequent users of social media (around 85% said they used social media recently) (see Figure S2 in the SM). We think these results give additional credence to this study’s conclusions about how users’ attitudes toward content moderation matter for anticipating if a critical mass to make platforms more responsive to the content they host could ever materialize.
Thus far, we have assumed that all respondents respond to treatments/targets in similar ways. And, indeed, across several characteristics (age, social media usage, etc.), we do not find any discernible differences. However, a key motivation behind choosing the targets we did, was to examine whether partisan groups might become more sensitive (and thus demand more moderation) when a group they feel warm to is the target of toxicity. As discussed earlier, Democrats might be more sensitive to the LGBTQ target, while Republicans might be more responsive to the Christian target.
The top-left panel of Figure 4 displays the differences in the predicted probability of demanding action between Democrats and Republicans that are exposed to the control group (anti-target without toxicity). The top estimate and the associated CIs correspond to the average difference between the two groups when exposed to the LGBTQ target suggesting that Democrats are 21% more likely to demand moderation compared to Republicans. The equivalent difference for the other two targets is close to 0, suggesting that both groups are equally likely to demand moderation (see the ATE analysis for the average estimates).
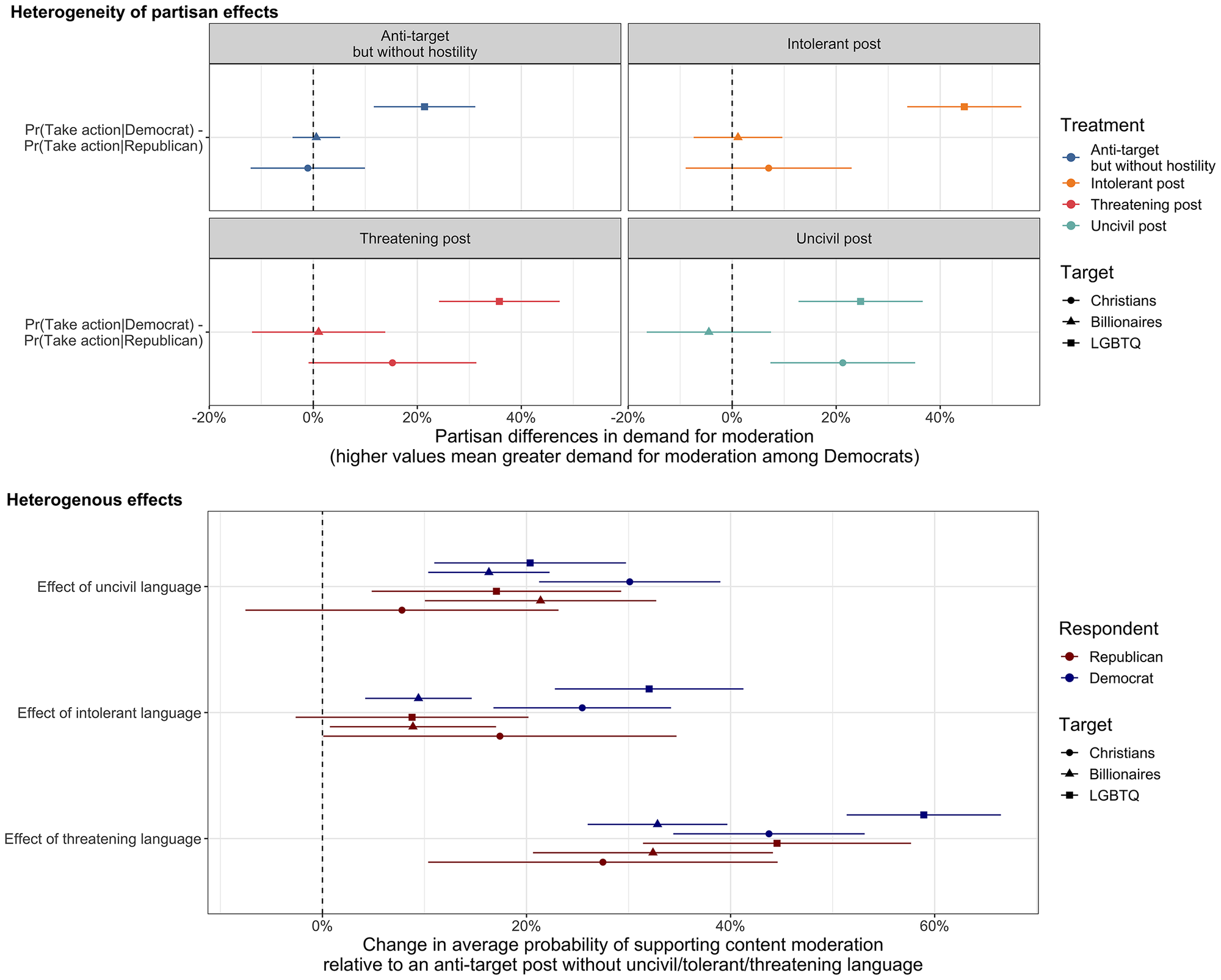
Figure 4. Heterogeneous Effects of Distinct Treatments on Support for Content Moderation
Note: Point estimates and the corresponding 95% confidence intervals of the top graph represent differences between Democrats and Republicans in the probability to demand content moderation by study and treatment. The bottom graph shows changes in the average probability for Democrats and Republicans when the treatments are compared to the anti-target control group. Results from the logit model can be found in the SM and are presented in Table S10 in the SM while predictions and contrasts are presented in Table S11 in the SM.
A very similar pattern is observed in the top-right plot, which shows partisan differences in predicted probabilities for those exposed to the intolerant treatment. Democrats, in this case, are 45% more likely to demand moderation in the LGBTQ study compared to Republicans, but, once again, there are no discernible differences when examining the other two targets. Importantly, the threatening and uncivil treatment groups that are displayed left and right in the second row of the graph show that, compared to Republicans, Democrats become more likely to demand moderation for both the Religious (uncivil
$ =21\% $
, threat
$ =15\% $
)Footnote 13 and the LGBTQ targets (uncivil
$ =25\% $
, threat
$ =36\% $
).
This means that while, as expected, Democrats are more protective of the LGBTQ community, they are also more protective of a social group the Republicans tend to identify with (i.e., the highly religious Christians). Note that, overall, Republicans still want some moderation (see the bottom panel of Figure 4), but Democrats are, on average, more sensitive to toxic content. This was also clear in the analysis we conducted for the ATEs across all targets.
Overall, respondents are able to distinguish between the treatments, and different dimensions of toxicity induce some demand for content moderation. However, we consider that, in most cases, the demand is modest at best. In other words, we do not see a critical mass emerging to request more platform intervention. Given these findings, we also considered whether a recent event might have caused a backlash making users more aware of what is at stake; the acquisition of Twitter by Elon Musk in October 2022. The acquisition, which came with a promise for “freedom of speech absolutism,” was prominent and was followed by concerns about moderation and the withdrawal of several advertisers from Twitter.Footnote 14 Given the vibrant discussion around it, it could have caused a backlash from some users who generally weigh protection from harmful content more heavily than freedom of speech. To examine whether the size of our effects had shifted in any way, we replicated the LGBTQ study in November 2022 (
$ N=\mathrm{1,200} $
), 2 weeks after Twitter’s acquisition by Musk. Our findings and conclusions remain unchanged. In the SM, we have a section dedicated to the post-Musk replication where we show the results and discuss further analysis that we performed.
Study II: Targeting Partisans
Study I confirmed that the target matters and affects the levels of demand for content moderation. It also showed that partisan groups might have distinct preferences over how platforms should treat toxic content. Our second study brings in an important empirical test for content moderation preferences for toxic speech; it invites respondents to report their desired platform response when partisans are attacked. On top of adding targets that are clearly political, this design brings another asset to our study: since we have both Democrats and Republicans in our sample, we can examine content moderation in a scenario where a sizeable proportion of respondents can identify with the victim or the perpetrator.
The research design in Study II is similar to the previous set of studies (varying incivility, intolerance, and threats while comparing them to a control and placebo group), but we now change the target to a Democrat (
$ N=\mathrm{1,877} $
) or a Republican (
$ N=\mathrm{1,857} $
) (for our preregistration, see https://aspredicted.org/LKB_N1R). To allow for valid comparisons, we conducted two parallel studies, one where a Republican is being attacked and one where a Democrat is the target.Footnote 15
As in Study I, we asked respondents the same questions about what best describes the post they witnessed and found a very similar pattern; most participants perceived civil as the best description of the social media post in the placebo group and in the anti-target group, and the majority of participants ranked the uncivil, intolerant, and threatening treatments as best fitting the corresponding interventions (see Table 2).
Table 2. Participants’ Perceptions of the Underlying Toxic Language Dimension (Study II—Targeting Partisans)

Note: The cells report percentages of participants who ranked as first one of the following features describing the post they were exposed to: (i) civil, (ii) uncivil, (iii) intolerant, and (iv) threatening. Ninety-five percent confidence intervals are reported in brackets (
$ N=\mathrm{3,734} $
). See row 3 of Table S2 in the SM for the exact wording.
When analyzing the ATEs, the picture we draw is similar to the one in Study I: intolerant or uncivil speech causes a 21–22 percentage point increase in demand for content moderation compared to the control group(s), and violent threats are the biggest driver of stricter moderation preferences, causing a 52 percentage point increase in support for post moderation (see the top panel of Figure 5; see Figure 6 for specific types of desired moderation).

Figure 5. Average (Top) and Heterogeneous (Bottom) Treatment Effects on Support for Any Form of Content Moderation (Study II)
Note: The top panel shows average marginal effects and the 95% confidence intervals (CIs). The bottom panel splits the estimates by partisanship. Full results of the logit models can be found in Tables S16 (upper panel) and S17 (lower panel) in the SM. Table S18 in the SM reports the predicted probabilities and 95% CIs for both graphs.
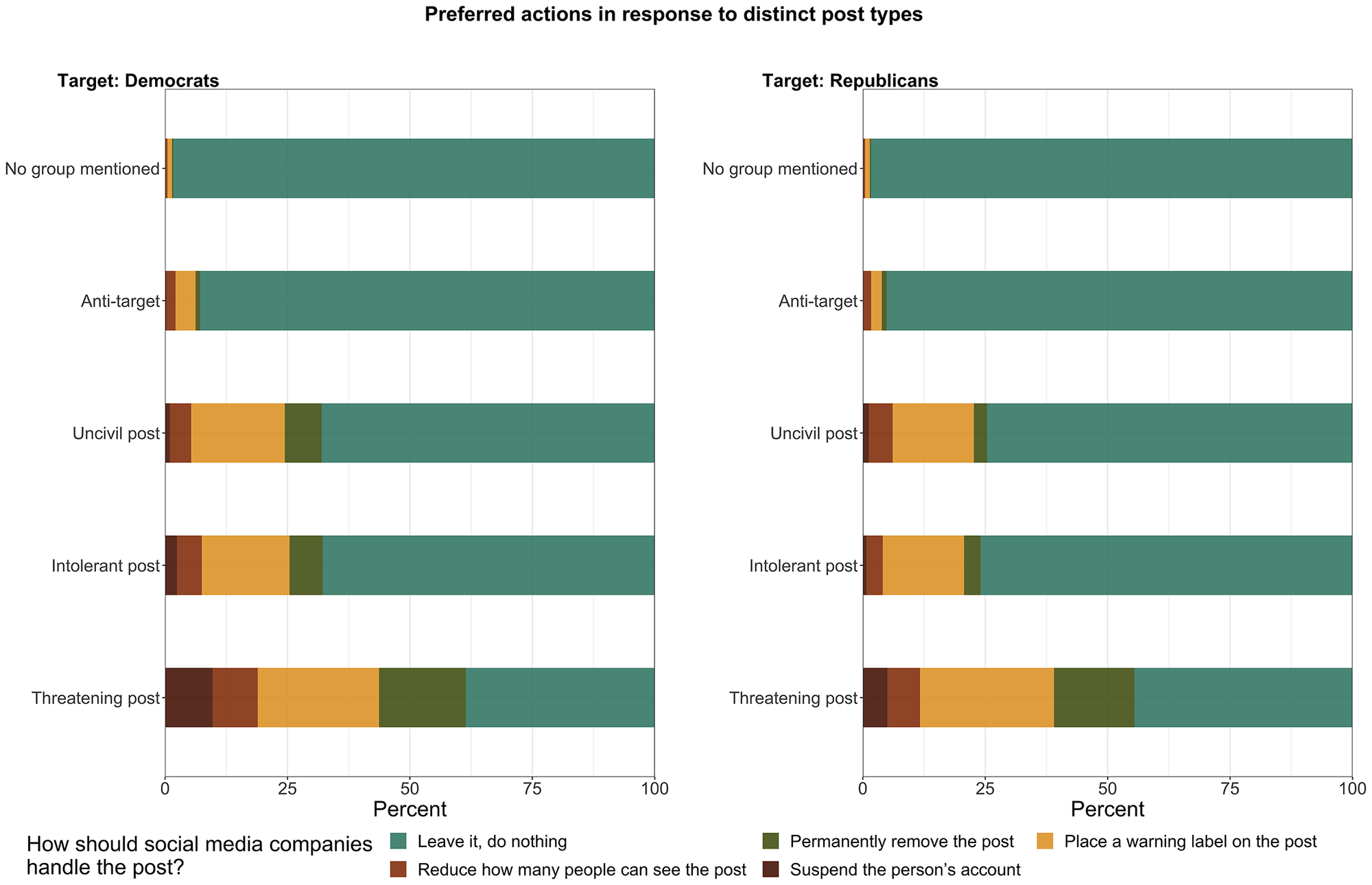
Figure 6. Preferences for Specific Types of Content Moderation by Treatment in Study II
Note: The bars show support for each type of action for the Democrat target study (left) and for the Republican target study (right). The actual percentages are reported in Tables S19 and S20 in the SM.
These findings give rise to an important question: is partisanship working as a perceptual screen that colors moderation preferences? Particularly, are Democrats (Republicans) more lenient when exposed to a Democrat (Republican) attacking a Republican (Democrat), and more sensitive when one of their ingroup members is under attack? Reversely, should we expect more demands for moderation when a co-partisan is the victim of toxicity?
While one could expect more sensitivity from our respondents when co-partisans are under attack, the bottom panel of Figure 5 shows that when a Democrat is targeted, Democrats do not demand significantly more moderation; conversely, Republicans do not support more moderation when their co-partisan is attacked. When we calculate average marginal effects from a model where the treatment is interacted with both the target and the party ID of respondents (bottom panel of Figure 5), we do not observe large partisan distortions to respondents’ reasoning about what constitutes inadmissible content. However, when we look at the overall preferences for moderation irrespective of the treatment (i.e., when we collapse our experimental groups into a single one), we do note the following: (1) Democrats compared to Republicans are around 10% (95% CI: 4.9%–16.5%) more likely to demand moderation; yet they are also 6.3% (95% CI: 0.7%–12%) more likely to demand moderation when a Republican is targeted. This finding is in line with what we found in Study I, where Democrats were more likely to demand moderation when the religious driver was under attack, and it also confirms studies that have examined partisan differences in content moderation and show similar patterns (Appel, Pan, and Roberts Reference Appel, Pan and Roberts2023).
To conclude, in Study II, our respondents—even if they identify with the victim or the perpetrator—are equally likely to demand moderation across different levels of toxicity, though some ingroup favoritism among Democrats is evident. Yet it is not symmetrical for Republicans and it is primarily driven by the more toxic treatments we used. Finally, and in line with the key finding of both studies, demand for any kind of action is narrow and it only moves when respondents are exposed to content that would be against the rules of the platforms themselves.
DISCUSSION
No achievement of social media has generated more praise and concern among academics, legal scholars, and policymakers than the capacity of these platforms to democratize the ability to reach a mass audience (Bollinger and Stone Reference Bollinger and Stone2022). But along with this expansion in the capacity for human expression, social media have also multiplied opportunities for toxic speech, with a new meta-analysis showing that their use is, indeed, associated with increases in hate-motivated behaviors and speech (Lorenz-Spreen et al. Reference Lorenz-Spreen, Oswald, Lewandowsky and Hertwig2023). While users may not actually enjoy seeing toxic speech, this does not automatically imply that they want this speech treated differently than other speech just because it does not conform to what the epistemic elite sees as inadmissible to public discourse. Taking this as our starting point, we developed a ladder of experimental treatments that represent different types of toxic speech—incivility, intolerance, and violent threats—and empirically connected them with another ladder of content moderation preferences for toxic speech. Our study yielded three important findings that bear consequences for political science and communication research. In what follows, we discuss the implications of our work along with possible avenues for future research.
First, we find that different types of toxic speech have different consequences. This diverges from much of past work on incivility that has focused mainly on manually or automatically labeled manifestations of the concept and has explored their prevalence in different types of written speech, such as user comments or tweets, yet without any reference to possible consequences they might instigate. We show that different types of toxic speech induce different user responses. We report evidence pointing to a new empirical insight that is tightly connected to current efforts to better conceptualize toxicity. While incivility, intolerance, and violent threats can indeed be thought of as a continuum, our analysis shows that users consider them as distinct constructs even though intolerance and incivility elicit similar content moderation responses. We suspect that the mechanisms behind users’ perceptions are in line with Rossini’s (Reference Rossini2022) assessment that incivility is a matter of tone—for example, foul language—while intolerance is a matter of substance—for example, discrimination.Footnote 16
Our second major finding is that support for content moderation of uncivil and intolerant content is generally low. The overwhelming majority of our respondents indicated that uncivil and intolerant content should be left online, with censorious forms of content moderation like banning users or removing content being among the least popular options in all studies. While the height of the benchmark for what is a sizeable demand for content moderation likely depends on one’s normative lens, this finding raises concerns. This is because, even if little support for content moderation for the uncivil treatment may not be very remarkable on the grounds that incivility—normatively speaking—is not a conversation stopper or necessarily poisonous for democratic discourse, the fact that 50% of our respondents did not want platforms to do anything with a post reading “no homos on TV or next to me or anywhere!” or “go elsewhere, your kind is not welcome here,” will trouble any advocate of civil discourse.Footnote 17 To be sure, other manifestations of intolerance (e.g., dehumanization; Bianchi et al. Reference Bianchi, Hills, Rossini, Hovy, Tromble and Tintarev2022) may be relevant and future work should consider treatments that move beyond ours that expose respondents to “language whose scope is discriminatory and/or exclusionary and/or derogatory” (see Rossini Reference Rossini2022, 404). Still, our results are clear and point to a limited demand for content moderation for toxic speech.
The only case where a large majority opts for content moderation when encountering toxic speech (and there, too, only about half of the respondents opt for the most severe options) is in the LGBTQ experiment, showing that the target matters. However, the type and number of groups we use here as targets is not exhaustive, nor is the language aimed at them nearly as appalling as much of what appears on the internet. Indeed, it is possible to imagine that racist, gender-based attacks or profanity against children would elicit stronger content moderation preferences. Yet it is also possible that the preferences of many users will be in line with Yishan Wong, former CEO of Reddit, who, following criticism in 2012 over sections where users shared images of (among other things) underaged girls, told the site’s moderators that legal content should not be removed, even if “we find it odious or if we personally condemn it […] We stand for free speech. This means we are not going to ban distasteful subreddits” (BBC 2012). We believe that it is imperative to consider additional targets to get a better sense of how users respond to different groups (women, racial minorities, immigrants, politicians, etc.)—always, of course, within what is ethically feasible and safe for respondents. While the text and target of a toxic social media post are likely to be the primary factors in how users evaluate a post, content moderation research could also benefit from experiments that manipulate additional features of a toxic post. In this way, we may gain systematic insights into how, for example, subtly manipulated visibility (e.g., a high number of likes and shares) or inferred influence on others (e.g., the social status of the post’s source) are incorporated by users in their content moderation decisions.
Third, our tests for heterogeneity reveal an interesting pattern that deviates from studies interested in motivated information processing and reasoning. In an era of affective polarization—with social media being considered its key driver (Barrett, Hendrix, and Sims Reference Barrett, Hendrix and Sims2021; Kubin and von Sikorski Reference Kubin and von Sikorski2021)—we only find limited evidence that users see moderation of toxic speech through their partisan lenses. Across our experiments, including those that expose respondents to attacks toward outgroup partisans, respondents are very consistent in their views with Democrats being more likely—in general—to demand moderation. Importantly, partisans are not particularly influenced by the identity of the victim (Republican or Democrat) showing comparable sensitivity to respondents who were exposed to own party perpetrators. We believe that this finding opens up a new and important research puzzle. Is Americans’ strong belief in the value of freedom of speech driving the results? This question makes up a promising research agenda, and while some recent research has already started going in this direction (Kozyreva et al. Reference Kozyreva, Herzog, Lewandowsky, Hertwig, Lorenz-Spreen, Leiser and Reifler2023), conclusive answers cannot be provided without comparative evidence. Needless to say, we think that studying content moderation preferences in countries with a different legal framework and more balanced public views concerning protection from harm and freedom of speech could yield important, and possibly, unexpected insights—as a global study by the Pew Research Center (2015) indicates.
Finally, our study raises important questions about the future of platforms’ content moderation strategies. Is a “minimalist approach” by platforms that focuses on extreme, violent, and threatening content while allowing milder forms of toxicity justified? Should extreme and violent content be kept on the platform if users do not think it crosses the line? Or should platforms follow a higher normative standard that might be at odds with users’ perceptions of toxic content? Overall, Americans in our study report very low levels of support for content moderation when encountering toxic speech, and this has important repercussions for the health of public debate. In the face of a deteriorating public discourse on social media, users interested in the health of public conversations have few affordances with which to counter speech that they consider toxic and flagging content is one of the most powerful options. However, a minimalist approach to content moderation can have dire consequences. Repeated exposure to milder forms of toxic speech may lead users to further normalize such content resulting in severe negative consequences for those targeted. While a minimalist approach will probably be more in line with the majority of users’ demands and will not violate free speech rights, there is a risk that marginalized groups and victims of violent threats will not be able to express themselves and participate freely in the public discourse (Howard Reference Howard2019; see also Ananny Reference Ananny2018 for the debate regarding an individual’s right to speak over a public’s right to hear). Striking the right balance between free speech and protection from harm will remain a complex challenge for platforms that will require multi-layered solutions, some of which are already debated in the scholarship on platform governance (Gorwa, Binns, and Katzenbach Reference Gorwa, Binns and Katzenbach2020; Kettemann and Schulz Reference Kettemann and Schulz2023).
SUPPLEMENTARY MATERIAL
To view supplementary material for this article, please visit https://doi.org/10.1017/S000305542300134X.
DATA AVAILABILITY STATEMENT
Research documentation and data that support the findings of this study are openly available at the American Political Science Dataverse: https://doi.org/10.7910/DVN/PPVWIG.
ACKNOWLEDGMENTS
We thank Anna Wohlmann and Natalia Allmi for the research assistance and Olga Gasparyan, Patrícia Rossini, Cristian Vaccari, Christopher Wlezien, and Kirill Zhirkov for discussions and feedback at various conferences and meetings. Earlier versions of this manuscript have been presented at the 12th Annual Conference of the European Political Science Association (EPSA) 2022 in Prague, the 118th American Political Science Association’s (APSA) Annual Meeting & Exhibition in Montréal, the 2023 CSMaPP conference in Florence, the lecture series “Digitization and Society” at the WZB, the Social Data Science Interest Group lecture series of the Alan Turing Institute, the Oxford Political Psychology seminar, and the seminar series of the empirical political science group at the University of Duisburg-Essen; we thank participants for their helpful comments. The authors are especially grateful to Robert Gorwa, Daniel Kreiss, Yptach Lelkes, Kevin Munger, Molly Roberts, Nils Weidmann, James Tilley, Rachel Bernhard, and Magdalena Wojcieszak for providing detailed feedback and ideas.
FUNDING STATEMENT
This research was supported by a TUM Think Tank grant and approved by the Oxford University Institutional Review Board (Reference DPIR_C1A_22_011).
CONFLICT OF INTEREST
The authors declare no ethical issues or conflicts of interest in this research.
ETHICAL STANDARDS
The authors declare the human subjects research in this article was reviewed and approved by the Central University Research Ethics Committee (CUREC) of the University of Oxford (approval number: SSHDPIR_C1A_22_011). The authors affirm that this article adheres to the APSA’s Principles and Guidance on Human Subject Research. All participants provided informed consent and were debriefed at the end of the experiments. For more information about the recruitment process and the experimental procedure, please see the respective SM in the APSR Dataverse (Pradel et al. Reference Pradel, Zilinsky, Kosmidis and Theocharis2024).
Comments
No Comments have been published for this article.