Polycystic ovary syndrome (PCOS) is the most common endocrine disorder in women in the reproductive period(Reference Diamanti-Kandarakis, Kouli and Bergiele1). Because of its heterogeneity, elusiveness regarding aetiology and diagnostic difficulties, PCOS is a complex endocrine and metabolic condition. The key risk factors which affect CVD risk in PCOS with complex mechanisms are dyslipidaemia, increased oxidative stress (OS)(Reference Macut, Bjekic-Macut and Savic-Radojevic2) and chronic low-grade inflammation(Reference Repaci, Gambineri and Pasquali3).
Recent studies have shown that women with PCOS, especially obese patients, have a significantly altered lipid profile characterised by qualitative changes of LDL, in terms of increased small, dense LDL particles (sdLDL), hypertriacylglycerolaemia and reduced HDL-cholesterol concentrations(Reference Phelan, O‘Connor and Kyaw-Tun4). This so-called ‘atherogenic lipoprotein phenotype’ represents a form of dyslipidaemia that contributes to greater CVD risk(Reference Rizzo and Berneis5). Namely, sdLDL comprises LDL particles smaller than 25·5 nm, which are considered as the most atherogenic LDL subfractions. In addition, increased levels of oxidised LDL were confirmed in women with PCOS, suggesting that lipid metabolism in PCOS is associated with OS(Reference Macut, Damjanovic and Panidis6).
OS is defined as an imbalance between excessive formation of reactive oxygen species and reduced antioxidant defence capacity. The pathophysiological mechanism of OS contribution in PCOS development remains vague, but numerous studies have found that PCOS is associated with OS(Reference Gonzalez, Rote and Minium7,Reference Murri, Luque-Ramirez and Insenser8) . We recently assessed OS biomarkers and proposed the evaluation of OXY-score as a comprehensive measure of OS status in women with PCOS(Reference Perovic Blagojevic, Ignjatovic and Macut9).
HDL constitutes a heterogeneous group of particles(Reference Blanche, Gon and Forte10) and previous studies suggested a relationship between altered HDL subclasses distribution and CVD risk(Reference Mueller, Chang and Deng11). The present research of anti-atherogenic roles of HDL particles is focused on evaluation of their functionality in addition to HDL subclasses distribution. However, data on HDL particles distribution and functionality in PCOS are scarce.
The antioxidative/anti-inflammatory role of HDL particles depends on the presence of antioxidant enzymes. Paraoxonase 1 (PON1) is a circulating esterase and lactonase associated with apo A1 on HDL particles(Reference Mackness and Mackness12). Antioxidant activity of PON1 is achieved by hydrolysis of lipid peroxides in oxidised lipoproteins(Reference Vekic, Kotur-Stevuljevic and Jelic-Ivanovic13,Reference Aviram and Vaya14) . OS contributes to reducing PON1 activity by changing the redox status of free sulfhydryl groups of the protein molecule of this enzyme(Reference Rozenberg and Aviram15). It is also clearly established that PON1 preferentially associates with small HDL 3 particles and then shifts to larger HDL 2 subclasses during the course of HDL maturation in the reverse cholesterol transport process(Reference Gugliucci, Caccavello and Kotani16). However, different pathological conditions may affect HDL maturation and, consequently, the distribution of PON1 between HDL 2 and HDL 3 particles(Reference Miljkovic, Stefanovic and Vekic17).
Taking all these facts together, we hypothesised that dyslipidaemia, OS and inflammation could be related to pro-atherogenic changes in lipoprotein subclasses profiles in women with PCOS. Therefore, the aim of this study was to analyse the association of OS status with HDL and LDL particle size and subclasses, as well as with PON1 activity distribution on HDL subclasses in women with PCOS, especially regarding their obesity status.
Materials and methods
Subjects and ethics
In total, 115 PCOS patients, sixty normal weight women (mean BMI: 21·24 (sd 1·98) kg/m2) and fifty-five overweight or obese women (mean BMI: 32·26 (sd 5·4) kg/m2), aged between 29 and 39 years, were included in this study. The patients were recruited at the Clinic of Endocrinology, Diabetes and Metabolic Diseases, Clinical Centre of Serbia, Belgrade. PCOS was defined based on the revised Rotterdam Consensus Criteria (2003)(18). All patients were recruited during the early follicular phase of the menstrual cycle, or at any time if they had severe oligomenorrhea or amenorrhea.
Written informed consent was voluntary obtained from all subjects. The whole study was planned and conducted respecting the principles of the Declaration of Helsinki (Edinburgh, 2000). The study protocol was evaluated and approved by Ethics Committees of the Faculty of Medicine and Faculty of Pharmacy, University of Belgrade.
Anthropometric measurements
Measurements of body weight and height, waist and hip circumferences were carried out in the morning in a fasting state, and BMI and waist-to-hip ratio were calculated.
Biochemical analyses
Blood samples were collected in the morning after a 12 h fasting period in the follicular phase of the cycle (on the 2nd or the 3rd day). Plasma and serum were separated and stored until analyses.
Fasting serum glucose, total cholesterol, HDL-cholesterol, TAG and C-reactive protein (CRP) concentrations were determined using commercially available enzymatic and turbidimetric tests on Dimension RxL Max (Siemens Healthcare GmbH) analyser. The concentration of LDL-cholesterol was calculated by the Friedewald formula(Reference Warnick, Knopp and Fitzpatrick19).
Total oxidative status (TOS), advanced oxidation protein products (AOPP), malondialdehyde, prooxidant-antioxidant balance (PAB), total antioxidative status (TAS), superoxide dismutase (SOD) and PON1 were measured on ILab 300+ (Instrumentation Laboratory). For redox status parameters, the intra-assay and inter-assay coefficients of variance for all assays were below 4·3 and 8·2 %, respectively.
Plasma HDL and LDL particles were separated using a non-denaturing 3–31 % polyacrylamide gradient gel electrophoresis method(Reference Rainwater, Moore and Gamboa20). The HDL and LDL particle size determination and subclasses analyses were described in detail and published elsewhere(Reference Vekic, Topic and Zeljkovic21). In brief, the sizes of LDL and HDL particles were determined based on migration distance of the major peaks in densitometric profile, from calibration curve made from high molecular weight protein standards, carboxylated polystyrene microsphere beads and human plasma with two LDL subclasses of known diameters. Relative proportions of LDL and HDL subclasses were determined by analysing areas under the peaks that correspond to the known ranges of particle diameters: LDL I (27·2–28·5 nm), LDL II (25·5–27·2 nm), LDL III (24·2–25·5 nm), LDL IV (22·0–24·2 nm), HDL 2b (9·7–12·0 nm), HDL 2a (8·8–9·7 nm), HDL 3a (8·2–8·8 nm), HDL 3b (7·8–8·2 nm) and HDL 3c (7·2–7·8 nm). Based on LDL size, the patients were further classified as the carriers of LDL phenotype A (LDL diameter > 25·5 nm) or LDL phenotype B, characterised by a predominance of sdLDL particles (LDL diameter ≤ 25·5 nm)(Reference Vekic, Topic and Zeljkovic21). PON1 activity distribution on HDL subclasses was determined by the zymogram method according to Gugliucci et al.(Reference Gugliucci, Caccavello and Kotani16). The areas under the peaks of densitometric scans of the sample represent the relative proportion of PON1 activity in particular HDL subclass, as previously described(Reference Miljkovic, Stefanovic and Vekic17).
Statistical analysis
The data are shown as mean values and standard deviation for normally distributed variables or as median and interquartile range for non-normally distributed parameters. Differences among continuous variables were evaluated using Student’s t test for parametric data or Mann–Whitney U test for non-parametric data. Since age differences between overweight/obese and normal weight PCOS patients were significant, ANCOVA and Quade’s tests (non-parametric ANCOVA) were applied for further analysing of parametric and non-parametric data, respectively, with age as a covariate. Spearman’s correlation analysis was performed to assess the association between lipoprotein subclasses, OS parameters, PON1 activity on HDL subclasses and risk scores. To determine the Dyslipidaemia score, we standardised all the variables by calculating Z scores for LDL-cholesterol, TAG and HDL-cholesterol using mean and standard deviation calculated from healthy population data from our laboratory. Thereafter, the Dyslipidaemia score was calculated according to the equation: average of the standardised variables (LDL-cholesterol + TAG)/2 minus standardised HDL-cholesterol. The OXY-score was calculated by subtracting the Protective score from the Damage score using the equation given by Veglia et al. (Reference Veglia, Cighetti and De Franceschi22). The Protective score was calculated as an average of standardised antioxidant variables (TAS, SOD and PON1 activity) while the Damage score was calculated as an average of standardised prooxidant factors (AOPP, TOS, PAB and malondialdehyde). The Inflammation score was determined in both groups after standardisation of CRP as an acute phase protein. Dyslipidaemia-Oxy-Inflammation score was calculated as the sum of the Dyslipidaemia score, OXY-score and the Inflammation score. All variables with non-normal distributions were log-transformed before computation. To determine the predictors of LDL phenotype B in PCOS, univariate logistic regression analysis was performed. Dependent variable was LDL phenotype, risk group included PCOS patients with LDL B phenotype, while the patients with LDL A phenotype formed the reference group. As independent variables, age, BMI and those that were significantly different between the patients with LDL phenotypes A and B (TAG, TAS, SOD and PON1 activity on HDL 2b, HDL 3b and HDL 3c subclasses) were tested. Multivariate logistic regression analysis (backward selection) was applied to predictors which in univariate analysis reached P value equal to or less than 0·100 (TAG, TAS, SOD and PON activity on HDL 2b, HDL 3b and HDL 3c subclasses) in order to determine the best model consisted of independent predictors of LDL phenotype B. Statistical analysis was performed using the Statistical Package for the Social Sciences (version 22, SPSS Inc.). P value < 0·05 was considered statistically significant for all analyses.
Results
The demographic, biochemical and lipoprotein subclasses distribution data in normal weight and overweight or obese PCOS patients are presented in Table 1. As expected, normal weight PCOS patients had significantly lower BMI, waist circumference and waist-to-hip ratio compared with overweight or obese PCOS patients. Overweight/obese PCOS patients were older than their normal weight counterparts. Fasting glucose concentration was similar in both groups, but the levels of CRP were significantly higher in overweight or obese PCOS patients. The levels of total cholesterol, TAG and LDL-cholesterol were significantly higher, while HDL-cholesterol was significantly lower in overweight or obese (P < 0·001) PCOS patients and the differences remained significant after adjustment for age. No significant differences were found in LDL particle size and subclasses distribution between the two groups of PCOS patients. On the other hand, overweight or obese PCOS women had significantly lower HDL particle diameters (P < 0·05) and higher proportion of HDL 3a subclasses compared with normal weight PCOS patients (P < 0·05), even after adjustment for age.
Table 1. Demographic, biochemical data and lipoprotein subclasses distribution in PCOS subgroups (Mean values and standard deviations; median values and interquartile ranges; compared by Student’s t test.)
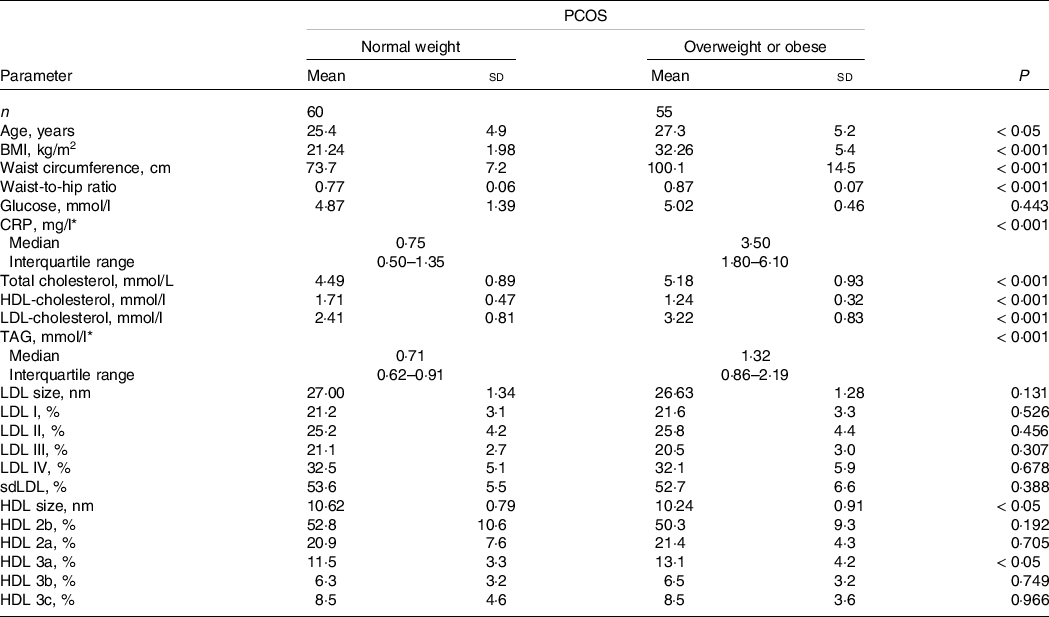
CRP, C reactive protein; sdLDL, small, dense low-density lipoprotein; PCOS, polycystic ovary syndrome.
* Data are presented as median (interquartile range) and compared by Mann–Whitney U test.
The characteristics of OS status in both PCOS groups are shown in Fig. 1. Significantly higher levels of prooxidant parameters (TOS and AOPP) were found in overweight or obese PCOS patients compared with normal weight PCOS patients. The observed differences between the two PCOS groups persisted after adjustment for age. We did not find significant differences in PON1 activity (Fig. 1) and PON1 distribution on HDL subclasses (data not shown) between normal weight and overweight or obese PCOS groups.

Fig. 1. Oxidative stress status in normal weight and overweight or obese PCOS patients. The box’s horizontal edges (lower and upper) indicate the 25th and 75th percentiles, respectively, and the horizontal line through the box indicates the median value. Horizontal lines at the lower and upper whiskers indicate minimum and maximum value, respectively. PCOS, polycystic ovary syndrome.
Table 2 shows the results of Spearman’s correlation analysis between lipoprotein data and parameters of OS status among all PCOS patients (overweight/obese and normal weight women). We found that the smaller LDL particle diameters were in correlation with higher levels of AOPP. Relative proportion of LDL I subclasses was positively associated with TAS and negatively with PAB. The proportion of small LDL IV subclasses was in positive correlation with PAB and in negative correlation with TAS. The level of PAB was in positive correlation with HDL 2b, while in negative correlation with the proportion of HDL 2a subclasses. A significant positive correlation was found between SOD activity and proportion of HDL 3b subclasses, as well as between concentration of malondialdehyde and proportion of HDL 3a subclasses.
Table 2. Correlations between lipoprotein subclasses and oxidative stress status in PCOS

TOS, total oxidant status; AOPP, advanced oxidation protein products; MDA, malondialdehyde; PAB, prooxidant antioxidant balance; TAS, total antioxidant status; SOD, superoxide dismutase; PON1, paraoxonase-1; PCOS, polycystic ovary syndrome.
Data are presented as Spearman’s correlation coefficients: *P < 0·05; **P < 0·01; ***P < 0·001.
Serum PON1 activity was positively associated with relative proportion of PON1 activity on HDL 2b (ρ = 0·204, P < 0·05) and HDL 2a (ρ = 0·335, P < 0·01), and negatively with the proportion on HDL 3a (ρ = -0·312, P < 0·01) and HDL 3b (ρ = -0·332, P < 0·01) subclasses. In addition, PON1 activity on HDL 3b particles positively correlated with TAS (ρ = 0·226, P < 0·05) and TOS (ρ = 0·207, P < 0·05), and negatively with PAB (ρ = -0·296, P < 0·01). PON1 activity on HDL 3c particles was in positive correlation with TOS (ρ = 0·207, P < 0·05).
To gain further insight in the interactive role of OS, dyslipidaemia and inflammation, risk scores were calculated. As shown in Fig. 2, all the scores, except Protective score, were higher in overweight or obese PCOS patients. Smaller LDL size was associated with higher Dyslipidaemia (ρ = -0·223, P < 0·05), Damage (ρ = -0·241, P < 0·05) and OXY (ρ = -0·213, P < 0·05) scores. An inverse association was found between HDL size and Dyslipidaemia score (ρ = -0·201, P < 0·05), as well as between PON1 activity on HDL 3 subclasses and Protective score (ρ = -0·228, P < 0·05).

Fig. 2. The scores of oxidative stress, dyslipidaemia and inflammation in normal weight and overweight or obese PCOS patients. The box’s horizontal edges (lower and upper) indicate the 25th and 75th percentiles, respectively, and the horizontal line through the box indicates the median value. Horizontal lines at the lower and upper whiskers indicate minimum and maximum value, respectively. PCOS, polycystic ovary syndrome.
Next, we assessed the impact of LDL phenotype on lipid, OS status and PON1 activity distribution on HDL subclasses in PCOS (Table 3). LDL phenotype B patients showed higher TAG concentration, but HDL-cholesterol concentrations and HDL size did not differ between the groups. The data also showed significantly higher levels of parameters of antioxidant protection (TAS and SOD) in LDL phenotype B group. PON1 activity on HDL 2b subclasses was significantly higher, whereas PON1 activity on HDL 3b and HDL 3c subclasses was significantly lower in women with LDL phenotype B. Serum PON1 activity was not different between PCOS patients with LDL A and B phenotypes.
Table 3. Lipid status, oxidative stress status and PON1 activity distribution on HDL subclasses in PCOS patients according to LDL phenotype (Mean values and standard deviations; median values and interquartile range)
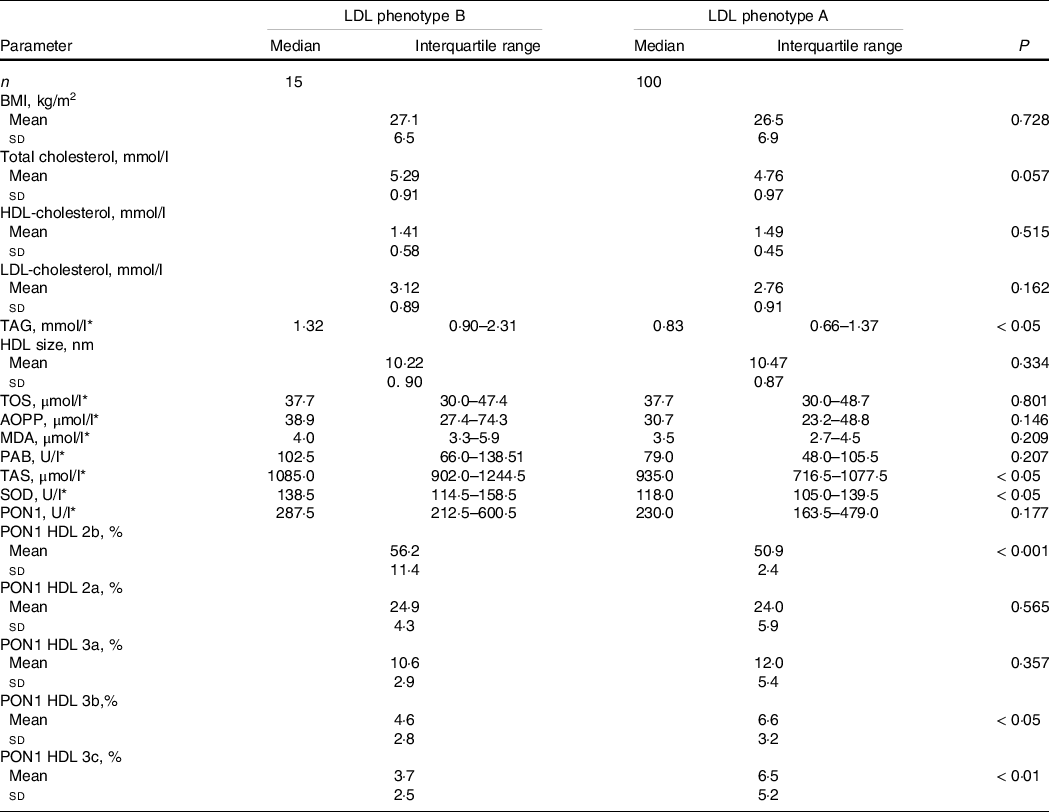
TOS, total oxidant status; AOPP, advanced oxidation protein products; MDA, malondialdehyde; PAB, prooxidant antioxidant balance; TAS, total antioxidant status; SOD, superoxide dismutase; PON1, paraoxonase-1.
* Data are presented as median (interquartile range) and compared by Mann–Whitney U test.
The concentration of TAG, activity of SOD in the serum and the proportion of PON1 activity on HDL 3b particles were indicated as significant predictors of LDL phenotype B (Table 4). In the multivariate analysis, PON1 activity on HDL 3b particles remained significantly associated with LDL phenotype B (OR = 0·781, 95 % CI 0·614, 0·993; P < 0·048), the association of SOD activity was of borderline significance (OR = 1·024, 95 % CI 0·999, 1·43; P = 0·059), while TAG lost significance revealed in univariate analysis.
Table 4. Univariate logistic regression analysis of LDL phenotype B predictors in PCOS (Odds ratio and 95 % confidence intervals)

TAS, total antioxidant status; SOD, superoxide dismutase; PON1, paraoxonase-1.
Discussion
Dyslipidaemia is the most common metabolic abnormality in PCOS, although the type and the extent of the disorder varies(Reference Macut, Bjekic-Macut and Savic-Radojevic2), as a result of complex interactions between hyperandrogenism, IR, environmental (nutrition, physical activity) and genetic factors(Reference Basar Gokcen, Akdevelioglu and Canan23,Reference Pala, Barbaro and Dicembrini24) . Previous studies have shown that obese women with PCOS may have reduced LDL particle size, due to the increased sdLDL particles(Reference Berneis, Rizzo and Hersberger25). In the study of Phelan et al. (Reference Phelan, O‘Connor and Kyaw-Tun4), both PCOS and BMI were associated with decreased LDL size and redistribution towards smaller, pro-atherogenic particles. They concluded that, despite the fact that exact mechanisms of changed lipoprotein metabolism in PCOS are not known, hyperandrogenemia and increased central obesity could be causal factors. We assume that excess of androgen early in life of normal weight PCOS patients might be the reason for the absence of a significant difference in LDL particle size and subclasses between our PCOS groups. It is known that many factors, including BMI, ethnic origin, complexity of the syndrome (anovulatory v. ovulatory) and diet have an impact on LDL-cholesterol in PCOS patients. In line with previous studies, the same factors may also influence LDL particles distribution(Reference Vekic, Topic and Zeljkovic21). So far, only few studies dealt with HDL subclasses distribution in PCOS. In the present study, overweight or obese PCOS patients had significantly smaller HDL particle diameters and higher proportions of small HDL 3a subclasses compared with normal weight patients (Table 1). This finding is in agreement with the available data which showed a decrease in the HDL particle size in obese PCOS patients and other groups with higher CVD risk(Reference Kim, Tfayli and Michaliszyn26), suggesting that obesity is the major contributor for the observed changes in HDL subclasses profile in PCOS.
The results of previous studies have shown that women with PCOS have elevated markers of OS, as well as that PCOS, independently of obesity, may trigger OS(Reference Murri, Luque-Ramirez and Insenser8). In our study, TOS, an aggregative index of plasma oxidant state, and AOPP, a marker of oxidant-mediated protein damage and a potent pro-inflammatory mediator, were increased in overweight or obese PCOS patients (Fig. 1). This agrees with the findings of Blair et al. which showed significant difference in TOS levels between PCOS patients with respect to BMI(Reference Blair, Kyaw-Tun and Young27). Our previous study showed that PCOS patients had lower PON1 activity compared with the control group(Reference Perovic Blagojevic, Ignjatovic and Macut9). In the present study, however, PON1 activity and PON1 distribution on HDL subclasses did not differ between normal weight and overweight or obese PCOS patients (Fig. 1). These results suggest that disease itself affects serum PON1 activity and distribution on HDL subclasses, regardless of BMI, which should be confirmed in the future studies in order to improve our understanding of PON1 status in PCOS.
The results of the presented study demonstrated significant interplay between markers of OS status and lipoprotein subclasses distributions in PCOS. The markers of enhanced OS (AOPP, PAB) were associated with sdLDL particles, which may indicate higher potential for their oxidative modifications. The levels of oxidised LDL are increased in PCOS patients and represent a reliable predictor of CVD, as has been confirmed in the study of Macut et al. (Reference Macut, Damjanovic and Panidis6). The observed associations between the levels of PAB and relative proportions of HDL 2 particles suggest that OS might also play the role in alterations of HDL metabolism in PCOS. In fact, OS is able to inhibit lecithin-cholesterol acyltransferase activity and consequently to affect the maturation of HDL particles, leading to the accumulation of small HDL 3 subclasses(Reference Miljkovic, Stefanovic and Vekic17). Furthermore, free radicals can also modify HDL particles(Reference Kotur-Stevuljevic, Bogavac-Stanojevic and Jelic-Ivanovic28). In support of this hypothesis, our data showed that higher proportions of HDL 3a subclasses were associated with increased malondialdehyde concentrations, which is consistent with previous results(Reference Vekic, Topic and Zeljkovic21). Yet, the question that still remains disputable is which HDL subclass provides the strongest cardiovascular protection. It has been suggested that larger HDL 2 particles have higher atheroprotective potential, because their levels are reduced in patients with CVD or higher in premenopausal women(Reference Gugliucci and Menini29). However, in vitro studies have demonstrated that smaller HDL 3 particles are more effective cholesterol acceptors and also have superior antioxidant properties than larger HDL 2 particles(Reference Camont, Lhomme and Rached30). Another controversy is centred around the exact localisation of PON1 on HDL subclasses. Previous studies have determined that PON1 activity is most commonly found on smaller HDL 3 particles(Reference Camont, Lhomme and Rached30). Recent HDL proteomic studies have confirmed these findings(Reference Heinecke31), supporting the concept that smaller HDL 3 particles have greater antioxidative potential. Recently, a hypothesis has been raised that the localisation of PON1 changes during maturation of HDL particles. Hence, the inverse association between serum PON1 activity with the proportions of PON1 activity on HDL 3 subclasses observed in this present study can be explained by the rapid transfer from smaller HDL 3 to larger HDL 2 particles(Reference Gugliucci and Menini29). Moreover, our analysis showed that the proportion of PON1 activity on HDL 3 subclasses was associated with OS biomarkers. This finding suggests that exacerbated OS in PCOS may affect HDL maturation that yields to the accumulation of PON1 on smaller HDL 3 subclasses. On the other hand, there is also a possibility that the observed associations reflect superior antioxidative properties of small HDL 3 particles in the prooxidative conditions, such as PCOS(Reference Perovic Blagojevic, Ignjatovic and Macut9). The observed inverse association between PON 1 activity on HDL 3 particles and Protective score, representing general antioxidative potential of plasma, may support this assumption.
Further analysis showed increased TAS and SOD activity in PCOS patients with LDL B phenotype, whereas the indices of enhanced OS did not differ between the two LDL phenotype groups (Table 3). LDL B phenotype denotes increased prevalence of sdLDL particles, which are more susceptible to oxidative modifications(Reference Macut, Damjanovic and Panidis6). A finding of increased levels of antioxidants in the patients with LDL phenotype B suggests up-regulation of antioxidative defence mechanisms to prevent OS exacerbation in PCOS. Analysis of PON1 distribution on HDL subclasses showed that women with LDL phenotype B had lower PON1 activity on HDL 3 subclasses, but higher activity on HDL 2b particles than the patients with LDL A phenotype. This finding can be a sign of reduced antioxidant capacity of HDL 3 particles in conditions associated with increased CVD risk(Reference Gugliucci and Menini29), such is the preponderance of sdLDL particles. A potential relevance of the observed associations was further confirmed in logistic regression analysis (Table 4). As we expected, TAG concentration was pointed out as an important predictor of LDL B phenotype, which is consistent with the fact that hypertriacylglycerolaemia is one of the features of atherogenic lipoprotein phenotype. Nevertheless, higher activity of SOD as well as reduced proportion of PON1 activity on small HDL 3b particles remained significantly associated with LDL B phenotype in multivariate analysis. It is now well established that atherogenesis starts at young age and continues throughout life, especially in the presence of risk factors such as obesity, dyslipidaemia, inflammation and elevated OS that are commonly seen in PCOS. Therefore, the evaluation of novel cardiometabolic risk factors and the understanding of the kinetics and function of PON1, particularly how it is affected in various conditions that alter maturation and remodelling of HDL particles, provide additional information to guide preventive measures in order to attenuate atherosclerosis progression.
Strengths and limitations
The present study represents a comprehensive evaluation of OS status and lipoprotein subclasses distributions and provides novel data on PON1 distribution on HDL subclasses in PCOS. The studies that investigate PON1 distribution on HDL subclasses might provide further insight into mechanisms responsible for HDL dysfunction in various diseases. This may be of particular importance in individuals with increased cardiometabolic risk, such as young women with PCOS. The cross-sectional design of our study represents the limitation because it does not allow us to demonstrate the causality of the observed relationships. Future prospective studies with larger number of patients would be needed to further expand our observations. Also, considering that this is a cross-sectional study and not an interventional study, we analysed two groups of patients on standard diet that were diagnosed based on Rotterdam criteria including only combination of clinical, hormonal and ultrasound indices. We believe that the impact of diet could be used in future investigations to show how dietary interventions influence inflammation, OS and dyslipidaemia in patients during prolonged period of follow-up or on specific therapeutic regimens.
Conclusion
Our data showed that OS is associated with pro-atherogenic changes in lipoprotein subclasses profiles in patients with PCOS. Obesity in the PCOS favours redistribution of HDL subclasses towards smaller particles with a lower antioxidant potential, particularly in the presence of sdLDL particles. Taking all into account, our data provide additional evidence in favour of advanced lipid and OS testing for future management of CVD risk in PCOS patients.
Acknowledgements
This work was supported by grants 200110 and by grants 451-03-9/2021-14/200161 from the Serbian Ministry of Science and Education.
All authors listed have contributed sufficiently to the study to be included as authors. I. M. P. B. – carrying out the study, analysing the data, interpreting the findings, writing the article. J. Z. V. – analysing the data, interpreting the findings. D. P. M. – formulating the research question(s), designing the study. S. D. I. – formulating the research question(s), designing the study. M. M. M.-T. – carrying out the study. A. R. Z. – analysing the data, interpreting the findings. V. V. S.-K. – formulating the research question(s), designing the study. I. B. B.-A. – carrying out the study, collecting the samples. J. D. B.-M. – carrying out the study, collecting the samples. B. A. K.-K. – carrying out the study, collecting the samples. Z. G. A. – carrying out the study, collecting the samples. D. S. I. – carrying out the study, collecting the samples. J. M. K.-S. – formulating the research question(s), designing the study.
There are no conflicts of interest.