Background
Antimicrobial Resistance (AMR) is one of the largest threats to public health across the globe (Government of Canada, 2017; WHO, 2018), causing an estimated 1.27 million deaths (due to bacterial AMR directly) globally in 2019 (Murray et al., Reference Murray, Ikuta, Sharara, Swetschinski, Robles Aguilar, Gray and Naghavi2022). Financial burdens also occur because of increased healthcare costs and loss of productivity; Europe loses 1.5 billion US dollars annually due to multi-drug resistant bacteria (The European Commission, 2018). AMR can also impact the agricultural sector via production losses from animals with resistant infections, and possibly decreased trade due to a fear of resistance (The European Commission, 2018).
The system that produces AMR is a complex system comprised of many drivers across many sectors (human, animal, and environmental sectors) and ecological scales, allowing for AMR to easily spread across sectors (Aarestrup et al., Reference Aarestrup, Wegener and Collignon2008; Government of Canada, 2017; The European Commission, 2018). Due to this complexity, modelling and combatting AMR through policy and interventions is challenging (Acar and Moulin, Reference Acar and Moulin2012; Wang et al., Reference Wang, Zhang, Liang and Bloom2016; Islam et al., Reference Islam, Aldstadt and Aga2019; Malik and Bhattacharyya, Reference Malik and Bhattacharyya2019; Søgaard Jørgensen et al., Reference Søgaard Jørgensen, Folke, Henriksson, Malmros, Troell and Zorzet2020). Complex systems are composed of many elements with multiple interactions between them, in which the elements adapt and react to the patterns the interactions create (Ladyman et al., Reference Ladyman, Lambert and Wiesner2013). These systems are composed of nonlinear relationships (small changes in one element do not necessarily cause a small change in another; Waterloo Institute of Complexity and Innovation, 2022) where microscopic interactions between elements can cause system-wide changes to emerge, thus leading to unpredictable behaviours or unintended consequences (Ladyman et al., Reference Ladyman, Lambert and Wiesner2013; Waterloo Institute of Complexity and Innovation, 2022). Knowledge about how the complex pathways intersect and interact to affect AMR is sparse, therefore, incorporating the entire One Health system into dynamic models and policy analysis is still in its early stages (Chen and Fu, Reference Chen and Fu2018; Malik and Bhattacharyya, Reference Malik and Bhattacharyya2019). For this study, the One Health system was defined as the complex web of environmental, human, and animal factors and socio-ecological drivers that interact to perpetuate the issue of AMR. Thus, we wanted to identify how current models across sectors or sub-systems have been combined and determine where data exists to provide the foundations to build and parameterize a proposed model of the Swedish One Health system as a case study to be able to assess interventions through a system lens.
Sweden has one of the lowest rates of AMR and antimicrobial use in Europe (The Public Health Agency of Sweden, 2023) due to the rapid response, enhanced surveillance, and the guidance and stringent measures put in place to reduce antimicrobial use in humans and animals (The Public Health Agency of Sweden, 2023; Wierup et al., Reference Wierup, Wahlström and Bengtsson2021). Sweden has a history of long-term efforts to control and eradicate infectious diseases in animals and coordinated activities for endemic diseases, thus reducing the need for antimicrobials (Wierup et al., Reference Wierup, Wahlström and Bengtsson2021). Finally, there is widespread stakeholder agreement on the need for AMR action and thus cooperation in creating and implementing measures across the country (The Public Health Agency of Sweden, 2023; Wierup et al., Reference Wierup, Wahlström and Bengtsson2021), and much trust in the government and adherence to guidelines from the public (Lambraki et al., Reference Lambraki, Cousins, Graells, Leger, Henriksson, Harbarth and Majowicz2022). Sweden’s response to AMR began in 1986, when the use of growth promoters were banned in food animal production (Grundin et al., Reference Grundin, Blanco-Penedo, Fall and Lewerin2020). In 2005, Strama (a national strategic programme in Sweden) was formed and the first One Health national strategy against AMR was presented, which has been continually updated, with the latest version published in 2023 (Grundin et al., Reference Grundin, Blanco-Penedo, Fall and Lewerin2020). This strategy includes an action plan to address seven core objectives as well as an inter-sectional coordinated mechanism to bring together expertise from sectors across sectors to work to reduce AMR (Grundin et al., Reference Grundin, Blanco-Penedo, Fall and Lewerin2020). Sweden’s policies incorporate the One Health aspect of the issue, involving agencies and organizations from human and veterinary medicine, the environment, and food production, outlining clear responsibilities and focussing on international collaboration, research, and innovation (Eriksen et al., Reference Eriksen, Björkman, Röing, Essack and Lundborg2021).
Systematic and scoping reviews have been conducted to identify models of the system that drives AMR. However, their scope has been limited to population-level mathematical models of AMR within human populations (Niewiadomska et al., Reference Niewiadomska, Jayabalasingham, Seidman, Willem, Grenfell, Spiro and Viboud2019), at the microbial or within-host level (Spicknall et al., Reference Spicknall, Foxman, Marrs and Eisenberg2013), or a combination of both (Opatowski et al., Reference Opatowski, Guillemot, Boëlle and Temime2011). Even reviews that aim to be more inclusive remain human-focused (Ramsay et al., Reference Ramsay, Invik, Checkley, Gow, Osgood and Waldner2018). Thus, it is necessary to expand the scope to identify models of the broader system of drivers which have potential to be adapted for the AMR context.
While a formal literature review is not required for parameterization, a clear framework can improve transparency in the data collection process, especially due to the vast number of factors to be parameterized. With a shift towards more inclusive (integrated) models, scoping reviews or literature scans have gained popularity as a primary step (Murphy et al., Reference Murphy, Carson, Smith, Chapman, Marrotte, McCann, Primeau, Sharma and Parmley2018; Primeau, Reference Primeau2020; Goltz, Reference Goltz2022), and may become the norm in One Health modelling of complex systems. Our proposed model includes 92 factors from a previously created qualitative model of the drivers of AMR (Lambraki et al., Reference Lambraki, Cousins, Graells, Leger, Henriksson, Harbarth and Majowicz2022), making a full scoping review extremely resource intensive. Also, many factors were unlikely to have quantitative data available. Thus, a broader search (quantitative and qualitative data from grey and published literature) was necessary.
Therefore, a literature scan inspired by scoping review methods (Arksey and O’Malley, Reference Arksey and O’Malley2005) provided a balance of structure and transparency with the flexibility to explore the literature more freely. The objectives of this study were to perform a literature scan to identify: (1) the different types of existing dynamic models (e.g., compartmental model, agent-based model, network model) across various parts of the broader AMR One Health system, and (2) the data sources and evidence that could be used to model the different parts of the Swedish One Health system (further referred to as “the system”).
Methods
To determine the scope of the One Health model of AMR, we used an existing causal loop diagram (“diagram”) from two participatory modelling workshops that were held in Stockholm, Sweden in September 2019 in which participants mapped the wider system of drivers of AMR in the European One Health system (refer to the workshops carried out by Lambraki et al., Reference Lambraki, Cousins, Graells, Leger, Henriksson, Harbarth and Majowicz2022 for the methods and full results). The resulting diagram contained 92 nodes and 331 relationships and represented the structure of a hypothetical One Health model of AMR in a European (specifically Swedish) food system context. Using this diagram to bound the scope and define the search terms, we conducted a literature scan, inspired by Arksey and O’Malley, Reference Arksey and O’Malley2005 framework for scoping reviews, to address our two objectives. The search took place from September 1st to December 31st, 2020.
Objective 1: Existing models
A literature search of peer-reviewed publications was performed in Google Scholar and PubMed to identify different types models, including: 1) mathematical models pertaining to the transmission of micro-organisms between humans, animals, and their environments; 2) mathematical models of AMR transmission or emergence; 3) models of AM decay and residue build up in waste, waste-water, and other settings; 4) economic models of agriculture and the One Health system, and; 5) economic models of health systems. Models were not limited to a specific geographical context; however, the search was conducted in English.
A snowball search approach was used, starting with broad, high-level search terms that aligned with major domains of the diagram (e.g., “model AND antimicrobial resistance AND humans/animals/One Health” or “model AND consumer demand AND food”). Models of E. coli were explicitly searched because E. coli has been identified as one of the major threats for AMR (Léger et al., Reference Léger, Lambraki, Graells, Cousins, Henriksson, Harbarth, Carson, Majowicz, Troell, Parmley, Jørgensen and Wernli2021), is commonly identified in and transmits between humans and animals (Léger et al., Reference Léger, Lambraki, Graells, Cousins, Henriksson, Harbarth, Carson, Majowicz, Troell, Parmley, Jørgensen and Wernli2021), and is heavily represented in the existing AMR surveillance data for animals and humans in Sweden (European Centre for Disease Prevention and Control, 2021; WHO Regional office for Europe, 2017). In instances where specific sections of the diagram were not well described, search terms were refined and the search was narrowed to capture more specific nodes or parts of the system (e.g., “infectious disease model AND antimicrobial resistance/resistant E. coli AND hospital/on-farm/abattoir,” “economic/supply-demand/consumer demand model AND chicken/beef/fish”). This approach allowed for a narrowing of the search criteria to ensure key models were captured in the search, that would be of use for our specific purpose. A full list of search criteria is given in Supplementary materials, Table S1. We found that the first 100 results generally yielded the best fit given our search criteria, and therefore, focused on the first 100 results for each search. Broad search terms and few exclusion criteria were deliberately used to capture a wide range of models from a variety of sectors. Citations about models that were strictly statistical (e.g., linear and logistic regression models) were excluded; we wanted models that simulated or encompassed the transmission or emergence of AMR or other parts of the system (economics) and not models that provided estimates of association between nodes (e.g., odds or risk ratios). Statistical models would provide an indication of how two nodes affect one another (AMU leads to AMR) and could be used to help inform or parameterize the relationships of a model that simulates AMR transmission, but they are not themselves models of simulation of the broader system.
The lead author (MC) screened the titles and abstracts for inclusion based on the criteria above, then reviewed the full text and excluded any sources that were not simulation models. The following information was extracted from relevant articles and organized into a database created in Microsoft Excel version 16.60: type and process of model (e.g., agent-based model, network model, compartmental model); the sector(s) represented (e.g., food-producing animals, humans, crops, environment); the micro-organism and/or antimicrobial(s) involved (e.g., fluoroquinolone-resistant E. coli); and other model characteristics (Tables 1–2; full database Cousins, Reference Cousins2022). Models were categorized to the environment sector if they represented anything that is external to a host (Porta, Reference Porta2016); included any area in which a person, animal, or plant is living or operating (Oxford Learner’s Dictionary, n.d.), which was dependent on the setting of the model and the population of interest (e.g., the bed or light switch, a river or surrounding landscape).
Table 1. Distribution of the 106 articles* referring to models of disease transmission of a single system (n = 102) and models of disease transmission with an economic component (n = 4) according to microbe and/or antimicrobial class and sector/population involved

* To find more details for each model that falls under the given category please refer to the complete database (Cousins, Reference Cousins2022). The complete list of articles is categorized with the same categories as appear in the table for easy cross-reference.
** AM, Antimicrobial.
Table 2. Distribution of the 106 articles* referring to models of disease transmission of a single system (n = 102) and models of disease transmission with an economic component (n = 4) according to study characteristics including the main population of interest, model type and specific model features, and the type of model system (sensitive microbes, resistant microbes, and antimicrobials (AMs**)
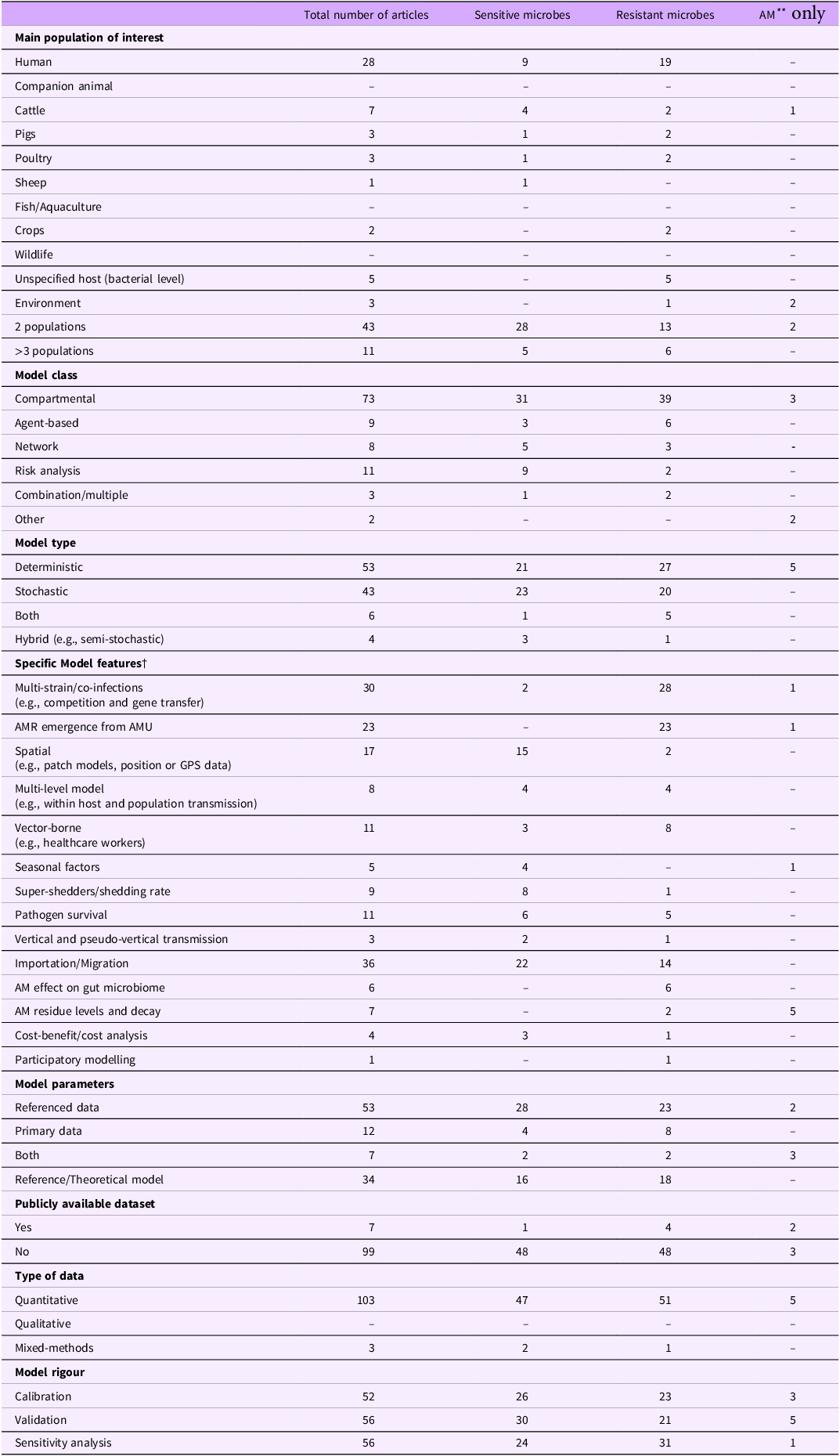
* To find more details for each model that falls under the given category please refer to the complete database (Cousins, Reference Cousins2022). The complete list of articles is categorized with the same categories as appear in the table for easy cross-reference.
** AM, Antimicrobial.
Identified models were visually situated within the diagram (Figure 1) to identify gaps in system coverage (e.g., missing human health, on-farm, or the environment) and depict the overlap between sectors (e.g., zoonotic transmission, food transmission, models including environmental reservoirs, or One Health models) captured by existing model.

Figure 1. The diagram of AMR adapted from Lambraki et al. (Reference Lambraki, Cousins, Graells, Leger, Henriksson, Harbarth and Majowicz2022) with the types of models found from the literature search categorized into broad themes overlayed to depict model coverage of the system. Note: this figure is zoomable in the PDF version to legible font size.
Objective 2: Existing data sources and evidence
A second literature search of peer-reviewed publications and grey literature was performed in Google Scholar, PubMed, and Google, to compile data to populate the proposed model using multiple types of data (quantitative and qualitative) from 1995 to present, prioritizing sources 2000 to present and those that were Sweden-focused. While our interest was to identify data from 2000 to present, 1995 was a cut-off to account for potential gaps in data collection (e.g., data collected every five years which causes a gap from 1997 to 2002). Furthermore, if no Sweden-specific data existed, northern Europe was used as a proxy, and if nothing was specific to northern Europe, then all of Europe or a European average was included. The search was not limited by language; all non-English publications were translated with Google Translate.
Search criteria were created based on the 92 nodes identified on the diagram (Lambraki et al., Reference Lambraki, Cousins, Graells, Leger, Henriksson, Harbarth and Majowicz2022). A search was created for each node. Some were refined further if found necessary; for the node “on-farm production,” a separate search was done for on-farm production of animal-based foods (e.g., chicken), fruits (e.g., apples), vegetables (e.g., potatoes), and other important crops (e.g., wheat). Examples of search criteria include: “Antimicrobial resistance AND human AND Sweden,” “Imports AND chicken AND Sweden.” Broad nodes were narrowed using specific examples given by the participants within the workshops (Lambraki et al., Reference Lambraki, Cousins, Graells, Leger, Henriksson, Harbarth and Majowicz2022); for the node “New and emerging food,” specific products in development or of interest to the European population were searched (insects, genetically modified foods, three-dimensionally printed foods, and lab-based meat). Nodes that were abstract (e.g., diverse experiences and opinions) or broad (e.g., AMU in countries other than of Sweden) were more difficult to create an adequate search strategy. We aimed to find search strategies that were specific enough to retrieve relevant information, while still capturing a broad range of data and sources. A full list of the search strategies used can be found in Supplementary materials, Table S2. As with objective 1, the searches for the 92 nodes each returned over 750 results, and therefore we reviewed titles from the first 100 results for each search string from each database. Citations that were excluded were: 1) those that did not contain information relevant to the list of nodes from the diagram, and 2) those published before 1995.
Due to the number of separate searches performed (at least one per node), the literature scan was time and resource intensive. Therefore, per recommendations by Arksey and O’Malley, Reference Arksey and O’Malley2005, a three-month cut-off date was used (December 31st, 2020) in which articles identified as potentially relevant by title and abstract during the search but were not directly related to the node being searched at the time were not read in full but noted in a separate list (see Supplementary materials, Table S3) for future use. These articles did not undergo the proceeding steps of full review for inclusion and data extraction.
MC reviewed the full text for titles and abstracts that were screened in and excluded any sources that did not contain data relevant to the nodes of interest. MC then extracted the data. Due to the variety of sources and types of reported data, an inter-coder reliability check was done with three members of our team (MC, KD, XMYK) to ensure the same data were being extracted from the articles. MC selected three articles that represented the spectrum of the articles identified for data extraction (two peer-reviewed and one grey literature that were quantitative, qualitative, and mixed-method) and a full article review was performed. MC, KD, and XMYK compared and discussed results to refine what needed to be extracted, or what was being missed.
The following information was extracted (MC) from relevant articles and organized into an excel database (MNV & MC): the data or parameter extracted (e.g., 1,000,000 prescriptions/year), the year the data was collected, the type of data (e.g., pharmaceutical sales, quantitative), the source (e.g., peer-reviewed or grey literature), they type of study (e.g., survey, cross sectional), the country or countries for which the data were available, and the date and country of publication (Table 3; full database; Cousins, Reference Cousins2022). The country of publication was identified by either the affiliation of the first author on a peer-reviewed publication, the country in which the author of an article (magazine, newspaper, blog) was located, or the country of the main headquarters of an organization, magazine, newspaper, or webpage.
Table 3. Distribution of the 414 sources of data relating to the nodes (n = 64) according to the study characteristics including the number of sources, number of data points, type of data, and regions and years covered within the data

* AM: Antimicrobial;
** EU/EEA: European Union/ European Economic Area;
† ARO: Antimicrobial-resistant organism;
‡ PR: Public relation.
The amount of data and an associated level for the node was then initially assigned by MC’s personal judgment, and then verified by the research team through discussion, with disagreements being resolved through consensus. The level of the node refers to the position of that node in the context of the Swedish One Health system on a scale of the amount, quantity, extent, or quality compared to a referent (e.g., Sweden vs. other countries within or outside of Europe, Sweden currently vs. historically). For example, there is “high” AMU in agriculture in Sweden in 2020 compared to 2010 or there is “low” AMR in Sweden compared to other countries in Europe. The following levels were assigned: very high, high, medium, low, very low, or none. A level was assigned to the nodes to indicate how the qualitative and quantitative data could be combined to generate a single estimate of the state of that node, which could then be used to inform a semi-quantitative model for example.
A level was able to be assigned to a node based on the decision criteria found in Figure 2; a node had to have enough data to create an accurate evaluation of the state of the node and had to have either: 1) a good comparator to be able to judge the node against (e.g., historical data or data from another country), and 2) the node could be accurately described by a single level (e.g., “Consumer choice, demand, and behaviour” could be split into many different nodes that represent how consumers feel about different commodities and therefore one level could not be assigned).
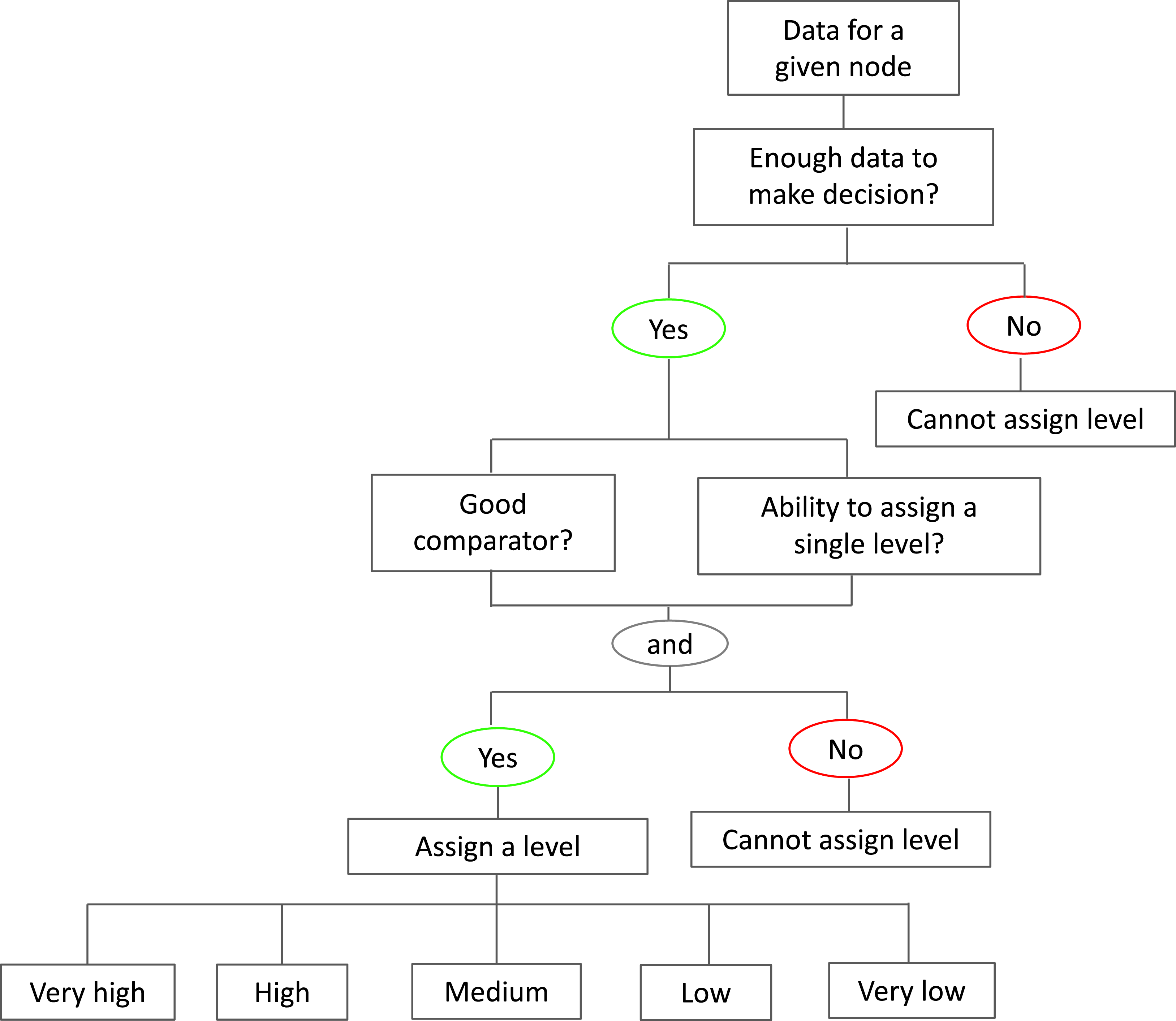
Figure 2. How each of the 64 nodes were categorized into ordinal levels (very high, high, medium, low, very low) given the quantitative and qualitative data found from the literature scan to inform the model the different parts of the Swedish food system.
The amount of data to inform each node was also assigned a categorical level (very little, a little, some, a lot, and most), which was based on the number of sources and data points (e.g., many sources or many years of data collected), and the amount of quantitative and qualitative data that existed for a given node (Figure 3). A data point was defined as a single piece of qualitative (e.g., a quotation) or quantitative data (e.g., a statistic) referring to a single node. A visual representation of the existing data for the nodes was overlayed on the diagram of AMR in the system to depict the amount and quality of data that exists and identify major data gaps (Figure 4).

Figure 3. How each of the 64 nodes were categorized into ordinal levels that describe the amount of data (very little, a little, some, a lot, most) given the number of source and amount of the data found from the literature scan to inform the model the different parts of the Swedish food system.

Figure 4. The diagram of AMR adapted from Lambraki et al. (Reference Lambraki, Cousins, Graells, Leger, Henriksson, Harbarth and Majowicz2022) to show the data sources and evidence found from the scoping review. Nodes colour represents the assigned level for the given node, the darkness of the shading represents the amount of data for the given node, and the relationships that were mentioned in the sources are coloured in black with numbered references (found in database; Cousins, Reference Cousins2022) provided in brackets. Note: this figure is zoomable in the PDF version to legible font size.
Although searches were conducted based on the nodes, data pertaining to the relationships between the nodes were also found and extracted into the database, but these were not categorized into categorical levels (Cousins, Reference Cousins2022). New relationships identified via the literature search were mapped on top of the original diagram (Supplementary materials, Figure S1), and the sources that had data pertaining to the existing relationships were also visually mapped (Figure 4).
Results
Objective 1: Existing models
We identified a total of 146 relevant peer-reviewed articles (Table 4) that provided good coverage of the One Health system (Figure 1). Each individual article and relevant information pertaining to the articles can be found in the database (Cousins, Reference Cousins2022). Most articles were published after 2000 (Supplementary Materials, Figure S2). Most (79/146, 54%) models were either non-geographic context specific or included countries from all over the world (e.g., international travel, global food demand; Cousins, Reference Cousins2022), four of which were situated in multiple countries of Europe. Many articles in which a country was defined were from high-income countries (58/146, 40%), as defined by World Bank (e.g., United States of America (USA), Sweden, Denmark) (World Bank Group, n.d.), and only 10 articles (7%) represented models from low- and middle-income countries. Our search yielded 14 articles that described economic models, however upon further analysis we found that these models were not useable for our purpose as they represented statistical models (e.g., econometric, time series) that reflect the associations between nodes, not simulation models. These excluded models are described in Supplementary materials, Table S4 (details about each model can be found in the database; Cousins, Reference Cousins2022) and are of interest for future research.
Table 4. Breakdown of the types of models found in the sources identified in the literature from a literature scan different types of existing models across various parts of the broader One Health system (n = 146)
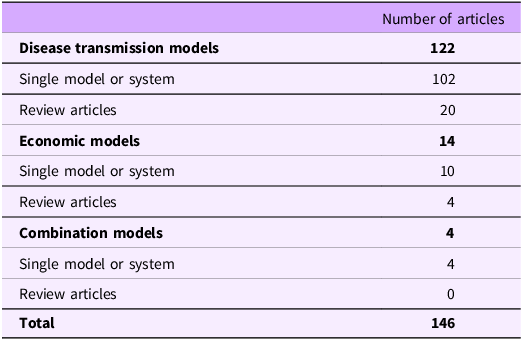
There were 126 articles that described models focused on disease transmission and/or AMR emergence and transmission, 102 of which were models of a single system, 4 were models of a single system that included an economic component (e.g., cost-effectiveness of an intervention or cost analysis of a disease outbreak), and 20 were review articles summarizing multiple models (not included in the following analysis). Table 5 summarizes the location the model represents (e.g., a hospital, on-farm, food), the type of transmission that is described (e.g., person-to-person, animal-to-animal), and whether the model was representing a sensitive microbe, a resistant microbe.
Table 5. Distribution of the 106 articles* referring to models of disease transmission (n = 102) and models of disease transmission with an economic component (n = 4) according to model processes, type of transmission, and type of model system (sensitive microbes, resistant microbes, and AMs**)
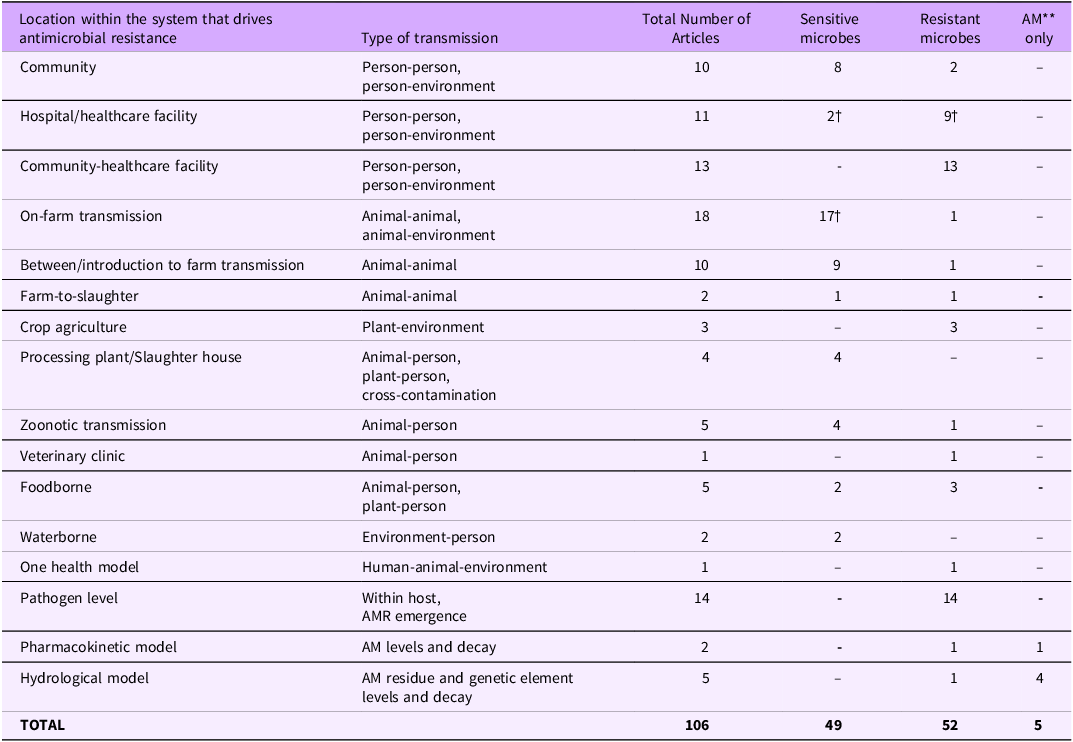
* To find more details for each model that falls under the given category please refer to the complete database (Cousins, Reference Cousins2022). The complete list of articles is categorized with the same categories as appear in the table for easy cross-reference.
** AM, Antimicrobial. †At least one of these models contain an economic component.
Table 1 summarizes the microbes and AMs that were modelled and the different sectors or transmission pathways these models encompassed. Less than half of the models addressed two or more sectors (46/106, 43%). Many articles were focused on humans (42/106, 40%). Models at the human-animal interface were described animal exposure as a risk factor for human illness. Models that included the environment as part of the transmission pathway looked at either the immediate surroundings of the host (contaminated pens, hospital equipment, and production equipment; 23/106, 22%) or the natural environment (water sources, soil, plants; 8/106, 8%). The environment as a target area for modelling was very limited (3/106, 3%), and was mostly framed in terms of causing human illness or as a reservoir for pathogens. There were very few models about plant/crop agriculture (5/106, 5%).
Resistant bacteria, specifically E. coli and methicillin-resistant Staphylococcus aureus (MRSA), were the most modelled compared to other resistant microbes (Table 1). Five models focussed on an AM instead of a target microbe (Table 1), specifically on the decay of antimicrobials in different settings (one in the gastrointestinal tract of a host, and four in water systems). One model also described AM’s effect on the development of AMR in a pathogen. Five models did not have a specified host (Table 1) and described the transmission of resistance genes and the development of resistance in a population of micro-organisms after exposure to an antimicrobial.
Transmission model characteristics including the type of pathogen (susceptible or resistant) and AM being modelled are shown in Table 2. The majority (42/106; 40%) were human-focused and were either at a population level (e.g., transfer of patients between hospitals and community members) or at a microscopic or micro-organism level (e.g., within-host models of gene transfer and AMR emergence), with very few including both mechanisms into a multi-level model (Table 2). There were very few animal-focussed models of AMR. Alternatively, models of microbes that did not state the resistance status were mainly animal centred and focussed on within-farm or between-farm transmission (Table 2).
Finally, most of the models described were compartmental (n = 73/106, 69%) (Grassly and Fraser, Reference Grassly and Fraser2008; Hohle, Reference Hohle2015; Blackwood and Childs, Reference Blackwood and Childs2018) and deterministic (53/106, 50%) (Grassly and Fraser, Reference Grassly and Fraser2008; Hohle, Reference Hohle2015), and used data from the peer-reviewed literature as inputs for model parameter values (53/106, 50%). This was especially true within the AMR-focused models (Table 2). The use of theoretical models was also widespread (34/106, 32%), especially within the AMR models. We defined theoretical models as models that provide a general structure and are solved mathematically but are not informed by empirical data. These models were occasionally tested with parameters from the literature or with wide ranges for parameters to capture many possible values. Models rarely included all indications of rigour (sensitivity analysis, validation, and calibration (Barlas, Reference Barlas1996; Martis, Reference Martis2006; van Kleef et al., Reference van Kleef, Robotham, Jit, Deeny and Edmunds2013) but over half of the models (56/106, 53%) included at least one of these features.
Objective 2: Existing data sources and evidence
A total of 414 articles were identified that addressed 64 of the 92 nodes from the qualitative model (see full database; Cousins, Reference Cousins2022). Despite the thorough review, there was no literature found for 28 nodes, including: AMU in wildlife; AMU and AMR in other countries; antimicrobial-resistant organisms (AROs) in plant agriculture; cost per unit set by quota; disposal of AMs (e.g., unused, unmetabolized); diverse experiences, education, and training; existing farm infrastructure; existing healthcare infrastructure; exposure to AROs through imported products; good farm practices; host microbiome; level of resistance AMR in other countries; national budgets money, and funding; non-AM infection prevention and control in plant agriculture, by the public, and in other social institutional settings; producer profitability; research, development, and innovation; resistance at the abattoir/processor; restocking with animals/eggs at higher risk of infection; retail availability of meat/eggs in domestic market; science and academia; time to market weight; treatment post-procedure; what is being farmed; and the wider environment microbiome (e.g., water, soil).
The data came from both peer-reviewed (149/414, 40%) and grey literature (228/414, 60%) which are outlined in Supplementary materials, Figure S3. These sources came from multiple countries (Supplementary Materials, Figure S4), with Sweden being the predominant country of origin as it was prioritized in the search (117/414, 28%). Sources were mainly published after 2017 (205/414, 50%; Supplementary materials, Figure S5). Many databases reported surveillance data or national/regional statistics and indicators which were particularly useful, including: Food and Agriculture Organization of the United Nations (FAO), Worldbank, Eurostat, Migration Data Portal, Our World in Data. Similarly, other useful statistical webpages depicted or combined information found in common databases: Statista, Knoema, TrendEconomy, and Indexmundi
Both qualitative and quantitative data was reported; the majority was quantitative (4445/5432 data points, 82%). However, more qualitative data were available to parameterize some nodes, due to either a lack of available quantitative data (e.g., development of alternatives to AM), or the nature of the node being described (e.g., animal welfare/low stress, consumer demand and behaviour). Ten of the nodes had “very little” amount and quality of data; one or very limited data sources (1 source) and data (1–15 data points). Six of the nodes had a “most” for amount of data; 16 to 30 data sources and 234 to 413 data points per node, with an average of 94% (88%–97%) being quantitative data points.
The literature search was targeted towards the nodes; however, valuable information was identified pertaining to the relationships (arrows) in the diagram. There were 325 relationships found in the literature, 86 from the original diagram and 239 newly identified during data extraction (see Supplementary materials, Figure S1).
Two new nodes, and included in the newly identified relationships, were added to the diagram: “Access to healthcare (doctors, hospitals, veterinarians, etc.)” and “Number of abattoirs.”
Discussion
We found a wide variety of dynamic models that described many of the transmission pathways within the system. These models however were segregated and had limited connection across sectors. We also found quantitative and qualitative data to help understand the current and past states of many of the nodes representing the system. The data were highly variable in terms of quantity, source, and type but overall, when combined, helped extend our understanding of the overall system and have the potential to be incorporated into future models of AMR.
Literature scan of models of, and factors associated with AMR
Scoping reviews are useful tools for summarizing and disseminating existing literature in a useable and concise format for use by other researchers, policy makers, and other stakeholders who may not have the skills or resources to perform such a task (Antman et al., Reference Antman, Lau, Kupeinick, Mosteller and Chalmers1992) review methodology, this literature scan: 1) provided a framework to improve the transparency of data collection for parameterization of a model of a complex system; 2) supports the building of a future comprehensive One Health model of AMR in a Swedish context; 3) assists other modellers by providing a comprehensive list of existing models of AMR (and related micro-organisms) in various sectors, where data exist within those sectors, and how to gain access to these data; and 4) provides insight into the areas in which more empirical data are needed before comprehensive quantitative models can be created. Major data gaps included AMR in the environmental sector (e.g., levels of resistance within the soil and waterways), in wildlife populations (e.g., the level of resistance in birds, rodents, and other wild animals and the relative contribution these pathways have to overall transmission to humans or food-producing animals), and in crop agriculture, and for nodes referring to economics, resources, and practices on-farm and in healthcare (e.g., healthcare and farm infrastructure, good farm practices, feed quality and feed efficiency). Furthermore, most models encompassed a single sector, and more research is needed into modelling methods to connect existing models from the various sectors.
Disease transmission models embedded within the One Health system
We found that the models provided good coverage of the main parts of the One Health system (human, animal, environment), especially for specific human populations (e.g., within hospital) and agriculture (e.g., on-farm transmission). We included models not directly related to AMR (e.g., Foot and Mouth Disease, Influenza) as they are embedded within the same system and model parts that have not yet been explored in AMR-specific models (e.g., importation or global spread). Human-focused models with limited connection between sectors has been identified as a major gap in AMR models in previous reviews (Antman et al., Reference Antman, Lau, Kupeinick, Mosteller and Chalmers1992; Birkegård et al., Reference Birkegård, Halasa, Toft, Folkesson and Græsbøll2018), but even with the inclusion of a wider range of models, there was still limited connection between the sectors, and nodes less directly related to human health and agriculture (e.g., development of new AMs, research and development) were missed.
Furthermore, models typically took a humanistic perspective, looking at how animals, crops, or the environment can lead to resistance in humans. However, humans also have the potential to introduce and spread resistant microbes to animals and into the environment (Government of Canada, 2017; Mcewen and Collignon, Reference Mcewen and Collignon2017; WHO, 2018). Therefore, a more One Health perspective, including cross-sector connections and evaluating all parts equally within models, is an important step towards an integrative modelling approach.
Smaller scale models, such as those in a single hospital or farm, included the environment as a source of transmission (e.g., shedding faeces, contaminated spaces or workers in hospital). In contrast, larger community models of AMR were simplified to human-human or animal-animal transmission. However, to better understand and address the complex issue of AMR, models need to account for these different transmission pathways.
The AMR-specific models identified were mainly quantitative, deterministic, compartmental models, aligning with past reviews of the literature (Birkegård et al., Reference Birkegård, Halasa, Toft, Folkesson and Græsbøll2018; Ramsay et al., Reference Ramsay, Invik, Checkley, Gow, Osgood and Waldner2018) and the evolution of models; AMR is a developing field, and compartmental models are typically developed first (Hohle, Reference Hohle2015). Individual-based models allow researchers to add more heterogeneity to the population and include additional population-level attributes (e.g., different contact rates, spatial elements, individual behaviours; Bonabeau, Reference Bonabeau2002; Maglio and Mabry, Reference Maglio and Mabry2011; Hohle, Reference Hohle2015). These models are useful but require more data about the populations and the pathogens they model and therefore can be more difficult to parameterize (Bonabeau, Reference Bonabeau2002; Maglio and Mabry, Reference Maglio and Mabry2011; Hohle, Reference Hohle2015). Also, many models were theoretical; models that outline the structure and transmission pathways, but do not use data to inform them. Although these theoretical models are useful to understand transmission dynamics, without data to inform them, their ability to be used to accurately model a system or to assess interventions is limited and untested (Birkegård et al., Reference Birkegård, Halasa, Toft, Folkesson and Græsbøll2018; Ramsay et al., Reference Ramsay, Invik, Checkley, Gow, Osgood and Waldner2018).
Compartmental models provide a good foundation and are typically the starting point for modelling new systems (Hohle, Reference Hohle2015). By creating a set of interlinked compartmental models from multiple areas of the system, we could create a more comprehensive model of the system. Compartmental models also lend themselves well to be expanded into integrated or multi-level models (e.g., within-host and population level; Hohle, Reference Hohle2015). However, there is a lack of data and knowledge about some of the associations between sectors, which can lead to larger uncertainty in results and difficulty in interpreting results. The use of mixed-methods compartmental modelling may be an intermediate step to include a wider range of data.
Economic models embedded within the One Health system
Economic modelling is becoming important in evaluating policies in many health (e.g., health interventions such for obesity (Gortmaker et al., Reference Gortmaker, Swinburn, Levy, Carter, Mabry, Finegood, Huang, Marsh and Moodie2011; Sacks et al., Reference Sacks, Veerman, Moodie and Swinburn2011), hospital interventions for disease control (Assimakopoulos, Reference Assimakopoulos1987; Schechner et al., Reference Schechner, Carmeli and Leshno2017)), and other fields (e.g., energy (Hafezalkotob, Reference Hafezalkotob2018), agriculture (Martínez-López et al., Reference Martínez-López, Ivorra, Fernández-Carrión, Perez, Medel-Herrero, Sánchez-Vizcaíno, Gortázar, Ramos and Sánchez-Vizcaíno2014), and climate change (Kahil et al., Reference Kahil, Connor and Albiac2015)). It has been deemed necessary to identify solutions that can have the most impact with the least amount of associated costs (Jamison, Reference Jamison, J., B., M. and Al2006; Levin and Chisholm, Reference Levin, Chisholm, V., D. and T.2016; Marseille et al., Reference Marseille, Larson, Kazi, Kahn and Rosen2015). Cost is especially important for agricultural and food production as there are many levels at which the costs can be applied (Animals, 1999; FAO, 2017). For example, if the ultimate goal is for impacts at the human population level (e.g., reducing AMR), but it is not economical for producers to implement the intervention (e.g., reducing AMU, changing farming practices, updating farm infrastructure), then it is not going to be adopted as easily as an intervention that allows producers to continue to profit (Aarestrup et al., Reference Aarestrup, Wegener and Collignon2008; FAO, 2017; Mcewen and Collignon, Reference Mcewen and Collignon2017; Wernli et al., Reference Wernli, Jørgensen, Morel, Carroll, Harbarth, Levrat and Pittet2017). Consumer demand is also a strong driver of food availability, cost, and ultimately AMR (Primeau, Reference Primeau2020; Lambraki et al., Reference Lambraki, Cousins, Graells, Leger, Henriksson, Harbarth and Majowicz2022). To provide enough food for the growing population at a price that consumers can afford, agricultural practices have adapted to high intensity farming where the higher animal density may drive the need for AMs for prevention or treatment of inevitable diseases (Lambraki et al., Reference Lambraki, Cousins, Graells, Leger, Henriksson, Harbarth and Majowicz2022). Therefore, engaging economists and economic modellers in AMR modelling is vital for cost-benefit analysis (e.g., weighing initial costs and long-term payoffs) of interventions that aim to control AMR at an international scale.
The evidence landscape of factors that drive AMR
Overall, we found the evidence landscape challenging to navigate. Data existed across many formats and sources, and required substantial interpretation, searching, deciphering jargon, and sifting through multiple databases, government webpages, and other literature. After the data were found, it was either: hard to compare between populations and contexts due to multiple metrics being reported, patchy, incomplete, or were not publicly available. Therefore, to adequately capture data for the 92 nodes identified (Lambraki et al., Reference Lambraki, Cousins, Graells, Leger, Henriksson, Harbarth and Majowicz2022), a separate literature scan may be needed to be conducted for each specific research question (node), especially those where data were not readily available. We estimated that this could take upwards of 276 person-months (given a cut-off of 3 months per node for 92 nodes), which would be resources intensive.
Some of the nodes were informed by quantitative data that captured many years and were specific to the Swedish context. However, in some cases, the way the data were collected or reported was not useful for quantitative modelling purposes; data reported monthly or yearly (as opposed to daily or weekly which is required for many quantitative models), not representative of the entire country, or did not capture the entire context (e.g., surveillance data for one hospital or farm) and could not be generalized to a population level. This quantitative data could, however, be reduced to qualitative categories to represent the state of the node within a semi-quantitative model. For example, the amount of AMU of three countries (e.g., 5 defined daily doses (DDD) per 100,000 population, 10 DDD per 100,000 population, and 18 DDD per 100,000 population (The Centre for Disease Dynamics & Economics & Policy, n.d.)) could be converted into categories; high (18 DDD per 1000,000 population), low (5 DDD per 1000,000 population), and medium (10 DDD per 100,000 population).
Some nodes were mainly represented in qualitative terms. These nodes are important to the system (as identified by the workshop participants; Lambraki et al., Reference Lambraki, Cousins, Graells, Leger, Henriksson, Harbarth and Majowicz2022)) and therefore incorporating this valuable data is necessary to capture the nuances of the One Health system, providing further evidence for qualitative or mixed-methods simulation modelling this complexity and breadth.
Limitations
This literature scan aimed to identify a breadth of models that fit our context and outcome of interest (One Health model of AMR in a high-income context), not to identify every possible model of AMR and zoonotic transmission. Therefore, it is likely that some models were missed that could provide additional insight into the system. However, many models were identified that provide wide coverage of the system and a good foundation for creating a One Health AMR model.
The search for models were not limited by geographical context, however, most of the models identified were from a high-income context. Although this aligns with our specific goal of creating a model in Sweden, a high-income country, this limits the generalizability of this review to modelling low- and middle-income contexts, and a targeted search may be necessary.
The literature scan of the existing evidence landscape was thorough, and searches for data to inform all 92 nodes were attempted by creating, testing, and refining of the search strings. However, it was more difficult to create searches that captured some nodes in their entirety or were narrow enough to capture some of the more detailed or niche aspects of the nodes (e.g., “Consumption of other (non-meat/egg) foods” could include fruits, vegetables, and grains, but also pop, snack foods, and alcohol). Similarly, a subset of nodes was broad and abstract in nature (e.g., diverse experience, knowledge, and training) and although important to AMR dynamics, are challenging to describe on a national and population level, making it difficult to inform population-based models (e.g., compartmental models). For example, an individual’s culture, educational background, and past experiences can shape their views and actions (e.g., how they access the healthcare system (Haenssgen et al., Reference Haenssgen, Charoenboon, Xayavong and Althaus2020; Lohm et al., Reference Lohm, Davis, Whittaker and Flowers2020; Lambraki et al., Reference Lambraki, Cousins, Graells, Leger, Henriksson, Harbarth and Majowicz2022), their trust in doctors and medicine (Blommaert et al., Reference Blommaert, Marais, Hens, Coenen, Muller, Goossens and Beutels2014; Lambraki et al., Reference Lambraki, Cousins, Graells, Leger, Henriksson, Harbarth and Majowicz2022)), can impact their risk of AMR. Furthermore, the 331 relationships (arrows) identified by the workshop participants (Lambraki et al., Reference Lambraki, Cousins, Graells, Leger, Henriksson, Harbarth and Majowicz2022) and additional 239 relationships identified through the literature scan need to be quantified with a targeted search. Finally, although the same search strategy was used throughout, searching the first 100 articles in each data base for each node, the three-month limit could have led to a deeper and more critical look at the titles and abstracts for the nodes that occurred early in the search compared to the end of the search. Finally, although the same search strategy was used throughout, searching the first 100 articles in each data base for each node, the three-month limit could have led to a deeper and more critical look at the titles and abstracts for the nodes that occurred early in the search compared to the end of the search. Overall, this literature search highlights that despite the availability of information, there are substantial challenges as data are not cohesive, accessible, or easy to find. Thus, a more comprehensive scan of the literature by a large inter-disciplinary team (including other fields such as economists or agroecology), to refine nodes for use in modelling and to identify potential data sources is necessary.
Finally, this literature scan was conducted as a pragmatic way of collecting data to outline and parameterize a large-scale model of AMR across a One Health system. Therefore, validating the quality of evidence to support the data or validity of the sources was not a primary aim of this review and not inherently part of the literature scan process. However, most sources were from peer-reviewed, government, or major newspapers and therefore, we have confidence in the data that were collected can be representative of the situation within a high-income country such as Sweden.
Conclusion and recommendations for future research
This literature scan identified disparate, primarily quantitative models that do not fully represent the One Health aspects of this AMR. Many data gaps exist, making it difficult to model the entire system empirically. Current quantitative models are unable to adequately capture the complexity, nonlinear relationships, and interconnectedness of the system and given the data availability, it is not possible to create a fully quantitative model of the complex systems of drivers of AMR in the Swedish One Health system context without including overtly simplifying assumptions. Further work is needed to determine how to integrate the wealth of knowledge found in this review to create a comprehensive model of the system.
To combine the plethora of valuable data found, including both the quantitative and qualitative data, a mixed-methods (semi-quantitative) compartmental model of the 64 nodes for which there were data could be created in the interim, while still advocating for further evidence to fill identified data gaps. Interdisciplinarity and cross-sector collaboration can foster communication, highlight different perspectives and expertise, share knowledge about existing or novel models and where and how data are being collected, to gain a more holistic and One Health view of the system of AMR.
Supplementary material
The supplementary material for this article can be found at https://doi.org/10.1017/one.2023.15.
Data availability statement
The data that support the findings of this study are openly available at the following:
Cousins, M. (2022). Mapping out a One Health model in the context of the Swedish food system using a modified scoping review methodology: Scoping Review Database. https://doi.org/10.5683/SP3/UQZJMB.
Acknowledgements
The authors would like to thank Kim D’Mello and Xenia Man Yuk Kwan for conducting the inter-coder reliability check.
Author contributions
All authors were involved in the study conception and design, analysis and interpretation of data, and drafting of the manuscript. MC conducted the literature scan, analysed and interpreted the results and drafted the original manuscript. MNV organized the data and sources for Objective 2. All authors contributed to the revision of the manuscript for important intellectual content. All authors read and approved the final manuscript.
Financial support
This study is funded through an operating grant of the 5th Joint Programming Initiative on Antimicrobial Resistance (JPIAMR 2017). Funding was provided by an operating grant from the Canadian Institutes for Health Research (Institute of Infection and Immunity, Institute of Population and Public Health, grant number 155210, PI SEM), a Swedish Research Council grant (grant number 2017-05981, PI and Project Coordinator PSJ); and an operating grant from the Swiss National Science Foundation (grant number 40AR40_180189, PI DW). The funders had no role in the design analysis or writing of this article.
Competing interests
Melanie Cousins and Irene A. Lambraki are employed at the Public Health Agency of Canada. E. Jane Parmley is engaged in research funded by the Canadian Institutes for Health Research, Natural Sciences and Engineering Research Council, Ontario Ministry of Agriculture, Food and Rural Affairs, the Public Health Agency of Canada, and the Canadian Safety and Security Program. She is currently President of the Board of Directors of the Centre for Coastal Health, president of the Canadian Association of Veterinary Epidemiology and Preventive Medicine, member of the Board of Directors of the McEachran Institute, member of the Advisory Council for Research Directions: One Health, and a member of the Royal Society of Canada One Health Working Group. Prior to February 2019, she was employed by the Public Health Agency of Canada. PSJ was funded via an ERC starting grant: INFLUX, grant number 101039376. I hold/have held grants as principal or co-investigator, from the following agencies and foundations all related to the topics of social-ecological systems and/or AMR: Swedish Research Council FORMAS, Wallenberg Foundations, IKEA Foundation, Erling Persson Family Foundation. Carolee A. Carson is employed by the Public Health Agency of Canada. In that role she has been a subject matter expert for the World Organisation for Animal Health and the World Health Organization (WHO). She is a member of the advisory committee for Animal Health Canada. She has previously been engaged in research funded by the Canadian Institutes for Health Research, Ontario Ministry of Agriculture, Food and Rural Affairs, and the Canadian Safety and Security Program. PJGH is partially funded by FORMAS Inequality and the Biosphere Project (2020-00454) and partially by CGIAR Trust Fund. Amy L. Greer, Elena Neiterman, Matthew N. Vanderheyden, Didier Wernli, and Shannon E. Majowicz declare no competing interests.
Ethics statement
Not applicable.
Comments
No accompanying comment.