Obesity and the closely related risk cluster of the metabolic syndrome (MS) increase cardiovascular (CV) risk, in turn raising the incidence and prevalence of CHD. Several major scientific bodies currently recommend a low-fat, high-carbohydrate, energy-deficient diet to manage weight and associated comorbidities(Reference Krauss, Eckel and Howard1–Reference Flock and Kris-Etherton3). On the other hand, diets rich in fat and protein, but low in carbohydrates, have become popular, with advocates de-emphasising the role of energy intake in weight gain. Several best-selling books endorse this strategy of carbohydrate reduction, and maintain that weight will be lost despite isoenergetic intake, accompanied by a reduction in CV risk. The low-carbohydrate diet score (LCDS) provides an effective tool to classify individuals according to their relative levels of fat, protein and carbohydrate consumption, embodied in a simple number(Reference Halton, Willett and Liu4). The LCDS is based on the percentage of energy consumed as carbohydrate, and reflects the concordance with low carbohydrate intake. The higher the individual score, the lower the carbohydrate, but the higher the protein and fat content, in that patient's diet. The LCDS has successfully been employed to show that a low-carbohydrate diet is positively associated with the risk of type 2 diabetes (T2D) in a large cohort of healthy men after 20 years of follow-up(Reference de Koning, Fung and Liao5).
Measurement of the peripheral arterial tonometry (PAT) hyperaemic response, embodied in the small artery reactive hyperaemia index (saRHI), is a relatively new technique considered a toll reflecting endothelial and other variables on small artery vascular function and dilation(Reference Kuvin, Patel and Sliney6–Reference Dhindsa, Sommerlad and DeVan9). The genesis, regulation and significance of changes in cutaneous digital hyperaemia differ from those affecting flow-mediated dilatation (FMD), and these determinants are not well known. We previously reported that high adherence to therapeutic lifestyle changes, including a Mediterranean dietary pattern, was associated with improved peripheral small artery vascular reactivity, as assessed by the saRHI, in conjunction with improvements in carotid intima–media thickness(Reference Ferre, Plana and Merino10). We also generated a hypothesis that chronic consumption of high percentages of dietary protein and fat, along with a low intake of carbohydrate, may lead to peripheral small artery vascular dysfunction. The aim of the present study was to evaluate the relation between dietary macronutrient composition, using the LCDS, on the saRHI in a group of patients with increased CV risk.
Subjects and methods
Design and study participants
This is a cross-sectional study in 247 men and women, aged 30–70 years, without a previous diagnosis of CVD, who attended the Vascular Medicine and Metabolism Unit of the University Hospital Sant Joan in Reus (Spain). Only patients with intermediate to high CV risk according to the Framingham risk score, who were diagnosed with diabetes or satisfied adult treatment panel III (ATPIII) criteria for the MS(Reference Grundy, Cleeman and Daniels11), were enrolled. We chose patients at intermediate to high risk primarily because re-classification of patients at this level is consistent with present practice guidelines, frequently leading to a change in therapy, and is therefore likely to be clinically meaningful and cost-effective. Patients with diagnosed CVD; chronic renal, hepatic, pulmonary or neurodegenerative problems; a history of prior neoplasia; or other serious chronic diseases were excluded. The present study was conducted according to the guidelines laid down in the Declaration of Helsinki and all procedures involving human patients were approved by the Ethical and Clinical Investigation Committee of the hospital. Written informed consent was obtained from all patients.
Clinical assessment
Each of our patients was evaluated with a review of lifestyle components (diet, physical activity and smoking), a complete physical examination including anthropometry, biochemical testing and vascular assessment with the saRHI. Physical activity was quantified in metabolic equivalent task/hours (MET/h) per week according to the Minnesota questionnaire adapted for a Spanish population(Reference Elosua, Garcia and Aguilar12). Tobacco status was assessed using standardised questionnaires administered by health care providers(Reference DiClemente, Prochaska and Fairhurst13). A 3-d food-intake register was used to evaluate the diet. The food data of dietary records were converted to energy and nutrient data by experienced dietitians and analysed using the PCN (CESNID Nutritional Program, version 2.0, University of Barcelona). The percentages of energy from carbohydrate, protein and fat were coded according to defined categories, using the LCDS method previously described by Halton et al. (Reference Halton, Willett and Liu4) in their Women's Health Study Cohort. Briefly, the carbohydrate categories were scored from 10 (lowest intake) to 0 (highest intake), whereas protein and fat categories were scored from 0 (lowest intake) to 10 (highest intake). Ranks were added to create a total score with a maximum value of 30, representing the highest intake of protein and fat and the lowest intake of carbohydrate (see Halton et al. (Reference Halton, Willett and Liu4), Table 1).
Table 1 Differences in demographic and lifestyle data according to low-carbohydrate diet score (LCDS) quartiles† (Mean values and standard deviations, percentages of patients, medians and interquartile ranges)

T2D, type 2 diabetes mellitus; MET, metabolic equivalent task.
Mean values were significantly different from Q1: *P< 0·05, **P< 0·005.
† Derived with an ANOVA or Wilcoxon test for continuous variables or χ2 test for categorical variables.
Peripheral small artery vascular reactivity assessment
The saRHI was measured using PAT technology (EndoPAT-2000; Itamar Medical Limited). Determinations were performed in a quiet room with a controlled temperature (22–24°C) after patients had fasted for 12 h and refrained from smoking or strenuous exercise for 24 h. The patients lay in a relaxed, quiet and evenly illuminated environment while the device recorded changes in pulse waves in the digital arteries. The technique involved in PAT has been described elsewhere(Reference Bonetti, Pumper and Higano14, Reference Hamburg, Keyes and Larson15). There is a 5 min baseline measurement, after which the cuff is inflated enough to produce ischaemia above baseline systolic pressure. After 5 min of occlusion, the cuff is rapidly deflated and PAT tracing is recorded for an additional 5 min. Blood flow measurements from two fingertips – one from each hand, one a test, the other a control – were compared after a stabilisation period, and a second comparison pair of measurements was taken before and after 5 min of brachial ischaemia in the test arm. The results were processed by specific software to calculate the post-ischaemia reflex vasodilatation observed when measurements from the test arm (before and after ischaemia) were compared to those from the control arm. The value generated was termed the saRHI. The variability of this technique in our laboratory was 17 %, the intra-class correlation coefficient was 0·52 and the within-subject variation was 0·19.
Biochemical determinations
Venous blood samples were obtained after a 12-h overnight fast, centrifuged immediately and stored at − 80°C until the assays were performed. Total cholesterol, TAG, glucose, direct LDL-cholesterol (LDL-C) and HDL-cholesterol (HDL-C), apoB100 and high sensitive C-reactive protein levels were measured using enzymatic and turbidimetric assays (Spinreact) adapted to the autoanalyser Cobas Mira Plus (Roche Diagnostics). Differential expression of human inflammatory cytokines was determined using the Human Cytokine Antibody Array (RayBiotech). The array is coated with six specific cytokine antibodies. It was probed with serum samples to allow for the comparisons of relative cytokine levels. The cytokine-antibody-biotin complex was visualised with the addition of a streptavidin-labelled fluorescent dye using a laser scanner.
Statistical analysis
Data are presented as means and standard deviations or as medians and interquartile ranges for continuous variables and as frequencies for categorical variables. Normality distribution of variables was assessed with the Kolmogorov–Smirnov test. Differences between diet, anthropometric, biochemical or vascular data were analysed using the ANOVA or Kruskall–Wallis test for continuous variables or χ2 test for categorical ones. Univariate association was tested by Spearman correlation analysis. A logistic regression model test was performed to assess the determinants of the saRHI in the present study group patients. The dependent variable of interest was the highest quartile of the saRHI defined as a quartile of individuals with a high saRHI. Independent variables were selected on the basis of univariate analysis and from the variables known to be associated with the dependent variable according to our previous studies. In this test, independent variables were age, sex, T2D, atherogenic dyslipidaemia defined as low HDL-C ( < 1·03 mmol/l in men and 1·29 mmol/l in women) and high TAG (>1·5 mmol/l), abdominal obesity and high blood pressure according to ATPIII criteria, high LDL-C ( ≥ 4·14 mmol/l), smoking, highest quartile of LCDS, highest quartile of physical activity, highest quartile of SFA intake, highest quartile of alcohol intake and highest quartile of fibre intake. P-values were calculated as two-sided; a P-value of less than 0·05 was considered statistically significant. SPSS version 19.0 (SPSS, Inc.) was used for all statistical analysis.
Results
Associations of low-carbohydrate diet score with biochemical and vascular data
The median LCDS in the present study group was 22 (sd 8) points. Participants were distributed into LCDS quartiles according to their macronutrient intake percentages (Table 1). The diet of individuals in the lowest LCDS quartile was composed of 45 % carbohydrate, 20 % protein and 32 % fat. Participants in the top quartile had an intake of 29 % carbohydrate, 24 % protein and 40 % fat. There were no differences between study participant quartiles other than the expected nutrient profile, as indicated in Table 1.
The differences in biochemical and vascular data between LCDS quartiles are shown in Table 2. We observed that individuals in the lowest quartile had a higher saRHI than individuals in the highest quartile (1·66 (sd 0·41) v. 1·52 (sd 0·22), P= 0·037). No significant differences were observed in the other vascular or biochemical parameters.
Table 2 Anthropometric, biochemical and vascular data between lowest and highest low-carbohydrate diet score (LCDS) (Mean values and standard deviations; medians and interquartile ranges)
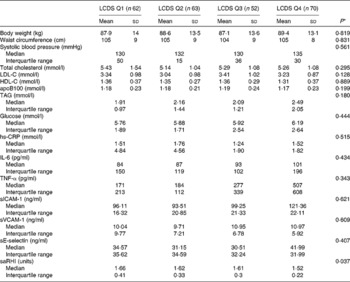
Q, quartiles; LDL-C, LDL-cholesterol; HDL-C, HDL-cholesterol; hs-CRP, high-sensitive C-reactive protein; sICAM-1, soluble intercellular adhesion molecule 1; sVCAM-1, soluble vascular adhesion molecule 1; sE-selectin, soluble E-selectin; saRHI, small artery reactive hyperaemia index.
* Differences regarding Q1 and Q4 study groups using one-way ANOVA or Kruskall–Wallis test.
Association of low-carbohydrate diet score, small artery reactive hyperaeamia index and metabolic diseases
An inverse association was observed between the LCDS and saRHI (r − 0·178, P= 0·005). A total of 160 participants fulfilled the ATPIII criteria for the MS. The MS patients in the lowest LCDS quartile (n 38) had higher saRHI values than the MS patients in the highest LCDS quartile (n 48; 1·82 (sd 0·32) v. 1·61 (sd.027), P= 0·021; Fig. 1). When the MS components were evaluated, the same association existed between each MS component, saRHI and the LCDS quartiles. The exception was in individuals with low HDL-C, in whom the saRHI was not different when comparing individuals according to the LCDS quartiles (1·74 (sd 0·35, n 9) v. 1·62 (sd 0·48, n 8), P= NS), as illustrated in Fig. 1.

Fig. 1 Differences in the small artery reactive hyperaemia index (saRHI) according to low-carbohydrate diet score (LCDS) quartile and the cardiovascular risk factors. □, LCDS quartile 1 patients; ■, LCDS quartile 4 patients. Data obtained with Kruskall–Wallis test. Values are medians with their interquartile ranges. * Median values were significantly different (P< 0·05). HDL-C, HDL-cholesterol; MS, metabolic syndrome; T2D, type 2 diabetes mellitus.
Among the eighty-four T2D patients, those in the lower quartile (n 21) had better saRHI values than those in the highest quartile (n 26; 1·78 (sd 0·31) v. 1·62 (sd 0·35), P= 0·011; Fig. 1). These results were not modified when pharmacological therapy was taken into account.
Determinants of small artery reactive hyperaemia index
To assess the effect of LCDS on the saRHI independent of potential confounders, a multivariate stepwise test was performed. We considered the highest quartile of the saRHI (2·14 (sd 0·39) as the dependent variable and age, sex, T2D, atherogenic dyslipidaemia defined as low HDL-C ( < 1·03 mmol/l in men and 1·29 mmol/l in women) and high TAG (>1·5 mmol/l), abdominal obesity and high blood pressure according to ATPIII criteria, high LDL-C ( ≥ 4·14 mmol/l), smoking, highest quartile of LCDS, highest quartile of physical activity (40 (sd 18) MET/h per week), highest quartile of SFA intake (11·6 (sd 2·8) g/d), highest quartile of alcohol intake (21 (sd 12) g/d) and highest quartile of fibre intake (22 (sd 7) g/d) as independent variables. As seen in Fig. 2, individuals in the highest LCDS quartile, as compared with the lowest quartile, had negative probabilities of high saRHI, with a estimated prognostic value of 75 % (OR − 0·85; 95 % CI 0·19, 0·92; P= 0·031). Saturated fat intake was not an independent predictor of saRHI (OR − 0·88; 95 % CI: 0·41, 1·93; P= 0·631).

Fig. 2 Determinants of small artery reactive hyperaemia index (saRHI). Multivariate stepwise binary logistic regression test. Dependent variable: highest quartile of saRHI (2·14 (sd 0·39)). Independent variables: age, sex, atherogenic dyslipidaemia (TAG>1·5 mmol/l and HDL-cholesterol < 1·03 mmol/l in men and 1·29 mmol/l in women), abdominal obesity and high blood pressure (BP) according to adult treatment panel III criteria, high LDL-cholesterol (LDL-C ≥ 4·14 mmol/l), smoking, highest quartile of low-carbohydrate diet score (LCDS), highest quartile of physical activity (40 (sd 18) metabolic equivalent task/h per week), highest quartile of SFA intake (11·6 (sd 2·8) g/d), highest quartile of alcohol intake (21 (sd 12) g/d) and highest quartile of fibre intake (22 (sd 7) g/d). Estimated prognostic value: 75 %. R 2 Nagelkerke: 0·62.
Discussion
Stratifying participants according to their unique dietary macronutrient composition (LCDS), we examined the impact of varying dietary carbohydrate content on small artery vascular function assessed by a recently homologated new tool, the saRHI. We observed that the higher the LCDS, reflecting a lower dietary carbohydrate content, the lower the small artery reactivity in the digital vascular bed. This inverse relationship was significant and independent of the analyses used and a characteristic of the metabolic risk factors, especially in patients with T2D, the MS or abdominal obesity. While differences in endothelial function (EF) biomarkers did not reach statistical significance, they varied in the same direction. In essence, patients consuming carbohydrate-poor diets had the worst EF profiles.
The literature suggests that diets with low or very low carbohydrate content may be associated with impaired small artery vascular function(Reference Dod, Bhardwaj and Sajja16–Reference Phillips, Jurva and Syed19). Randomised clinical trials have shown that weight loss per se is the main determinant of improvement in peripheral small artery reactivity using a low-carbohydrate diet or low-fat diet(Reference Foster, Wyatt and Hill20–Reference Ferrara, Innelli and Palmieri25). In fact, obesity and visceral adiposity are associated with endothelial dysfunction in resistance and conduit arteries, and weight loss brought about by lowered energy intake improves EF, even in the absence of heart disease or CV risk factors(Reference Pierce, Beske and Lawson26, Reference Brook27).
However, in clinical trials without changes in body weight, low-carbohydrate/high-protein diets may be significantly associated with twice the amount of arterial plaque, a reduction in the number of circulating and bone marrow endothelial progenitor cells in murine models of atherosclerosis(Reference Foo, Heller and Wykrzykowska28) and lower FMD in healthy and obese subjects(Reference Phillips29). One dissenting randomised clinical trial included forty obese and overweight subjects with hypertriacylglycerolaemia, showing an inverse association between carbohydrates and FMD. However, these data were collected postprandially and cannot be directly extrapolated to basal conditions(Reference Volek, Ballard and Silvestre30). The present results therefore reinforce the known negative impact of very low-carbohydrate diets on vascular function assessed by FMD under fasting conditions, using the PAT technique.
The components and variables affecting EF, assessed by FMD, as compared with PAT differ, and although there may be some correlation, the two techniques provide distinct information about vascular function in conduit arteries v. the peripheral small arteries of the finger(Reference Hamburg, Palmisano and Larson31).
The FMD is highly dependent on NO production, whereas the reactive hyperaemia index (RHI) is not, even though NO has been reported to contribute to RHI(Reference Celermajer8, Reference Bacon, Lavoie and Arsenault32). While the European Society of Cardiology classifies RHI with other techniques to measure ‘EF’(Reference Lekakis, Abraham and Balbarini33), the data available at present indicate that each of these methods probably has a unique blend of physiological determinants, and each provides particular information about different vascular beds(Reference Hamburg, Palmisano and Larson31, Reference Schnabel, Schulz and Wild34). A recent direct comparison of methods did not reveal a strong correlation(Reference Dhindsa, Sommerlad and DeVan9). However, FMD is difficult to perform compared to the RHI, and the correlation between the two and other data derived are sufficient to continue clinical investigation(Reference Dhindsa, Sommerlad and DeVan9, Reference Schnabel, Schulz and Wild34). Indeed, the saRHI correlates with coronary endothelial dysfunction(Reference Bonetti, Pumper and Higano14), accurately measures vascular effects when the net CV risk burden improves(Reference Chouraqui, Schnall and Dvir35), correlates with carotid intima–media thickness and can predict future adverse CV events(Reference Rubinshtein, Kuvin and Soffler36). Information from non-invasive PAT studies is useful to assess static or serial vascular function in a variety of patient phenotypes with raised CV risk(Reference Plana, Ferre and Merino37).
Our group has shown that saRHI-measured endothelial dysfunction is also associated with increased soluble E-selectin plasma concentrations, suggesting that at least some mechanisms are shared by both large and small artery function(Reference Versari, Daghini and Virdis38). In patients without the MS but with risk-related elevations in soluble E-selectin and soluble vascular adhesion molecule 1, there was a strong correlation between saRHI values and levels of oxidised LDL(Reference Aragones, Ferre and Girona39). A uniting theme is that the saRHI is clearly associated with metabolic parameters, mainly waist circumference, and plasma concentrations of TAG, HDL-C and apoA1(Reference Ferre, Aragones and Plana40). In the present study, we observed significant differences in saRHI between LCDS quartiles composed of hypertriacylglycerolaemic and abdominal obesity subjects; a lack of a similar relationship in subjects with low HDL-C might be due to the small sample size of patients with low HDL-C criteria (Q1 = 9 and Q4 = 8).
Limitations
The present study had other limitations. One was related to the score used in the study and the cut-off values. The LCDS was performed using US population data, and the percentiles of diet relate to healthy American white men and women. However, as the diet consumed by the present study population was similar to the diet of a corresponding American population with equally high CV risk(Reference Yeboah, Crouse and Hsu41), the comparison is reasonable. We did not grade or independently consider dietary amounts of animal or vegetable protein or dietary fat, as the primary focus was the influence of low carbohydrate intake on small artery vascular reactivity. As the study was conducted in patients with increased CV risk, results cannot be generalised to healthy individuals. The present study design did not control all possible confounding variables. Errors associated with completion of food diaries are possible. Although not described, either the pharmacological agents that our patients were taking themselves, or interaction with macronutrient changes, could have influenced EF. Uncontrolled dietary ingredients, such as n-3 fatty acids and polyphenols, could potentially confound the results. Finally, a cross-sectional study cannot establish causality. In view of the strength of our main finding, the quantitative importance of these limitations appears small.
Conclusion
In a cross-sectional study of patients with increased CV risk, a dietary pattern characterised by a high LCDS (high protein and fat, but low carbohydrate content) was associated with poorer peripheral small artery function compared with individuals consuming a diet with a lower LCDS. The association was strong in patients with two different metabolic diseases studied: the MS and T2D. This relationship needs confirmation with larger cohorts in randomised clinical trials.
Acknowledgements
The present work was supported by grants from ISCIII, Madrid, Spain (PI 05/1954). CIBERDEM is an initiative of ISCIII, Spain. The authors declared no conflict of interest. The authors’ responsibilities were as follows: R. F., N. P. and L. M. designed the study; J. M., G. A., R. F. and N. P. conducted research; M. H. performed the biochemical analyses; J. M., R. F. and L. M. performed the statistical tests and wrote the final manuscript. R. K. reviewed the manuscript, and contributed significantly to the final form of the introduction and the discussion. All authors read and approved the final manuscript.