Introduction
Climate change is recognized as being one of the main threats to global biodiversity and yet for many species its effects are largely unknown (McMahon et al., Reference McMahon, Harrison, Armbruster, Bartlein, Beale and Edwards2011). There is increasing evidence that species are changing their distribution as a result of environmental warming, particularly in montane ecosystems (Nogués-Bravo et al., Reference Nogués-Bravo, Araújo, Errea and Martínez-Rica2007; La Sorte & Jetz, Reference La Sorte and Jetz2010), and that organisms living at high altitudes are particularly at risk because of their restricted climatic niches and specific adaptations. These species also tend to occur over small areas, and many are endemic. Studies indicate that across mountain regions plants and animals are shifting their distributions upwards as a result of global warming (e.g. Parmesan, Reference Parmesan2006; Seimon et al., Reference Seimon, Seimon, Daszak, Halloy, Schloegel and Aguilar2007; Lenoir et al., Reference Lenoir, Gégout, Marquet, de Ruffray and Brisse2008) and becoming trapped in mountaintop refugia, in progressively smaller and more fragmented populations, which increases their likelihood of extinction as a result of demographic stochasticity and reduced genetic variability.
Predicting the potential distributions of threatened species in future climates can provide useful insights into their responses to climate change (Guisan & Thuiller, Reference Guisan and Thuiller2005; Franklin & Miller, Reference Franklin and Miller2009) and the degree to which suitable habitats may be protected within existing networks of protected areas (e.g. Zimbres et al., Reference Zimbres, de Aquino, Machado, Silveira, Jácomo and Sollmann2012). As species show individualistic responses to climate change, species distribution models are better implemented at single species level (Thomas et al., Reference Thomas, Cameron, Green, Bakkenes, Beaumont and Collingham2004; Pearman et al., Reference Pearman, D'Amen, Graham, Thuiller and Zimmermann2010; Swab et al., Reference Swab, Regan, Matthies, Becker and Bruun2015). On the other hand, models developed for rare highland species, for example, will also provide insight into the responses of organisms with similar adaptations, and for which physiological and distributional data are also generally scarce.
This is the case for the Andean cat Leopardus jacobita in South America, which is categorized as Endangered on the IUCN Red List (Villalba et al., Reference Villalba, Lucherini, Walker, Lagos, Cossios, Bennett and Huaranca2016) and is one of the least known felids (Brodie, Reference Brodie2009). The climatic niche of the Andean cat is characterized by arid and cold conditions, predominating in the High Andes of Argentina, Bolivia, Chile and Peru, and in areas of Northern Patagonia in Argentina at the southern tip of the species’ range (Marino et al., Reference Marino, Bennett, Cossios, Iriarte, Lucherini and Pliscoff2011). We expect Andean cat habitats to contract as low temperatures advance up the Andean slopes, yet the impacts of climate change may vary across its distribution (e.g. as for the mountain hare Lepus timidus, Bisi et al., Reference Bisi, Wauters, Preatoni and Martinoli2015), given that the species’ range spans latitudes of 10–40°S over 3,400 km, dropping towards lower altitudes in Northern Patagonia. Considering its vulnerability to warming, evaluating future shifts in suitable conditions can have important implications for the conservation of the species. The long-term survival of the Andean cat may depend on our ability to adjust conservation planning to protect habitat relicts and/or new suitable areas, and thus also protect other Andean species of conservation concern, such as the vicuña Vicugna vicugna, the chinchillas Chinchilla lanigera and Chinchilla brevicaudata, and the queñoa tree Polylepis tarapacana.
We developed species distribution models to estimate changes in the suitability of habitats for the Andean cat over time, given various climate change scenarios, with a view to applying them for conservation planning. To assess levels of protection for the species in the future we tested alternative thresholds for binary predictions (habitat–non habitat) and overlaid a dataset of existing protected areas. However, producing accurate predictions of the distribution of rare species in future climates is a challenging task, complicated by incomplete knowledge of biotic factors and interactions, and uncertainties about projected climates and extrapolations (Soberón & Nakamura, Reference Soberón and Nakamura2009; Wiens et al., Reference Wiens, Stralberg, Jongsomjit, Howell and Snyder2009; Mesgaran et al., Reference Mesgaran, Cousens and Webber2014). To counteract these limitations, we analysed a robust set of Andean cat presence records (Marino et al., Reference Marino, Bennett, Cossios, Iriarte, Lucherini and Pliscoff2011) and predicted habitat suitability using the Maximum Entropy algorithm (Elith et al., Reference Elith, Graham, Anderson, Dudik, Ferrier and Guisan2006; Phillips et al., Reference Phillips, Anderson and Schapire2006), which is proven to perform well when applied to specialized organisms with small sample sizes, such as the Andean cat (Hernandez et al., Reference Hernandez, Graham, Master and Albert2006; Wisz et al., Reference Wisz, Hijmans, Li, Peterson, Graham and Guisan2008). We used an improved regional climatic dataset (Pliscoff et al., Reference Pliscoff, Luebert, Hilger and Guisan2014) and measured the extent of extrapolation into novel climates (Elith et al., Reference Elith, Kearney and Phillips2010). Benefiting from a previous ecological niche model (Marino et al., Reference Marino, Bennett, Cossios, Iriarte, Lucherini and Pliscoff2011), we selected biologically meaningful climatic predictors, and controlled for overfitting and robust transferability (Warren & Seifert, Reference Warren and Seifert2011; Syfert et al., Reference Syfert, Smith and Coomes2013; Moreno-Amat et al., Reference Moreno-Amat, Mateo, Nieto-Lugilde, Morueta-Holme, Svenning and García-Amorena2015). We also modelled future habitat suitability for the mountain viscacha Lagidium viscacia, the main prey of the Andean cat (Walker et al., Reference Walker, Novaro, Perovic, Palacios, Donadio and Lucherini2007; Napolitano et al., Reference Napolitano, Bennett, Johnson, O'Brien, Marquet and Barria2008; Marino et al., Reference Marino, Lucherini, Villalba, Bennett, Cossíos, Iriarte, Macdonald and Loveridge2010), as its capacity to track changes in climate will have implications for the future availability of this critical prey resource (Morin & Lechowicz, Reference Morin and Lechowicz2008).
Consequential changes in the representativeness of suitable habitats within existing protected areas can have important implications for the conservation of the Andean cat and other Andean species. We propose research and monitoring priorities to improve the robustness of species distribution models and to enhance our capacity for adaptive conservation in the face of climate change.
Study area and species
The study area spans > 3,400 km along the Andes Cordillera, comprising areas of the Puna ecoregion in Argentina, Bolivia, Chile and Peru, and the steppes of Northern Patagonia in Argentina (Marino et al., Reference Marino, Bennett, Cossios, Iriarte, Lucherini and Pliscoff2011). In these arid regions the vegetation is sparse, dominated by grasslands and scrublands, with patches of greener vegetation associated with wetlands. Human habitation is low and land uses are restricted to mining and small-scale agriculture and pastoralism (llamas). The Dry Puna contains numerous endemic species adapted to extreme temperature, aridity and altitude, such as the vicuña and the queñoa tree. There is a high diversity of aquatic birds in lagoons and saltpans, including three sympatric species of flamingos (Phoenicopterus chilensis, P. andinus and P. jamesi). Comparatively less is known about the populations of carnivores, among them the Andean cat, the Pampas cat Leopardus colocolo, the culpeo fox Pseudalopex culpaeus and the puma Puma concolor.
The Andean cat is a small felid (4–4.5 kg) typically associated with rocky outcrops in the Andes of Argentina, Bolivia, Chile and Peru (Yensen & Seymour, Reference Yensen and Seymour2000; Villalba et al., Reference Villalba, Lucherini, Walker, Cossios, Iriarte and Sanderson2004; Marino et al., Reference Marino, Lucherini, Villalba, Bennett, Cossíos, Iriarte, Macdonald and Loveridge2010). Its diet is dominated by rodents and birds, but mountain viscachas (2–2.5 kg) contribute the most biomass across Andean cat populations (Walker et al., Reference Walker, Novaro, Perovic, Palacios, Donadio and Lucherini2007; Napolitano et al., Reference Napolitano, Bennett, Johnson, O'Brien, Marquet and Barria2008; Marino et al., Reference Marino, Lucherini, Villalba, Bennett, Cossíos, Iriarte, Macdonald and Loveridge2010). Mountain viscachas are present across the Southern Andes and in the Northern Patagonian steppes (Walker et al., Reference Walker, Novaro and Branch2003). They are generalist herbivores that use rocky outcrops to rest, sunbathe, and escape predators (Walker et al., Reference Walker, Pancotto, Schachter-Broide, Ackermann and Novaro2000).
Methods
Presence data
The Andean cat presence records used include data collected by the authors and other members of the Andean Cat Alliance during 1988–2010 (Marino et al., Reference Marino, Bennett, Cossios, Iriarte, Lucherini and Pliscoff2011; Supplementary Material 1). We do not expect this temporal breadth to include a period of significant change in distribution, as Andean cats live in remote and hostile environments with minor human interference. The dataset includes direct sightings, genetically identified faeces, camera-trap photographs, skins and skulls, all of which underwent a rigorous process of quality control. As Andean cats are elusive and exist at low density, their detectability is low. Thus the records available tend to be clustered around the focal areas of research and conservation programmes, typically optimal habitats (Fig. 1a). To control for this geographical bias we subsampled the dataset, over-imposing a 3-km grid and selecting at random one record per cell (Kramer-Schadt et al., Reference Kramer-Schadt, Niedballa, Pilgrim, Schröder, Lindenborn and Reinfelder2013). As a result, the dataset was reduced to 135 presence points. The spatial filter reduced autocorrelation amongst predictors (see below), except for Mean Diurnal Temperature Range and Precipitation Seasonality, for which autocorrelation remained significant (see Supplementary Material 2 for Moran's indexes).
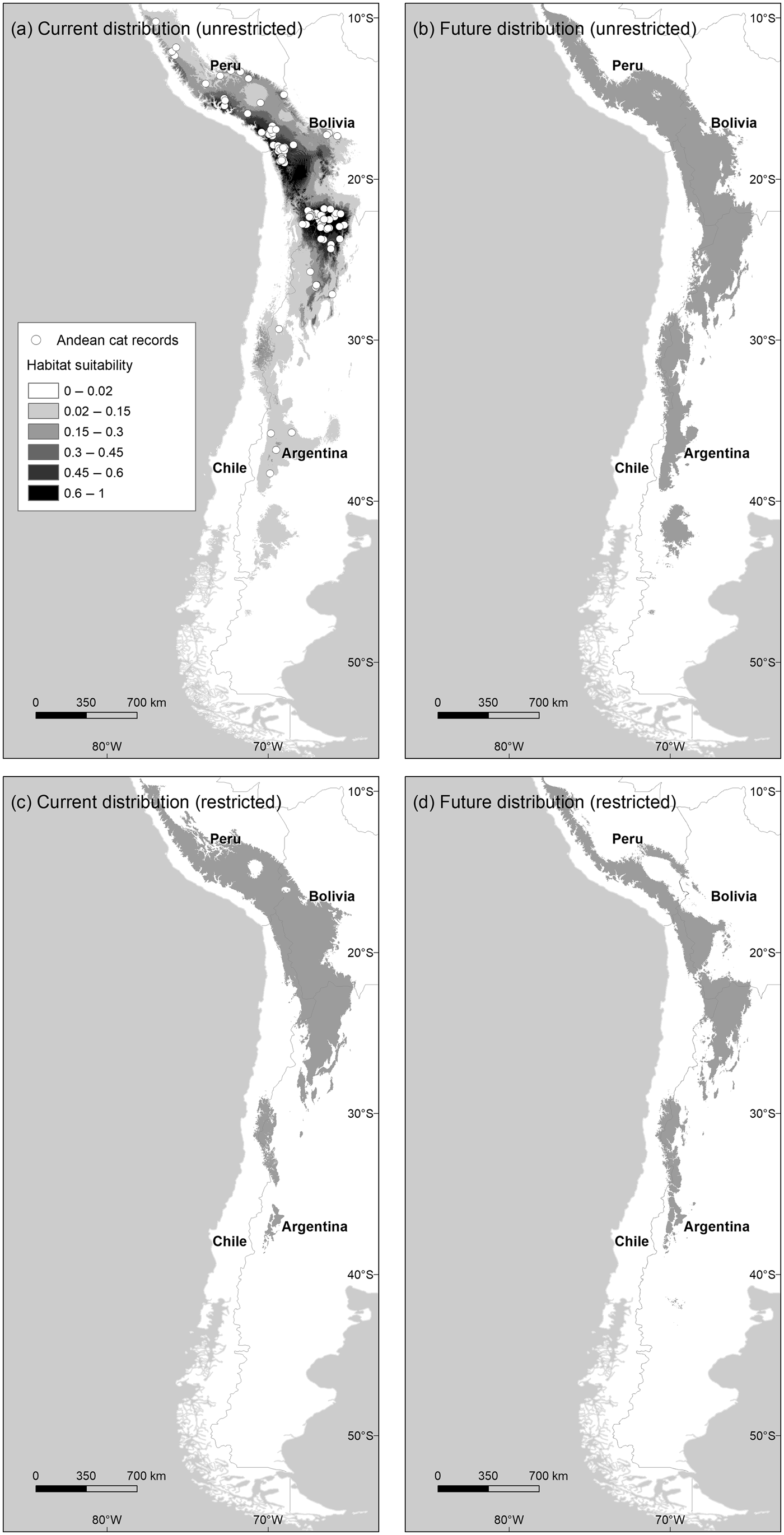
Fig. 1 Distribution of habitats suitable for Andean cats Leopardus jacobita under current and future (2080 A2) climatic conditions, using the unrestricted (a and b) and restricted (c and d) habitat suitability thresholds. Map (a) also shows the Maxent logistic output above the habitat cutting point, and the locations of 135 Andean cat presence records used to train the model.
The dataset of mountain viscacha records comprised published data (Marquet et al., Reference Marquet, Abades, Armesto, Barria, Arroyo and Cavieres2010), data collected by the authors and the Andean Cat Alliance, and data from the Global Biodiversity Information Facility (GBIF, 2014; sources listed in Supplementary Material 1). The data span 1980–2012 and include direct sightings and the location of faecal pellets; these are easily identified in the field because of their relatively large size in comparison to those of other rodents, and their typical disposition in piles in rocky outcrops (Walker et al., Reference Walker, Pancotto, Schachter-Broide, Ackermann and Novaro2000). In the case of the external data (GBIF, 2014) we corroborated that all presence records fell within the known range of the species. A 3-km spatial filter was also implemented to minimize the negative impacts of sampling biases, resulting in a database of 230 location points.
Climatic variables
Data from 19 climatic variables at 1-km spatial resolution were obtained from climatic surfaces produced by Pliscoff et al. (Reference Pliscoff, Luebert, Hilger and Guisan2014), who improved the Hadley Centre Coupled Model v. 3 (HADCM3) by including additional information from 930 weather stations in Chile, Peru, Bolivia and Argentina. We considered climatic scenarios for 2080, and two levels of CO2 emissions: A2 (high emissions) and B2 (low-to-medium emissions). Although other models and emissions scenarios exist (e.g. IPCC, Reference Stocker, Qin, Plattner, Tignor, Allen and Boschung2013), Pliscoff's dataset is the only one enhanced with local meteorological data, a critical step for the modelling of species distributions in mountains, where climate is not easily interpolated (e.g. Bennett et al., Reference Bennett, New, Marino and Sillero-Zubiri2016, found poor agreement between empirical and climate surfaces in the Andean Puna).
Model development and validation
To predict the potential distributions of Andean cats and mountain viscachas we applied a maximum entropy algorithm using Maxent 3.3.0 (Phillips et al., Reference Phillips, Anderson and Schapire2006). Maxent is widely used to predict relative habitat suitability from presence data, and is comparatively more successful than alternative approaches when applied to specialized species (Elith et al., Reference Elith, Graham, Anderson, Dudik, Ferrier and Guisan2006; Phillips et al., Reference Phillips, Anderson and Schapire2006) and species with narrow ranges and/or small sample sizes (Wisz et al., Reference Wisz, Hijmans, Li, Peterson, Graham and Guisan2008). Decisions about model complexity, sampling biases, study area and biological assumptions, however, will determine the robustness of Maxent and other species distribution models, including their transferability across space and time (Barbet-Massin et al., Reference Barbet-Massin, Jiguet, Albert and Thuiller2012; Braunisch et al., Reference Braunisch, Coppes, Arlettaz, Suchant, Schmid and Bollmann2013; Syfert et al., Reference Syfert, Smith and Coomes2013; Moreno-Amat et al., Reference Moreno-Amat, Mateo, Nieto-Lugilde, Morueta-Holme, Svenning and García-Amorena2015). Within the limitations of relatively small samples, we accounted for these factors during model development and validation.
Firstly, we selected variables representing the Andean cat's climatic niche and indicative of the species’ tolerance for cold temperatures and aridity (following Marino et al., Reference Marino, Bennett, Cossios, Iriarte, Lucherini and Pliscoff2011). We reduced co-linearity by excluding pairs of variables with Pearson's correlation > 0.7, and built and compared models with various combinations of predictors (Supplementary Material 3). To avoid overfitting we selected the simplest Maxent features, namely linear, quadratic and product feature types, and maintained the default regularization at its default value (ß multiplier = 1, as recommended by Phillips & Dudik, Reference Phillips and Dudik2008). For each model we ran 1,000 iterations and 10 replicates, averaged in the final model. The extent of the background (latitude 10–55°S and longitude 63–80°W) included the known distribution of both the Andean cat and the mountain viscacha, and a good representation of the asymmetric climatic conditions at each side of the Andean Cordillera, as well as the Patagonian steppes. For each model replicate we selected 10,000 random background pseudo-absences, a sample demonstrated to provide higher accuracy in Maxent models (Phillips & Dudik, Reference Phillips and Dudik2008); the random selection of a large number of pseudo-absences is known to maximize model specificity (Barbet-Massin et al., Reference Barbet-Massin, Jiguet, Albert and Thuiller2012), a desired outcome given conservation planning objectives (see threshold justification below).
To assess model performance, we split the training (80%) and testing (20%) datasets randomly and evaluated the resulting AUC (area under the receiver operating curve) values; values > 0.75 are considered to be indicative of robust models (Elith & Burgman, Reference Elith, Burgman, Scott, Heglund, Morrison, Haufler, Raphael, Wall and Samson2002; Franklin & Miller, Reference Franklin and Miller2009). As AUC measures the model's ability to distinguish test sites from random pseudo-absences, it is more difficult to interpret than in the presence–absence case (Phillips et al., Reference Phillips, Anderson and Schapire2006) and when the objective is to select among projections under future climatic scenarios. In the latter case, studies comparing selection based on AUC and on Akaike information criterion have used known past distribution or a true distribution of a virtual species as validations (Warren & Seifert, Reference Warren and Seifert2011; Moreno-Amat et al., Reference Moreno-Amat, Mateo, Nieto-Lugilde, Morueta-Holme, Svenning and García-Amorena2015). These studies demonstrated that models of intermediate complexity provide the best trade-off to predict species distributions across time; over-parameterized models tend to underestimate the availability of suitable habitat when transferred into a new time period, and under-parameterized models tend to overestimate it, even when they show identical behaviour in the present. We therefore selected for our projections a model of intermediate complexity from a set of candidate models (Supplementary Material 3). All models produced similar results but the selected one was the most realistic (others predicted suitable habitat in southern Patagonia not known to be occupied by Andean cats). All alternative models had high values of AUC (> 0.94, both for training and testing datasets), the highest corresponding to the model with most predictors (Supplementary Material 3). The six variables in the selected model were suitable descriptors of arid conditions (i.e. little rainfall and mostly during the summer months: Annual Precipitation, Precipitation Seasonality, and Precipitation of the Coldest Month) and of marked variations in temperature (i.e. broad diurnal ranges and extreme cold weather: Mean Diurnal Range, Temperature Seasonality, and Minimum Temperature of the Coldest Month). We considered models that were different from those published previously (Marino et al., Reference Marino, Bennett, Cossios, Iriarte, Lucherini and Pliscoff2011) because correlations between variables changed with the use of the corrected climatic surfaces; as a result some combinations were excluded but the variables originally identified as being most important were maintained. Following a similar process we selected five climatic predictors for the mountain viscacha model (Mean Diurnal Range, Temperature Seasonality, Minimum Temperature of Coldest Month, Mean Temperature of Wettest Quarter, and Precipitation of Warmest Quarter).
High predictive power (high AUC values) can be expected because Andean cats have a narrow climatic niche, and not necessarily as a result of overfitting. Efforts were made to avoid overfitting (see above) and we obtained smooth response curves in the models despite the geographically biased sample (Supplementary Material 4 & 5). It is possible that a relatively large background contributed to the model's high performance, as this encompasses neighbouring areas where Andean cats are not known to occur at present (e.g. towards the Atacama Desert in Chile and the Amazon in Peru). This choice of background, however, facilitated capturing broad physiological responses of the species, as opposed to more local idiosyncratic effects driven by the clustered sample, and predicting future suitable habitats outside the current known range of the species. To project the models under future climatic conditions we used clamping, the default setting in Maxent, to minimize extrapolation errors (by making the response constant outside the range of the training data; Elith et al., Reference Elith, Kearney and Phillips2010). To analyse the extent of extrapolation (i.e. when models predicted areas with climatic conditions not sampled in the presence data) we used multivariate environmental similarity surfaces, implemented in Maxent. These measure similarity between new climates and those in the training sample, by comparing any given point to a reference set of points for a given predictor (giving negative values for dissimilar points) and mapping these values across the prediction region (Elith et al., Reference Elith, Kearney and Phillips2010).
Thresholds and habitat shifts
We selected two commonly used thresholds to convert the continuous Maxent output into binary ‘suitable’ or ‘unsuitable’ habitat points for further analysis; in this way we tacitly accounted for this source of uncertainty and adapted interpretation to our objectives. (1) The Minimum Training Presence threshold (hereafter referred to as unrestricted threshold) corresponds to the lowest presence probability value. This cutting point ensures zero omission errors and maximum sensitivity, and maintains all areas that were at least as suitable as those where the Andean cat is known to be present (Hernandez et al., Reference Hernandez, Graham, Master and Albert2006; Pearson et al., Reference Pearson, Raxworthy, Nakamura and Peterson2007; Cordeiro et al., Reference Cordeiro, Fragoso, Crawshaw and Oliveira2016). This results in less restricted distributions and helps to build a hypothesis about the potential suitability of poorly surveyed areas. (2) The Maximum Training Sensitivity plus Specificity threshold (restricted threshold) produces more restrictive distributions while still accommodating survey gaps and imperfect detection (recommended by Liu et al., Reference Liu, Berry, Dawson and Pearson2005). As our objective is to predict whether protected areas will continue to protect Andean cats in the future, indicating where protected areas could be created or extended, higher specificity is favourable (using a higher threshold for correctly classifying presences, as the cost of concluding that an area is suitable when it is not is higher than the converse).
The model selected for the habitat projections also had the advantage of providing the highest value of the restricted threshold and the lowest value of the unrestricted threshold from among the set of alternative models, and was therefore a good compromise between over and under-predicting (Supplementary Material 3). Using the two binary maps we measured spatial changes in habitat suitability under the various climatic scenarios, in terms of area and with respect to latitude and altitude (derived from the Shuttle Radar Topography Mission; Rabus et al., Reference Rabus, Eineder, Roth and Bamler2003).
Representativeness within protected areas
We overlapped the IUCN World Database of Protected Areas (WDPA, 2009) with the projected distributions of Andean cat habitats under current and future climatic conditions, to calculate the representation of climatically suitable habitats for Andean cats within the network of protected areas in Argentina, Bolivia, Chile and Peru. The original WGS84 datum of the IUCN layers was projected to Universal Transverse Mercator coordinates for calculations. All spatial analyses were performed in ArcGIS 10 (ESRI, Redlands, USA).
Results
Habitat suitability projections
The model describing current habitat suitability for the Andean cat has as its main predictors the Minimum Temperature of the Coldest Month, Precipitation Seasonality, and Precipitation of Coldest Quarter, the first two having the most useful and unique information (Supplementary Material 4). The model predicted between 192,643 km2 and 147,854 km2 of suitable habitat in South America (unrestricted and restricted suitability thresholds, respectively); the largest extension was found in Peru and Bolivia, or Argentina, depending on the cutting point (Fig. 2). The predictive map revealed a cluster of highly suitable habitats, or core range, in the Central Andes, encompassing the central and northern populations of Andean cats, and a southern range, with less suitable habitats and at lower altitude (Fig. 1a,c; Table 1). The extent of suitable habitat predicted varied with the choice of suitability threshold (Fig. 2), particularly the southern areas in Northern Patagonia, which were better represented and 400 m lower, on average, in the less conservative scenario.
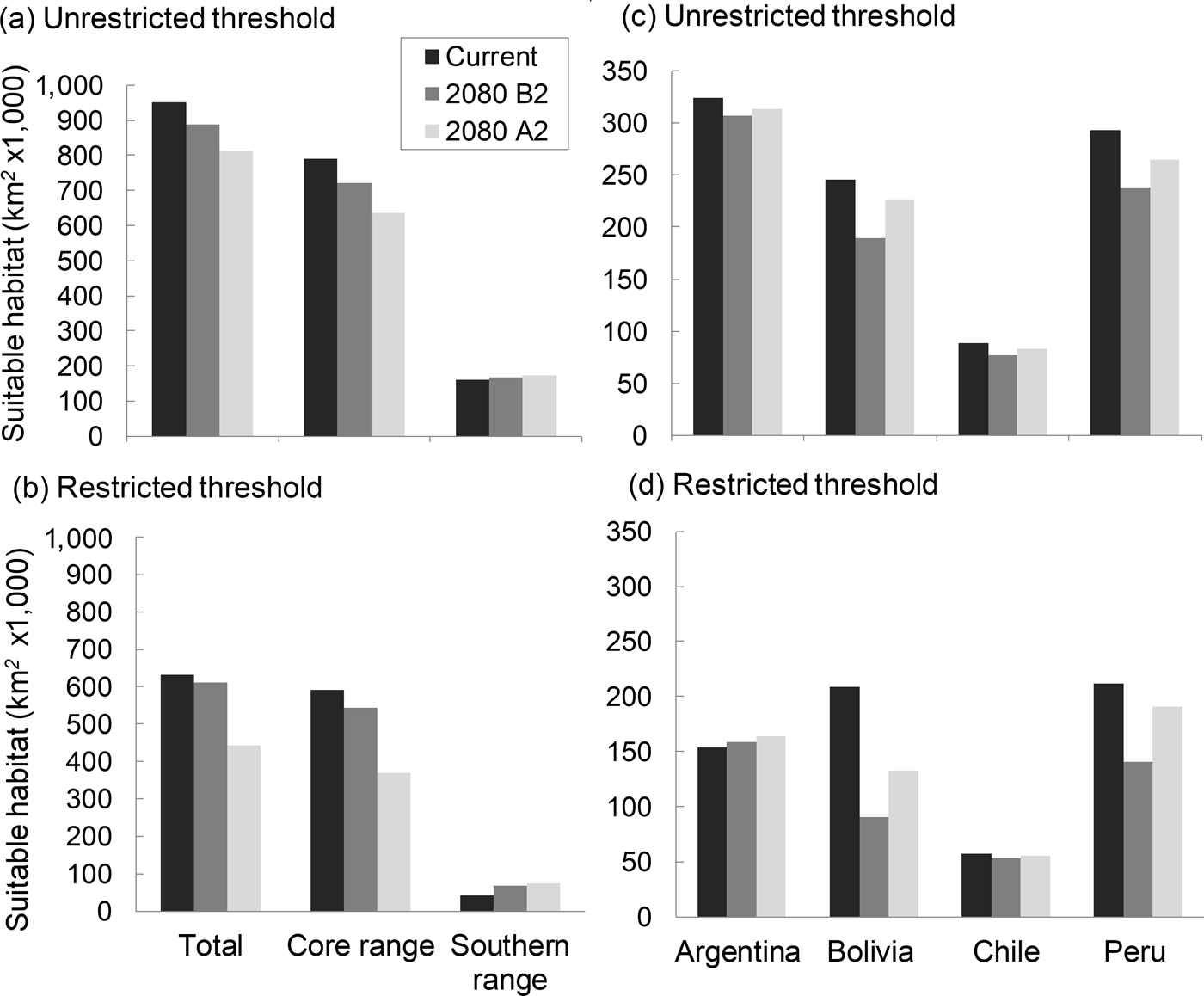
Fig. 2 Distribution of habitats climatically suitable for Andean cats, according to distribution ranges (a and b) and country (b and c), using the restricted and unrestricted habitat suitability thresholds.
Table 1 Mean altitude of the area climatically suitable for Andean cats Leopardus jacobita using two habitat suitability thresholds, under current climate conditions and in 2080 under two carbon emissions scenarios: B2 (low to medium) and A2 (high) (Pliscoff et al., Reference Pliscoff, Luebert, Hilger and Guisan2014).

By 2080 the area of suitable habitat for the Andean cat may have declined by 14.7–29.8% (according to the unrestricted and restricted thresholds, respectively) under the most pessimistic emissions scenario (Figs 1 & 2), but when considered in isolation, suitable areas in the southern range were predicted to expand (Fig. 2). Concomitantly, suitable habitats were predicted to shift upwards by c. 100 m, on average, with the maximum displacement predicted for the core range (225 m, compared with 111 m for the southern range) (Table 1). The models also predicted a mean displacement of suitable habitats of 80–180 km southwards (Table 2); this shift was more evident in the southern range, with the less restrictive habitat threshold, but affected mostly the core range when the more conservative suitability threshold was applied, as this results in more habitat loss in the northern extreme of the range (Table 2). The multivariate environmental similarity surface analyses detected novel climatic conditions in only 1.2–3.3% of the area predicted to be suitable for the Andean cat in 2080 (with the more or less conservative thresholds; Supplementary Material 6).
Table 2 Predicted latitudinal shifts in the habitats suitable for Andean cats by 2080, calculated as the distance between the centroids of current and future (2080) habitat polygons defined by the unrestricted and restricted suitability thresholds. Negative values indicate southwards displacement.

The habitats predicted to be suitable for the mountain viscacha (model AUC 0.923, SD 0.022) were characterized by high variation in temperature, both daily and yearly (Supplementary Material 5). With the current climate, suitable areas for the viscacha overlapped extensively with those for the Andean cat (56%) but extended more broadly into the Southern Andes. The restricted suitability threshold produced more realistic predictions and this was applied for further analyses. The projection based on future scenarios of climate change indicated a major contraction in the northern range of the mountain viscacha (north of 20°S latitude), and also in the southern extreme (south of 40°S) (Fig. 3), which would result in a 46% decline in overlapping areas between the Andean cat and its main prey (Table 3).

Fig. 3 Distribution of habitats suitable for mountain viscachas and Andean cats under (a) current and (b) future (2080 A2) climatic conditions, using the restricted habitat suitability threshold.
Table 3 Area and mean altitude of habitats suitable for mountain viscachas Lagidium viscacia and for both mountain viscachas and Andean cats, under present and future climatic conditions (2080 A2), using the unrestricted and restricted habitat suitability thresholds.

Representativeness within protected areas
Suitable habitats for the Andean cat overlapped with 47 protected areas, of which 14 contained presence records of the species. Under the current climate, Andean cat habitats are proportionally less well represented within the network of protected areas of Chile and Peru (5,771 km2 in five protected areas and 5,910 km2 in 9 protected areas, respectively), compared with Argentina and Bolivia (24,598 km2 in 14 protected areas and 19,087 km2 in 19 protected areas, respectively; Tables 4 & 5), in all cases following the results of the restricted suitability threshold.
Table 4 Percentage loss of suitable Andean cat habitat, and of overlapping Andean cat and mountain viscacha habitat, in Argentina, Bolivia, Chile and Peru, based on current and future (2080 A2) climatic conditions, using restricted and unrestricted habitat suitability thresholds.
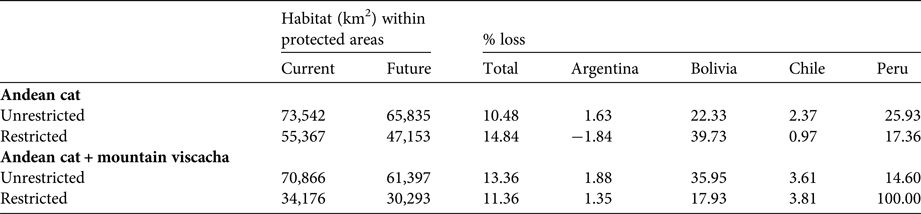
Table 5 Numbers of protected areas in Argentina, Bolivia, Chile and Peru containing suitable habitat for Andean cats, and for both Andean cats and mountain viscachas, under present and future (2080 A2) climatic conditions, using unrestricted and restricted habitat suitability thresholds.

By 2080 representativeness within protected areas is predicted to decline by 11–15%, depending on the threshold used, with the largest declines in habitat protection predicted for Bolivia (40%) and Peru (25%). With the more conservative prediction, the number of protected areas would diminish by five, from 47 to 42, with Bolivia losing eight and Peru two, but Chile adding four and Argentina one. The less conservative threshold predicts 11 fewer protected areas (64 to 53) and more evenly distributed losses, including three in Argentina.
Areas with overlapping suitability for the Andean cat and the mountain viscacha are predicted to decline by 11–13% in 2080 (highest decline in Bolivia, with 18%); considering the more conservative habitat threshold, these would be found in 19 protected areas, compared with 22 at present. Five protected areas in Bolivia, and all in Peru, will no longer encompass these overlapping areas. The unrestricted threshold predicts a decrease of 12 protected areas across the range (Table 5).
Discussion
The climatic niche of the Andean cat is present along Andean grasslands and scrublands, from the Wet Puna in Peru through the Dry Puna, and south into the Patagonian steppes, corresponding to arid and cold climates, with dry winters and extreme daily variations in temperature. In the coming decades climate change may restrict these climatic conditions to smaller areas, with implications for the effectiveness of existing protected areas in protecting threatened species such as the Andean cat. Our species distribution modelling to extrapolate the climatic niche of this rare and poorly known felid, and that of its prey, into the future shows that Andean cats are susceptible to the effects of climate change and that by 2080 they may be displaced from up to a third (10–30%) of their current range. Although measurements of habitat loss and of displacement varied across climatic scenarios and methods of habitat thresholding, the patterns of predicted changes were consistent.
As expected, the model predicted an upwards shift of the climatic conditions suitable for the Andean cat in response to climate change, a phenomenon described for other specialist organisms living in mountains, including plants (Lenoir et al., Reference Lenoir, Gégout, Marquet, de Ruffray and Brisse2008) and bird species (Buermann et al., Reference Buermann, Chaves, Dudley, McGuire, Smith and Altshuler2011). This kind of displacement results from increasing temperatures pushing organisms to higher elevations on mountains, without creating openings for colonization elsewhere. In the case of Andean species, however, land at c. 5,000 m and above, virtually cold deserts, may become more suitable in the future. The simultaneous polewards displacement may also open up new suitable areas for the Andean cat at the southern part of its range, descending into the arid Patagonian steppes, whereas the northern ranges are predicted to contract the most. Such latitudinal displacements are known to affect other species (e.g. Parmesan & Yohe, Reference Parmesan and Yohe2003; Chen et al., Reference Chen, Hill, Ohlemüller, Roy and Thomas2011; Luo et al., Reference Luo, Jiang and Tang2015), and illustrate the diversity of potential impacts of climate change on a single species, including on species adapted to highlands (e.g. the mountain hare, Bisi et al., Reference Bisi, Wauters, Preatoni and Martinoli2015).
In understanding the responses of carnivores to climate change it is crucial to consider prey–predator dynamics, as their options will be modulated by contemporary changes in the distribution of their prey. This is particularly relevant for a trophic specialist such as the Andean cat, an efficient hunter of rock-dwelling viscachas and chinchillas (Walker et al., Reference Walker, Novaro, Perovic, Palacios, Donadio and Lucherini2007; Napolitano et al., Reference Napolitano, Bennett, Johnson, O'Brien, Marquet and Barria2008; Marino et al., Reference Marino, Lucherini, Villalba, Bennett, Cossíos, Iriarte, Macdonald and Loveridge2010), an adaptation that seemingly confers a competitive advantage over the sympatric Pampas cat and culpeo fox. Our first attempt to model the distribution of the mountain viscacha indicated that, as expected, its climatic niche overlapped considerably with that of the Andean cat, with some variations: viscachas appear to tolerate better the more humid conditions of the Southern Andes, and to be less tolerant of the warming of the Northern Andes than the Andean cat. Our habitat modelling supported the overall co-occurrence of the Andean cat and the mountain viscacha in the future, indicating that prey availability should not prevent the Andean cat from adapting to climate change, with the possible exception of the Peruvian Andes, where the habitat of the mountain viscacha is predicted to undergo the largest contraction. Lack of data on the Peruvian viscacha Lagidium peruanum, a species endemic to the Peruvian Andes, prevented its inclusion in this study, thus limiting our conclusions.
As it becomes evident that climate change necessitates adaptive conservation planning, species distribution models are providing opportunities to predict the future distribution of species and communities, and to analyse their future representativeness within existing protected areas (Zimbres et al., Reference Zimbres, de Aquino, Machado, Silveira, Jácomo and Sollmann2012). In the case of the Andean cat, its habitat is bound to decrease, yet 85% of the areas currently protected will remain so under future climate projections. However, < 10% of the predicted range is currently protected, within 47 protected areas, and the species’ presence has been confirmed in only 14 of these. In Peru and Bolivia the impacts of climate change will render many protected areas ineffective, and there is a need for continued and improved protection of areas that are expected to remain suitable for the Andean cat in the future. The opposite is the case in Argentina and Chile, where the polewards shift in distribution opens new opportunities for conservation in the southern range, in areas previously considered to be marginal but with potential to preserve important genetic variability (Cossíos et al., Reference Cossíos, Walker, Lucherini, Ruiz-García and Angers2012). Options to enhance the protection of the Andean cat in the face of climate change include focusing on existing protected areas that are predicted to remain suitable in the future, and reinforcing habitat protection and connectivity between protected areas, including across international boundaries at the triple frontier of Argentina, Bolivia and Chile, and the frontier between Bolivia and Peru. Arguably such adaptive planning will also have positive effects on other Andean species, such as vicuñas, viscachas, chinchillas and queñoa trees, indicating that the Andean cat has potential to be an effective umbrella species in the High Andes.
As we lacked direct information to evaluate the effects of biotic interactions on the present or future distributions of the Andean cat, we cannot ascertain that habitat transitions will happen quickly enough to ensure that the species does not become more threatened, or whether the cat's prey will migrate and adapt to new habitats quickly. Addressing these issues should be a priority for the next generation of species distribution models (see below). Nevertheless, we can speculate on how some biotic interactions or dispersal limitations may affect the ability of the Andean cat to move in response to climate change. For example, our predictions indicated that climate change would reinforce the effects of major climatic barriers already imposing biogeographical boundaries on the species, evident in the structuring of their genetic diversity (Marino et al., Reference Marino, Bennett, Cossios, Iriarte, Lucherini and Pliscoff2011; Cossíos et al., Reference Cossíos, Walker, Lucherini, Ruiz-García and Angers2012) (Fig. 4) and in the distribution of other species of plants and animals (e.g. Chesser, Reference Chesser2000; Marin et al., Reference Marin, Casey, Kadwell, Yaya, Hoces and Olazabal2007). The strengthening of the climatic transition known as the Andean knee in Bolivia could almost subdivide the core range of the Andean cat (Fig. 4), and the intensification of the South American Arid Diagonal will contribute to diminishing connectivity between the core and southern ranges in Chile and Argentina, a process that started with post-glacial warming (Cossíos et al., Reference Cossíos, Walker, Lucherini, Ruiz-García and Angers2012) and that could limit the future polewards dispersal of the Andean cat. The areas occupied by the Andean cat are largely unsuitable for human activities, but if mining operations and persecution of carnivores continue to increase (Lucherini & Merino, Reference Lucherini and Merino2008) they will pose additional limitations on the species’ dispersal and survival in the future.

Fig. 4 Protected areas and the predicted distribution of suitable habitat for Andean cats under the climatic scenario 2080 A2, using the restricted habitat suitability threshold.
Improving predictions for future conservation planning
Species distribution models provide a way to incorporate future conditions into conservation planning and management decisions, provided that the uncertainties of the projected distributions are understood and well balanced with the risks of taking the wrong actions or the costs of inaction (Wiens et al., Reference Wiens, Stralberg, Jongsomjit, Howell and Snyder2009). Within the data limitations inherent in the study of rare carnivores we attempted to document the sources and magnitude of uncertainty and to consider the ability of the Andean cat to track and adapt to climate change on the basis of the existing knowledge. We count among the strengths of our study the use of a robust dataset of records, locally improved global climate surfaces, and thoughtful methodological decisions to control for biases, to avoid overfitting, and to account for uncertainties associated with alternative climatic scenarios and suitability thresholds. On the other hand, there is much room for improvement and more robust models could be designed in the future, and we recommend (1) extending the presence dataset, and including absences; (2) validating models with an independent set of records; (3) accessing higher resolution climate data, and including topography and land uses as predictors; (4) addressing the incompleteness of the IUCN protected area database; (5) improving prey models and extending them to other important prey species; (6) including other biotic interactions, such as the distribution of potential competitors, particularly mesocarnivores; (7) incorporating knowledge on dispersal behaviour as it becomes available; and (8) intensifying survey effort in the southern extreme of the distribution, and modelling core and southern ranges separately, which could produce alternative and better projections, and an understanding of how intraspecific variation could buffer against adverse effects of climate change (Pearman et al., Reference Pearman, D'Amen, Graham, Thuiller and Zimmermann2010; Valladares et al., Reference Valladares, Matesanz, Guilhaumon, Araújo, Balaguer and Benito-Garzón2014; Swab et al., Reference Swab, Regan, Matthies, Becker and Bruun2015).
Acknowledgements
We are grateful to the Andean Cat Alliance (www.gatoandino.org) and all contributors to the Andean Cat Database for the use of these data, and thank The Darwin Initiative, Wildlife Conservation Network and Born Free Foundation for financial support. Magdalena Bennett thanks Comisión Nacional de Investigación Científica y Tecnológica (CONICYT) Becas Chile, Santander Academic Travel Awards, Wolfson College at Oxford for financial support. Pablo Marquet acknowledges support from the projects ICM- P05-002 and CONICYT PFB-023.
Author contributions
MB designed the study, collected and analysed data, and wrote the article. JM, PAM and CSZ assisted in developing the study design, supervised the data analysis, and edited the article.
Biographical sketches
Magdalena Bennett's research focuses on spatial ecology, biogeography and climate change, with application to practical conservation efforts in South America. Pablo Marquet works in macroecology and theoretical ecology and is interested in understanding the impacts of global change on ecological systems. Claudio Sillero-Zubiri is a conservation biologist with a special interest in carnivores and human–wildlife conflict, and is Chair of the IUCN Canid Specialist Group. Jorgelina Marino is an ecologist, and her research applies to the conservation of threatened carnivores in Africa and South America.