Anxiety disorders are the most common psychiatric disorders, with a lifetime prevalence of ~30%. Reference Kessler, Berglund, Demler, Jin, Merikangas and Walters1 They are a major cause of global disability, and impose considerable economic burdens on society. Reference Fineberg, Haddad, Carpenter, Gannon, Sharpe and Young2,Reference Baxter, Vos, Scott, Ferrari and Whiteford3 They commonly have their onset in childhood or adolescence and have been linked to the occurrence of later disorders, including depression and conduct disorder. Reference Kessler, Berglund, Demler, Jin, Merikangas and Walters1,Reference Bittner, Egger, Erkanli, Costello, Foley and Angold4 Adults with anxiety disorders show rates of childhood anxiety diagnoses significantly above baseline. Reference Gregory, Caspi, Moffitt, Koenen, Eley and Poulton5 Given this potential gateway effect, and the distress caused by these disorders, there is a need to optimise and understand treatment effectiveness in childhood.
Cognitive–behavioural therapy (CBT) is a first-line treatment for anxiety disorders in the UK, with 59% remission reported immediately post-treatment. 6,Reference James, James, Cowdrey, Soler and Choke7 Despite this high reported efficacy, variability exists in patient response that may be influenced in part by genetic variants. Multiple studies examining the genetics of differential response to psychological therapies (therapygenetics Reference Eley, Hudson, Creswell, Tropeano, Lester and Cooper8 ) have been undertaken, and variants in seven genes (5HTT/SLC6A4, TPH2, MAOA, COMT, NGF, BDNF and GRIK4) have been implicated at least once in studies of CBT for anxiety disorders. Reference Lester and Eley9 However, findings have proven difficult to replicate, Reference Lester, Roberts, Keers, Coleman, Breen and Wong10 and the direction of effects found inconsistent. These problems may result from the low power of small cohort sizes, resulting in a high rate of false positives, and a narrow focus on a few genes that may have limited relevance to the phenotype.
Genome-wide association studies (GWAS) provide a hypothesis-neutral alternative, agnostic to prior assumptions of relevance and with the potential to discover novel findings at a single variant level. By analysing thousands of variants across the genome, GWAS yield more information than the candidate gene approach, allowing for the acknowledgement and control of confounds such as ancestry and the quality of genotyping. Genome-wide information can also be used to investigate associations between phenotypic change and different levels of the genetic architecture, including the effect of all variants in a given gene, and the effect of all genotyped variants across the genome. However, the explicit requirement for multiple testing correction in GWAS imposes a need for large sample sizes.
Although GWAS have not been used to study response to CBT, they have shown early promise in studying anxiety disorders. Genetic influences on the development of anxiety disorders may indicate processes underlying treatment response, and provide interesting genetic candidates. Reference Uher11 A detailed review of the genetics of anxiety disorders is available elsewhere. Reference Shimada-Sugimoto, Otowa and Hettema12 In brief, one variant, rs7309727 (TMEM132D), was associated with panic disorder in a cohort of European ancestry (P = 1.1 × 10−8 , odds ratio (OR) = 1.45 (95% CI 1.20–1.72). Reference Erhardt, Akula, Schumacher, Czamara, Karbalai and Muller-Myhsok13 A variant in the TMEM16B gene was reported at genome-wide significance in a Japanese cohort with panic disorder, but was not significant in replication analyses. Reference Otowa, Kawamura, Nishida, Sugaya, Koike and Yoshida14 Two GWAS of post-traumatic stress disorder (PTSD) have identified variants at genome-wide significance in the TLL1 gene (rs6812849, P = 3.13 × 10−9, OR not reported) Reference Xie, Kranzler, Yang, Zhao, Farrer and Gelernter15 and PRTFDC1 (rs6482463, P = 2.04 × 10−9, OR = 1.47 (95% CI 1.35–1.59)). Reference Nievergelt, Maihofer, Mustapic, Yurgil, Schork and Miller16 However, these results require replication in larger studies; for example, variants in the RORA gene previously implicated in a GWAS of PTSD failed to attain significance in a larger replication effort. Reference Guffanti, Ashley-Koch, Roberts, Garrett, Solovieff and Ratanatharathorn17 No significant findings from the anxiety literature to date had previously been considered in candidate gene studies. Reference Shimada-Sugimoto, Otowa and Hettema12
To our knowledge, this is the first GWAS to examine response to psychological therapy in any disorder, and the first to examine treatment response of any kind in anxiety disorders. Participants were drawn from the Genes for Treatment (GxT) study, an international, multisite investigation of clinical, demographic and genetic predictors of response to CBT for anxiety in childhood and adolescence. Reference Lester, Roberts, Keers, Coleman, Breen and Wong10,Reference Hudson, Keers, Roberts, Coleman, Breen and Arendt18 Two analyses of association between single nucleotide polymorphisms (SNPs) and response to CBT were conducted, investigating change in symptom severity between baseline and immediately post-treatment (post-treatment), and between baseline and 6 months after treatment cessation (follow-up).
Method
Study design and sample
A detailed description of the participants and the treatment programmes from which they were drawn is provided elsewhere (online supplemental material). Reference Hudson, Keers, Roberts, Coleman, Breen and Arendt18 In brief, participants provided DNA for the GxT study between 2005 and 2013, at 11 sites across the USA, Australia and Western Europe. Children and adolescents (5–17 years old, 94% aged 5–13) were included if they met DSM-IV criteria 19 for a primary anxiety disorder diagnosis, with further psychiatric diagnoses made as appropriate. Exclusion criteria were significant physical or intellectual impairment, and the presence of psychotic symptoms. All participants completed a full course of individual-based CBT (with or without parental involvement), group-based CBT or guided self-help either as part of a trial or as treatment as usual within a clinical research department. All treatments were manualised and treatment protocols across all sites were comparable for core elements of CBT including teaching of coping skills, cognitive restructuring, and exposure.
Assessments were made using the Anxiety Disorders Interview Schedule for DSM-IV, Parent and Child Versions (ADIS-IV-C/P), Reference Silverman and Albano20 except at Bochum (Germany) and Basel (Switzerland) where the German equivalent, Kinder-DIPS, Reference Schneider, Unnewehr and Margraf21 was used. All participants were assessed prior to and immediately after treatment, with further assessments made at 3-, 6- or 12-month follow-up where possible. Output from the ADIS (or equivalent) was converted into Clinical Severity Ratings (CSR) on a scale of 0–8. A diagnosis was made when the child met the diagnostic criteria and received a CSR of 4 or more, usually based on a composite of parent and child report. Diagnoses were made from the ADIS for multiple anxiety disorders, and primary status allocated to the most severe, defined as the highest CSR, with ties resolved by clinical judgement (online Table DS1(b) and (c)).
To minimise differential assessment across sites, raters at Reading (UK), Oxford (UK) and Aarhus (Denmark) all received training in evaluation from the Sydney (Australia) site, and clinicians at Aarhus received additional training in the ADIS from W.K.S., principal investigator of the Florida (USA) site. As such, standardised assessments were made for at least 85% of the analysed sample (for further details see the online supplement).
Definition of the treatment response phenotype
As in previous analyses of the GxT sample, outcome was assessed across two periods: baseline to post-treatment and baseline to follow-up. Although dichotomised treatment outcomes are often used in clinical decision making in treatment response, a continuous measure of change in severity provides substantially more power for analyses. Reference Streiner22
Response post-treatment was therefore defined as percentage change in CSR score between baseline and immediately following treatment. Percentage change, rather than absolute change, was used as it has been shown to better reflect clinical ratings of improvement by its successful use in pharmacogenetics GWAS. Reference Uher, Perroud, Ng, Hauser, Henigsberg and Maier23 For follow-up analyses, a range of time points were available; assessments taken at the 6-month time point were used, as these were the most complete (n = 483). Missing data at this time point was imputed using the best linear unbiased estimates from linear mixture models fitted to the GxT data as part of analyses predicting response from clinical variables alone. Reference Hudson, Keers, Roberts, Coleman, Breen and Arendt18 The mixture models included the linear and quadratic effects of time as well as gender, age, primary diagnosis, treatment type and the random effects of individual and trial (for a full explanation, see Hudson et al Reference Hudson, Keers, Roberts, Coleman, Breen and Arendt18 ). This allowed us to compute response at follow-up as the percentage improvement in CSR score from baseline to 6 months after the end of treatment. Analyses were performed on residual scores generated from a linear regression of the percentage change measure adjusted for baseline severity, age, gender, treatment type, diagnosis and trial.
Both sets of residual scores were created as output variables from our previous study, which found a number of significant non-genetic influences on treatment outcome (online supplement). Reference Hudson, Keers, Roberts, Coleman, Breen and Arendt18
DNA extraction and genotyping
DNA was collected and extracted using standard protocols, from buccal swabs Reference Freeman, Smith, Curtis, Huckett, Mill and Craig24 and saliva kits (OG-500 / PrepitL2P, DNAgenotek, Kanata, Canada). Sample preparation (including concentration and quantification) prior to genotyping is described in the online supplement. Genotyping was performed on Illumina HumanCoreExome-12v1.0 microarrays (Illumina, San Diego, California, USA), using a standard protocol. Reference Gunderson, Steemers, Ren, Ng, Zhou, Tsan, Alan and Brian25 Samples were genotyped in two batches, and randomized by site on each microarray.
Quality control
SNPs were mapped to build version 37/hg19 of the human genome. Initial genotype calls were made with GenCall software (GenomeStudio, Illumina, San Diego, California, USA), reprocessed to remove poorly performing samples, re-clustered, and manually recalled where appropriate. Further recalling, targeted at improving the identification of rare variants (such as the exonic content of the microarray) was performed using ZCall. Reference Goldstein, Crenshaw, Carey, Grant, Maguire and Fromer26 Following recalling, the data were transferred to a multinode computing cluster, and quality control was performed following previously published protocols (online supplement).
Quality controlled data were imputed to the December 2013 release of the 1000 Genomes Project reference (for autosomes; March 2012 release for the X chromosome 27 ), using the posterior-sampling method in IMPUTE2 with concurrent phasing. Reference Howie, Donnelly and Marchini28 SNPs imputed with an info metric >0.8 and a minor allele frequency (MAF) >1% were considered best-guess genotypes, and converted back to PLINK binary format using GTOOL (Freeman and Marchini, available at www.well.ox.ac.uk/~cfreeman/software/gwas/gtool.html). SNPs with a genotype probability of <0.9 were set as missing, and those present in <98% of the sample were excluded from the analysis.
Statistical analysis
Two analyses were performed, examining adjusted percentage change in CSR score from baseline to post-treatment, and from baseline to 6-month follow-up, as described above. Principal component analysis (PCA) of the genotype data was performed to attempt to control for population stratification. However, this yielded components that were not sensitive to differences in outcome. This was likely due to the quantitative nature of the phenotype, the fact that multiple covariates were controlled for in constructing the phenotype, and because participants were drawn from a variety of sites across the globe (online supplement). Accordingly, PCA was deemed unsuitable for controlling for population stratification, prompting the adoption of mixed linear modelling for the association analyses (MLMA). MLMA uses genome-wide genotype data to derive a genomic relationship matrix (GRM), which is used to control for genetic similarity between participants as a random effect. Reference Yang, Zaitlen, Goddard, Visscher and Price29
MLMA association analysis was performed in GCTA, using the mlma-loco option for autosomes and the mlma option for the X chromosome (online supplement). Reference Yang, Lee, Goddard and Visscher30 For each SNP in the study, percentage change in CSR was regressed on the number of copies of the reference allele of the SNP (0, 1 or 2), weighted by its additive effect. A random effect of genetic similarity (from the GRM) was included as a covariate, as were fixed effects of sample concentration at genotyping, sample type (buccal swab or saliva), and ultrafiltration status (whether the sample was filtered in preparation for genotyping; online supplement). Using the assumptions of this approach, power for the GWAS was estimated using the Genetic Power Calculator. Reference Purcell, Cherny and Sham31 The sample of 980 participants has 80% power to detect a variant explaining ~4% of variance and 1% power to detect variants explaining 1%.
Results from the association analysis were clumped according to P-value using PLINK. Reference Purcell, Neale, Todd-Brown, Thomas, Ferreira and Bender32,Reference Chang, Chow, Tellier, Vattikuti, Purcell and Lee33 Each clump is represented by a sentinel SNP (that with the lowest P-value in the clump), and contains all SNPs in linkage disequilibrium with the sentinel (R 2>0.25, within 250kb of the sentinel). One imputed sentinel SNP in the 6-month follow-up analysis was on the borderline of genome-wide significance (rs72850669, P = 7.54 × 10−8), and was re-genotyped post hoc (LGC Genomics, Teddington, UK). This showed the genotype calling of rs72850669 was unreliable (data not shown), and it was removed from the analyses.
To assess the ability of the GWAS to replicate previous findings, the association of SNPs implicated in CBT response in previous candidate gene studies was examined. Reference Lester and Eley9 Exploratory secondary analyses were performed to assess the combined effects of SNPs on response (details can be found in the online supplement). The proportion of variance in CSR change across time accounted for by all the SNPs in the study was assessed with univariate genomic-relatedness-matrix restricted maximum likelihood (GREML), performed in GCTA using the GRM derived for the GWAS. Polygenic risk score profiling was used to investigate the ability of external data-sets to predict CBT response, using risk profiles from publicly available GWAS of major depressive disorder Reference Ripke, Lewis, Lin and Wray34 and schizophrenia, 35 as well as from a meta-analysis of response to antidepressants. Reference Tansey, Guipponi, Perroud, Bondolfi, Domenici and Evans36 To test the ability of the GxT data to predict response to CBT, five analyses were performed. Participants with generalised anxiety disorder, separation anxiety, social phobia and specific phobia, and those from the Reading (UK) site, were separately removed from the dataset and risk profiles derived from the remaining participants. Each profile was then used to predict outcome in the relevant set of removed participants.
Ethics
All trials and collection of samples were approved by site-specific human ethics and biosafety committees. Parents provided informed consent, children provided assent. The storage and analysis of DNA was approved by the King's College London Psychiatry, Nursing and Midwifery Research Ethics Sub-Committee.
Results
Sample and SNP exclusions are shown in online Fig. DS1. Phenotype and high-quality genotype data were available for 939 participants in the analysis post-treatment, with an additional 41 participants available for analysis at 6-month follow-up (n = 980). Baseline demographic information for these 980 participants is described in online Table DS1(a). The position of the samples on principal component axes derived from the HapMap reference populations suggests 92% of the sample are of White Western European ancestry. Reference Consortium37 A total of 260824 common SNPs passed quality control, which rose to 3017604 SNPs when imputed genotypes were added.
No SNPs were found at formal genome-wide significance for either analysis (all P>5 × 10−8). In the post-treatment analysis, four independent clumps passed threshold for suggestive significance (P<5 × 10−6; Table 1 and Fig. 1). Quantile–quantile plots show no departure from the chi-squared distribution of P-values expected under the null hypothesis, suggesting there is no underlying inflation of association statistics by uncontrolled confounds (lambda median = 0.972, Fig. 2). Three independent clumps were suggestive of significance in the 6-month follow-up analysis (Table 2 and Fig. 3), with no evidence of inflation (lambda = 1.02, Fig. 4). All clumps with P<1 × 10−4 are displayed in online Table DS2.
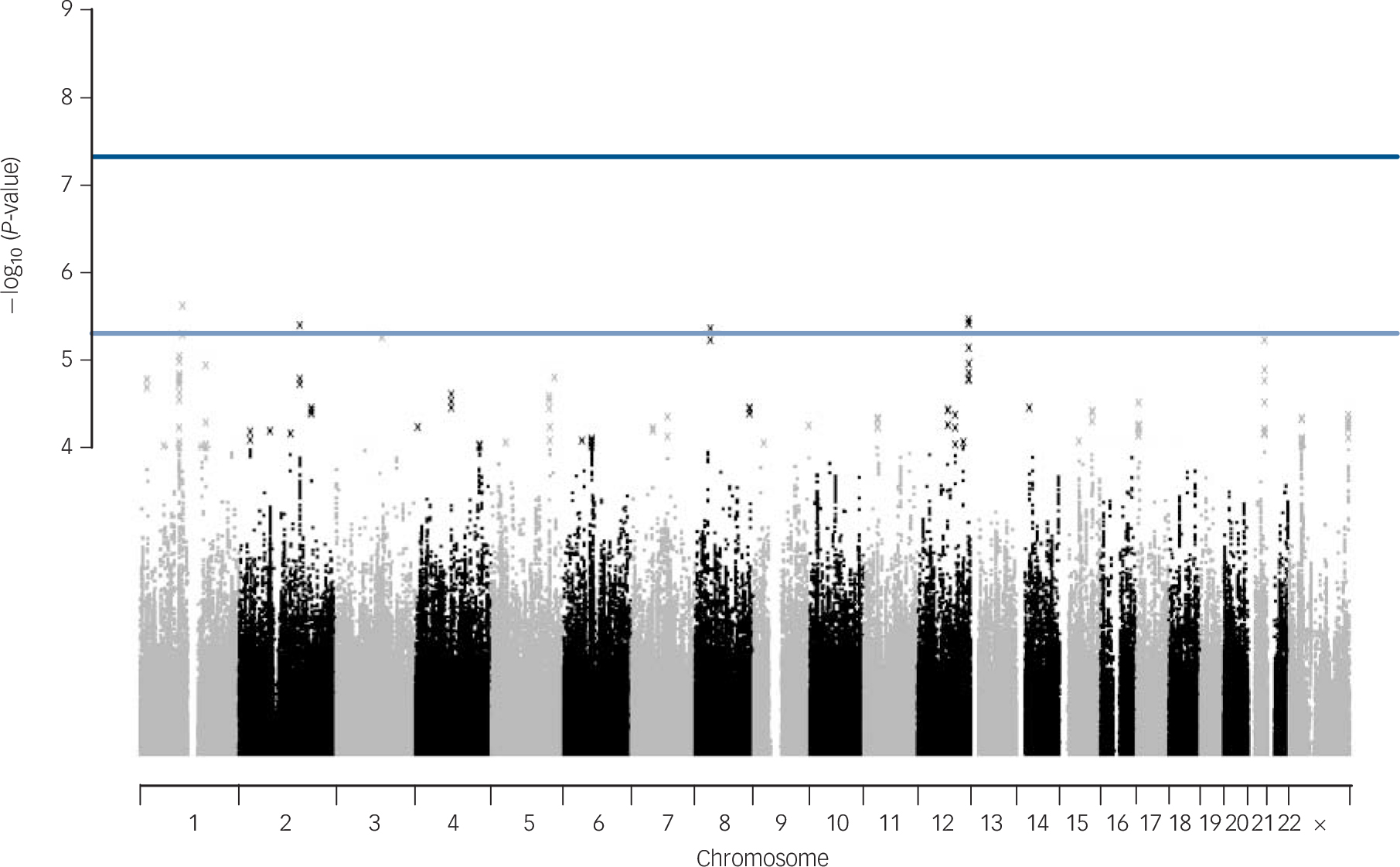
Fig. 1 Manhattan plot of genetic associations with cognitive–behavioural therapy response baseline to post-treatment.
X-axis shows the top million most associated single nucleotide polymorphisms, arranged by position on the chromosome. Lines show conventional thresholds for genome-wide significance (P = 5 × 10−8) and suggestive significance (P = 5 × 10−6).

Fig. 2 Quantile–quantile plot of P-values (pruned for linkage disequilibrium) from genetic associations with cognitive–behavioural therapy response post-treatment.
X-axis shows spread of P-values expected under the null chi-squared distribution. Y-axis shows observed data. Grey region shows rough 95% confidence intervals around each point on the line x = y. Lambda median is a measure of inflation of the observed distribution of associations compared with expected null distribution. Lambda ⩽1 implies no inflation.

Fig. 3 Manhattan plot of genetic associations with cognitive–behavioural therapy response baseline to 6 months after treatment.

Fig. 4 Quantile–quantile plot of P-values from genetic associations with cognitive–behavioural therapy response baseline to 6-month follow-up, including lambda median.
Table 1 Independent clumps associated with cognitive–behavioural therapy response at post-treatment with P<5 × 10−6

Sentinel SNP | CHR | Clump BP | Sentinel SNP P |
Sentinel SNP MAF |
Sentinel SNP information |
Genes +/−100kb |
---|---|---|---|---|---|---|
rs10881475 | 1 | 108113663–108203647 | 2.45 × 10−6 | 0.187 | 0.993 | NTNG1, VAV3 |
rs11834041 | 12 | 128232721–128239057 | 3.50 × 10−6 | 0.135 | Genotyped | – |
rs12464559 | 2 | 152498699–152679462 | 4.09 × 10−6 | 0.0410 | 0.941 | NEB, ARL5A, CACNB4 |
rs881301 | 8 | 38322346–38332318 | 4.46 × 10−6 | 0.403 | Genotyped | WHSC1L1, LETM2, FGFR1, C8orf86 |
SNP, single nucleotide polymorphism; CHR, chromosome; BP, base pair; MAF, minor allele frequency.
Table 2 Independent clumps associated with cognitive–behavioural therapy response at 6-month follow-up with P<5 × 10−6

Sentinel SNP | CHR | Clump BP | Sentinel SNP P |
Sentinel SNP MAF |
Sentinel SNP information |
Genes +/−100kb |
---|---|---|---|---|---|---|
rs72711240 | 4 | 135657189–135695807 | 4.49 × 10−7 | 0.0269 | 0.903 | – |
rs9875578 | 3 | 13707416–13810670 | 1.43 × 10−6 | 0.424 | 0.994 | FBLN2, WNT7A |
rs6813264 | 4 | 146509970–146631854 | 4.68 × 10−6 | 0.410 | Genotyped | SMAD1, MMAA, C4orf51, ZNF827 |
SNP, single nucleotide polymorphism; CHR, chromosome; BP, base pair; MAF, minor allele frequency.
A secondary analysis with increased power was performed restricted to nine SNPs previously associated with response to CBT in candidate gene studies (five other SNPs have been previously implicated in CBT response, but did not pass quality control). Assuming a significance threshold of 0.005455 (0.05/9), none of the nine previously associated SNPs was significant (Table 3 and online supplement). The sample had 80% power to detect an SNP accounting for 1.4% of variance at this significance threshold, suggesting any effect of these SNPs in this data-set is smaller than this.
Table 3 Genome-wide association study P-values of single nucleotide polymorphisms (SNPs) previously associated with cognitive–behavioural therapy response. Reference Shimada-Sugimoto, Otowa and Hettema12,a

Gene | SNP | P (post-treatment) | P (follow-up) |
---|---|---|---|
SLC6A4 | rs25531 | Imputed with info <0.8 | Imputed with info <0.8 |
HTR2A | rs6311 | 0.4717 | 0.9692 |
rs6313 | 0.5451 | 0.8109 | |
rs6314 | Imputed with info < 0.8 | Imputed with info <0.8 | |
rs7997012 | Completeness after imputation <0.98 | Completeness after imputation <0.98 | |
TPH2 | rs4570625 | Completeness after imputation <0.98 | Completeness after imputation <0.98 |
COMT | rs4680 | 0.7699 | 0.5956 |
NGF | rs6330 | 0.5093 | 0.4559 |
BDNF | rs6265 (val158met) | 0.3408 | 0.9078 |
rs7934165 | 0.5231 | 0.9880 | |
rs1519480 | 0.8211 | 0.5013 | |
rs11030104 | 0.3158 | 0.9675 | |
GRIN2B | rs1019385 | Imputed with info <0.8 | Imputed with info <0.8 |
GRIK4 | rs1954787 | 0.1315 | 0.1914 |
a. No P-value is significant after multiple testing correction.
Exploratory secondary analyses (GREML, gene-wide analyses and polygenic risk score profiling) were performed. No significant estimate of SNP heritability could be obtained from GREML, and the effect of adding principal components was minimal. In the post-treatment analysis, all estimates were non-significant. In the 6-month follow-up data the highest estimate was 0.0797 (95% CI −0.194 to 0.35) without principal components. The power of univariate GREML in the sample was estimated for a range of true heritabilities. Reference Visscher, Hemani, Vinkhuyzen, Chen, Lee and Wray38 Power ranged from 9 to 46% assuming true heritability between 0.2 and 0.6. To achieve 80% power within this range of heritabilities will require 1450–4450 samples (for heritabilities between 0.6 and 0.2).
Polygenic risk score profiling failed to generate predictions that were consistently significant, either for external GWAS or in the internal predictions of response.
Discussion
Main findings
We report the first genome-wide association study of psychological therapy. Although no region reached genome-wide significance, the single SNP and polygenic results are consistent with the wider literature of treatment genetics in psychiatry, given the sample size studied. Genome-wide significant variants detected in GWAS of psychiatric phenotypes have shown small effect sizes (with the exception of late-onset dementia), requiring thousands of participants to discover. The pattern of results in psychiatric genomics to date suggests that a critical number of participants (varying by disorder) are required before robust findings begin to be made. In studies of schizophrenia, this critical number was ~9000 cases. Reference Hyman39 Our results, although preliminary, suggest response to CBT could be a complex phenotype at the early point of this trajectory, although the critical sample size is not yet clear.
The purpose of this study was to identify genetic variants capable of predicting change in symptom severity during treatment. No common, high-effect SNPs were identified, suggesting that it is very unlikely a single variant could be used as a predictor. This also places an upper bound on expected effect sizes in studies of CBT response. This is relevant considering that neither GWAS replicated previous findings from the literature. This does not appear to be due to insufficient statistical power. For example, the COMT val158met polymorphism (rs6265) was reported to account for 8% of variance in CBT response in adults with panic disorder, well above the 4% of variance explained for which this GWAS was powered. Reference Lonsdorf, Ruck, Bergstrom, Andersson, Ohman and Lindefors40 Failure to replicate previous findings from the candidate gene literature has proved common in psychiatric genetics, whereas GWAS is proving more reliable. 35,Reference Bosker, Hartman, Nolte, Prins, Terpstra and Posthuma41 The failure to replicate any published variants suggests previous assumptions of gene relevance may be erroneous, resulting from underpowered candidate gene studies that overestimated the likely effect sizes of studied variants, and that reported variants are likely to be false positives, or to have effect sizes inflated due to winner's curse. Reference Ioannidis42 Proximity to a gene does not imply an effect on gene expression, so the failure to replicate the effects of candidate SNPs does not exclude a role for candidate genes, as the SNPs assessed may not capture true functional variation.
Not all candidate variants are SNPs, and one limitation of GWAS is the difficulty of assessing structural variants not captured by the probes on microarrays. For example, we cannot comment on the previously reported role of the MAOA-u variable number tandem repeat in CBT response. Reference Reif, Richter, Straube, Höfler, Lueken and Gloster43 Nor could we assess the effect of the 5HTTLPR variant of SLC6A4, previously associated with remission from anxiety disorders at follow-up; however, we directly genotyped this variant in this cohort, and were unable to replicate our earlier finding. Reference Eley, Hudson, Creswell, Tropeano, Lester and Cooper8,Reference Lester, Roberts, Keers, Coleman, Breen and Wong10
Although small when compared with high-profile studies such as the PGC studies in schizophrenia and depression, Reference Ripke, Lewis, Lin and Wray34,35 our sample is similar in size to studies in the depression pharmacogenetic literature. Reference Uher, Perroud, Ng, Hauser, Henigsberg and Maier23,Reference Ising, Lucae, Binder, Bettecken, Uhr and Ripke44 The first of these used a multistage design (n = 1532) and identified several associations at nominal significance, but none remained significant after correction for multiple testing. Reference Ising, Lucae, Binder, Bettecken, Uhr and Ripke44 The second (n = 706) found one genome-wide significant locus (for response to nortryptiline treatment) and six loci at suggestive significance across four subanalyses. Reference Uher, Perroud, Ng, Hauser, Henigsberg and Maier23 More recent meta-analyses were unable to find genome-wide significant variants. Reference Tansey, Guipponi, Perroud, Bondolfi, Domenici and Evans36 However, a significant GREML estimate of SNP-chip heritability of 42% (95% CI 6%–78%) was identified, suggesting useful information about treatment response can be obtained at the whole-genome level. Reference Tansey, Guipponi, Hu, Domenici, Lewis and Malafosse45 Future studies in psychological therapy-genetics should aim to build a cohort of sufficient size to estimate SNP-chip heritability and bivariate genetic correlations, enabling further comparison with pharmacogenetic studies. Such a cohort could act as a target data-set for polygenic risk scoring, exploring the predictive value of variants associated with potentially relevant phenotypes assessed in other GWAS.
Limitations
There are parallels between the antidepressant GWAS literature and this study, including the necessity of combining many studies to obtain sufficient participants for analysis. Herein, we examined a naturalistic clinical cohort, drawn from CBT trials or from treatment as usual. As all participants received CBT, there was no placebo group for comparison. Therefore, the results may reflect natural regression to the mean, rather than an effect of treatment. Theoretically, a parallel GWAS of change in severity could be performed on wait-list controls to identify associations with regression to the mean. Results from the GWAS of CBT response could be weighted by the likelihood that any given association resulted from regression to the mean. However, this would require deliberate non-treatment of thousands of wait-list controls over a period of at least 7 months for the purpose of comparison only. As CBT is effective in this age group, with significant improvement seen in treated groups relative to wait-list controls, non-treatment would raise serious ethical concerns. Reference James, James, Cowdrey, Soler and Choke7 The aim of therapygenetics is to discover predictors of differential response to treatment. These predictors need not capture a treatment effect per se; they may describe processes separate to treatment that nonetheless lead to better (or worse) response. Nevertheless, in the absence of a control group, this study specifically examines the association between genetic variation and change in CSR across the period of CBT treatment and follow-up, not the biological mechanism of response to CBT.
The naturalistic nature of the cohort creates heterogeneity, including differences in the details of the treatment given, the target disorder of the treatment, and several participant characteristics. The effectiveness of CBT is influenced by a variety of environmental factors. Some of these can be considered within the design, such as treatment type, diagnosis and severity. Others are less easily accounted for, including therapeutic alliance and other social influences, which may only be partly controlled for by the inclusion of trial as a covariate. Reference Hudson, Keers, Roberts, Coleman, Breen and Arendt18,Reference Hudson, Kendall, Chu, Gosch, Martin and Taylor46 This reduces the statistical power of analyses, but should not be viewed as an argument against therapygenetics. The ability to offer personalised advice to patients about treatment could avoid considerable amounts of unnecessary distress and expense. Obtaining a set of genes able to assist in clinical prediction will require a cohort that is powerful enough to detect true variants while remaining clinically representative. Thus, a degree of heterogeneity is unavoidable in studying response to CBT, and similar difficulties in pharmacogenetic GWAS suggest this limitation applies to treatment response genomics more generally.
Combining data from trials at multiple sites necessitated compromises in study design. Participants were included if they completed treatment, but drop-out from treatment is common and likely to be related to poorer response. As such, future studies should aim to include severity data for non-completing participants. This would require appropriate modelling of the treatment period, and the proportion of the treatment process completed, before participation ceased. Similarly, combining measurements from different sites and from participants with varying diagnoses required the use of a general, widely applicable outcome measure. The ADIS fit these requirements well, but relies on clinical judgement derived from parent and child report. It may be less sensitive to the effects of CBT than a self-report measure, and be more vulnerable to site-specific biases. However, a suitable diagnosis-general self-report scale was unavailable, and standardising outcomes to combine multiple diagnosis-specific scales is likely to lead to a generalised and difficult-to-interpret result.
Future directions
This study represents the first GWAS of psychological therapy. Although no genome-wide significant findings emerged, the spread of significance in the associations captured is similar to other early general psychiatric and pharmacogenetic GWAS. The best approach in the immediate future is to increase the sample size available through combining existing cohorts in mega- and meta-analyses. Such a cohort would allow replication of the findings presented in this paper to be attempted, which currently is not possible due to the lack of an independent cohort of suitable size. However, individual variants are likely to have small effect sizes, so future studies should utilise higher-order approaches such as polygenic risk scoring and GREML to leverage the predictive effects of the whole genome. This would also provide an estimate of heritability, which is difficult to obtain through traditional family-based approaches. If the heritability of CBT response were around 30% (similar to that of anxiety disorders), a high-powered polygenic risk score could capture 10–15% of variance, which could be clinically useful when combined with known environmental risk factors. Reference Dudbridge47 However, creating such a score will require a sample size of at least 10 000, which would involve considerable effort to obtain.
Alternative approaches may also yield interesting findings. Response to CBT is a behavioural change following exposure to a positive environment, so epigenetic studies investigating how these exposures influence gene expression via DNA methylation will be informative. Reference Roberts, Lester, Hudson, Rapee, Creswell and Cooper48 Similarly, it will be useful to examine changes in gene transcript expression across treatment and in the longer term. Used in parallel to these approaches, studying specific genetic variants remains a potential method of predicting response to CBT (and understanding its biological basis) and genome-wide investigations represent the most promising avenue in which to focus the gathering momentum of therapygenetics. Reference Eley49
Funding
Combined study supported by UK Medical Research Council grant to T.C.E. Individual trials support by Australian Research Council grant to J.L.H., R.M.R. and T.C.E.; Australian NHMRC grants to R.M.R., J.L.H. and H.J.L. (), H.J.L, J.L.H and R.M.R (), and J.L.H and R.M.R. (); TrygFonden grant () to M.T.; Edith og Godtfred Kirk Christiansens Fond grant () to M.T.; Swiss National Science Foundation grant () to S.S., Western Norway Regional Health Authority grant to E.R.H. (); UK Medical Research Council Clinical Fellowship () to R.M-S.; NIMH R01 () to W.K.S.; UK NIHR grants to C. Creswell and P.C. () and P.C. and C. Creswell (); UK Medical Research Council Grants to P.C. and C. Creswell (), K.T., P.C. and C. Creswell (), P.W., C. Creswell and P.C. (), and C. Creswell (). Grant was managed by National Institute for Health Research (NIHR) on behalf of the MRC-NIHR partnership. This study presents independent research part-funded by the NIHR Biomedical Research Centre at South London and Maudsley NHS Foundation Trust and King's College London. The views expressed are those of the author(s) and not necessarily those of the NHS, the NIHR or the Department of Health.
Acknowledgements
The authors would like to thank: Irma Knuistingh Neven, Marianne Bjerregaard Madsen, Lisbeth Jørgensen, Carmen Adornetto, Kristine Fonnes Griffin, Krister Fjermestad, Elisa Kulewski, Jonas Großekathöfer, Nora Dirks, Evelyn Kmelnitski, Sabrina Heuser, Adrian Boyle, Clare Dixon, Harma Moorlag, Nienke Boersma, Sanne Hogendoorn, Sue Cruddace, Marie Weber, Zoe Hughes, Kiri Clarke, Francoise Hentges, Liz White, Nynke Wagenaar, Luci Motoca, Yesenia Rodriguez, Klaudia Perreira, Erin Hedemann, Cristina Del Busto, Gillian O'Neill, Lucy Lindley, Rachel Banham, Nora Delvendahl, Hjalti Jonsson, Judith Blatter-Meunier, Chantal Herren, Odd E. Havik, Karen Krause, Anna McKinnon, Patrick Smith and Yasmin Rey; and all participants in the study.
eLetters
No eLetters have been published for this article.