Introduction
The United States (U.S.) swine industry grappled with uncertainty on multiple fronts in 2018 and 2019. The U.S. imposed trade actions against China and several other countries in 2018, resulting in retaliatory tariffs of 15 to 25% on 93 agricultural line items from the U.S., and the loss of approximately $10 billion in exports in 2018 compared to 2017 (CRS, 2019). The retaliatory tariffs amplified trade tensions between the U.S., a global exporter of pork, and China, the world’s largest producer and consumer of pork (USDA-FAS, 2020). On April 2, 2018, China’s Ministry of Commerce imposed a 25 percent retaliatory tariff on U.S. pork and pork products (Buckley, Reference Buckley2018). In 2018 and 2019, the actions, collectively referred to as the U.S.–China Trade War, escalated before the Phase One Trade Agreement was signed in January 2020. Although the Phase One Trade Agreement did not explicitly remove retaliatory tariffs, it set the stage for de-escalating the trade war (Muhammad and Jones, Reference Muhammad and Jones2021).
Early in the trade war, on August 3, 2018, China reported its first outbreak of African swine fever (ASF), a disease known to have high mortality rates in adult swine and high abortion rates in breeding herds. The ASF outbreak significantly decreased the Chinese swine inventory (Shao, Zhang, and Hayes, Reference Shao, Zhang, Ji and Hayes2018). Because of China’s relative size in production and trade, the outbreak had sizable consequences on world pork and swine product markets. This outbreak also generated extensive media interest domestically and abroad. The contentious U.S.–China trade war and China’s ASF outbreak resulted in market disruptions globally in the near term. Both events have long-term implications for the U.S. domestic and international pork industry.
Media dissemination of disturbing, disruptive, or controversial events can quickly increase and amplify collective focus during and after crises (Bento et al., Reference Bento, Nguyen, Wing, Lozano-Rojas, Ahn and Simon2020). Throughout 2018 and 2019, newspapers, television, and social media documented the evolution of these events, with some media focusing on the global impacts of ASF and China–U.S. trade disputes (Ivanova and Harris, Reference Ivanova and Haris2018; Darrah, Reference Darrah2019; Lee, Reference Lee2019). When markets are efficient, information is processed quickly and effectively and reflected in price expectations. Futures prices should reflect relationships between the U.S. and international trade partners regarding information received from or reported by media outlets. Futures prices proxy what traders believe the price will be, subject to information disseminated at some point in time in addition to existing information (Leuthold, Reference Leuthold1974).
This study aims to explain the linkage between market expectations on lean hog futures prices and uncertain or unexpected information reported by news media. The effect of anticipated reports on futures prices is well studied. However, less work has focused on unexpected global events like ASF, trade negotiations, or trade wars between countries. No study has been able to analyze the effects of information when two world events, which should move markets in opposite directions, coincide. Because both ASF and the trade war have different implications for the swine industry in the short- and long-term, the study also analyzes the influence of information on traders’ expectations in nearby-, medium-term, and distant horizons.
Similar to Attavanich et al. (Reference Attavanich, McCarl and Bessler2011)’s work, which analyzed the effect of media coverage influences from newspaper headlines after the 2009 H1N1 outbreak on lean hog futures prices, this study complements their study by expanding the type of media data used and applying a state-space modeling procedure to account for changes in price dynamics following unanticipated ASF and China–U.S. trade war disruptions. We also investigate the influences of public sentiments, discussed below, which are attached to information bytes circulated by media coverage.
Findings from this study will add to our understanding of the influences of a “constant-contact” media environment on market dynamics, using the example of lean hog futures prices. The findings will interest traders and policymakers in understanding how media sources shape world events and their impact on futures markets in the short- and long term. When United States Department of Agriculture (USDA) information is unavailable (e.g., government shutdowns preventing releases of USDA reports) and even when it is available, consumers resort to other informational sources, which vary in quality, to acquire news about current events and supply and demand conditions (Huffstutter and Polansek, Reference Huffstutter and Polansek2019). Studies like this contribute toward a more accurate understanding of how media influences market movements with implications for managing market risk.
Scope of the issue
In 2018, the U.S. swine industry was operating at record production levels, with an estimated 72.1 million head on March 1, 2018, and there was no indication of decline (NASS, 2018). The U.S. global share of exports for the pork industry has increased since events in 2018 and 2019. Most U.S.-produced pork is consumed domestically, but expectations of an expanding export market influence the domestic production decisions of swine integrators and domestic hog prices.
Amid record hog and pork production, U.S. and China trade tensions developed up to 2018. Tensions intensified after the Trump administration implemented tariffs and trade barriers on Chinese markets. Before the trade war, China became an importer of growing importance for U.S. pork producers. The trade war caused uncertainty in international markets, which affected demand for a record domestic supply of pork and pork products. Following the implementation of retaliatory tariffs, media outlets began publishing headlines, some of which amplified the gravity of the situation and its impact on the U.S. domestic hog industry. From April to October 2018, the U.S. experienced a $31 million decline in pork and pork product exports to China (GATS, 2020).
As the U.S.–China trade war heated, so did a growing swine health concern globally, African swine fever or ASF. ASF is lethal to domestic and wild swine, with some herd mortality rates as high as 100 percent (Gallardo et al., Reference Gallardo, Reoyo, Fernández-Pinero, Iglesias, Muñoz and Arias2015). Countries in Asia, Europe, and Africa have contended with the highly contagious disease for decades, but cases have increased since 2016.Footnote 1 Currently, neither a cure nor an effective vaccine is available to slow the spread of the virus.
Before ASF, China accounted for approximately half of the global hog herd. From August 2018 through 2019, the ASF outbreak led to a 41 percent reduction in the Chinese sow inventory. Chinese pork production declined by 10 percent (USDA-FAS, 2019). The Chinese breeding sows inventory losses associated with ASF exceeded the total U.S. hog inventory that year (USDA-FAS, 2019). As a result, opportunities arose for the U.S. and other pork-exporting countries to increase shipments to meet China’s shortfall and to generate a domestic increase in hog prices. The retaliatory tariffs on pork limited the extent to which the U.S. could capitalize on those opportunities. However, as more world pork was diverted to China, the U.S. also had an opportunity to form new trade relationships with other partners. Reports indicate exports to China from the U.S. still more than doubled in 2019 year over year (USDA-FAS, 2020).
Volatility in futures prices is caused by uncertainty in supply and demand relationships (Anderson, Reference Anderson1986). Colling and Irwin (Reference Colling and Irwin1990) found that unanticipated changes in the Hogs-and-Pigs reports impact live hog futures prices. Mann and Down (Reference Mann and Down1996) found that live hog and pork belly futures market volatility in trade and trade volume increased following published Hogs-and-Pigs reports. Isengildina-Massa et al. (Reference Isengildina-Massa, Karali, Irwin, Adjemian and Cao2016) found that the impact of USDA inventory reports on futures markets declined substantially following published Hogs-and-Pigs reports, but “surprising” report estimates significantly influenced markets. Anticipation of reports can also affect markets, given the uncertainty around what type of information is released. A commonality between the studies above is that they focused specifically on the influence of USDA reports and expected announcements on futures prices.
Event study methods are widely used to understand the impacts of public information on market trends (Colling and Irwin, Reference Colling and Irwin1990; Mann and Down, Reference Mann and Down1996; Attavanich et al., Reference Attavanich, McCarl and Bessler2011; Isengildina-Massa et al., Reference Isengildina-Massa, Karali, Irwin, Adjemian and Cao2016). The concept of an event study is straightforward, as Campbell et al. (Reference Campbell, Lo and MacKinlay1997) suggested. Suppose prices in efficient markets respond to informational announcements (an “event”). In that case, the information reported has value to market participants. The U.S. hog industry has an opportunity to increase its global market share when adverse events in other countries, such as ASF in China, deplete global supplies. However, the hog industry faces challenges when unanticipated adverse events occur domestically. One such event is the retaliatory tariffs on U.S. pork and pork products destined for China.
The current media environment is large and expansive and contains information from various platforms. “Content information” varies in accuracy and quality. The accuracy of various sources is questionable, and individual reactions to an event may over- or understate the future impact of current events (Liu, Han, and Yin, Reference Liu, Han and Yin2018). Media over-reporting of events may also amplify the volatility of lean hog futures prices. Previous studies examined market movements following media reporting (Pudenz and Schulz, Reference Pudenz and Schulz2020). The current study examines how media reports on China–U.S. trade disputes and the ASF outbreak in China influenced the short- and long-term price expectations of U.S. lean hog futures as the events coincided.
Data
The period utilized begins in 2015 to capture pre-event markets. It ends in 2020 to prevent noise and influence from the COVID-19 pandemic. Three different lean hog daily price series were collected: 1) the nearby futures price, which is rolled over one week before expiration; 2) a mid-horizon futures price; and 3) a distant-horizon futures price. Similar to previous studies (Hudson, Koontz, and Purcell, Reference Hudson, Koontz and Purcell1984; Mann and Down, Reference Mann and Down1996), considering the impacts of media on the nearby time horizon and the distant horizon is required, given the long-term implications of both events on the swine industry.
The price horizon definitions used here are similar to those used by Mann and Down (Reference Mann and Down1996) and are found in Table 1. The nearby futures price is the daily closing price of the next contract expiring. The mid-horizon futures price is the daily closing price of the lean hog contract expiring in 6 months. The distant-horizon futures daily closing price is the contract’s price expiring in the next 12 months. Prices were collected from the Livestock Marketing Information Center (LMIC). Given media influences, this characterization of contract prices allows for including price expectations. If media announcements play a role in influencing futures prices, then the procedure used here can determine if that influence is relatively stronger for nearby contracts or for contracts set to expire at a later date, given expectations arising from event reporting. Examining both near and distant time horizons captures the immediate impacts on the market hog inventory and the long-term repercussions on the breeding hog inventory. Soybean daily closing futures prices, measured in U.S. cents per bushel for 2015 to 2020, were also collected from LMIC.
Table 1. Variable definitions
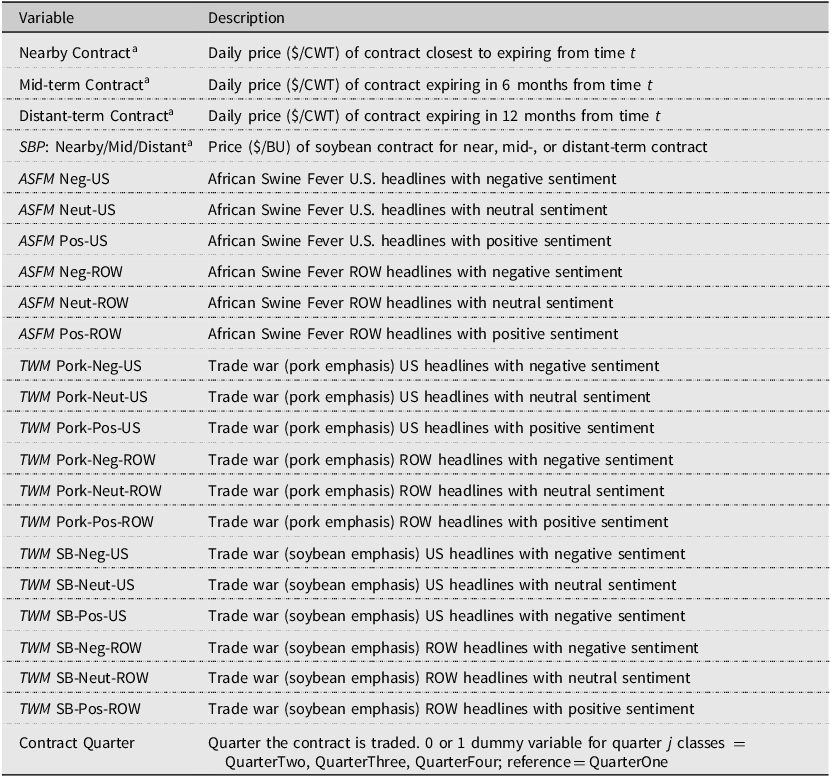
a $/CWT = USD per hundredweight; $/BU = USD per bushel.
The media sentiment data are from Meltwater Software, a global company that provides online media monitoring and conversation capturing.Footnote 2 Using machine learning, Meltwater tracks conversations containing keywords on specific topics monitored across various sources such as online and televised news platforms, social media, print, broadcasts, and podcasts. Meltwater utilizes natural language processing to extract and analyze content from various platforms (Orsi, Reference Orsi2023). For this analysis, keywords used to measure the influences of both world events included “African Swine Fever” and “Trade War and Pork.” One of the above phrases must be present in the dialog for a headline to be included in the data.
Meltwater provided every headline, broadcast, or conversation that included a discussion concerning the above terms during the requested period. Sources ranged from newspaper headlines, television reports, radio broadcasts, and social media conversations on the events. The analysis also contained information on the headline’s location and the information’s sentiment. The location of the headline was further categorized as originating in the U.S. or elsewhere (rest of the world, ROW). Questioning similar impacts of media coverage related to H1N1 (swine flu) on futures prices, Attavanich et al. (Reference Attavanich, McCarl and Bessler2011) concluded that media coverage negatively influenced domestic demand for pork and pork products, which resulted in lower futures prices. Attavanich et al.’s analysis was limited to using only newspaper headlines to account for media coverage. The information conveyed in the headlines was classified as either having a positive (Pos), neutral (Neut), or negative (Neg) tone, similar to the current analysis. Similar to Attavanich et al. (Reference Attavanich, McCarl and Bessler2011), the number of daily headlines about each topic and the number of positive, neutral, or negative headlines were enumerated to control for the influence of media headlines on futures prices. A summary of the data can be found in Table 2. Negative information tends to impact an individual’s mindset more than positive information (Baumeister et al., Reference Baumeister, Bratslavasky, Finkenauer and Vohs2001; Rozin and Royzman, Reference Rozin and Royzman2001). Wang and de Beville (Reference Wang and de Beville2017) also used the connotation of newspaper articles. However, they grouped articles by their assumed positive, neutral, or negative effect on consumer demand.
Methods and procedures
The influence of shocks on futures markets is a common theme in event studies literature, with considerable interest in modeling the impact of market reports on prices (Colling and Irwin, Reference Colling and Irwin1990; Lusk and Schroeder, Reference Lusk and Schroeder2002; Isengildina-Massa et al., Reference Isengildina-Massa, Karali, Irwin, Adjemian and Cao2016). First, we conduct unit-root tests to determine if the variables we use are stationary. The Phillips–Perron test is used to test for unit roots (Phillips and Perron, Reference Phillips and Perron1988). The null hypothesis of this test is that the variable in question has a unit root. A variable with a unit root is non-stationary. Suppose the null hypothesis of the Phillips–Perron test cannot be rejected. In that case, the variable is first-differenced to remove the unit root. The variables tested include the nearby-, mid-, and distant-term lean hog futures prices, and soybean prices.
Second, we use state-space regression procedures to model the effects of ASF outbreaks and trade war tariff hikes on lean hog futures. We hypothesize that these variables are latent, unobserved state variables that affect transitions in the futures series’ evolution. These variables are unobserved because the timing and magnitude of ASF outbreaks or new tariff rate levels are unanticipated surprises not captured by the available data. Most time-series models can be equivalently written as linear state-space models (SSM) (Hamilton, Reference Hamilton1994). A generic SSM is:


where equations 1 and 2 are observation and state equations, respectively. Figure 1 is a visual representation of the SSM procedure used in this analysis.

Figure 1. Trade dispute-ASF state-space model. Notes: ASF occurrences and tariff levels may lead to possible changes in state, also called structural breaks. Two state equations are used to capture the unknown influences of China-U.S. trade negotiations and ASF on lean hog prices futures, given exogenous variables hypothesized to influence the series.
For the observation equation, the variable
${\bf y}_{t}$
is a p × 1 vector of lean hog futures prices observed in period t and p refers to the daily lean hog futures price series. The object
${\rm \bf A}_{t}$
is a p × 1 vector of intercepts for period t. The object
${\rm \bf B\ }$
is a q × 1 vector of time-invariant coefficients, and
${\bf s}_{t}$
is a p × q matrix of latent state variables, corresponding q with the number of ASF outbreaks and implemented tariff hikes (discussed below) in period t. The stochastic terms
${\rm \bf \varepsilon}_{t}$
are observation random disturbances with a covariance matrix
${\rm \bf \Omega}_{(1)}$
(Gu and Yung, Reference Gu and Yung2013).
Variables known to influence futures prices directly but do not necessarily lead to time series breaks are included in the observation equation. One such variable is soybean futures prices. It is important to account for known relationships between the two commodity prices in the observation equation but not the state equation. The matrix
${\bf x}_{t}$
in the observation equation includes vectors of exogenous variables hypothesized to influence prices. The parameters in
${\rm \bf \Gamma\ }$
relate the exogenous variables to lean hog future prices.
State equations control for the influence of unobservable factors on the observation equation. State variables enter the observation equation where the endogenous variable is a price expectation series (i.e., futures prices). The state equation consists of a vector of unobserved state variables,
${\bf s}_{t}$
, where
${\rm \bf \unicode{x03B2}}$
is a q × q transition matrix for the lagged state variables. The
${\bf u}_{t}$
terms are random disturbances with a covariance matrix
${\rm \bf \Omega}_{(s)}$
(Gu and Yung, Reference Gu and Yung2013). State equations determine the likelihood of a price series transitioning from one state to another. For example, tariffs implemented during the US-China trade may cause futures prices to transition to a new state, given an expected reduction in demand, and be driven by a tariff level variable appearing in the state equation. Public expectations of the future may also influence price expectations, but these expectations are unobservable (Hamilton, Reference Hamilton1994). Matrix
${\rm \bf \unicode{x03B2}}$
, therefore, captures the dynamic interactions between tariff levels and ASF outbreaks by permitting the lagged values of unobserved tariff and outbreak shocks to influence the current state associated with tariff levels and confirmed Chinese ASF outbreaks.
The vector
${\bf w}_{t}$
in the state equation includes exogenous variables thought to influence next-period states. Including an observed variable in the state equation increases the precision of media impact estimates on futures prices. The current state is updated as surprising information enters the equations (e.g., new tariff levels or newly confirmed ASF outbreaks).
The SSM is estimated with maximum likelihood using Stata’s sspace procedure (StataCorp, 2021). The state-space portion of the model is utilized to derive the log-likelihood of the observed endogenous variables conditional on their past levels and other exogenous variables. This procedure utilizes two forms of the Kalman filter to obtain conditional means and variances for both the state and observation equations (Kalman, Reference Kalman1960).
The extended SSM for lean hog futures prices for k = the mid- and far-term futures is:



Equation 3, the observation-level equation, regresses daily lean hog futures prices (LHP t k , in U.S. dollars per hundred pounds) for all contract months on the current-period state variables, ASFS t (ASF state) and TARS t (tariff level state), in addition to other exogenous variables that influence lean hog prices. The evolution of the state variables, ASFS t and TARS t , is determined by the previous level of the state variable, the announcement of confirmed an ASF outbreak (ASFO t , Eq. 4) and the announcement of a new tariff level (PTAR t , Eq. 5). ASFS t and TARS t are indicator variables that include actual events at the time they occurred and, as such, are objective measures of when event impacts began. For example, potential tariff increases may be reported in the media for some duration before tariff levels change. The variable PTAR contains real tariff level changes over time and is from the USDA Foreign Agriculture Service (2020). Similarly, ASFO takes a value of “1” starting when China’s first ASF case was reported until the last case of ASF was reported to the World Animal Health Organization. The state equations are identified by normalizing the variance terms to one (Hamilton, Reference Hamilton1994).
Exogenous variables in the observation-level equations include media content on African swine flu (
${\bf ASFM}_{t}$
) and the trade war (
${\bf TWM}_{t}$
), soybean futures contract prices (SBP
t
), and quarterly dummy variables to control for seasonal effects on lean hog futures (
${\bf QTR}_{t}$
). The media content variables in
${\bf ASFM}_{t}$
and
${\bf TWM}_{t}$
count the number of headlines that contained positive, negative, or neutral sentiments on ASF outbreaks and the trade war.
In animal agriculture, lagged production cycles play a role in the expectations of futures prices (Anderson, Reference Anderson1974). This information indicates whether traders’ recovery expectations for Chinese pork supply and global demand from market shocks affect nearby, mid-, and distant-price horizons. The nearby, mid-term and far-term soybean futures contracts, is the first covariate that addresses this source of heterogeneity in each respective price horizon, given the relationship between soybean prices (a feed cost to producers) and lean hog futures prices. The nearby futures series is estimated separately due to the need to account for rollovers in the price series. With rolling over the contract one week before expiration, data points for this time series did not match the mid- and distant-term price series.
Results and discussion
Unit roots were identified in the data series. According to the Phillips–Perron test, all variables failed to reject the null hypothesis of non-stationarity at a 1 and 5% significance level as found in Table 3. First differences of the lean hog futures contracts and soybean futures contracts were taken to correct for non-stationarity (Table 4). Stationary processes were confirmed for the first-differenced variables.
Table 2. Summary statistics
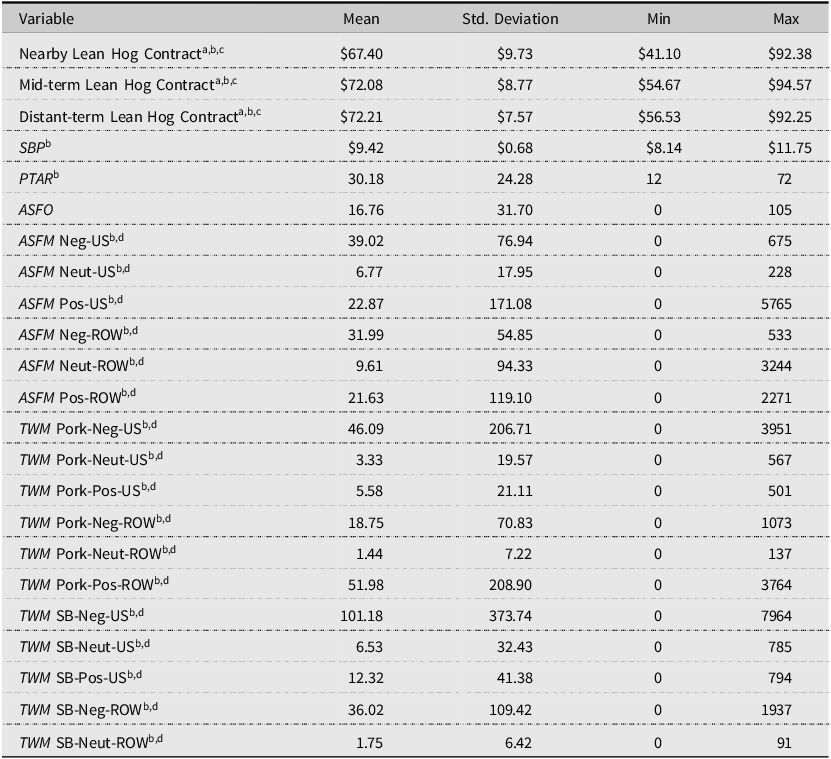
a Nearby = Contract set to expire next; Mid- = Contract expiring in 6 months; Distant- = Contact expiring in 12 months.
b Prices collected from Livestock Marketing Information Center; Sentiment data collected from Meltwater Software; Tariff levels collected U.S. Meat Export Federation; ASF Cases collected from World Organisation for Animal Heatlh.
c Nearby Contract: N = 1,111; Mid-term and Distant-term contacts: N = 1,321; 2015–2020.
d Sentiments of Headlines: Pos = Positive Sentiment, Neut = Neutral Sentiment, Neg = Negative Sentiment; US = United States, ROW = Rest of World.
Table 3. Stationarity test (Phillips–Perron)

Table 4. Lean hog futures price regressions
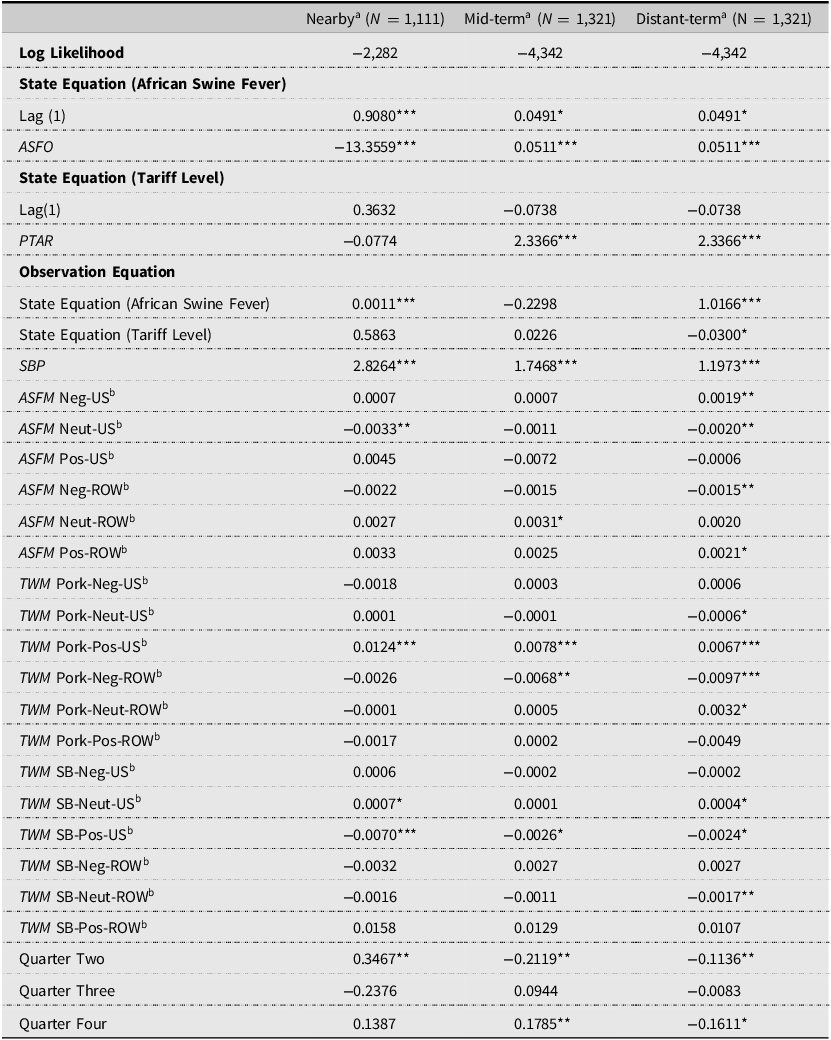
a Nearby = Contract set to expire next; Mid- = Contract expiring in 6 months; Distant- = Contact expiring in 12 months.
b Sentiments of Headlines: ASFM = African Swine Fever media content; TWM = Trade War Media Content; Pos = Positive Sentiment, Neut = Neutral Sentiment, Neg = Negative Sentiment; US = United States, ROW = Rest of World (e.g. ASFNegUS = United States headlines pertaining to African Swine Fever with a negative sentiment).
Note: ***Significantly different from zero at significance level α = 0.01, **at α = 0.05, and *at α = 0.1.
State equation regression
Results suggest that as confirmed ASF occurrences increased, the likelihood of a state change in the LHP increased for mid- and distant-horizon contracts (Table 4). This finding may result from the more significant effect of ASF on the Chinese breeding herd and the length of time required to rebuild that sector of the pork industry. Both the magnitude of the outbreak and the expected timeframe of inventory recovery have consequences for the long-term impacts on global pork demand. When media outlets reported the gravity of the situation in China, market analysts may have been uncertain as to whether China would return to its previous hog population size and, thus, domestic production levels, even as pork demand remained strong among Chinese consumers. As ASF occurrences increase, the likelihood of a state change in the nearby contract decreases. This finding again suggests the repercussions of losing a significant portion of the world hog inventory; prices would remain strong in the nearby contracts.
Results also indicate that as tariff levels increased, the likelihood of a state change increased in the mid- and distant-horizon contracts (Table 4). As the trade war slowly developed and incremental retaliatory tariff increases were implemented, results suggest that the increases were more likely to cause a state change in the futures prices. The increased likelihood of a state change may result from trader expectations that although tariff levels would revert to standard tariff levels on goods after negotiations were completed, the demand for U.S. pork products in the future may not return to previous levels. Also, while negotiations with China and retaliatory tariffs on pork exports destined for China may have dominated the media data, there were ongoing negotiations for what would eventually become the United States–Mexico–Canada Agreement. The successful conclusion of those negotiations may have generated positive expectations for a similarly successful conclusion of the U.S.–China trade negotiations.
Lean Hog futures price regression
The null hypothesis that the state equations do not affect lean hog futures prices (observation equation) was rejected for multiple time horizons (Table 4). The nearby- and distant-term contracts were related to the observation equation in the ASF state equation, suggesting that the latent components influenced futures contract prices. As the likelihood of a state change increased, there was a 0.11% increase in prices for the nearby contract and a 101.6% increase in the distant-horizon contract, respectively. This price increase following ASF outbreaks was expected, as was the relatively strong effect on the distant-term contracts. The market hog inventories and the breeding hog inventory in China were depleted during the ASF outbreak. The impact of the loss in the market hog inventory was felt immediately in China and worldwide as supplies diminished. Prices increased with the loss of hogs in China and pork-exporting countries as suppliers sought to meet pork demand. With the significant loss of breeding hogs, a price increase on the distant horizon was not unsurprising, as trade and production practices would have to shift to accommodate the consequences of ASF.
The state equation for tariff levels suggested that changes in tariff levels did not influence nearby lean hog futures prices. History and economic theory typically demonstrate that tariffs and trade wars lead to adverse outcomes and expectations for a country’s economy. The findings indicate that traders believed the trade war and tariffs would negatively influence markets in the next twelve months (i.e., distant-horizon contract).
This explanation is corroborated by producers’ expectations in the Purdue Ag Economy Barometer (Purdue University, 2018-2019). Throughout 2018, the question of how agricultural producers viewed the trade war was posed multiple times. Producers were concerned about the immediate impacts of higher tariffs on their net farm incomes from the loss of exports during the trade war in the first six to twelve months. Producers were still cautiously optimistic though, with an average of 56% from April 2018 to January 2019 believing that agricultural exports would increase over the next five years (Purdue University, 2018-2019).
The SSM results suggest that media information related to ASF and the retaliatory tariffs influence futures prices for all horizon contracts. Results suggest that media headlines in the U.S. as well as the ROW can both positively and negatively influence expectations of contracts in the distant future for all three-time horizons.
Futures prices also reflected the response to information disseminated outside the United States. Negative domestic ASF headlines were associated with an increase in futures prices of 0.19% for the distant-horizon contract. Negative media attention concerning ASF in the rest of the world led to a decline in futures prices by approximately 0.15% for distant-horizon contracts. Neutral ROW headlines created a potential 0.31% increase in futures prices for the mid-horizon contract however. At the peak of the ASF outbreak, many ROW headlines included reports of new outbreaks globally, not just in China. This negative response to world headlines may stem from disease risk concerns. As outbreaks increased globally, the concern of an outbreak in the U.S. increased. Consequently, the expectation of potential gains from global pork shortfalls was more than outweighed by concerns about protecting domestic pork inventories.
There was an increase in lean hog futures prices for all three-time horizons as positive information about the trade war on pork in the U.S. circulated. However, U.S. information containing positive sentiments toward the trade war and soybeans was associated with a decline in lean hog futures prices across all three contracts. This result accords with economic theory, as higher prices were potentially negotiated for soybeans, an input in hog production. For negative headlines in the ROW about the trade war and pork products, U.S. lean hog futures prices experienced a decline in the mid- and distant horizon contracts. When reviewing headlines for this classification, negative headlines typically discussed the increased tariff rate on U.S. pork products and seeking alternatives to U.S. pork products. This information would lead to a negative expectation of lean hog futures prices, given that exports to China should decline in typical circumstances.
The results of the seasonality dummy variables were as expected with typical cycles for hog prices (Table 4). For nearby soybeans contracts, though, the story is more complicated. Economic theory postulates that lean hog prices should decline as soybean prices increase. However, this analysis indicates that lean hog futures prices also increased as soybean prices increased. This result may have stemmed from other market circumstances; demand increased strongly during the same period. The cumulative effect of a strong domestic economy in 2019 with robust pork demand and global pork market dynamics led to a significant increase in pork prices domestically. Some export demand resulted from opening new foreign markets for U.S. pork, and some from increased year-over-year pork exports to China despite the retaliatory tariffs. Although soybean prices increased, lean hog futures prices were increasing to match the demand. It is evident, though, that as time from the expiration of the contract increased (i.e., distant horizon), the impact of soybeans prices had less of an impact as markets would adjust farther out, but demand would still be present.
Conclusions
Futures price expectations are established based on many types of information. While much of that information is based on market fundamentals, unexpected disruptions are also incorporated into future price expectations. Media influences from news platforms and social media play important roles in influencing futures markets. Previous research could not discriminate between the effects of negative and positive media information on markets. This study analyzed the impacts of media on nearby (1-month), mid-term (6-months), and distant-term (12-months) futures contracts and the influences of media’s coverage of uncertain events. The analysis used state-space modeling procedures to determine how much media coverage and digital conversations on ASF and the trade war in 2018–2019 influenced lean hog futures markets. Specific attention was given to direct (retaliatory tariff levels), and indirect (global swine inventories and soybean export demand) influences on commodity futures.
Results suggest that two simultaneous events can, to some extent, be examined separately. This finding is particularly useful when aggregate measures of total exports or lean hog futures prices digress from the expected movements based on price theory. Findings indicate that traders’ price expectations of event impacts may differ depending on the expiration date of the selected contracts and the information received. As economic theory predicts trade wars will generate negative expectations on futures prices due to the added cost of retaliatory tariffs, traders’ price expectations during the U.S.–China trade war were also found to be negative for futures contracts expiring 12 months later.
Agriculture is subject to the influence of unexpected occurrences, sometimes called “black swan” events. Aggregate economic measures can mask the complexities of market dynamics during unexpected simultaneous disruptions. In the future, multiple sources of information beyond fundamentals, including sentiment in media coverage, can be examined to explore the impacts of events on agricultural futures prices in the U.S. Improved understanding of how two conflicting pieces of information affect markets has meaningful policy impact. The Market Facilitation Program (MFP) was developed to address excess market losses to agricultural producers in response to retaliatory tariffs placed by China and also by Mexico in the course of renegotiations of the U.S. Mexico Canada Free Trade Agreement. Ex post analysis found that the MFP overpaid producers of most field crops being overpaid (Janzen and Hendricks, Reference Janzen and Hendricks2020) and the largest overpayments attributed to soybean producers estimated at $5.4 billion (Adjemian et al., Reference Adjemian, Smith and He2021). Swine and dairy producers received a smaller portion of MFP funds, about 4.1% in 2018 and 3.9% in 2019 (GAO, 2020). Although pork was the second most targeted commodity behind soybeans, products were still competitive in a global market impacted by ASF in China. Results from studies like this one can be used to refine damage estimates from globally disruptive events in designing ad hoc disaster programs.
This study has several limitations that could be addressed in future research. First, the study did not examine the implications of the continued recovery of China’s swine herd from ASF or the impact of ongoing pork tariffs and Phase One Trade Agreement implementation that was still ongoing as of March, 2020, when this dataset was truncated. The addition of data after COVID-19, which disrupted many aspects of the U.S. economy, was felt to add noise to the analysis rather than clarity. Therefore, this analysis did not consider the full recovery period, but it could be used in future research. It is also acknowledged that additional analysis could consider policy interventions occurring due to the trade war that affected the financial viability of the broader U.S. agricultural sector, namely the Market Facilitation Program payments received by producers in 2018 and 2019. Additional analysis could also be conducted on the influence of media regarding soybean futures prices.
Data availability statement
Media sentiment data are from Meltwater Software, a global company that provides online media monitoring and conversation capturing. The Meltwater analysis was obtained through a subscription by Oklahoma State University. Meltwater tracks conversations containing keywords on specific topics monitored across various sources such as online and televised news platforms, social media, print, broadcasts, and podcasts. Keywords and phrases must be present in media dialog for a headline to be included in the data. Sources ranged from newspaper headlines, television reports, radio broadcasts, and social media conversations on the events.
Author contributions
Conceptualization, T.M.G., A.D.H Methodology, T.M.G, A.D.H. D.M.L; Formal Analysis, T.M.G, A.D.H, D.M.L ; Data Curation, T.M.G and R.I.; Writing-Original Draft, T.M.G; Writing- Reviewing and Editing, T.M.G, A.D.H, D.M.L. R.I.; Supervision, A.D.H., D.M.L. ; Funding Acquisition, A.D.H.
Financial support
This work was supported in part by the OSU Agricultural Experiment Station and Hatch agreement OKL03128.
Competing interests
The authors declare no competing interest.
AI contributions to research
AI was not used in any way in the generation of this paper.