There has been a recent focus on out-of-area (OOA) placements, whereby a patient admitted to their local mental health trust for treatment is sent elsewhere to receive treatment. This is primarily because of lack of local bed availability. This can be traumatic for the patient, who loses contact with their support network of family and friends at a vulnerable time. Furthermore, OOA placements have been found to be two-thirds more expensive than best practice.Reference Edwards, Meier and Killaspy1 For these reasons the Royal College of Psychiatrists aims for OOA placements to be ‘minimised’,Reference Edwards, Wolfson and Killaspy2 and the Department of Health and Social Care has stated a target of eliminating ‘inappropriate’ OOA placements by 2020/2021.3 Moreover, mental health trusts in England must now report the monthly number of OOA placements.Reference Blackburn4
To reduce OOA placement rates bed availability must be better matched to local need. In-patient care is a complex system, with patients constantly arriving and leaving from a unit of finite capacity. Occupancy rates are also dependent on the capacity of neighbouring hospitals and out-patient services. Increasing bed availability is not always desirable or cost-effective. Over the past two decades, in England, specialised community services, such as ‘early intervention in psychosis’ (EIP) and ‘crisis teams’ have been implemented. There is evidence that some of these have reduced mental health bed occupancy via reduced length of stay and/or admission rates.Reference Glover, Arts and Babu5, Reference McCrone, Singh, Knapp, Smith, Clark and Shiers6 Thus, understanding the relative influence of referral rates and length of stay (LOS) would be valuable in planning which community services to invest in if OOA placements are to be avoided.
Discrete event simulation
As a complex system, modelling the flow of patients through an in-patient service is not amenable to conventional statistical approaches. However, numerical simulation techniques have previous been used as tools to support the planning of health services.Reference Zhu, Hoon Hen and Liang Teow7–Reference Vassilacopoulos9 One such simulation approach is discrete event simulation (DES), which models a system (for example a hospital ward) as a sequence of separate events (such as a patient presenting for treatment, a patient being discharged) which occur over a set period of time. DES is commonly used to model systems that can be described in this way, such as queues, manufacturing processes and financial investments. Here we use DES to create a ‘virtual ward’. We can change the conditions of the simulation which, in this case, allows us to estimate the number of OOA placements that may occur for varying referral rates and LOS. This report aims to demonstrate the potential of DES in mental health service planning.
Method
A virtual local in-patient unit was created with a fixed number of beds (n = 20). ‘Patients’ then presented, at any time, requesting admission. If a bed was available they were admitted to the (local) unit and treated for a variable amount of time. Once treatment was complete they were discharged. If no bed was available when a virtual patient required admission they were classed as an OOA placement. Thus, the simulation provided a simplified representation of reality, assuming that bed availability depended only on referral rate and LOS independently.
We assumed referral rate and LOS each followed ‘random distributions’ that had specified means and variances.Reference Forbes, Evans, Hastings and Peacock10 These values could initially be set to those observed in the actual service data provided by the Trust for one general, working-age, mental health ward. LOS is positively skewed (i.e. a small number of patients have unusually long admissions). Thus, we used a log-normal distribution for LOS. We chose an exponential distribution for the referral rate since this distribution describes the length of time between arrivals. We set initial values for the distributions based on observed data and that approximated those OOA rates reported in reality. These values could then be systematically varied so that the impact on the OOA rates could be evaluated. Specifically, by varying one parameter and holding the other constant, we could assess which factor had the largest impact on OOA rate. We then altered both parameters simultaneously to determine what combinations of reductions of LOS and referrals could plausibly achieve lower rates of OOA placements. Each simulation, implemented in the Python package SimPy, was repeated 1000 times and the results averaged.11
Results
Our real-world data showed 529 patients requested treatment at the ward over 851 days, equivalent to 1.61 days per request. The average patient spent 2.5 log (days) on the ward, and 17.2% of the patients could not be treated on the ward and thus must be treated elsewhere. Informed by the data we set the exponential distribution parameter (‘lambda’) as 1.61, which describes a process where patients arrive at the hospital on average 1.61 days after the previous patient arrived. We initially chose a log-normal distribution with mean 2.5 log (days) and standard deviation of 1.2. However, this value resulted in the simulation overestimating the OOA rate. Through trial and error we instead chose a mean of 2.2 log (days). This choice, along with the aforementioned choice of exponential distribution, produced an OOA rate in the simulation of 16.9% – close to the actual Trust-reported rate at that time of 17.2%.
Figure 1 shows the OOA rates for various values of the two parameters. Simulation showed that, around the initial values of the simulation, small changes in each parameter had a linear impact on the OOA rate. Holding LOS constant and simulating the effect of a 5% reduction in referral rate gave an OOA rate of 14.5%, a reduction of 2.4 percentage points. Similarly, holding the referral rate constant and simulating the effect of a 5% reduction in mean LOS gave an OOA rate of 14.3%, a reduction of 2.6 percentage points. Thus, OOA rates appeared to be equally sensitive to referral rate and LOS, over the range of values evaluated.
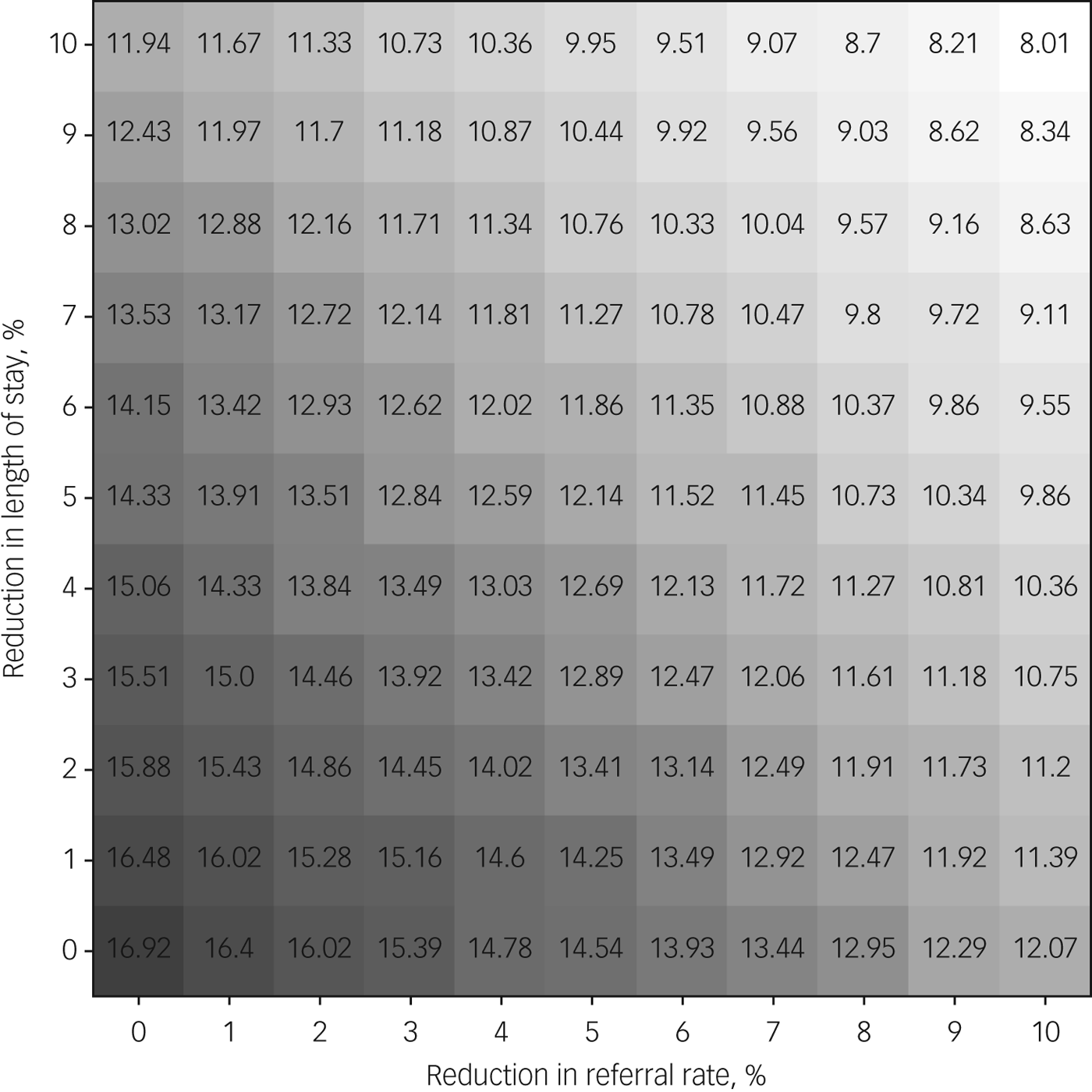
Fig. 1 Out-of-area admission rates from 1000 simulations of the ‘virtual’ ward for various reductions in referral rate and length of stay.
Figure 1 also allows us to determine which combination of referral rate and LOS may achieve lower OOA placements. For example, an OOA rate in the region of 8.6% (i.e. half the Trust-reported rate at that time) could be achieved by reducing the referral rate by 8% and LOS by 10%, or alternatively by reducing the referral rate by 10% and LOS by 8%.
Discussion
The results of our simulations suggest that reducing LOS and lowering referral rates have a similar impact on OOA rates. Reducing the amount of time a patient spends on a ward is perhaps more practical – clinically it is not always possible to avoid admission if there are high levels of perceived risk to the patient or others. However, resources can often be coordinated to facilitate a rapid discharge. Integrated models of care, where community services stay involved with patients during an admission, may be effective at reducing LOS (for example in EIPReference Tiffin and Hudson12). Indeed, the data we used in this study came from an area with crisis teams and EIP implemented, which will have already had a positive impact on the referral rate and LOS. Nevertheless, our findings suggest that, in the absence of increased in-patient capacity, substantial reductions in both demand and LOS would be needed to achieve OOA placement rates of under 10%.
The presented model is clearly a simplification of the real-world problem. However, the ability to mimic the real-world data suggests that even such a simple simulation has some utility. Modelling an interdependent system of wards and hospitals and the possible dependency between referral rate and LOS would demand a more complex approach, although it is feasible within a DES framework.
Our results suggest that further investing in community services that both reduce LOS (such as EIP) and referral rates (for example crisis teamsReference Glover, Arts and Babu5) are likely to be effective in reducing OOA placement rates. We noted that the median LOS in the Trust data used to develop the model was somewhat lower than the national value for 2013–2014 (15 v. 23 days).13 This may be because the Trust in question already has well-embedded specialist community services. Moreover, the population served is characterised by relatively low levels of urbanicity. This suggests that services with longer baseline LOS values may be able to achieve somewhat greater reductions in OOA rates via the use of community services. It is also likely that reduced social care provision will lead to delayed discharges in some cases, perhaps predominantly affecting those patients with longer hospital stays. Such influences could be modelled via more complex simulation studies that could guide the optimum allocation of resources at a time of increased financial restraints.
Funding
This work was funded by the Tees, Esk and Wear Valleys NHS Foundation Trust.
eLetters
No eLetters have been published for this article.