1 Introduction
Forecasting is a vital part of intelligence analysis that shapes national security policymaking (Kent, 1994a, 1994b). Forecasts constitute a large proportion of strategic intelligence assessments (Reference Mandel and BarnesMandel & Barnes, 2014), most of which are made by subject-matter experts with input from peers and managers. Given that intelligence failures can cost billions or even trillions of dollars and incalculable human loss and grief, even small improvements in forecasting accuracy easily justify multi-million dollar investments in methods that improve the accuracy of intelligence forecasts. For this reason a recent article in this journal by Stastny and Lehner (2018 [S&L2018]) entitled, “Comparative evaluation of the forecast accuracy of analysis reports and a prediction market” should generate considerable interest, especially among those tasked with improving the intelligence tradecraft.
S&L2018 aimed to compare the forecast accuracy of traditional intelligence analysis with a novel method for the intelligence community (IC): a prediction market operated on a classified network, which only US government intelligence analysts could access. Foreshadowing the apparent benefits of the IC prediction market (ICPM) over traditional analysis, the authors point to the putative value of “crowd wisdom methods” (p. 202). Yet it is unclear precisely what this means. Prediction-market forecasts integrate opinions from multiple forecasters (assuming more than one trade per topic), but arguably so do forecasts from traditional analysis, which if not made by analytic teams will at least typically require peer review and managerial oversight. Forecasts on weighty topics might be further subjected to structured challenge-function techniques designed to pry open potentially closed minds through adversarial collaborations.
Performance differences could in fact be caused by a variety of methodological differences. For instance, ICPM forecasters choose what topics they want to bid on — a luxury that is not extended to analysts forecasting in the traditional mode where topics are usually assigned or at least shaped in consultation with managers. ICPM forecasters also determine when to forecast and when to update, whereas information requests by policymakers are invariably time sensitive and may not afford opportunities for updating. ICPM forecasters get unambiguous feedback on the accuracy of their forecasts, which provides them with a basis for personalized calibration training (Reference RieberRieber, 2004). ICPM analysts are also self-selected forecasters representing a small subset of the overall US analytic community. Selection bias in the ICPM may favor not only analysts who like making forecasts and are comfortable with quantitative assessments but also those who are better at it. For instance, superforecasters prefer the opportunity to express their forecasts on granular, numeric response scales (Reference Tetlock and GardnerTetlock & Gardner, 2015), which the ICPM affords but which traditional analysis denies. Analysts working in the traditional mode are also under far greater accountability pressures, a factor that might explain why strategic intelligence forecasts of greater policy importance have been found to be more underconfident than those of lesser policy relevance (Reference Mandel and BarnesMandel & Barnes, 2014). In short, determining why the ICPM might outperform traditional analysis, if indeed that were the case, would be no simple matter based on a direct comparison of the two approaches.
S&L2018 does in fact conclude that the ICPM outperformed traditional analysis in terms of forecasting accuracy. This is stated in the abstract as the study’s primary result: “First, the primary result is that the prediction market forecasts were more accurate than the analysis reports. On average prediction market probabilities were 0.114 closer to ground truth than the analysis report probabilities” (p. 202). Likewise, S&L2018’s Discussion states: “In general crowd wisdom forecasts are more accurate than expert forecasts, no matter how the expert forecasts are expressed” (p. 210). The claim of significantly better performance by ICPM than traditional analysis is the principal reason why this paper is likely to attract attention from IC professionals seeking advances in analytic methods.
How confident should the IC — or any reader for that matter — be in the claim that the ICPM outperformed traditional analysis in terms of forecasting accuracy? And how confident should they be that the benefit ICPM confers in terms of accuracy is due to harnessing the wisdom of the crowd? I think readers should be somewhat skeptical — and this view does not reflect my ideological priors. I have written in several recent papers about the limitations of traditional intelligence analysis and have recommended that the IC take a close look at post-analytic methods such as recalibration and aggregation, which pair easily with methods like prediction markets and forecasting tournaments (e.g., Mandel, in press; Reference Mandel, Karvetski and DhamiMandel, Karvetski & Dhami, 2018; Reference Mandel and TetlockMandel & Tetlock, 2018). I have also recommended that the IC adopt transparent accuracy monitoring processes, such as those that the ICPM can effectively deliver (Reference MandelMandel, 2015; Reference Mandel and BarnesMandel & Barnes, 2018). My skepticism is instead based on consideration of the limitations in S&L2018’s research methods as well as additional results from S&L2018’s study that were unreported. My aim in expressing such doubt is certainly not to diminish the substantial research effort undertaken. This type of research is as important as it is scarce and difficult to execute. I will not belabor small points.
At the crux of the matter is the method used to estimate forecasts in the traditional analytic products examined. In brief, S&L2018 identified assessments in finished intelligence products and drafted a set of well-defined forecasting questions from them. Not all questions passed Tetlock’s (2005) clairvoyance test, but I will not quibble about the validity of including the fuzzy questions (28 out of a total of 99 questions), which in any case appear to have been readily answerable. I will note, however, that efforts could have been made to establish inter-rater reliability on ground truth (Reference Mandel and BarnesMandel & Barnes, 2014), which is important because the “reality” used to score forecast accuracy was judged by subject-matter experts. However, this is of secondary concern because instructions for categorizing occurrences and non-occurrences were quite detailed (except, of course, for the fuzzy subset).
The forecasting questions were launched on the ICPM and the elicitation from forecasters who responded through that system seems uncontroversial. However, this is not so for the estimation of forecasts from the traditional analysis. In this case, a subset of five analysts who served as forecast imputers did the following: First, before reading the intelligence product from which a forecast question was developed, they were given the question and asked to provide their personal forecast. Afterwards, they were asked to read the entire intelligence product from which a given question was developed, and then they imputed the forecast that the product as a whole conveyed. Next, they were asked to provide an updated imputation based on new information available since the report was written. Finally, they provided an updated personal forecast. All four forecasts were made on the same 0–100 percent-chance probability scale.
The serial process of eliciting personal forecasts followed by imputation of forecasts from reports (i.e., the first two forecasts elicited from imputers) raises concerns about the validity of the resulting data. It is psychologically implausible that imputers could simply put their recently elicited forecasts aside to focus on extracting the forecast implied in entire intelligence reports. Why should they be credited with such abilities if, for instance, judges cannot effectively disregard inadmissible evidence even when clearly knowing that they should (Reference Wistrich, Guthrie and RachlinskiWistrich, Guthrie & Rachlinski, 2005)? S&L2018’s design establishes fertile ground for mental contamination (Reference Wilson and BrekkeWilson & Brekke, 1994) and the expression of ironic processes of mental control (Reference WegnerWegner, 1994). What is more, it is no easy feat to draw a point-estimate forecast on a specific question from an entire intelligence report. We have no basis for knowing whether imputers can do this reliably because their test-retest reliability was not examined. It is plausible that given the difficulty of the task, imputers would be prone to reach for low-hanging cue-substitution opportunities, such as drawing on accessible personal beliefs recently generated in just the right response format for the task at hand (Reference Kahneman, Frederick, Gilovich, Griffin and KahnemanKahneman & Frederick, 2002). One would expect them to be automatically prone to substitute their personal forecasts for the imputed forecasted they were asked to make.
Perhaps anticipating methodology-focused objections such as these, S&L2018 addressed the threat to validity posed by the fixed ordering of the imputers’ forecast elicitations by using a distance-free reference-dependent measure of agreement that could only be applied to forecasting questions in which at least two imputers made forecasts. In these 210 cases (out of 258), the average imputed forecast for a question was calculated and the imputer’s personal and imputed forecasts were coded as either consistent (i.e., both above or both below the average) or inconsistent (i.e., one above and one below the average). S&L2018 found that a significantly greater proportion (61%) was consistent than was inconsistent (39%), but emphasized the smallness of the effect (d = 0.11), subsequently concluding: “On balance these results suggest that the professional analysts who were our readers did a reasonable job of putting aside their personal views when making imputation judgments, but that they were not immune from this effect” (p. 206).
2 Additional analyses
Is the claim that imputers did a “reasonable job” of putting aside their personal views when making imputation judgments justified? I do not think it is. Consider a more standard measure of association that does not require case exclusions or arbitrary reference points. From the authors’ dataset, one can verify that the Pearson correlation between imputers’ personal and imputed forecasts is r(256) = .52, p < .001. This is a large effect size by conventional standards. However, the correlation between mean Brier scores for their personal and imputed forecasts across the five imputers approached the limit: r(3) = .98, p = .005. The claim that imputers did a reasonable job of separating their personal views from their imputations cannot be sustained given these findings. To the contrary, their imputations are strongly related to their personal beliefs — and the accuracy of the latter largely predicts the former. Therefore, S&L2018 have effectively compared the ICPM forecasts to five out-of-market analysts.
We can conduct further validity tests. For instance, one might reasonably assume that, if imputed forecasts were cold readings of implicit forecasts in intelligence reports, then the accuracy of imputed forecasts would surpass the accuracy of the imputers’ personal forecasts. Yet we find the opposite result: as Table 1 shows, the mean Brier score of imputers’ initial personal forecasts (M = 0.194, SD = 0.238) is significantly lower (i.e., more accurate) than their initial imputed forecasts (M = 0.252, SD = 0.273), t(257) = −3.66, p < .001, d = 0.23. S&L2018 also reported this difference using a different accuracy measure. The authors argue that there is little reason to expect that the analysts who produced the reports would provide more accurate forecasts than the imputers, because Tetlock (2005) found that experts forecasting on topics in which they had expertise were no more accurate than “dilettante” experts who forecasted on topics in which they did not have expertise. However, this does not explain why dilettante imputers would be significantly better than the experts the US government chose to assign to the topics.
Table 1: Mean Brier scores by forecast type for total and non-fuzzy item sets
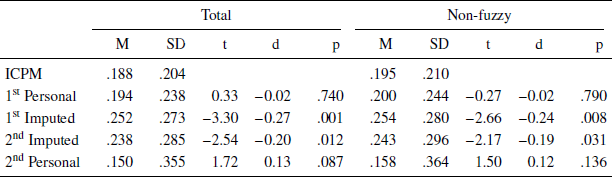
Note. The t-tests compare ICPM accuracy to the accuracy of the forecast type indicated in the row; df = 257 for the total item set and df = 212 for the non-fuzzy item set.
Furthermore, an unreported fact in S&L2018, which can be seen in Table 1, is that imputers’ personal forecasts do not significantly differ in accuracy from the ICPM forecasts. This is true of the total set of items and for the non-fuzzy subset that passed the clairvoyance test. In other words, imputers’ personal forecasts made on their own in the absence of any crowd wisdom were, on average, just as accurate as crowd–based ICPM forecasts. Moreover, among the total set of forecasting items, imputers’ updated personal forecasts (made after reading the intelligence reports) were marginally more accurate than ICPM forecasts. Taken together, the findings suggest that imputers are much like other analyst forecasters on the ICPM in terms of their forecasting skill — perhaps even better since their accuracy did not benefit from aggregation or the exchange of rationales for forecasts permitted on the ICPM. However, imputers’ accuracy declines when they are required to infer what forecast an intelligence report conveys about a forecasting topic rather than forecasting on the topic directly. This is as one might expect given the difficulty of the imputation task. Imputers are likely to use their personal estimates as cues to the imputation task — and to do so automatically and without conscious awareness. Moreover, any conscious efforts to suppress such a process that they might undertake would be likely to add noise to the estimates as imputers over- or under-correct for these inaccessible influences on judgment. The expected net effect is a reduction of signal value in the imputed forecasts, which is precisely what is observed.
For the total set of forecasting items, the accuracy of the five imputers ranged from a (best) mean Brier score of 0.145 (SD = 0.193) to a (worst) score of 0.362 (SD = 0.275). The size of this effect is large — almost a full standard deviation: Cohen’s d = −0.91. Clearly, how accurate the traditional analysis appears to be in this study will depend on whether imputers are better or worse forecasters. The purported accuracy of traditional analysis will depend substantially on variance in rater accuracy. Both the best and worst forecasters among the five imputers provided imputations that were highly correlated with their personal forecasts: r(73) = .49 for the best forecaster and r(31) = .53 for the worst forecaster among the imputers.
Additional evidence for the present skeptical assessment could be provided. For instance, the calibration curves of personal forecasts and imputed forecasts are highly similar, both indicating overprediction bias, a tendency associated with underweighting of base rates when they happen to be low (Reference Koehler, Brenner, Griffin, Gilovich, Griffin and KahnemanKoehler, Brenner & Griffin, 2002). However, the reporting of such analyses is of little value beyond making the case already made. We do not need to know about the calibration of five imputers.
3 Conclusion
If the IC wants to know how well traditional analysis stacks up against the ICPM or other alternative approaches, it should sponsor less ambiguous trials. A set of forecasting questions could be given to individual analysts or small teams of analysts who would use a conventional approach to reach their forecasts and the same set of questions could be given on the ICPM. Different trials could attempt to isolate the effect of alternative causes of putative performance differences. For instance, analysts in one condition could use the set of seven linguistic probabilities approved for use in Intelligence Community Directive 203 (Office of Director of National Intelligence, 2015). The linguistic probabilities could be translated into numerical probabilities by eliciting best equivalents from either the analysts or from mock or real intelligence consumers (e.g., Reference Ho, Budescu, Dhami and MandelHo, Budescu, Dhami & Mandel, 2015; Reference Mandel and BarnesMandel & Barnes, 2018; Reference Wintle, Fraser, Wills, Nicholson and FidlerWintle, Fraser, Wills, Nicholson & Fidler, 2019). Alternatively, researchers could use the midpoint of the numeric ranges used to set bounds on the interpretation of those terms in the IC directive. In another condition, analysts might be instructed to use numeric probabilities from the start.
The comparison of analytic methods as used by analysts is not difficult to conduct, in principle. It is difficult in practice mainly because of the rare opportunities the IC creates to run such tests. Yet these tests are vital to bridge the yawning gap between current analytic practices and the results produced by multi-million-dollar Intelligence Advanced Research Projects Activity (IARPA) programs such as Aggregative Contingent Estimation (better known as ACE) and ongoing IARPA programs such as Hybrid Forecasting Competition (HFC) and the Forecasting Counterfactuals in Uncontrolled Settings (FOCUS). The IC should create opportunities to field test the most promising methods that come from these programs and use traditional analytic methods as baseline measures. The ICPM is a rare example of forecasting-science experimental uptake in the IC and a close approximation to a proposal made by Looney (2004) in response to the Defense Advanced Research Projects Activity’s highly controversial Policy Analysis Market (PAM), which was cancelled a day after being announced. The ICPM and other similar ventures deserve fair comparative tests to allow researchers and decision-makers to gauge their potential to inform policy decision-making in government.
As for the test of the ICPM that S&L2018 provides, the results are not positive. Five analysts who made forecasts on their own without the benefit of any crowd wisdom methods produced forecasts that were as accurate, on average, as those produced from the ICPM. If given the chance to update their forecasts after having read an intelligence report that addressed the topic, these analysts were marginally more accurate than those forecasting on the ICPM. The threats to the validity of the study, however, cut both ways. The present findings do not rule out the benefit of prediction markets in the IC, let alone other post-analytic methods that can be used to recalibrate and aggregate forecasts. Like a button-lipped witness, they neither confirm, nor deny.