Impact statement
Extinction is the irreversible loss of unique life forms. Ongoing climate change is predicted to cause significant loss of biodiversity, meaning loss of species, genes and ecosystems. This could lead to multiple negative consequences for human society as important ecosystem functions are also being lost. Understanding and predicting species extinctions for scenarios of future climate change is thus of main interest for science and people. Most estimates of future extinction risk rely on correlative species distribution models (SDMs). These relate the observed distribution of the focal species to observed environmental characteristics and then make forecasts where the species will find suitable environmental conditions in the future. We summarise how these models can be used to predict extinctions and what are the challenges and limitations of this approach. For example, these models ignore how long it might take until species go extinct after the loss of their habitat. Many processes and factors determining the loss of the few last individuals of a species are currently not well understood, and we highlight where particular care must be taken in the model building steps and where more detailed investigations into these processes are needed to improve predictions of species extinction risks. Despite prevailing challenges, there is high demand for estimates of future extinction risk. Overall, SDMs currently provide the most accessible method to estimate climate-related extinctions across multiple species, and those predictions, although uncertain, are needed by society to prepare adaptive strategies and policies for mitigating the consequences of human-induced climate change.
Introduction
Species distribution models (SDMs) are the main source to estimate the magnitude of climate change-related species extinctions (Urban, Reference Urban2015; Warren et al., Reference Warren, Price, Graham, Forstenhaeusler and VanDerWal2018), although inferring extinction risks from SDMs is controversial (Hampe, Reference Hampe2004; Dormann, Reference Dormann2007; Araújo and Peterson, Reference Araújo and Peterson2012). Currently, SDMs are the most widely used tools for assessing climate change impacts on biodiversity (Araújo et al., Reference Araújo, Anderson, Barbosa, Beale, Dormann, Early, Garcia, Guisan, Maiorano, Naimi, O’Hara, Zimmermann and Rahbek2019) and for evaluating current and future ranges of species (e.g., Thomas et al., Reference Thomas, Cameron, Green, Bakkenes, Beaumont, Collingham, Erasmus, de Siqueira, Grainger, Hannah, Hughes, Huntley, van Jaarsveld, Midgley, Miles, Ortega-Huerta, Peterson, Phillips and Williams2004). Many SDM studies have attempted forecasts under climate scenarios, for example, assessing climate change vulnerability of species in terms of potential range loss (e.g., Zhang et al., Reference Zhang, Nielsen, Stolar, Chen and Thuiller2015; Martín-Vélez and Abellán, Reference Martín-Vélez and Abellán2022), differences in seasonal range loss for species with different IUCN Red List status (Zurell et al., Reference Zurell, Graham, Gallien, Thuiller and Zimmermann2018) or contrasting effects of dispersal or local adaptation on climate-related extinction risk (Thuiller et al., Reference Thuiller, Broennimann, Hughes, Alkemades, Midgley and Corsi2006; Román-Palacios and Wiens, Reference Román-Palacios and Wiens2020). Also, SDM-derived extinction risk estimates regularly inform political processes (IPCC, Reference Pörtner, Roberts, Tignor, Poloczanska, Mintenbeck, Alegría, Craig, Langsdorf, Löschke, Möller, Okem and Rama2022, Chapter 2.5).
Despite the widespread use of SDMs in climate change research, they also remain criticised and regularly spark debate (Dormann et al., Reference Dormann, Schymanski, Cabral, Chuine, Graham, Hartig, Kearney, Morin, Römermann, Schröder and Singer2012; Thuiller et al., Reference Thuiller, Münkemüller, Lavergne, Mouillot, Mouquet, Schiffers and Gravel2013). For example, when Thomas et al. (Reference Thomas, Cameron, Green, Bakkenes, Beaumont, Collingham, Erasmus, de Siqueira, Grainger, Hannah, Hughes, Huntley, van Jaarsveld, Midgley, Miles, Ortega-Huerta, Peterson, Phillips and Williams2004) inferred global estimates of extinction risks across plants and animals by synthesising studies that applied SDMs under future climate change scenarios, their results were criticised in several commentaries (Buckley and Roughgarden, Reference Buckley and Roughgarden2004; Thuiller et al., Reference Thuiller, Araújo, Pearson, Whittaker, Brotons and Lavorel2004). Main criticisms referred to conceptual challenges related to how SDM predictions relate (or not) to extinction risk of species, to underlying SDM assumptions and to methodological challenges. Still, the number of SDM applications, also in relation to global change, is constantly increasing (Araújo et al., Reference Araújo, Anderson, Barbosa, Beale, Dormann, Early, Garcia, Guisan, Maiorano, Naimi, O’Hara, Zimmermann and Rahbek2019) and meta-analyses indicated that of all studies that estimate extinction risk under future climate, 76% are based on SDMs (Urban, Reference Urban2015). In the absence of better-suited alternative methods to estimate climate change-related extinction risk, SDMs seem to remain the most practical methodology despite well-founded criticism. In this review, we first explain the basics of SDMs and provide a literature overview over the use of SDMs for quantifying climate change impacts and extinction risk. Then, we describe how SDMs are currently used to inform extinction risk estimates and discuss the contentions from conceptual and methodological viewpoints. Finally, we summarise current good practice.
What are species distribution models?
Correlative SDMs (a.k.a. habitat suitability model, ecological niche model and environmental envelope model, among others; Elith and Leathwick, Reference Elith and Leathwick2009) relate geographic occurrences of organisms to prevailing environmental conditions (abiotic, biotic or both) within a statistical or machine-learning framework (Guisan and Zimmermann, Reference Guisan and Zimmermann2000; Guisan and Thuiller, Reference Guisan and Thuiller2005). The inferred model describes the species–environment relationship informing how habitat suitability scales with different environmental predictors. This relationship can be projected into geographic space using layers of environmental predictors to predict suitable habitat under current environment or scenarios of future (or past) environments (Figure 1). In many cases, SDMs are fit to climatic predictors, especially when predicting future scenarios. When prediction is the goal, predictive accuracy and transferability to new times and places should be validated, which is not trivial as independent data are often missing (Araújo et al., Reference Araújo, Pearson, Thuiller and Erhard2005; Yates et al., Reference Yates, Bouchet, Caley, Mengersen, Randin, Parnell, Fielding, Bamford, Ban, Barbosa, Dormann, Elith, Embling, Ervin, Fisher, Gould, Graf, Gregr, Halpin, Heikkinen, Heinänen, Jones, Krishnakumar, Lauria, Lozano-Montes, Mannocci, Mellin, Mesgaran, Moreno-Amat, Mormede, Nowaczek, Oppel, Crespo, Peterson, Rappaciuolo, Roberts, Ross, Scales, Schoeman, Snelgrove, Sundblad, Thuiller, Torres, Verbruggen, Wang, Wenger, Whittingham, Zharikov, Zurell and Sequeira2018; Zurell et al., Reference Zurell, Franklin, König, Bouchet, Serra-Diaz, Dormann, Elith, Fandos Guzman, Feng, Guillera-Arroita, Guisan, Leitão, Lahoz-Monfort, Park, Peterson, Rapacciuolo, Schmatz, Schröder, Thuiller, Yates, Zimmermann and Merow2020). By applying a threshold approach (Liu et al., Reference Liu, Berry, Dawson and Pearson2005, Reference Liu, White and Newell2013), the model output of habitat suitability can be transformed into predicted presence (and predicted absence), which can be interpreted as potential distribution of the species given the environmental conditions. The realised distribution of the species might deviate from the predicted potential distribution because of underlying ecological processes and methodological challenges (Figure 1; Soberón, Reference Soberón2007; Elith and Leathwick, Reference Elith and Leathwick2009), which we will further discuss below.
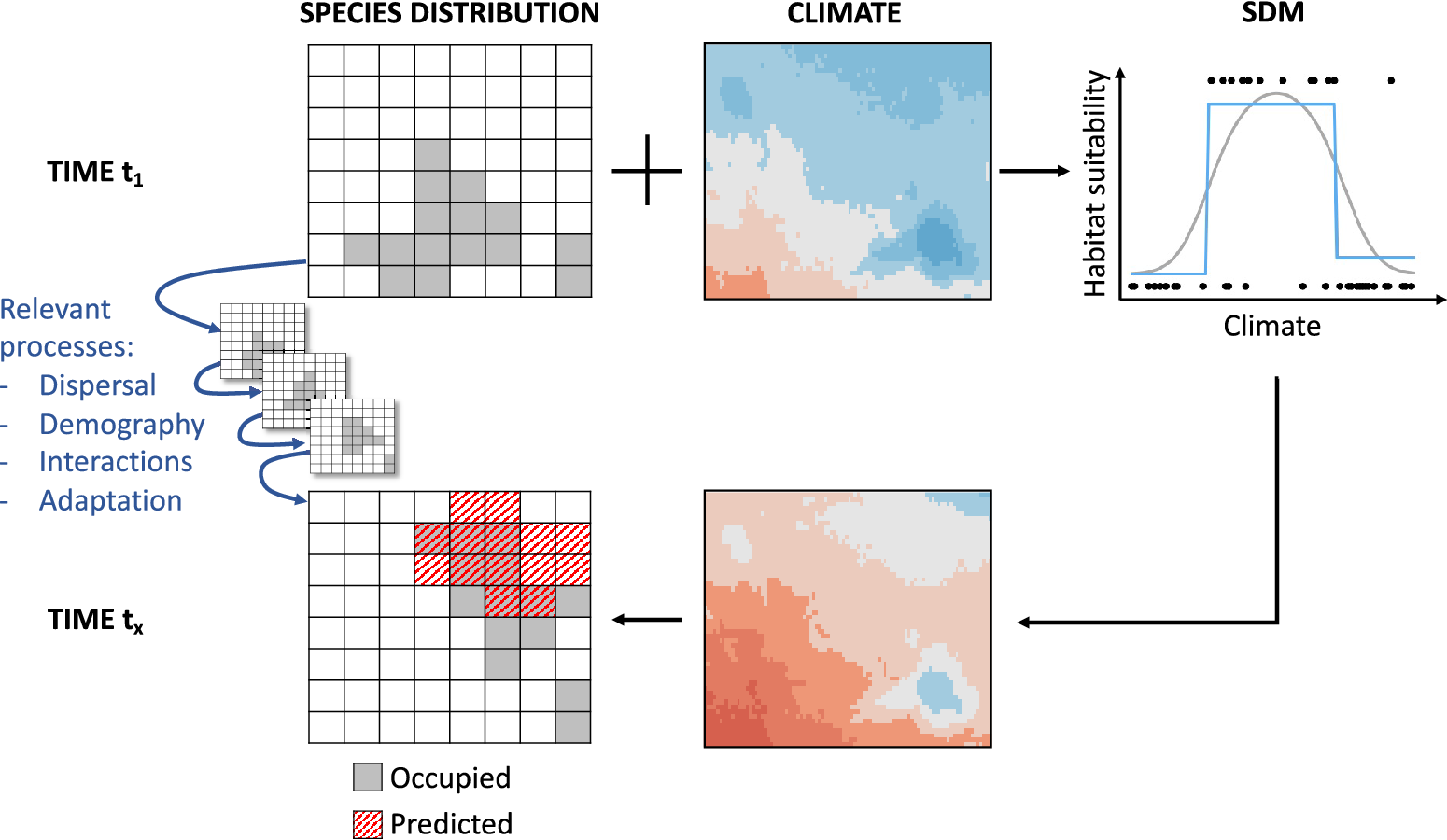
Figure 1. Conceptual overview of correlative species distribution models (SDMs) used for prediction under climate change. SDMs are fitted to observed occurrence data and climatic (or, more generally, environmental) data in time step t 1 (upper row of figures) using adequate statistical and machine-learning approaches (top-right plot shows two example approaches as grey curve and blue step function). The fitted species–environment relationship is then used to make predictions of habitat suitability and potential distribution at time step tx given future climate (or environmental) layers (lower row of figures). The potential future distribution derived from SDMs can differ from the true distribution at time step tx as the latter will be co-determined by the biological processes of dispersal, demography, species interactions and genetic or behavioural adaptation leading to transient dynamics (small figures in the middle).
Recent years have seen considerable advances in SDM algorithms and accompanying methods for fitting SDMs (Valavi et al., Reference Valavi, Guillera-Arroita, Lahoz-Monfort and Elith2021). Also, digital availability of biodiversity data and environmental data including climate scenarios has increased strongly (Wüest et al., Reference Wüest, Zimmermann, Zurell, Alexander, Fritz, Hof, Kreft, Normand, Cabral, Szekely, Thuiller, Wikelski and Karger2020). These factors have contributed to widespread use of SDM techniques. To quantify how many SDM-related studies exist and how many of these target climate change and species extinctions, we conducted a keyword-based literature search in the Web of Science on 21 July 2022 for papers published in 1900–2021 (see Table 1 for list of keywords). First, we identified all studies that mention SDMs (or synonyms). This revealed more than 40,000 studies published over all disciplines, with the first SDM mention in 1969 and a steady increase since 1990 (Figure 2). We then refined the list of papers by adding keywords related to climate change (Table 1). Of all SDM studies, climate change was mentioned in c. 20% and with increasing frequency through time (Figure 2C). Finally, we further refined the list of papers by adding keywords related to extinction or population declines (Table 1). Interestingly, extinction was mentioned only in one study related to SDMs and climate change before 2002. Since then, the absolute number of climate change-related SDM studies mentioning extinction increased, culminating in 171 such studies published in 2021. Yet their relative proportion decreased over time (average proportion c. 18%; Figure 2C).
Table 1. Web of Science search terms used in the literature search on 21 July 2022
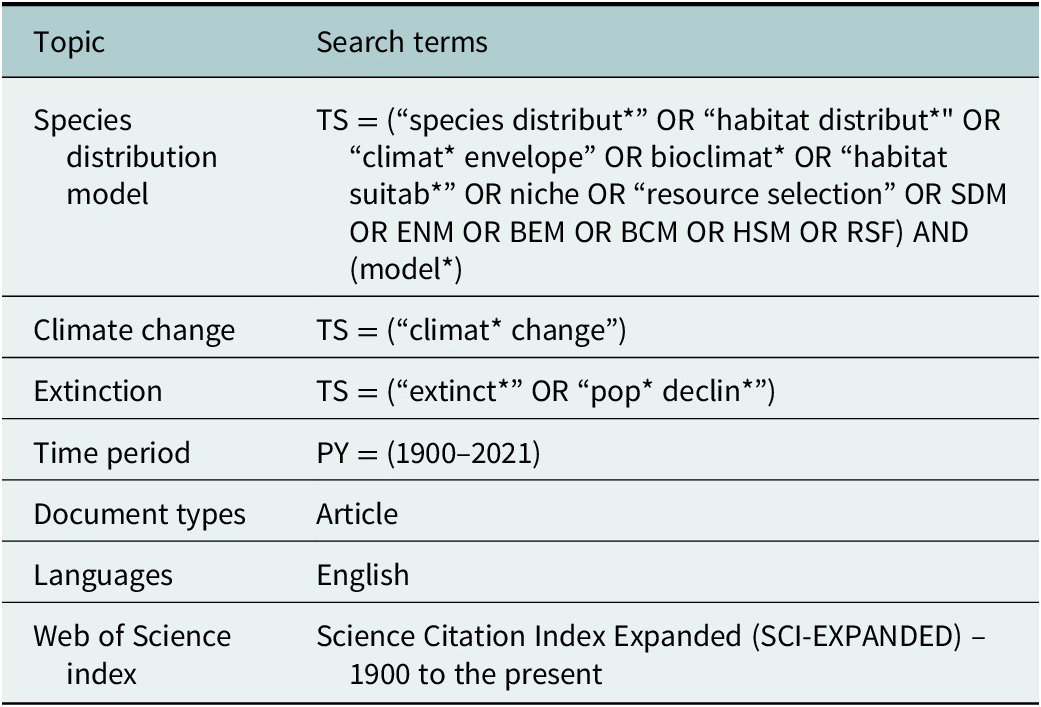
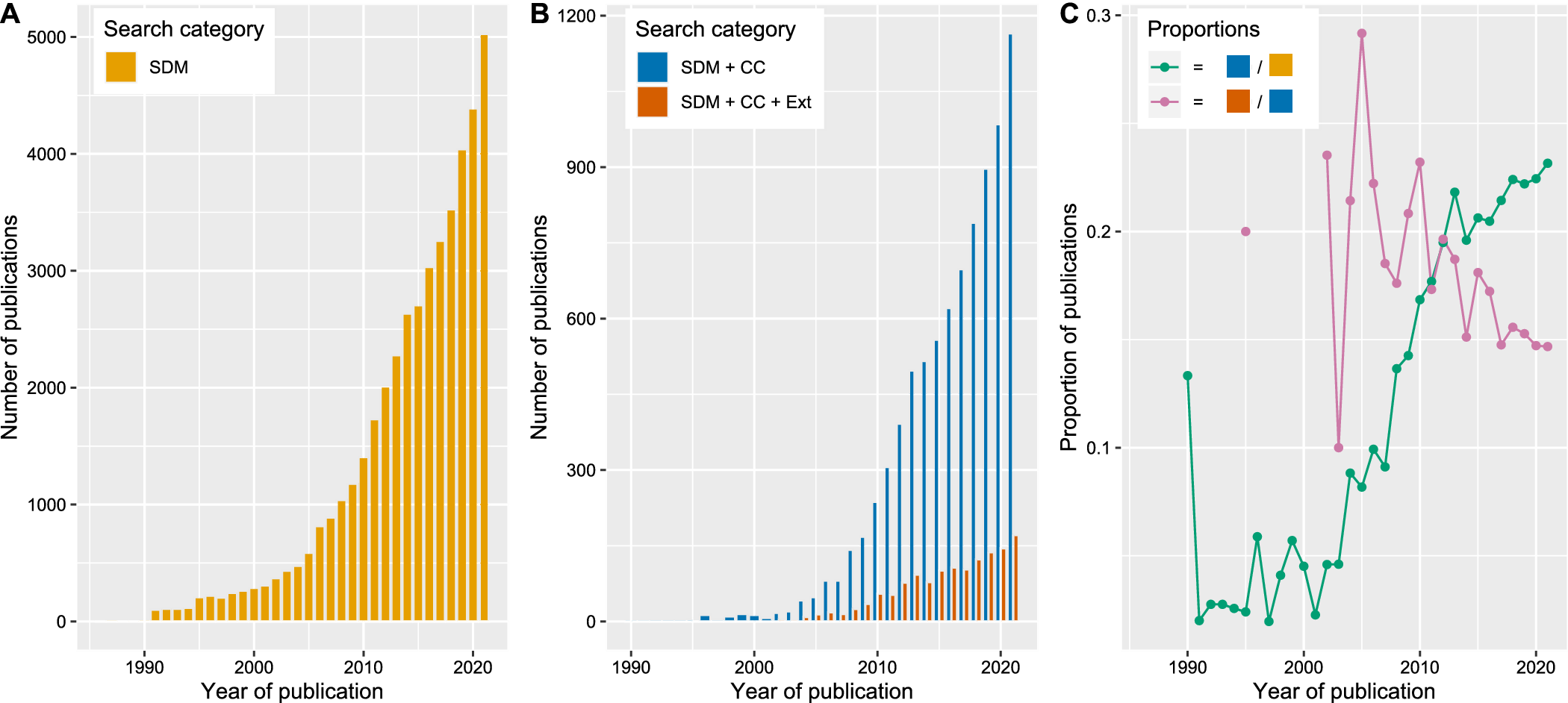
Figure 2. Use of correlative species distribution models (SDMs) over the last three decades. We extracted all studies from the Web of Science (see the keywords in Table 1) between 1900 and 2021 and classified them according to whether they were used in a climate change context and whether they mentioned extinctions or population declines. Earliest SDM studies appeared in 1969 with one to three publications per year until 1985. For easier visualisation, we only show publications published after 1985. (A) shows the absolute number of SDM publications per year. (B) shows the absolute number of SDM publications that mention climate change (CC) and those that mention both CC and extinctions (Ext). (C) shows the proportion of different SDM studies per year: green indicates the proportion of all SDM studies per year that mention climate change and purple indicates the proportion of all climate change-related SDM studies per year that mention extinction or population decline.
As this simple keyword search could provide an overoptimistic number of hits, we assessed a randomly drawn subset of 300 publications from the final set of articles that mentioned SDMs, climate change and extinction in more detail (see the Supplementary Material). All articles were screened by the same assessor, first screening the abstracts for determining whether the study applied SDMs and then screening the entire article for inclusion of climate change scenarios and quantitative estimates of extinction risks. Of the 300 articles, 203 publications indeed used SDMs, 161 applied SDMs under climate scenarios (150 under future scenarios and 11 under historic scenarios), 134 quantified future climate-related range changes from SDMs and 74 of these studies implied or provided inference on extinction risk (see the Supplementary Material). Thus, while the large majority of SDM studies do not explicitly aim at predicting extinctions, SDMs are clearly used for deriving species’ extinction risk under climate change. In fact, most extinction risk estimates reported in the IPCC AR6 are based on SDMs (IPCC, Reference Pörtner, Roberts, Tignor, Poloczanska, Mintenbeck, Alegría, Craig, Langsdorf, Löschke, Möller, Okem and Rama2022, pp. 256–261).
How is extinction risk derived from species distribution models?
SDMs can predict climatically suitable areas (or, more generally, environmentally suitable areas). An assumed increase in extinction risk with the decline in suitable habitat underlies most estimates of extinction risks from SDMs. They reflect theoretical understanding from island biogeography that smaller areas can harbour less individuals and smaller populations face a higher risk of extinction (species–area relationship [SAR]; MacArthur and Wilson, Reference MacArthur and Wilson1967). While generally accepted, the precise relationships between range size decline, population decline and extinction probability are unknown for most species (reviewed in Mace et al., Reference Mace, Collar, Gaston, Hilton-Taylor, Akçakaya, Leader-Williams, Milner-Gulland and Stuart2008). Often, guidelines from the extinction risk classification formalised by the IUCN Red List of Threatened Species (IUCN, 2001, 2022) are used for translating SDM-derived estimates of range-size declines to extinction probabilities (e.g., Ahmadi et al., Reference Ahmadi, Hemami, Kaboli, Malekian and Zimmermann2019; IPCC, Reference Pörtner, Roberts, Tignor, Poloczanska, Mintenbeck, Alegría, Craig, Langsdorf, Löschke, Möller, Okem and Rama2022), while this simplified translation lacks empirical evidence (Akçakaya et al., Reference Akçakaya, Butchart, Mace, Stuart and Hilton-Taylor2006). Originally, the IUCN “categories of threat […] provide an assessment of the likelihood that […] the species will go extinct within a given period of time” (Mace and Lande, Reference Mace and Lande1991). Based on quantitative analyses such as a population viability analysis (PVA), species are, for example, classified as “critically endangered” when they face a >50% likelihood of extinction within the coming 10–100 years (depending on generation times), and “endangered” with a likelihood of extinction of >20% (criterion E; IUCN, 2001, 2022). However, due to insufficient or uncertain training data for PVA models, extinction probability estimates based on quantitative population viability analyses are missing for most species.
As alternative to quantitative analysis (criterion E), simpler estimates of species range decline can be used in the IUCN framework, for example, to classify a species as “critically endangered” or “endangered” if it is predicted to lose ≥80% or ≥50% of its range, respectively, over the longer of 10 years or three generations (subcriteria A3 and A4). Criterion A was devised for observed population decline, but it is now also applied to SDM-derived estimates of future range size declines (Mace et al., Reference Mace, Collar, Gaston, Hilton-Taylor, Akçakaya, Leader-Williams, Milner-Gulland and Stuart2008; IUCN, 2022). However, it is important to note that while the IUCN allows using a future decline of range size (subcriteria A3 and A4) or an extinction probability estimate (criterion E) for classifying species into the same extinction risk category with well-funded arguments, this does not mean that a certain decline in range size can be translated into a specific quantitative extinction risk (Akçakaya et al., Reference Akçakaya, Butchart, Mace, Stuart and Hilton-Taylor2006; Mace et al., Reference Mace, Collar, Gaston, Hilton-Taylor, Akçakaya, Leader-Williams, Milner-Gulland and Stuart2008). Accordingly, the IUCN Red List guidelines state that “the risk-based thresholds of criterion E should not be used to infer an extinction risk for a taxon assessed […] under any of the criteria A to D″ (IUCN, 2022, p. 62). To illustrate this, a projected range loss of ≥80% may be used to classify a species as “critically endangered” (according to subcriteria A3 and A4), but this does not mean that its probability to go extinct within three generations is larger than 50% only because the latter would also be a valid criterion for being classified as “critically endangered” (according to criterion E). Yet such a use of SDM-based range changes paired with IUCN criteria for extinction risk assessment is also – misleadingly – stated in the latest IPCC report (IPCC, Reference Pörtner, Roberts, Tignor, Poloczanska, Mintenbeck, Alegría, Craig, Langsdorf, Löschke, Möller, Okem and Rama2022, p. 257), where central quantifications of extinction risks in IPCC AR6 are based on this approach (Warren et al., Reference Warren, Price, Graham, Forstenhaeusler and VanDerWal2018). Here, we want to echo Akçakaya et al. (Reference Akçakaya, Butchart, Mace, Stuart and Hilton-Taylor2006) and Mace et al. (Reference Mace, Collar, Gaston, Hilton-Taylor, Akçakaya, Leader-Williams, Milner-Gulland and Stuart2008) and caution against such interpretation.
When using SDMs to project extinctions, a better understanding of the relationship between range-size or abundance declines and extinction risk is thus central. Recent meta-analyses came to mixed conclusions on whether habitat suitability is a reasonable proxy of abundance (Weber et al., Reference Weber, Stevens, Diniz-Filho and Grelle2017; Lee-Yaw et al., Reference Lee-Yaw, McCune, Pironon and Sheth2022). This relationship of suitable habitat and extinction risk is likely dependent on species-specific characteristics (e.g., life-history strategy and generation times). Most importantly, the relationship between population size and habitat (suitable area) may not be linear (Blackburn et al., Reference Blackburn, Cassey and Gaston2006). In fact, SDMs only predict the potential distribution, while demographic and ecological processes and random events may prevent the species from occupying all suitable habitat (Figure 1). Here, the debate on whether SDMs capture fundamental or realised niches is relevant (e.g., Soberón, Reference Soberón2007; Holt, Reference Holt2009).
On a conceptual level, the process of extinction is very hard to quantify with any method. An alternative method to predict extinction under future climate change relies on SARs, that is, on strong empirical evidence for ubiquitous relationships between species richness and geographical area size (Matthews et al., Reference Matthews, Triantis and Whittaker2021). These SARs can be utilised to predict changes in species richness given projected changes in area, for example, in the geographic extent of a given habitat under climate change (Pimm et al., Reference Pimm, Russell, Gittleman and Brooks1995). However, this approach has been criticised because empirical SARs depend on species and environmental characteristics (Matias et al., Reference Matias, Gravel, Guilhaumon, Desjardins-Proulx, Loreau, Münkemüller and Mouquet2014; Schrader et al., Reference Schrader, König, Triantis, Trigas, Kreft and Weigelt2020), because extinction may often lag substantially behind habitat loss (Triantis et al., Reference Triantis, Borges, Ladle, Hortal, Cardoso, Gaspar, Dinis, Mendonça, Silveira, Gabriel, Melo, Santos, Amorim, Ribeiro, Serrano, Quartau and Whittaker2010), but most importantly because methods to construct a SAR are unable to adequately integrate the distribution of last individuals and to differentiate underlying sampling problems from the actual loss of these last individuals (He and Hubbell, Reference He and Hubbell2011, Reference He and Hubbell2013; Kitzes and Harte, Reference Kitzes and Harte2014). Further, the method is not species-specific but relies on defining relevant areas where habitat will be lost, as the amount of lost area is used to predict species richness (rather than extinction probability for individual species). SARs are thus better suited to deal with land use-related habitat loss rather than climate change-related extinction, and they cannot be used to inform about the risk of single species that would be relevant for conservation.
In summary, SDMs currently provide the most workable, species-specific prediction tool for threat classification under climate change, but should be used with caution. The translation of a decline in range size as projected by SDMs into quantitative extinction risk estimates (as by the IUCN criterion E) is fraught with difficulty, because the basic underlying extinction–range decline relationship is unclear, and likely to differ among taxa and environments. However, in the absence of more appropriate methods, careful application of IUCN criteria to SDM projections allows categorising species-level extinction risk from future climate change under specific assumptions. In the following two sections, we review conceptual and methodological challenges of SDMs that are particularly relevant to this process.
Conceptual challenges
Using SDMs for making predictions about future extinctions hinges on the expectation that these models make reliable predictions into the future. There are several reasons why this is not necessarily true. SDMs make several critical assumptions when applied to global change scenarios, most importantly that species are in equilibrium with current environment and will achieve (instantaneous) new equilibrium in the future, and that all environmental constraints are adequately understood and considered in the model (Elith and Leathwick, Reference Elith and Leathwick2009; Zurell et al., Reference Zurell, Franklin, König, Bouchet, Serra-Diaz, Dormann, Elith, Fandos Guzman, Feng, Guillera-Arroita, Guisan, Leitão, Lahoz-Monfort, Park, Peterson, Rapacciuolo, Schmatz, Schröder, Thuiller, Yates, Zimmermann and Merow2020). Here, we discuss why these critical assumptions are unlikely to be met in many cases. In addition, SDMs assume that species will conserve their niches into the future, for example, that no change in the species–environment relationship will occur through adaptive evolution of thermal tolerance, which is probably unlikely given strong selective pressure (Dawson et al., Reference Dawson, Jackson, House, Prentice and Mace2011; Buckley and Kingsolver, Reference Buckley and Kingsolver2012).
There is increasing evidence that range-shifting species are lagging behind their climatically suitable habitat (Svenning et al., Reference Svenning, Normand and Skov2008), leading to suitable habitat not yet colonised (“colonisation credit”) and indicating departure from the equilibrium assumption underlying SDMs. Impacts from other global change drivers such as habitat destruction and land fragmentation can prevent a species from tracking suitable climate, leading to a much higher extinction risk than can be suggested by an SDM (Travis, Reference Travis2003; Hof et al., Reference Hof, Levinsky, Araújo and Rahbek2011). The dispersal ability of a species will (co-)determine the climate tracking ability, yet reliable empirical estimates are largely missing (Bullock et al., Reference Bullock, Mallada González, Tamme, Götzenberger, White, Pärtel and Hooftman2017; Fandos et al., Reference Fandos, Talluto, Fiedler, Robinson, Thorup and Zurell2023). When comparing potential current and potential future distribution, SDMs often assume full dispersal or no dispersal (Thuiller et al., Reference Thuiller, Guéguen, Renaud, Karger and Zimmermann2019). In the first case, we assume that species fully track the changing climate in space. In the second case, we assume that species are not at all shifting their range but simply lose currently suitable climate area. Inference of range size declines can dramatically differ between these extreme assumptions and are also strongly influenced by the geography of the study area (Figure 3). Additionally, climate tracking and range shifting can be affected by demographic processes, adaptive evolution and species interactions (Buckley and Kingsolver, Reference Buckley and Kingsolver2012; Svenning et al., Reference Svenning, Gravel, Holt, Schurr, Thuiller, Münkemüller, Schiffers, Dullinger, Edwards, Hickler, Higgins, Nabel, Pagel and Normand2014; IPBES, Reference Ferrier, Ninan, Leadley, Alkamade, Acosta, Akçakaya, Brotons, Cheung, Christensen, Harhash, Kabubo-Mariara, Lundquist, Obersteiner, Pereira, Peterson, Pichs-Madruga, Ravindranath, Rondinini and Wintle2016; Schleuning et al., Reference Schleuning, Neuschulz, Albrecht, Bender, Bowler, Dehling, Fritz, Hof, Mueller, Nowak, Sorensen, Böhning-Gaese and Kissling2020). For example, the presence of competitors, generalist consumers or predators can slow down range expansion (Davis et al., Reference Davis, Jenkinson, Lawton, Shorrocks and Wood1998). At the same time, long life expectancy of species can result in (temporary) survival under unfavourable conditions and delayed local extirpations (extinction debts; Kuussaari et al., Reference Kuussaari, Bommarco, Heikkinen, Helm, Krauss, Lindborg, Öckinger, Pärtel, Pino, Rodà, Stefanescu, Teder, Zobel and Steffan-Dewenter2009). To some extent, these violations of the equilibrium assumption can be captured by the two extremes of full versus no dispersal scenarios. Yet it is highly uncertain towards which of these extreme assumptions a specific species will lean. Therefore, the IUCN (2022) recommends to derive and overlap future SDM predictions at one-generation intervals to assess climate-tracking potential. Ideally, this should be coupled with reasonable assumptions about potential spread of species in the face of the above-mentioned processes.
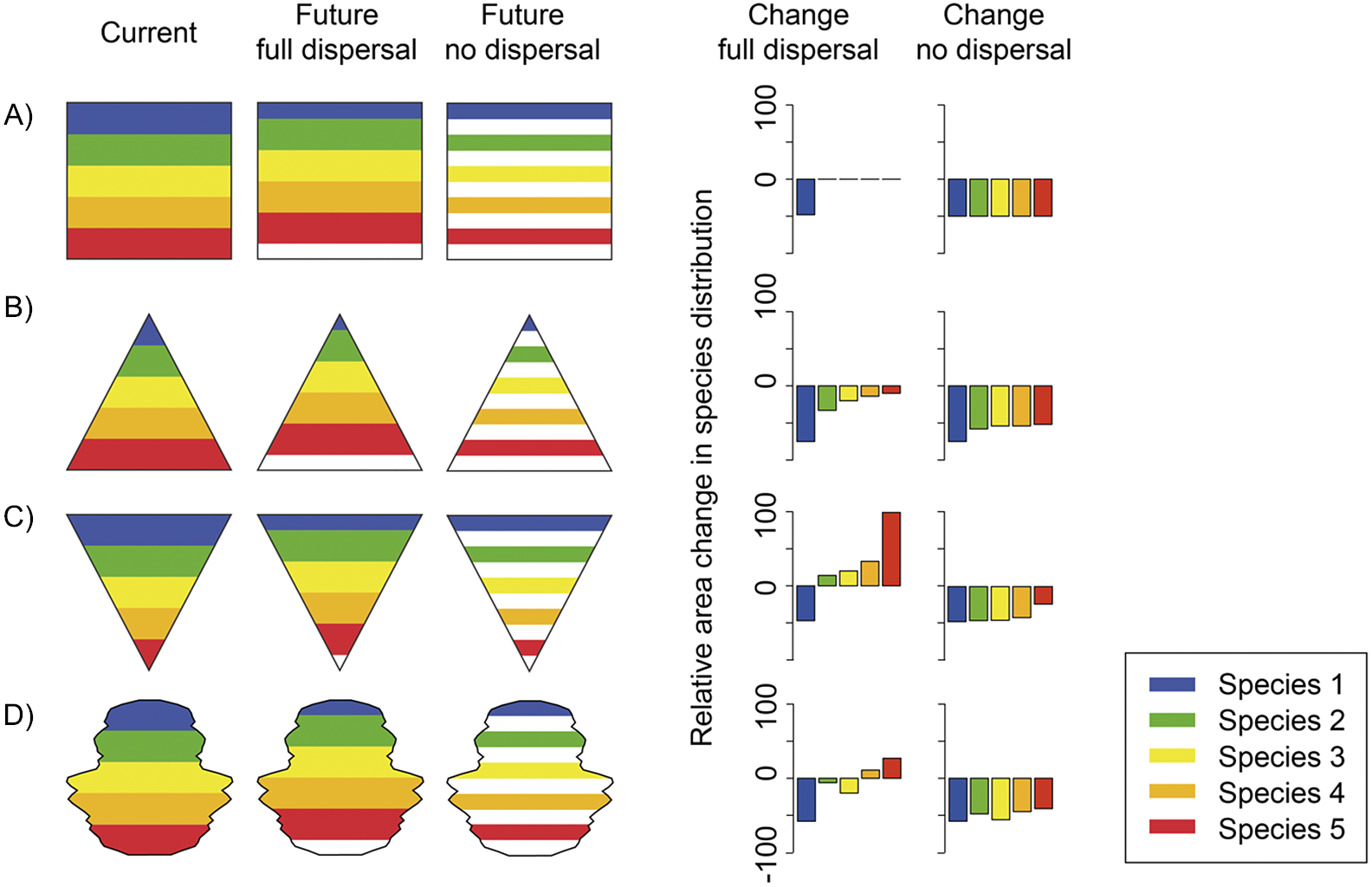
Figure 3. Shape of the study area as well as dispersal assumptions influence predictions of correlative species distribution models (SDMs). This is shown here for theoretical continents characterised solely by a linear gradual decrease of temperature to the upper part of the study area. We assume that each temperature band is occupied by one hypothetical species. In the future, temperature isoclines will move upwards on the shown study areas (imitating global warming; sketch maps on the left). Under the full-dispersal assumption, species will fully track their suitable temperature band. Under the no-dispersal scenario, species will lose climatically suitable area but will not shift their range. These two extremes reflect the most common dispersal assumptions in SDM-based projections under climate change. Extinction risk estimates derived from SDMs strongly depend on the geographical shape of the study area, and the dispersal assumption (bar charts on the right showing relative area change for each species). Fun fact: the continent map in (D) is a rough representation of the area–latitude relationship of western Europe.
It is almost impossible to incorporate all relevant environmental constraints into SDMs or project these into the future, even though this is a core assumption underlying SDM methodology. Ecological processes are highly scale-dependent. Climatic conditions may govern the broad-scale species distribution, while the fine-scale distribution may be determined by local resources (Guisan and Thuiller, Reference Guisan and Thuiller2005) or microclimate (Suggitt et al., Reference Suggitt, Gillingham, Hill, Huntley, Kunin, Roy and Thomas2011). Consequently, climate niche tracking and range shifting may ultimately be limited by fine-scale resource distributions (Skov and Svenning, Reference Skov and Svenning2004; Dormann, Reference Dormann2007; Suggitt et al., Reference Suggitt, Wilson, Isaac, Beale, Auffret, August, Bennie, Crick, Duffield, Fox, Hopkins, Macgregor, Morecroft, Walker and Maclean2018). Particularly among plants, facilitative effects of other species may strongly influence habitat suitability under unfavourable conditions (like nurse plants in alpine or dry environments; Steinbauer et al., Reference Steinbauer, Beierkuhnlein, Arfin Khan, DE, Irl, Jentsch, Schweiger, Svenning and Dengler2016; Gallien et al., Reference Gallien, Zurell and Zimmermann2018). Not considering these fine-scale environmental or biotic predictors in SDMs may bias predictions, and lead to under- or over-estimation of suitable habitat. Additionally, predictions into the future require availability of environmental scenarios. Climate models are well advanced, and the climate science community produces regular updates on climate scenarios for the IPCC (Knutti et al., Reference Knutti, Masson and Gettelman2013). For land use, which is another major determinant of species distribution, future scenarios are less well developed and more uncertain due to unknown political and economic development (Cabral et al., Reference Cabral, Mendoza-Ponce, da Silva, Oberpriller, Mimet, Kieslinger, Berger, Blechschmidt, Brönner, Classen, Fallert, Hartig, Hof, Hoffmann, Knoke, Krause, Lewerentz, Pohle, Raeder, Rammig, Redlich, Rubanschi, Stetter, Weisser, Vedder, Verburg and Zurell2022).
A further challenge arising with future predictions is unidentified constraints in species distributions. Environmental or land use factors that constrain the distribution of a species can only become apparent as effective predictors in SDMs if they limit the current distribution of a species. If, for instance, soil characteristics are largely suitable within the current range of a species that is currently constrained by climatic factors, an SDM will not identify soil as a relevant predictor variable and will be unable to identify that a projected future range may be largely uninhabitable due to unsuitable soil conditions. Particularly, edaphic variation is a major determinant of plant distributions (Hulshof and Spasojevic, Reference Hulshof and Spasojevic2020) but is often neglected in SDMs, possibly because soil characteristics on macroscales correlate with climate (e.g., along latitude; Huston, Reference Huston2012). In fact, the identification of relevant variables only based on explanatory power may be very misleading, as random spatial variables may be able to predict spatial distribution pattern as well as commonly used environmental predictors (Fourcade et al., Reference Fourcade, Besnard and Secondi2018). As SDMs are phenomenological models, it is important not to mistake correlation for causation (Dormann et al., Reference Dormann, Schymanski, Cabral, Chuine, Graham, Hartig, Kearney, Morin, Römermann, Schröder and Singer2012). Also, phenomenological relationships might not hold in the future if species adapt to novel abiotic and biotic conditions.
Methodological challenges
When using models to estimate extinction risks and to inform management and provide policy support, it is important that models are fit for this particular purpose. This may be challenged by conceptual problems, as discussed above, and by methodological challenges, for which we give a brief overview here. Although SDMs are commonly perceived as a simple method, only few studies achieve quality standards that will match the standards specified by the IUCN for extinction risk assessment (IUCN, 2022). Several recent papers provide guidance and propose best-practice standards for ensuring SDM credibility for decision-making and biodiversity assessment (Araújo et al., Reference Araújo, Anderson, Barbosa, Beale, Dormann, Early, Garcia, Guisan, Maiorano, Naimi, O’Hara, Zimmermann and Rahbek2019; Sofaer et al., Reference Sofaer, Jarnevich, Pearse, Smyth, Auer, Cook, Edwards, Guala, Howard, Morisette and Hamilton2019). Nogués-Bravo (Reference Nogués-Bravo2009) discussed how SDMs can be used to predict past distributions of species’ climate niches and derived a set of recommended practices for hindcasting, arguing that inadequate methods can lead to “a cascade of errors and naïve ecological and evolutionary inferences” (Nogués-Bravo, Reference Nogués-Bravo2009). Although focused on hindcasts, the identified methodological challenges also apply to forecasting SDMs as a basis for estimating extinction risk. We summarise these below as (1) model specification, (2) selection of environmental predictors, (3) model validation and (4) uncertainty through non-analogue climates (Barry and Elith, Reference Barry and Elith2006; Nogués-Bravo, Reference Nogués-Bravo2009; IUCN, 2022; Figure 4A).
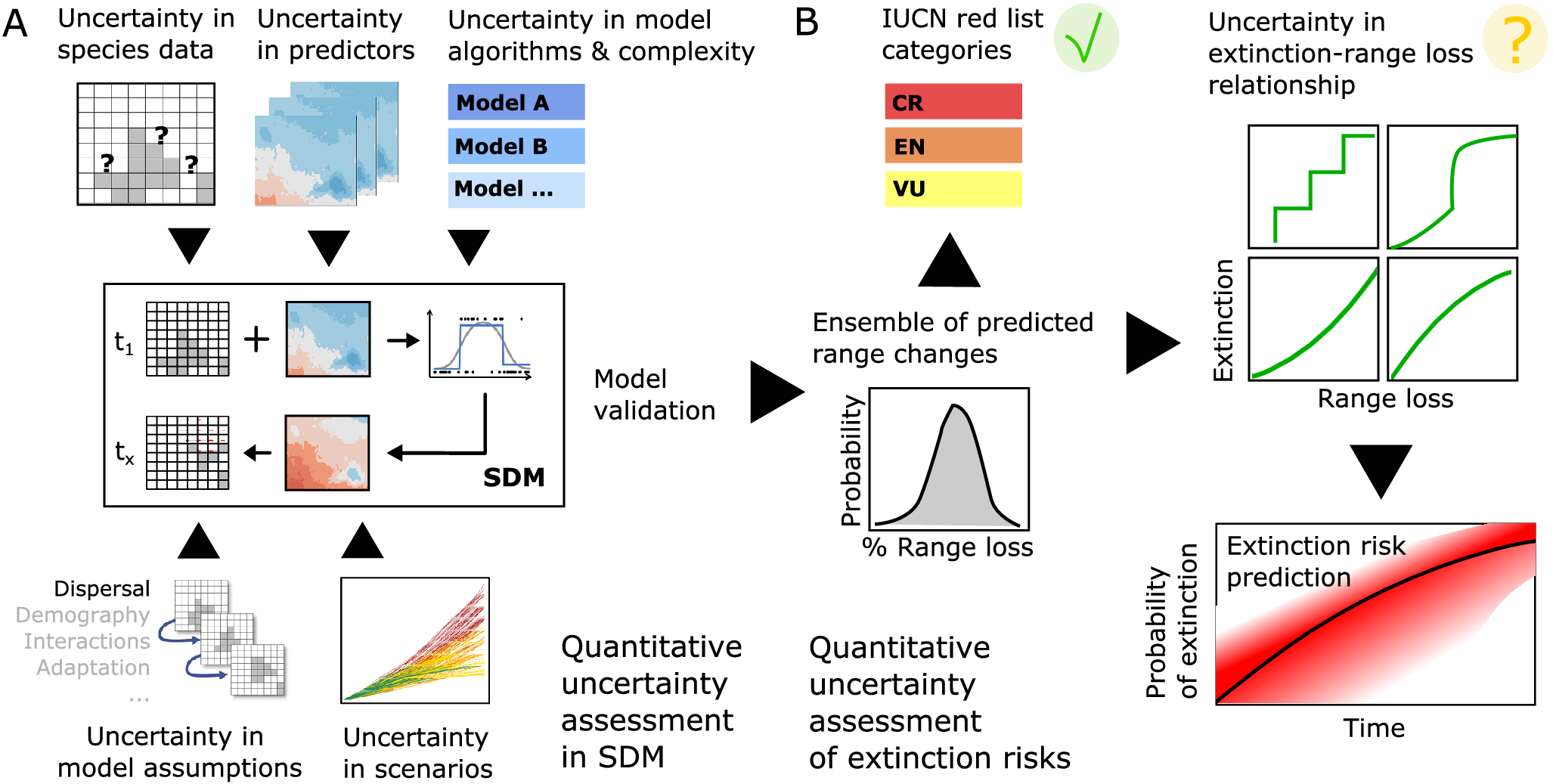
Figure 4. Workflow and challenges for deriving adequate range loss predictions from correlative species distribution models (SDMs) and subsequent estimates of extinction risk. (A) Several methodological and conceptual challenges should be considered in SDM development, and resulting uncertainty should be adequately communicated. Current best practices for achieving or assessing model credibility are summarised in Araújo et al. (Reference Araújo, Anderson, Barbosa, Beale, Dormann, Early, Garcia, Guisan, Maiorano, Naimi, O’Hara, Zimmermann and Rahbek2019) and Sofaer et al. (Reference Sofaer, Jarnevich, Pearse, Smyth, Auer, Cook, Edwards, Guala, Howard, Morisette and Hamilton2019). (B) While predicted range loss can be readily translated into IUCN Red List categories for threatened species following the IUCN Red List guidelines (IUCN, 2022), the IUCN advices against deriving quantitative extinction risk estimates from SDM predictions. At the very least, further research is required regarding adequate extinction–range loss relationships and adequate uncertainty propagation (IUCN Red List categories: CR, critically endangered; EN, endangered; VU, vulnerable).
(1) Several studies have shown that algorithmic choices can strongly affect current and future range predictions (Buisson et al., Reference Buisson, Thuiller, Casajus, Lek and Grenouillet2010; Thuiller et al., Reference Thuiller, Guéguen, Renaud, Karger and Zimmermann2019). Algorithms range from simple profile or envelope methods, regression-based approaches to complex machine-learning methods (Guisan et al., Reference Guisan, Thuiller and Zimmermann2017). Machine-learning methods derive complex species–environment relationships that closely fit the observed data and have often been reported to achieve highest prediction accuracy (Elith et al., Reference Elith, Graham, Anderson, Dudik, Ferrier, Guisan, Hijmans, Huettmann, Leathwick, Lehmann, Li, Lohmann, Loiselle, Manion, Moritz, Nakamura, Nakazawa, Overten, Peterson, Phillips, Richardson, Scachetto-Pereira, Schapire, Soberon, Williams, Wisz and Zimmermann2006; Valavi et al., Reference Valavi, Guillera-Arroita, Lahoz-Monfort and Elith2021). Yet overfitting might also lead to reduced transferability to new times and places, and simpler models might thus be preferable for predicting future species ranges and extinction risk (Merow et al., Reference Merow, Smith, Edwards, Guisan, McMahon, Normand, Thuiller, Wüest, Zimmermann and Elith2014; Brun et al., Reference Brun, Thuiller, Chauvier, Pellissier, Wüest, Wang and Zimmermann2020). For making biodiversity predictions under scenarios of climate change, the IUCN advises to use at least three different SDM algorithms of intermediate complexity to capture the uncertainty related to species–environment relationships (IUCN, 2022). (2) Similarly, the number of predictor variables included in a model should be kept reasonably small when predicting into the future (Brun et al., Reference Brun, Thuiller, Chauvier, Pellissier, Wüest, Wang and Zimmermann2020). Also, uncertainty in available environmental data should be considered, for example, when alternative data sources are available. As SDMs are increasingly used in global change research, the question of transferability also becomes more urgent (Yates et al., Reference Yates, Bouchet, Caley, Mengersen, Randin, Parnell, Fielding, Bamford, Ban, Barbosa, Dormann, Elith, Embling, Ervin, Fisher, Gould, Graf, Gregr, Halpin, Heikkinen, Heinänen, Jones, Krishnakumar, Lauria, Lozano-Montes, Mannocci, Mellin, Mesgaran, Moreno-Amat, Mormede, Nowaczek, Oppel, Crespo, Peterson, Rappaciuolo, Roberts, Ross, Scales, Schoeman, Snelgrove, Sundblad, Thuiller, Torres, Verbruggen, Wang, Wenger, Whittingham, Zharikov, Zurell and Sequeira2018). (3) Due to a lack of independent test data, validation is typically done based on data partitioning (Araújo et al., Reference Araújo, Pearson, Thuiller and Erhard2005). Newest developments in model validation now advocate for spatial or environmental block cross-validation approaches that strategically hold out data that are spatially or environmentally clustered, and by this force extrapolation during validation (Bagchi et al., Reference Bagchi, Crosby, Huntley, Hole, Butchart, Collingham, Kalra, Rajkumar, Rahmani, Pandey, Gurung, Trai, Quang and Willis2013; Roberts et al., Reference Roberts, Bahn, Ciuti, Boyce, Elith, Guillera-Arroita, Haubenstein, Lahoz-Monfort, Schröder, Thuiller, Warton, Wintle, Hartig and Dormann2017; Valavi et al., Reference Valavi, Elith, Lahoz-Monfort and Guillera-Arroita2019). Although an important step forward for assessing prediction uncertainty, we are still missing clear guidance about adequate block design to ensure robust estimates of prediction accuracy of future ranges. Validation of prediction accuracy into the future is further complicated by the fact that global change could lead to non-stationarity in the processes that govern the inferred species–environmental relationship (Rollinson et al., Reference Rollinson, Finley, Alexander, Banerjee, Hamil, Koenig, Locke, DeMarche, Tingley, Wheeler, Youngflesh and Zipkin2021), potentially violating the assumption of niche constancy. (4) Lastly, when making predictions to the future, we also need to consider uncertainty through extrapolating to novel environments. Different algorithms will exhibit different extrapolation behaviour and it is thus advisable to explicitly assess environmental novelty (Elith et al., Reference Elith, Kearney and Phillips2010; Zurell et al., Reference Zurell, Elith and Schröder2012). The IUCN (2022) lists several of these methodological issues that need to be considered and communicated to include SDM results and predictions in Red List assessments, and we highly recommend consulting these guidelines when planning studies estimating extinction risk (Figure 4A). Best practices for achieving or assessing model credibility are also summarised in Araújo et al. (Reference Araújo, Anderson, Barbosa, Beale, Dormann, Early, Garcia, Guisan, Maiorano, Naimi, O’Hara, Zimmermann and Rahbek2019) and Sofaer et al. (Reference Sofaer, Jarnevich, Pearse, Smyth, Auer, Cook, Edwards, Guala, Howard, Morisette and Hamilton2019).
Moving forward: Predicting uncertainty is better than wrong predictions
While the outlined conceptual and methodological challenges illustrate why using SDMs for predicting extinction risk is potentially problematic, SDMs are still the most widely applicable tool currently available for predicting species’ potential future distributions under climate change. Our survey of SDM-related challenges also highlights crucial future research questions that need to be addressed to improve the use of SDMs for predictions of extinction risk from climate change (Figure 4B). In particular, improved understanding of the relationship between species’ extinction probability and (SDM-derived) range decline is of central importance for more robust predictions. Particularly, the non-random distribution and extinction probability of species that have declined to very small population sizes are not well understood and pose the largest uncertainty when estimating extinction probabilities. In the absence of clearly identifiable climate-related extinctions over the last centuries, science cannot build on empirical evidence when assessing climate change-related extinction risk, at least not in the recent past. In fact, until now, only two global species extinctions in modern times can be attributed with confidence to human-induced climate change (IPCC, Reference Pörtner, Roberts, Tignor, Poloczanska, Mintenbeck, Alegría, Craig, Langsdorf, Löschke, Möller, Okem and Rama2022, p. 237). This absence of observed climate-related extinctions does not contrast with model predictions, but it limits our current understanding of extinction processes and constraints testing the precision of predictive models (Brook et al., Reference Brook, Sodhi and Bradshaw2008). The most promising ways forward for a better understanding of the extinction–range loss relationship may thus be the investigation of local extirpation patterns, of spatially explicit simulations, and, above all, of the rich information on past biotic responses to climate changes provided by the fossil record (Calosi et al., Reference Calosi, Putnam, Twitchett and Vermandele2019; Fordham et al., Reference Fordham, Jackson, Brown, Huntley, Brook, Dahl-Jensen, Gilbert, Otto-Bliesner, Svensson, Theodoridis, Wilmshurst, Buettel, Canteri, McDowell, Orlando, Pilowsky, Rahbek and Nogues-Bravo2020).
A second area of future research relates back to critical methodological issues of SDMs. In many studies, species with very small range size (or a low number of occurrences) are excluded, because SDMs need a certain number of data points, yet these highly endemic species are among the most relevant for conservation (Lomba et al., Reference Lomba, Pellissier, Randin, Vincente, Moreira, Honrado and Guisan2010; Breiner et al., Reference Breiner, Nobis, Bergamini and Guisan2018). As rarity could have several reasons and relate, for example, to a narrow niche or to climatic rarity (Ohlemüller, Reference Ohlemüller2011), the extinction risk–range loss relationship might even be different for species that have evolved to occur in small areas or at low populations size compared with species that are forced to do so. Also, little consensus exists yet regarding adequate assumptions for considering potential future spread in SDM predictions. Many researchers have called for integrating more process detail into distribution models to account for relevant transient dynamics under global change (Figure 1; IPBES, Reference Ferrier, Ninan, Leadley, Alkamade, Acosta, Akçakaya, Brotons, Cheung, Christensen, Harhash, Kabubo-Mariara, Lundquist, Obersteiner, Pereira, Peterson, Pichs-Madruga, Ravindranath, Rondinini and Wintle2016). Yet, until such process-based models are available for large numbers of species, an intermediate solution could be to agree on standards for incorporating reasonable assumptions about species spread (mediated by dispersal, demography, and species interactions, among other processes) into predictions of range changes.
In the face of the climate and biodiversity crises, there is a clear demand of future extinction risk estimates (IPCC, Reference Pörtner, Roberts, Tignor, Poloczanska, Mintenbeck, Alegría, Craig, Langsdorf, Löschke, Möller, Okem and Rama2022). Thus, while advancing on central research questions related to improve our fundamental understanding of extinction processes, we advocate that well-conducted SDMs should initially fill the knowledge gap and make predictions on extinction risk – but only when following good practice and when openly communicating the limitations and uncertainty (Araújo et al., Reference Araújo, Anderson, Barbosa, Beale, Dormann, Early, Garcia, Guisan, Maiorano, Naimi, O’Hara, Zimmermann and Rahbek2019; Feng et al., Reference Feng, Park, Walker, Peterson, Merow and Papeş2019; Zurell et al., Reference Zurell, Franklin, König, Bouchet, Serra-Diaz, Dormann, Elith, Fandos Guzman, Feng, Guillera-Arroita, Guisan, Leitão, Lahoz-Monfort, Park, Peterson, Rapacciuolo, Schmatz, Schröder, Thuiller, Yates, Zimmermann and Merow2020; IUCN, 2022). Particularly, SDMs used for estimating future extinction risk must be used with caution and constructed with care, and the application of IUCN Red List criteria to SDM results must follow the published guidelines. According to these, predicted range size declines from well-constructed SDMs can readily be used to classify a species as threatened from climate change. In contrast, it is seen as problematic to back-infer quantitative extinction risks from this classification, yet this is currently being done in policy-relevant reports such as IPCC (Reference Pörtner, Roberts, Tignor, Poloczanska, Mintenbeck, Alegría, Craig, Langsdorf, Löschke, Möller, Okem and Rama2022). We thus suggest as middle ground that the uncertainties associated with translating range declines into quantitative extinctions risks should be more adequately communicated while at the same time increasing research efforts to better understand the extinction risk–range decline relationship (Figure 4). In addition, we need to acknowledge that SDMs will only provide predictions of suitable area while ignoring other relevant processes affecting range shifts and extinction. It is thus important that we also assess uncertainty in model predictions induced by alternative assumptions about the climate-tracking potential of species, for example, through phenotypic plasticity, local genetic adaptation or variability in dispersal. We follow recent publications in arguing that the SDM community is largely aware of these issues and has developed improved standards (Araújo et al., Reference Araújo, Anderson, Barbosa, Beale, Dormann, Early, Garcia, Guisan, Maiorano, Naimi, O’Hara, Zimmermann and Rahbek2019; Feng et al., Reference Feng, Park, Walker, Peterson, Merow and Papeş2019; Sofaer et al., Reference Sofaer, Jarnevich, Pearse, Smyth, Auer, Cook, Edwards, Guala, Howard, Morisette and Hamilton2019; Zurell et al., Reference Zurell, Franklin, König, Bouchet, Serra-Diaz, Dormann, Elith, Fandos Guzman, Feng, Guillera-Arroita, Guisan, Leitão, Lahoz-Monfort, Park, Peterson, Rapacciuolo, Schmatz, Schröder, Thuiller, Yates, Zimmermann and Merow2020) that will allow future studies to address methodological issues and handle conceptual issues carefully.
Open peer review
To view the open peer review materials for this article, please visit http://doi.org/10.1017/ext.2023.5.
Supplementary materials
To view supplementary material for this article, please visit http://doi.org/10.1017/ext.2023.5.
Data availability statement
The review table of the 300 randomly selected papers is available in the Supplementary Material.
Author contributions
Conceptualisation (equal): D.Z., S.A.F., M.J.S.; Investigation (equal): A.R.; Investigation (lead): D.Z.; Methodology (equal): D.Z., S.A.F., M.J.S.; Methodology (support): A.R.; Supervision (lead): D.Z.; Visualisation (equal): M.J.S.; Visualisation (lead): D.Z.; Writing – original draft preparation (equal): S.A.F., M.J.S.; Writing – original draft preparation (lead): D.Z.; Writing – review and editing (equal): D.Z., S.A.F., M.J.S.; Writing – review and editing (support): A.R.
Financial support
The work was supported by the Deutsche Forschungsgemeinschaft DFG (D.Z., grant ZU 361/1-1; M.J.S., grant STE 2360/2-1 embedded in the Research Unit TERSANE FOR 2332), the Leibniz Association (S.A.F., grant number Leibniz Competition P52/2017) and the ERC H2020 research and innovation programme (M.J.S., project HOPE grant 741413).
Competing interest
The authors declare none.
Comments
Dear Editors,
We are grateful for the opportunity to contribute to the launching of this new journal and like you to consider our manuscript "Predicting extinctions with species distribution models" for consideration as review article. In it, we review the literature for the use (and misuse) of correlative species distribution models for predicting future extinction risk under climate change, provide a critical appraisal of the conceptual and methodological challenges, and detail why these models are still among the best workable approaches we have available.
We hope that this review will provide a broad overview on relevant literature to to beginners in the field, but will also spark critical thoughts among the more proficient modellers and users and inspire new research avenues.
The work is not submitted or under consideration elsewhere and all authors agree with the contents and declare no conflict of interest.
We are looking forward to your assessment.
On behalf of all authors, kind regards,
Damaris Zurell