1. Introduction
Institutional economists are increasingly interested in social constructs such as civic norms. Civic norms are typically defined as rules of behaviour that regulate social dilemma games and help a group to overcome free-rider problems (Guiso et al. Reference Guiso, Sapienza, Zingales, Benhabib, Bisin and Jackson2011). According to Knack and Keefer (Reference Knack and Keefer1997: 1254), civic norms impose ‘constraints on narrow self-interest, leading individuals to contribute to the provision of public goods of various kinds’. Examples of civic norms are for instance norms against tax avoidance, claiming government benefits to which one is not entitled, or littering. To measure civic norm compliance, institutional economists largely draw on social survey data sets (e.g. Knack and Keefer Reference Knack and Keefer1997; Letki Reference Letki2006; Owen and Videras Reference Owen and Videras2006). They extrapolate items that capture normative beliefs regarding the justification of certain pro-social behaviour, and take such beliefs as an indication of norm compliance.
In social philosophy, the nature and function of norms, as well as the mechanisms of norm-driven behaviour, are central topics on the field’s research agenda. In this context, social norms are defined as implicit rules of behaviour that regulate strategic interactions. They are represented via game-theoretic structures and standard examples are fairness, reciprocity or promise-keeping. To measure norm compliance, social philosophers design laboratory and field experiments where they typically elicit two kinds of expectations, the so-called empirical and normative expectations (Bicchieri Reference Bicchieri2006). Empirical expectations refer to the beliefs an individual has regarding others’ behaviour in a certain situation – whether others follow the norm in the appropriate circumstance. Normative expectations refer to the beliefs an individual has regarding what others think that he/she ought to do in that situation. Within this framework, both types of expectations are essential and distinctive properties to infer the existence of a social norm in a group (Bicchieri Reference Bicchieri2006).
As this brief overview already shows, civic and social norms exhibit important similarities: they both refer to social dilemmas and prescribe how to deal with them in a group. However, it is also evident that the operationalization for measurement purposes differs: institutional economists operationalize civic norms as normative beliefs; social philosophers take normative and empirical expectations as key properties for norm compliance. In light of the analogy in the definition of civic and social norms, the differences in their operationalization are surprising and call for an explanation. Footnote 1
The aim of this paper is to combine insights from institutional economics and social philosophy, in order to develop a novel approach to measure civic norm compliance. A central element is the theoretical distinction between empirical and normative expectations (Bicchieri Reference Bicchieri2006). We show that current measures of civic norm compliance in institutional economics benefit from incorporating empirical expectations as a key dimension of the construct.
Following the institutional economics literature, we use data from the European Values Study to explore the role of empirical and normative expectations for civic norm compliance. The European Values Study covers approximately 160,000 individuals from almost 40 countries in the period 1981–2008. The main results show that by focusing only on beliefs about justification, institutional economics has provided an equivocal measure of civic norms, whose outcome significantly changes when incorporating empirical expectations. To this aim, we first conduct a factor analysis at the individual level, where we find that both normative beliefs and empirical expectations measure key dimensions of civic norms. After that, we illustrate their economic consequences by examining their effect on trust – a well-known case in the institutional economic literature. We find, in contrast to results from institutional economics (Knack and Keefer Reference Knack and Keefer1997; Tabellini Reference Tabellini2008, Reference Tabellini2010; Aghion et al. Reference Aghion, Algan, Cahuc and Shleifer2010), that the relationship between trust and civic norms is largely driven by the existence of empirical expectations.
Our findings contribute to the existing literature in two main interrelated ways. First, our results illustrate how the field of institutional economics can benefit from a more solid theoretical foundation to measure civic norm compliance. In particular, the results show that the incorporation of empirical expectations is a viable measurement strategy to account for the inter-subjectivity of norms. This aspect of civic norm compliance has largely been overlooked in the empirical institutional economic literature (Leung and Morris Reference Leung and Morris2015), but not in economics in general. For example, the notion of multiplier effects (Glaeser et al. Reference Glaeser, Scheinkman and Sacerdote2003; Cutler and Glaeser Reference Cutler, Glaeser and Wise2010), as well as the notion of social network effects (Videras et al. Reference Videras, Owen, Conover and Wu2012), illustrate the importance of the social context for behaviour (Manski Reference Manski2000). As of yet, however, the role of empirical expectations has largely been neglected in institutional economics. By building upon a solid theoretical framework, this paper effectively illustrates how to incorporate beliefs about others’ behaviour. Promising extensions of this work lie in the application of the theoretical framework to other norms that qualify as social norms, such as generosity or solidarity (Beine et al. Reference Beine, Docquier and Schiff2013; Burda et al. Reference Burda, Hamermesh and Weil2013; Helliwell et al. Reference Helliwell, Wang and Xu2016), as well as to other economic research that engages with the social context.
Second, studies on norms in social philosophy mainly consist of theoretical analyses and experimental studies, whereas institutional economists often turn to social survey data (such as the European Social Survey (ESS), the European Values Study (EVS) and the World Values Survey (WVS)). The current study illustrates that in social philosophy too survey data have the potential to yield interesting and novel insights. Our approach complements experimental studies in two main ways. First, in comparison to laboratory experiments, social survey data allow us to access an impressive data set for a large number of countries, this way supporting extensions to cross-cultural studies. Moreover, opportunities to gain insights in, for example, norm change exist within reach when using panel data. It can be easily shown, for example, how expectations have changed over the years across generations. This result can be taken as a first indication of norm change, which can then be further explored in combination with other methods of empirical analysis.
The remainder of this paper is structured as follows. Section 2 looks more closely at the accounts of norms in social philosophy and institutional economics. Section 3 introduces the data and methodology. Section 4 lays out the measurement results and section 5 evaluates the robustness of the results. Section 6 concludes by discussing the implications of results and directions for future conceptual and empirical work on civic norm measurement.
2. Norms in social philosophy and institutional economics
2.1. Norms in social philosophy
One of the most influential accounts of social norms in the philosophical literature is that of Bicchieri (Reference Bicchieri2006). Bicchieri’s analysis of social norms is developed in a rational choice framework and, as such, it shares the same underlying approach with institutional economics (see sections 2.2 and 2.3).
According to Bicchieri, social norms transform mixed-motive games into coordination games, this way providing incentives for individuals to contribute to the common good. To see how this works, consider a standard prisoner’s dilemma game. When there are no social norms prescribing to contribute to a common good, the incentives of the game are such that rational players prefer to defect from cooperation. However, when social norms are present, the payoffs of the game change: the incentives to defect decrease by a factor that measures the psychological (or material) cost of deviation. This cost arises when a player expects that others cooperate and thinks that others expect her to cooperate. As it was briefly mentioned before, these expectations are called empirical and normative expectations.
More formally, a behavioural rule is a social norm when the following three conditions hold for individuals that are part of a community or group:
(1) An individual knows that a rule exists and applies to a particular type of situation (behavioural condition);
(2) An individual prefers to conform to the rule if:
(a) she expects that others will conform to the rule in situations of the specified type (empirical expectations (EE));
(b) she believes that others expect her to conform to the rule in situations of the specified type. This condition may or may not involve punishment (normative expectations (NE)).
Only when a sufficiently large part of the community – a subset – adheres to these three conditions, it can be inferred that a social norm exists in that community.
The first condition entails that an individual knows that the rule applies to a certain context. This condition implies that for a behavioural rule (for example that you should not litter in public spaces) one should know that the rule prescribes you to act in a certain way when you find yourself in such a situation (e.g. to throw your rubbish in a bin when you find yourself in a public space). Condition (2a) specifies that an individual expects that others will conform to the norm in similar situations, e.g. that others will throw their rubbish in a bin when they find themselves in a public space. This expectation may arise through inference: when observing that others do not litter, it is plausible to assume that their behaviour remains constant over time and that they will therefore not litter in the future. Footnote 2,Footnote 3 The third and final condition (2b) refers to the belief that an individual has about others expecting him/her to conform to the norm, e.g. that others expect you to put your rubbish in a bin when you are in a public space. For some people this belief is motivated by observing that a form of punishment, such as shame or social ostracism, is enforced. Normative expectations have a non-social counterpart that specifies ‘personal beliefs about what should be done’, so-called normative beliefs (Bicchieri et al. Reference Bicchieri, Lindemans and Jiang2014: 3).
We highlight an additional feature of Bicchieri’s model. As Bicchieri shows, normative and empirical expectations motivate norm conformity. When individuals are asked why they followed a certain norm, they typically report both kinds of expectations. However, there may be cases where normative and empirical expectations are not both simultaneously present for the same individual. For example, consider a group where recycling is not a common practice. In this group, there may be an individual who does not have empirical expectations (she thinks others typically do not recycle) but may hold normative expectations (she thinks that others believe that she ought to recycle). Vice versa, an individual may hold empirical expectations (she thinks others typically do recycle) but lack normative expectations (she thinks that others believe that she ought not to recycle). This theoretical distinction is examined in an experimental study, in which Bicchieri and Xiao (Reference Bicchieri and Xiao2009) analyse the relative importance of empirical and normative expectations for pro-social behaviour. In particular, the study shows that empirical expectations tend to overrule normative expectations when the former are present but the latter are not. In other words, it is more likely that someone will follow the norm when only empirical expectations are present than when only normative expectations are present. An intuitive explanation for this is that, given that social norms prescribe to contribute to a common good, if an individual believes that others do not contribute to a common good but believes that others expect her to do so, she might decide to defect as she realizes she would not benefit from the advantages that derive from mutual cooperation.
2.2. Norms in economics
In the institutional economics literature, we encounter the notion of civic norms. Civic norms have been defined in a variety of ways. As was briefly mentioned in the introduction, Knack and Keefer (Reference Knack and Keefer1997) define civic norms as constraints on narrow self-interest that give people incentives to contribute to the public good. Guiso et al. (Reference Guiso, Sapienza, Zingales, Benhabib, Bisin and Jackson2011: 3) define civic norms as ‘those persistent and shared beliefs and values that help a group overcome the free-rider problem in the pursuit of socially valuable activities’ (see also Nannicini et al. Reference Nannicini, Stella, Tabellini and Troiano2013). Related views are found in the work of Owen and Videras (Reference Owen and Videras2006: 814), who state that civic norms arise when ‘people cooperate and contribute to public goods more than is expected given their incentives to free-ride’. Other studies refer to civic virtues or civic morality, which are informed by ethical theories such as virtue ethics (Letki Reference Letki2006). These accounts stress the habit of forming civic virtues such as trust, honesty and reciprocity. It is assumed that such virtues generate a sense of responsibility for the public good that fosters cooperation and deters people from corruption and free-riding. Other, more general, definitions describe civic norm compliance as acts in favour of the community (Aghion et al. Reference Aghion, Algan, Cahuc and Shleifer2010) or as an individual’s perception of how citizens should behave in relation to the state (Kotzian Reference Kotzian2014).
Oftentimes, civic norms are related to social capital (Letki Reference Letki2006; Guiso et al. Reference Guiso, Sapienza, Zingales, Benhabib, Bisin and Jackson2011; Kotzian Reference Kotzian2014): a phenomenon that generates trust and cooperation (Knack and Keefer Reference Knack and Keefer1997; La Porta et al. Reference La Porta, Lopez-de-Silanes, Shleifer and Vishny1997), fosters economic growth and financial development (Knack and Keefer Reference Knack and Keefer1997; Guiso et al. Reference Guiso, Sapienza and Zingales2004), and contributes to well-functioning institutions (Letki Reference Letki2006; Nannicini et al. Reference Nannicini, Stella, Tabellini and Troiano2013). Footnote 4 The social capital literature analyses the impact of civic norm conformity on various aspects of society. In their seminal paper, Knack and Keefer (Reference Knack and Keefer1997), for example, find a significantly positive relationship between civic norm conformity, trust and economic development. Other studies have related civic norm conformity to citizens’ participation in society (Dalton Reference Dalton2008), to citizens’ perception of their neighbourhood, and to citizens’ trust in others (Tabellini Reference Tabellini2008; Aghion et al. Reference Aghion, Algan, Cahuc and Shleifer2010). Also the roots of civic norms have received attention (see for example the work of Letki Reference Letki2006; Dinesen et al. Reference Dinesen, Nørgaard and Klemmensen2013; Kotzian Reference Kotzian2014).
To operationalize civic norm conformity, scholars in institutional economics typically use social survey databases (ESS, EVS and WVS among others). Footnote 5 They extrapolate survey items that inform us about one’s individual beliefs (Knack and Keefer Reference Knack and Keefer1997; Owen and Videras Reference Owen and Videras2006; Guiso et al. Reference Guiso, Sapienza, Zingales, Benhabib, Bisin and Jackson2011). In particular, survey items are selected that ask whether behaviour, such as avoiding taxes or claiming government benefits to which one is not entitled, is justified. Such types of behaviour are illustrative examples of instances in which civic norms play a role; tax avoidance as well as claiming benefits to which one is not entitled are both acts that can be considered as free-riding, since individuals choose to maximize their own private gain instead of public gains. The selected survey-items ask respondents to rate their beliefs regarding behavioural rules on a 10-point scale, where 1 is ‘never justifiable’ and 10 is ‘always justifiable’. Depending on the aim of the analysis, scholars sum the individual items for each individual (Knack and Keefer Reference Knack and Keefer1997), recode the items using only the extreme responses (Owen and Videras Reference Owen and Videras2006), or reduce the items to an underlying factor using factor analysis (Letki Reference Letki2006; Guiso et al. Reference Guiso, Sapienza, Zingales, Benhabib, Bisin and Jackson2011). Moreover, analysis is conducted at the individual level (Dalton Reference Dalton2008), national level (Knack and Keefer Reference Knack and Keefer1997) or at multiple levels (Letki Reference Letki2006; Kotzian Reference Kotzian2014).
Notice that the operationalization of civic norms considers whether an individual believes that the civic norm in question is justified, but not what an individual believes others will do. This is striking for various reasons. Accounts within the institutional economics literature incorporate the interaction between the individual and the group in their definitions, by taking civic norms as rules of behaviour that the members of a group follow so as to contribute to the common good and to overcome free-riding behaviour. As we saw before, also Bicchieri considers the inter-subjectivity of norms to be a crucial aspect of norm compliance. Moreover, within cross-cultural psychology and cross-cultural economics group-norms and their influence on individuals’ behaviour is increasingly taken into account. Perceived normative beliefs have been found to affect behaviour, and are in turn affected by (one’s perception of) group behaviour (Fishbein and Ajzen Reference Fishbein and Ajzen1975; Cialdini et al. Reference Cialdini, Kallgren and Reno1990). Footnote 6 Fischer (Reference Fischer2006) highlights the difference between self- and culture-referenced value ratings at the individual level. He argues that social norms regulate the latter. Relatedly, other scholars have argued that it is one’s perception of group behaviour, instead of the actual group behaviour or personal beliefs (Shteynberg et al. Reference Shteynberg, Gelfand and Kim2009), that directs norm conformity (Leung and Morris Reference Leung and Morris2015). The importance of social ties is also examined via social networks, in which individual beliefs are modelled as being affected by one’s connections with others (Bandiera and Rasul Reference Bandiera and Rasul2006; Videras et al. Reference Videras, Owen, Conover and Wu2012). Norms are one of the several mechanisms that are proposed as explanation of social linkages.
Furthermore, among others, Leung and Morris (Reference Leung and Morris2015) note that an ‘inter-subjective approach’ that accounts for interaction between individuals is not put forward in the institutional economics literature. Footnote 7 Inglehart and Welzel (Reference Inglehart and Welzel2005) provide more detailed evidence that indicates that civic norm measures are not as ‘civic’ as the literature suggests. Instead of measuring norm conformity, they capture a resistance to conformity pressures (Delhey et al. Reference Delhey, Newton and Welzel2011), which is, according to Bicchieri (Reference Bicchieri2006), an individual psychological parameter that might modulate norm compliance but does not determine it.
2.3. Comparing the approaches
At this point, we may want to take stock and reflect on the sense in which social norms and civic norms can indeed be compared in a meaningful way. After all, Bicchieri’s operationalization requires behavioural, empirical and normative expectations; survey measures, on the other hand, capture normative beliefs. The respondent’s expectations regarding others and his or her beliefs regarding the expectations of others are not taken into account.
One option would be to say that civic and social norms are simply different and their measurement procedure reflects such differences. Remember, however, that the concept of civic norms largely overlaps with the definition of social norms by Bicchieri (Reference Bicchieri2006). Both civic norms and social norms indicate rules that impose constraints on self-interest so as to solve social dilemma games, i.e. situations where the interest of a single individual is in conflict with the interest of the group. Furthermore, both economists and philosophers refer to behavioural rules that can be captured by one’s knowledge and beliefs.
Given the analogies, it would be surprising if different properties are operationalized in order to test such similar outcomes. Moreover, institutional economists seem to agree that the role of the group is crucial for civic norms, but they simply do not include it in their measurement. In this respect, it is important to notice that the operationalization of a definition is affected in important ways by the empirical method that is used for testing a related hypothesis. It may be the case that Bicchieri’s definition is too demanding when working with social survey data. Scholars in institutional economics are constrained by the availability of survey items, which have not been originally collected with the purpose of testing civic norm conformity. Other accounts that highlight the inter-subjectivity of norms may be less demanding than Bicchieri’s definition, yet they do not offer a theoretical framework in which expectations are conceptualized as essential conditions for norm conformity. While perhaps more demanding, Bicchieri’s account formalizes the role of the group in such a way that we can gain more insight into people’s motivation for norm conforming behaviour (as motivated by NEs and EEs). To examine this further, in the next section we suggest a possible way to operationalize Bicchieri’s three conditions while using social survey data, which can be used for standard examples of civic norms.
3. Data and methodology
Following institutional economists, we use the European Values Study. The EVS has been conducted four times since 1980, resulting in four waves. These are contained in a publicly available survey file that can be obtained from the GESIS Data Archive for Social Sciences. Footnote 8 This file covers European countries: 16 in the first wave, 29 in the second, 33 in the third, and 46 in the fourth and final wave. Footnote 9 With the exception of some small countries such as Malta, at least 1,000 respondents per wave per country have been interviewed.
For every country a representative sample of the population is constructed. The survey draws from the population aged 18 and older and takes ‘regions, districts, census units, election sections, electoral registers or voting stations, and central population registers’ into account (Beugelsdijk and Klasing Reference Beugelsdijk, Klasing, Costa-Font and Macis2017: 142). Moreover, the sampling procedure accounts for the degree of urbanization using so-called Primary Sampling Units. All data are gathered through face-to-face or phone interviews.
3.1. Operationalization of conditions
In order to assess ways to operationalize civic norm conformity while using social survey data, we take Bicchieri’s three conditions as point of departure. To start with, we are interested in measuring conformity to, for instance, the following behavioural rule: to pay a fare when travelling by public transport. In an ideal scenario, following Bicchieri’s framework, we would devise survey items that reflect each condition. We would ask for the behavioural condition ‘When travelling by bus or train, is there a rule requiring passengers to pay a fare?’. Footnote 10 Upon a positive answer to the previous question, the empirical question would ask: ‘When travelling by bus or train, to what extent do you expect that others pay their fare?’. Finally, the normative question would ask: ‘To what extent do you think that others expect you to pay your fare?’. Given Bicchieri’s framework, conformity to the norm is more likely when all three conditions are present, in other words, when the respondent answers positively to the three questions: ‘I know that, when travelling by bus or train, there is a rule requiring passengers to pay a fare’ – ‘I expect that (a sufficient number of) others pay their fare’ – ‘I expect that (a sufficient number of) others expect me to pay my fare’.
Following this line of thought, we looked for survey items that match the ideal scenario. To this purpose, we translated Bicchieri’s three conditions (behavioural, empirical and normative) into keywords that reflect aspects of each respective condition. In a next step, the keywords were used to extrapolate items from the EVS, and as such to operationalize each condition. We briefly describe our approach below.
For the behavioural condition, the EVS does not contain items that ask directly if a respondent is aware of the existence of a rule in a certain situation. However, we take the answer an individual gives about how he or she would behave in a situation as the closest indication of the behavioural condition. To do so, we translate the behavioural condition into keywords that ask for the respondents’ behaviour, for example: whether and how often they engage in certain activities, whether they are willing to engage in certain activities in the future, and whether they have done so in the past. Footnote 11
The empirical condition is represented by keywords that ask the respondents questions regarding other people’s behaviour, using words such as: most people, people in general, or compatriots. Who these ‘other people’ are is determined by the country of residence of the respondent. Survey items are adapted accordingly. In this manner the expectations towards other citizens are captured. For the operationalization of the normative condition, we note that the EVS does not contain items that ask whether the respondent expects that others believe that they should act in a certain way. Footnote 12 We thus follow the approach of existing measures and use normative beliefs as first-best operationalization of normative expectations. Footnote 13 We select keywords that are normatively laden, for example: moral rule, must, need to, one should or duty. In doing so, we assume that if an individual believes that x should be done or is justified, it is plausible to expect that this individual believes that others think that x should be done. Table A1 gives an overview of all keywords.
Table 1. Relation NE and EE conditional upon socio-demographic characteristics.

The nine columns show the regression results for nine separate regression models, in which the dependent variable is the NE for a particular behavioural R and the independent variable of interest is the EE for the same behavioural rule R. The table depicts clustered standard errors at the country level in parentheses. The regression models are estimated with country fixed effects. *** p < 0.01, ** p < 0.05, * p < 0.1.
3.2. Item selection
We use the keywords to search for items that operationalize the three conditions for social norm conformity. The search yields a large number of items (> 600). From this list, we find that there are 18 items that capture normative (NE) and empirical expectations (EE) with respect to the same behavioural rule. Footnote 14 For these rules, there are no items that can be used to test the behavioural condition together with the normative and the empirical conditions. Omission of the behavioural condition is, however, not as problematic as omitting the empirical or normative condition. The latter two conditions together define a conditional preference, which is, as argued in section 2.1, a prerequisite for social norm conformity.
The 18 extrapolated items ask for nine behavioural rules denoted as R: ‘Do you think it is justified to do [R]?’ (NE) and ‘According to you, how many of your compatriots do [R]?’ (EE). R captures civic behaviour that is related to the common good and is situated in the public sphere, such as cheating on your taxes or avoiding a fare when using public transport. The nine behavioural rules and accompanying items are listed in Table A2. Answers to the NE items are given on a 10-point Likert scale, where 1 reflects ‘never justified’ and 10 ‘always justified’. EE items are measured on a 4-point Likert scale. An answer of 1 reflects the belief that ‘almost all compatriots do it’ and 4 that ‘almost none do it’. We inverse the scale of the NE items such that their interpretation is in line with the interpretation of the EE items: a higher score means a greater likelihood that expectations are present. Footnote 15 Consequently, a lower score should be interpreted as a lower likelihood that expectations are present.
Table 2. NEs and EEs as two dimensions of civic norms.

Factor outcomes are rotated using an oblique rotation. N = 13,902.
Recall that norm compliance requires the respondent’s normative and empirical expectations to be both present (Bicchieri Reference Bicchieri2006). For the extrapolated items, this entails the following. Taking the rule claiming government benefits to which someone is not entitled as an example, an individual that answers the NE item with ‘never justified’ and the EE item with ‘almost no compatriot does it’ believes that claiming government benefits to which you are not entitled is never justified and that almost no compatriot claims government benefits. For the individual in question, both expectations are present and it is therefore likely that this individual conforms to the civic norm and contributes to the common good by, in this case, not claiming government benefits to which he or she is not entitled. Alternatively, when she answers the NE item with ‘never justified’ and the EE item with ‘almost all compatriots do it’, empirical expectations are absent while normative expectations are present. Following Bicchieri, the likelihood that this individual conforms to the civic norm is lower than in the previous case where both EEs and NEs are present. Similar interpretations hold for the other eight paired items.
While the NE items are available across multiple waves, the EE items have only been asked in wave 3 of the EVS (1999–2001). Footnote 16 We therefore use data from this wave in our analyses. Descriptive statistics can be found in Table A3. These statistics show that the mean values of the nine matched NE and EE items differ. T-tests of the difference in (normalized) means for each matched pair show that the differences are indeed statistically different as Bicchieri’s model would predict: it suggests that the expectations are empirically distinct and thus the likelihood to conform varies across individuals. In the next section we further examine the relation between the two types of expectations.
Table 3. The impact of NEs and EEs on trust.

Clustered robust standard errors at the country level in parentheses. Estimated with country fixed effects.
*** p < 0.01, ** p < 0.05, * p < 0.1.
4. Results
To examine the role of normative and empirical expectations for civic norm compliance more closely, we proceed in three interrelated ways. First, we assess the relationship between NEs and EEs. The second step is to determine whether they are empirically different aspects of civic norm compliance, while potentially part of the same construct. If NEs and EEs were to be empirically equivalent, the incorporation of either in empirical applications would suffice as measure of civic conformity. Third, we examine the relative importance of NEs and EEs further by using the case of trust as illustration.
4.1. The relation between NEs and EEs
Bicchieri’s model requires that expectations are both present for norm compliance; NEs and EEs should thus be positively related. To examine this further, we design a regression model for each behavioural rule that explains each NE item by its EE counterpart. A significantly positive coefficient signals that NE and EE are positively related. For example, for the behavioural rule not claiming government benefits we examine whether an individual who believes that his compatriots do not claim government benefits, is also more likely to believe that claiming government benefits is not justified. For each of the nine behavioural rules denoted as R, we estimate the following regression model:

where
$NE_{ij}^R$
captures normative expectations of individual
$i$
in country
$j$
for the behavioural rule R. A higher value reflects a greater likelihood that normative expectations are present.
$EE_{ij}^R$
reflects empirical expectations for individual
$i$
in country
$j$
for the same behavioural rule R, where a higher value reflects a greater likelihood that empirical expectations are present. We control for a set of socio-demographic characteristics included in the vector
${C_{ij}}$
, such as age, gender, highest attained level of education, income, employment status, marital status and number of children (summary statistics in Table A3). Moreover, since it is likely that individual expectations are affected by time invariant country specific factors, we include country fixed effects, as well as standard errors clustered at the country level to account for within-country correlation. We estimate the model by means of a limited probability model.
Footnote 17
The results in Table 1 indicate that for each of the nine matched behavioural rules, NEs and EEs are positively and significantly related. Thus for individuals for whom NEs are present, EEs are more likely to be present too, keeping other factors constant. This is in line with Bicchieri’s theory that posits a positive relation between NEs and EEs in the case of norm compliance. The size of the coefficients warrants more explanation however. From Bicchieri’s theory we can infer that if all individuals were to conform to the norm at all times, we would observe the
$EE_{ij}^R$
coefficients to be equal to 1. This is not the case: the coefficients range between 0.10 and 0.77.
Footnote 18
Bicchieri’s theory offers various explanations for this result. On the one hand, social norm conformity arises when a sufficiently large part of the community adheres to a social norm. Given that norm conformity requires the presence of both expectations, for individuals in this group of followers we would expect a large coefficient. It might thus be that a low coefficient pertains to a subset of non-followers. On the other hand, social norm conformity may arise for some individuals with a low coefficient, if for them norm conformity is driven solely by EEs. In these cases, NEs are absent while EEs are present. Overall, on the basis of the available information it is not possible to conclude what exactly a low coefficient implies regarding the motivation of the respondents, but it does not necessarily exclude norm following.
In addition we find that, on average and keeping other factors constant, women’s NEs are higher than those of men. The same holds for individuals that are married or elderly. This may signal that these groups are more likely to comply with civic norms, on the condition that empirical expectations are present too. We also find that for individuals without a job, normative expectations are less likely to be present, keeping other factors constant. This negative covariation might signal cases where individuals are less inclined to conform to civic norms, especially if empirical expectations are absent.
4.2. The two components of civic norms
The selected items reflect various aspects of civic norms and may therefore be correlated. We conduct a factor analysis at the individual level that exploits the correlational structure among the 18 items of interests. The results give further insights into the relation between NEs and EEs across the nine behavioural rules and their potential for empirical applications.
Factor analysis is a technique for data reduction. The technique looks for linear combinations of the variables that explain as much of the variability as possible, exploiting the correlation between observed variables (in our case: the 18 extrapolated items). In this way a set of new variables – latent variables – is defined that underlie the observed patterns in the data. Our latent construct of interest is civic norm conformity, potentially showing two dimensions that reflect NEs and EEs as hypothesized. The estimation results are rotated using a so-called oblique rotation, yielding factors that are unconstrained. Footnote 19 Following the literature, we use a factor loading greater than 0.4 as the cut-off value (Stevens Reference Stevens1992). Variables with a lower loading can be interpreted as uninformative, as they are only marginally correlated to the underlying factor.
We select the appropriate number of factors using several selection criteria: Cattell’s scree-test (also known as the ‘elbow criterion’) and the Kaiser-criterion. The first criterion prescribes that we should retain the number of factors below the kink of the scree plot (as depicted in Figure A1) (Cattell Reference Cattell1966). We observe a kink at three factors, suggesting that we should retain two. The second criterion uses the eigenvalues as benchmark. Factors with an eigenvalue greater than 1 are retained. Based on this criterion, we should also retain two factors (see Figure A1). Given the selection criteria, we conclude that a two-factor model is the best representation of the underlying factor structure.
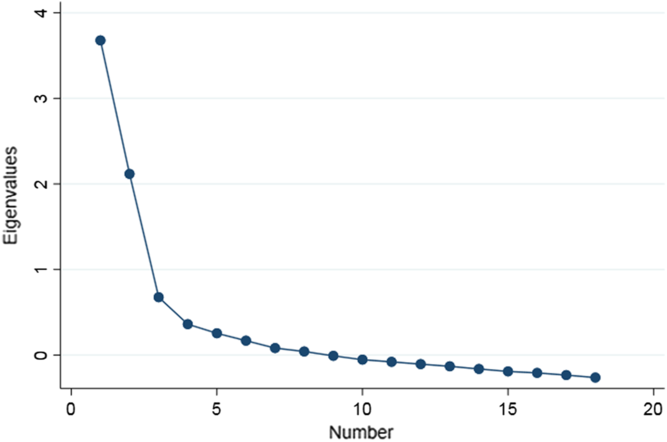
Figure A1. Scree plot of eigenvalues.
Table 2 shows that the two-factor model yields a clear pattern: we find two factors that respectively reflect NEs and EEs. Items that ask whether certain behavioural rules are justified load on one factor – factor 1 – whereas items that ask whether compatriots display certain behaviour, load on factor 2. The two dimensions are only weakly correlated, as the significant correlation coefficient of 0.08 indicates (Pearson correlation coefficient). While significantly different from zero, the correlation coefficient is sufficiently low to support the claim that NEs and EEs are empirically distinct dimensions, while at the same time part of the same construct. Additionally, the factor output indicates that NEs and EEs regarding a particular behavioural rule, such as claiming government benefits to which one is not entitled, are related to expectations regarding other kinds of behavioural rules, such as paying a fare when using public transport. A norm-abiding citizen seemingly contributes to the many different facets of the common good, or in other words, when contributing to the common good, reciprocity arises as a natural by-product.
In the subsequent step we approximate the two latent factors using the average values of the nine items that load on each factor, labelling them NEs and EEs. Averages are calculated even if the respondent has not answered all items. This is a generally accepted approach, given that the latent factors underlie the patterns that we observe (see for example Welzel Reference Welzel2013). Footnote 20 We do so in order to maintain as many observations as possible (N = 38,278). Some items have only a limited number of observations; if we would only rely on these respondents our sample would be greatly restricted (N = 13,902). Footnote 21 Since the approximated latent factors correlate perfectly with the latent factors, we are confident that they measure the same construct.
4.3. An illustration: EEs explain trust
To examine the empirical difference between NEs and EEs in greater detail, we revisit the relationship between civic norms and trust. Within the institutional economics literature, this case has received much attention since the seminal paper of Knack and Keefer (Reference Knack and Keefer1997). They have shown that trust and civic norm compliance enable economic growth. The study spiked scholars’ interest in the topic (Beugelsdijk et al. Reference Beugelsdijk, de Groot and van Schaik2004; Tabellini Reference Tabellini2008, Reference Tabellini2010; Aghion et al. Reference Aghion, Algan, Cahuc and Shleifer2010; Guiso et al. Reference Guiso, Sapienza, Zingales, Benhabib, Bisin and Jackson2011). Scholars found that civic norms foster cooperation, thereby instilling a sense of respect and trust towards others (so called generalized trust).
As explained in sections 2.2 and 2.3 of this paper, the empirical evidence supporting the claim that civic norm conformity and trust are positively correlated relied on measures of normative expectations (amongst others, see the work of Knack and Keefer Reference Knack and Keefer1997; Owen and Videras Reference Owen and Videras2006; Guiso et al. Reference Guiso, Sapienza, Zingales, Benhabib, Bisin and Jackson2011). The empirical expectations regarding others’ civic behaviour have not been considered in these applications. We therefore revisit the relationship between civic norm conformity and trust to examine the relative importance of NEs and EEs further. While these analyses yield interesting insights into the relationship between civic norm conformity and trust, we will not deal with them in a detailed manner as they fall outside the scope of this paper.
To tease out the relative importance of NEs and EEs, we reproduce results of existing studies in the first stage (eq. 1). Hereafter we run the same regression for EEs (eq. 2). After we have established the effect of NEs and EEs separately, we include both to see which one of the two survives in a ‘horse-race’ regression (eq. 3). To the fourth and final regression we add an interaction term
$E{E_{ij}}*N{E_{ij}}$
to see whether the effects of NEs and EEs are interrelated (eq. 4). More formally, we estimate the following set of equations:




${Y_{ij}}$
is a binary variable that reflects generalized trust for individual
$i$
in country
$j$
. We measure trust using the following EVS-item: ‘Generally speaking, would you say that most people can be trusted or that you need to be very careful in dealing with people?’ (Knack and Keefer Reference Knack and Keefer1997; Tabellini Reference Tabellini2008; Guiso et al. Reference Guiso, Sapienza, Zingales, Benhabib, Bisin and Jackson2011).
Footnote 22
A value of 1 indicates that the respondent trusts most people. We control for the same set of socio-demographic characteristics in the vector
${C_{ij}}$
as in section 4.1. The variables of interest –
$N{E_{ij}}$
and
$E{E_{ij}}$
– are constructed as described in the previous section. Moreover, we include country fixed effects, as well as clustered standard errors at the country level, to control for any time invariant country specific factors.
We estimate the four equations using a fixed effects logistic regression model to account for the binary nature of our dependent variable. We estimate the four equations on the same sample of respondents for matters of comparability. Respondents are included if they have an average value for both
$N{E_{ij}}$
and
$E{E_{ij}}$
. Table 3 tabulates the estimation results. We only report the coefficients of interest (
$N{E_{ij}}$
,
$E{E_{ij}}$
and
$E{E_{ij}}*N{E_{ij}}$
).
Footnote 23
Coefficients are reported as log odds, which are the natural logarithm of an odds ratio. Positive log odds indicate a positive relationship, and vice versa. To give an account of the effect sizes, we refer to odds ratios in the text. Odds ratios indicate the ratio of the odds of success for one group, divided by the odds of success for another group. In our case, we look at the odds of trusting most people versus the odds of feeling that one needs to be careful when dealing with other people.
Table 3 shows that citizens that are likely to conform to civic norms tend to be more trusting towards others. More specifically, the odds of saying that most people can be trusted are 1.5 times higher for each point increase in the level of EEs, all else equal. Interestingly, we find that only EEs drive this result; NEs have an insignificant relation to trust even when we do not control for EEs (column 1). These findings are striking given that the literature has relied predominantly on measures of NEs when examining the relationship between civic norm conformity and trust. Our results suggest that generalized trust is enforced when individuals expect that others adhere to civic norms (columns 2–4). This implies that we tend to be more trusting when we expect that others are engaged, cooperating citizens. Whether we control for NEs or not, the significantly positive effect of EEs on trust remains.
The findings illustrate that empirical expectations also have explanatory power. This is in line with findings of Bicchieri and Xiao (Reference Bicchieri and Xiao2009) who establish that empirical expectations regarding the behaviour of others matter relatively more for individual decision making than normative expectations. Moreover, the results show that NEs should not be taken as conclusive in assessing civic norm conformity; in line with Bicchieri’s theory the results suggest that NEs and EEs are inherently different aspects of norm conformity.
One may be concerned that the results in Table 3 are affected by the choice of generalized trust as dependent variable. The generalized trust item can be rephrased as ‘According to you, how many of your compatriots do things that make you trust them (…)?’. Phrased this way, generalized trust appears an empirical expectation (following the operationalization in section 3) and then it is unsurprising that EEs are positively correlated with generalized trust. For this to be true, generalized trust should depend on the behaviour of others. In institutional economics, a body of literature has shown that general trust is an inherited value (Glaeser et al. Reference Glaeser, Laibson, Scheinkman and Soutter2000; Guiso et al. Reference Guiso, Sapienza and Zingales2006; Uslaner Reference Uslaner2008; Algan and Cahuc Reference Algan and Cahuc2010; Dohmen et al. Reference Dohmen, Falk, Huffman and Sunde2012). Trust persists via the parental transmission (Guiso et al. Reference Guiso, Sapienza and Zingales2006) and is even shown to have roots that go further back into time (Uslaner Reference Uslaner2008). Moreover, a study of Delhey et al. (Reference Delhey, Newton and Welzel2011) shows that the generalized trust item works well as a measure of generalized trust in Western and affluent nations, but less so in other countries. For the sample of countries included in our analyses this implies that generalized trust can be considered as a deeply rooted value that extends beyond the family onto people that differ from yourself (Uslaner and Conley Reference Uslaner and Conley2003). Given these properties, we have no reason to suspect that our measurement results are biased.
5. Robustness analyses
To corroborate our findings we run several additional analyses. The analyses confirm that the main findings are robust to alternative specifications and estimation techniques.
5.1. Response-style biases
We assess whether survey responses are affected by factors that are unrelated to the survey item’s content (Baumgartner and Steenkamp Reference Baumgartner and Steenkamp2001; Beugelsdijk and Klasing Reference Beugelsdijk, Klasing, Costa-Font and Macis2017). Such response-styles may bias our results and therefore warrant attention. Previous research has shown that extreme response styles (ERS) can be explained by country-level extraversion (Harzing Reference Harzing2006), and are more likely to be encountered in countries with high uncertainty avoidance and high power distance (Hofstede Reference Hofstede1980). ERS refers to responses that are at the extreme ends of an answer-scale (negative or positive). In contrast, people may also have a tendency to give responses that lie in the middle of the answer-scale, so-called middle response styles (MRS). Such styles are argued to be more prevalent in collectivist societies (Hofstede Reference Hofstede1980). For further details regarding these hypotheses we refer to the work of Harzing (Reference Harzing2006) and Beugelsdijk and Klasing (Reference Beugelsdijk, Klasing, Costa-Font and Macis2017).
We test for ERS and MRS biases in the following manner: negative ERS is the fraction of people within a country that has given either the response 1 (EE) or 9/10 (NE). Positive ERS is defined as the fraction of people within a country that has given the response 4 (EE) or 1/2 (NE). MRS on the other hand focuses on the middle range responses: the fraction of people that has answered 2/3 (EE) or 5/6 (NE). We run a set of regressions at the country level with negative ERS, positive ERS and MRS as dependent variables and the degree of extraversion (Lynn and Martin Reference Lynn and Martin1995; Hemert et al. Reference Hemert, van de Vijver, Poortinga and Georgas2002), individualism, power distance and uncertainty avoidance as independent variables (Hofstede Reference Hofstede1980, Reference Hofstede2001). Significant effects of the four independent variables indicate we should be concerned for the consistency of the main findings. However, we find no significant relations between the dependent and independent variables: all coefficients are not significantly different from zero (see Table A4). This suggests that the results are not liable to response style biases.
Table 4. The impact of NEs and EEs on trust (sensitivity analysis).

Clustered robust standard errors at the country level in parentheses. Estimated with country fixed effects.
*** p < 0.01, ** p < 0.05, * p < 0.1.
5.2. Operationalization of NEs and EEs
NEs and EEs are measured on two different scales, i.e. a 10-point and 4-point scale. We check whether the results in Table 3 change if we rescale the NE items such that both NE and EE items are measured on a 4-point scale: for all NE items we construct a measure that attributes the values 1, 2 and 3 to a value 4; the values 4 and 5 to a value 3; the values 6 and 7 to a value 2; and the values 8, 9, and 10 to a value 1. Footnote 24 We take the average value of the nine survey items to reconstruct our NE measure and use this measure in our regression model (eq. 1–4). For matters of brevity, Table 4 displays the estimation results of eq. (3) in column (1) (Table A5 part (a) displays the full results). The main findings are corroborated: the coefficients on NEs and EEs are of the same size and significance as those reported in Table 3. This gives us no reason to think that the results are driven by methodological considerations.
Table 5. Multilevel analyses of trust (sensitivity analysis).

Standard errors in parentheses. *** p < 0.01, ** p < 0.05, * p < 0.1.
Additionally, we examine whether the results in Table 3 are affected by the operationalization of NEs and EEs as approximated latent factors. As explained in section 4.2, we approximate the two latent factors by calculating the average value of the nine items that load highly on the respective factor even if the respondent has not answered all items. While these measures perfectly correlate with predicted factor scores, we assess whether this decision has affected the main results. We therefore approximate the two latent factors by the average values for only those respondents that have answered all 18 items. This restricts our sample from 38,278 respondents living in 33 countries to 13,902 respondents that live in 12 countries. Footnote 25 We re-estimate the regression model (eq. 1–4) with the newly constructed approximated latent factors. Table 4 displays the results for equation (3) in column (2) (Table A5 part (b) displays the full results). When comparing the tabulated results in Table 4 with those tabulated in Table 3, we can observe that the EE coefficient is of comparable size and significance. Interestingly, the NE coefficient is significantly different from zero. While the coefficient is small, especially considering the effect size of EEs, we explore this finding further.
A subset of the 18 items has been surveyed in a very limited set of countries.
Footnote 26
We expect that the limitation of the country sample explains the findings displayed in column (2). We therefore construct a dummy (
${\delta _{ij}}$
) that indicates whether respondents are in the restricted country sample (
${\delta _{ij}}$
= 1) or in the unrestricted sample (
${\delta _{ij}}$
= 0). We add this dummy to our estimation model (eq. 1–4) as well as an additional interaction term with either NE, EE or both. The displayed results (columns 3–7 in Table 4) show that the interaction-effect is only significantly different from zero when interacted with NE (columns 3, 5 & 7). The effect of EEs on generalized trust, conditional upon controls, remains significantly positive (columns 4–6). These results suggest that country sample matters especially for the effect of NEs on trust and not so much for the effect of EEs on trust. This is in line with other studies that have shown that norm conformity at the country level is highly diverse and that there are various institutional factors that affect this (Gelfand et al. Reference Gelfand, Nishii and Raver2006, Reference Gelfand2011).
5.3. Multilevel analysis
The individuals in our data set live in various European countries. Observations are therefore likely to be clustered within countries, which is why we have estimated equations 1–4 in Table 3 using clustered standard errors at the country level. Another way to account for the nested structure of the data set is to use hierarchical modelling. Such a model differs from our previous analysis in that it assigns a statistical model to each hierarchical level (in our case, individual versus country level) and accounts for the varying group sizes (in our case, country sizes). Therefore, when working with nested data, it has been argued that such models yield more efficient estimates (Snijders and Bosker Reference Snijders and Bosker2012).
We estimate an empty hierarchical model that accounts for the nested structure. We thus remain agnostic as to the explanation of any country level variation. Similar to our previous analyses, we use a fixed effects logistic regression model to account for the binary nature of our dependent variable. Moreover, we include the same set of socio-demographic factors as done in equations 1–4 but do not display these results (see online appendix for results). The results in Table 5 indicate that the key findings are robust to the specification of our model. As expected, the estimates of the hierarchical logistic model are more efficient than those of the logit model as shown by the smaller standard errors.
5.4. Disaggregating the effect of NEs and EEs
Based on the factor analysis in Table 2, we have constructed approximated latent variables of NEs and EEs. We examine whether the findings tabulated in Table 3 are robust at the item level. We conduct the same analysis as in section 4.3, yet instead of including the average value of NEs and EEs, we relate the separate behavioural rules to generalized trust. We report the coefficients on NEs and EEs for equations 1–4 in Table 6 for the first behavioural rule, namely Claiming state benefits to which one is not entitled. Results for the other items resemble those displayed in Table 6 and are tabulated in Table A6 in the appendix. The results indicate that the positively significant relation between EEs and trust also holds at the item level, keeping other factors constant. We find that the presence of EEs strengthens trust, even when NEs are controlled for. In contrast to the results in Table 3, the relation between EEs and trust becomes insignificant when moving from specification (3) to (4) for the majority of behavioural rules. It may be the case that the variation that is unique to each rule obscures the relationship that we found at the dimensional level. Factor analysis is not liable to such variation, since it relies on the variation that is common to all input variables. We therefore recommend the use of a data reduction technique, such as factor analysis, in similar applications.
Table 6. The impact of NEs and EEs on generalized trust (item level).

Clustered robust standard errors at the country level in parentheses. Estimated with country fixed effects. *** p < 0.01, ** p < 0.05, * p < 0.1.
6. Discussion and conclusion
This paper proposes a novel approach to measure civic norm compliance, introducing a dimension that has not been considered before in the institutional economics literature. Central to our argument is the theoretical distinction between empirical expectations and normative expectations (Bicchieri Reference Bicchieri2006). Interestingly, we note that survey studies on civic norm compliance have not included empirical expectations – an individual’s beliefs regarding civic behaviour of others. Our main findings illustrate that empirical expectations do matter: normative and empirical expectations are correlated, yet they capture distinctive dimensions of civic norms. In particular, we show that they have distinct effects on general trust.
To construct our measure of norm compliance, we have conducted an extensive search for survey items that can operationalize Bicchieri’s conditions for norm conformity. We find nine items that capture normative expectations and nine items that capture empirical expectations for civic norm conformity. To examine the two types of expectations, we conduct a factor analysis at the individual level to assess the factor structure of our items. The results confirm that there are two dimensions to civic norm conformity that capture the notions of empirical and normative expectations. We examine the relationship between the two expectations in greater detail by assessing their relative importance, using the well-known example of generalized trust. We find that normative expectations’ effect on trust is not significantly different from zero, whereas expectations regarding other people’s behaviour contribute to trust. This implies that trust mainly depends on what we expect others will do: if one expects that other people contribute to the common good, the inclination to trust is greater. Robustness analyses indicate that this finding cannot be explained by response-style biases or choices made with regards to the operationalization of our variables of interest.
The results indicate that, in the case where normative and empirical expectations are not simultaneously present, empirical expectations still motivate behaviour. This finding not only calls for a re-evaluation and reconsideration of methods to measure civic norms compliance, but has similar implications for studies that assess other norms such as generosity or solidarity (Beine et al. Reference Beine, Docquier and Schiff2013; Burda et al. Reference Burda, Hamermesh and Weil2013; Helliwell et al. Reference Helliwell, Wang and Xu2016). These norms qualify as social norms from Bicchieri’s point of view, yet their measures focus mainly on normative beliefs or stated preferences. While the current work does not allow us to draw any conclusions regarding these norms, it can motivate efforts to examine related issues in the proposed directions. Moreover, there may be alternative approaches to model and analyse cases in which normative and empirical expectations are not simultaneously present. Such an extension would be an interesting option for future research.
Our results have not answered the question why empirical expectations trump normative expectations. On the one hand, it may be the case that deviant behaviour is not (sufficiently) punished and therefore the threat of punishment is no longer sufficient for norm conformity. Normative expectations are then relatively less powerful in comparison to empirical expectations, which would explain our finding. On the other hand, our operationalization of normative expectations as personal normative beliefs may not fully capture the theoretical notion of normative expectations. However, the use of personal normative beliefs in other studies did yield the hypothesized effect (Knack and Keefer Reference Knack and Keefer1997; Aghion et al. Reference Aghion, Algan, Cahuc and Shleifer2010; Guiso et al. Reference Guiso, Sapienza, Zingales, Benhabib, Bisin and Jackson2011). Future research is needed that differentiates between these types of expectations and beliefs, such that their interaction with norm conformity and economic decision making can be further investigated. Other promising extensions of the current work lie in the generation of new social survey data that follow Bicchieri’s definition of social norms more closely. Potentially such methods can be combined with the experimental designs that we find in philosophy, as Rauhut and Winter (Reference Rauhut and Winter2010) have suggested in earlier work. In this manner, the complexity of survey research can be combined with measures of revealed preferences in experimental settings.
Acknowledgements
Earlier versions of this paper were presented at the International Network for Economic Methodology in San Sebastián, Spain (2017), the European Network for the Philosophy of Social Sciences in Kraków, Poland (2017), the OZSW conference in Doorn, the Netherlands (2017) and the International Conference of Economic Philosophy in Lyon, France (2018). We wish to thank those present for their valuable comments and feedback. We also thank Boudewijn de Bruin, Sjoerd Beugelsdijk, Robbert Maseland, Maite Laméris, Johannes Kleinhempel and two anonymous referees for their comments and suggestions that significantly improved this paper.
Supplementary material
For supplementary material referred to in this article, please visit https://doi.org/10.1017/S0266267120000152
Appendix
Table A1. Keywords to extrapolate items from EVS.

Table A2. Extrapolated items from EVS.

Table A3. Descriptive statistics.

Table A4. Response style biases.

Standard errors in parentheses. *** p < 0.01, ** p < 0.05, * p < 0.1.
Table A5. The impact of NEs and EEs on trust (sensitivity analysis).

Clustered standard errors at the country level in parentheses. Estimated with country fixed effects.
*** p < 0.01, ** p < 0.05, * p < 0.1.
Table A6. The impact of NE and EE on generalized trust (item level).

Estimated with country fixed effects. Robust standard errors in parentheses. *** p < 0.01, ** p < 0.05, * p < 0.1.
Juliette R. de Wit is a PhD candidate in economics at the University of Groningen, Faculty of Economics and Business. Working on the intersection between philosophy and economics, she is interested in social constructs, their measurement, and how they can be incorporated into economic theory. URL: https://www.rug.nl/staff/juliette.de.wit/
Chiara Lisciandra is Assistant Professor at the University of Groningen, Faculty of Economics and Business. She is a Board Member of the Centre for Philosophy, Politics and Economics at the University of Groningen and an Associate Member of the Centre for the Philosophy of the Social Science, University of Helsinki. She has published extensively on norms in a wide range of scientific journals, such as Philosophical Studies, Synthese and Politics, Philosophy & Economics. Her primary research interests are in general philosophy of science, philosophy of economics and social philosophy. URL: https://www.chiaralisciandra.com