Genomics and the origins of ‘big data’ in understanding human biology
As a scientific discovery that befitted the turning of a millennium, the initial sequencing of the human genome by two independent groups was announced jointly by the president of the USA and the prime minister of the UK to much fanfare in June 2000(1). Published the following February in tandem, in the journals Nature (Reference Lander, Linton and Birren2) and Science (Reference Venter, Adams and Myers3), these initial draft sequences were the result of several decades of technological achievements(Reference Roberts, Davenport and Pennisi4) and represented biomedical science's first major foray into ‘big science’(Reference Collins, Morgan and Patrinos5). Multiple incremental advances in several fields, including molecular biology, chemistry, physics and robotics, led to the revolutionary innovation of capillary-based DNA sequencing instruments. These, alongside advances in computer science, ultimately permitted the reconstruction of these first draft sequences(Reference Green, Watson and Collins6).
At the time of completion of the human genome project (HGP), the estimated cost of sequencing a single human genome was US$100 million, and could be achieved in 9 months using 350 of the state-of-the-art capillary DNA sequencers running in parallel(Reference Venter, Smith and Adams7). In the two decades since, further remarkable advances in sequencing technology have driven the cost of sequencing a human genome down exponentially, with costs approaching only US$1000 per genome since 2015(Reference Wetterstrand8). Large-scale massively parallel sequencing, or next-generation sequencing technologies, now make possible the shotgun sequencing of several thousand human genomes a month(Reference Venter, Smith and Adams7). By necessity at each stage, advances in sequencing technologies have been accompanied by advances in bioinformatics and data analysis pipelines that have inextricably linked the fields of genomics and computational biology(Reference Mardis9). This has permitted the identification of variation in the human genome in a variety of different contexts in an unprecedented manner.
Since the HGP, multiple large-scale genomics efforts have focused on identifying and understanding the scale of human genetic variation. The first of these, the International HapMap project begun in 2002, aimed to catalogue common human genetic variants (SNPs) and how they linked together (a haplotype). Initially focused on characterising common SNPs, present at 5 % or greater allele frequency, in four populations with ancestry from Africa, Europe and Asia(10), HapMap was subsumed into the 1000 Genomes project begun in 2008 after the introduction of next-generation sequencing, which ultimately provided much greater resolution of genetic variation in fourteen populations(Reference Abecasis, Auton and Brooks11). In addition to characterising 38 million SNPs present at 1 % or greater allele frequency, the 1000 Genomes project mapped 1⋅4 million short insertions and deletions (indels), and more than 14 000 larger deletions. Such mapping efforts greatly expanded our understanding of the breadth of human genetic variation and made feasible genome-wide association studies (GWAS) relating multiple genetic variants to common complex diseases.
The path towards precision medicine
Essentially large case–control cohort studies, GWAS compare the distribution of SNPs in thousands of people with and without a particular disease. The first raft of these studies was published in 2007, providing insight into multiple common chronic diseases and prompting Science magazine to declare human genetic variation the breakthrough of the year(Reference Pennisi12). Perhaps most significant, and considered ‘paper of the year’ by the Lancet (Reference Summerskill13), was an unprecedented study from the Wellcome Trust Case Control Consortium, a group of fifty research groups across the UK. This work identified genetic associations in cohorts of 2000 patients with one of seven chronic diseases (type 1 and type 2 diabetes, hypertension, coronary artery disease, Crohn's disease, rheumatoid arthritis and bipolar disorder) in comparison to a set of 3000 control participants(14). Indeed, since its participation in the international HGP, the UK has consistently remained at the forefront of large-scale efforts in genomics, with the Wellcome Trust Case Control Consortium laying the groundwork for the subsequent UK Biobank and 100 000 Genomes projects.
Initiated in 2006, the UK Biobank is a prospective population-cohort of 500 000 individuals that has gathered genome-wide genetic data along with linked detailed physical and clinical information on the participants who were aged 40–69 years at recruitment(Reference Bycroft, Freeman and Petkova15). Notable both for its scale and commitment to data sharing, the project follows participants through health-related records and national registries for hospital admissions, cancer diagnoses and deaths. Whereas the UK Biobank used array technology to analyse 825 927 genetic markers in healthy volunteers followed over time; the more recent 100 000 Genomes project, begun in 2013 after a significant reduction in the cost of next-generation sequencing, has applied whole-genome sequencing to patients with either rare diseases or cancer(Reference Turnbull, Scott and Thomas16). Rare diseases are typically Mendelian, caused by single gene defects, and manifest before age 5 years. Accurate genetic diagnosis can make an enormous difference in disease management for the patient and inform families about the risk of recurrence. Similarly, understanding what genomic alterations have taken place in cancer can provide diagnostic and prognostic information and has been critical in the development of targeted therapies for select epithelial malignancies(Reference Stevens and Rodriguez17).
Inherent in these large-scale genomics projects has been the belief that with a better understanding of genetics will come improved treatments for individuals. Therefore, a not insignificant aim of the 100 000 Genomes project was to imbed the infrastructure required to provide a genomic medicine service within the UK National Health Service(Reference Turnbull, Scott and Thomas16). It has long been recognised that many chronic diseases such as cancer, which phenotypically look broadly similar, vary significantly in molecular aetiology. Consequently, the same medication given to a group of heterogeneous patients may be beneficial in some patients and not in others, and potentially also toxic for some patients and not for others. The worst-case scenario for patients would be to receive medicine that has no benefit and is toxic. Stratified medicine (see Table 1 for definitions) simplistically aims to subgroup and identify patients that will benefit from treatment without experiencing toxicity. Subgroups can be based on a combination of disease subtypes, clinical features, demographics, risk profiles, biomarkers or molecular assays. Possibly the best known example of stratified medicine has been the molecular subtyping of breast cancer based on hormone receptor (the oestrogen and progesterone receptors) and human epidermal growth factor receptor 2 expression(Reference Prat, Pineda and Adamo18). While the most successful applications of stratified medicines to date have largely been in cancer and genetic diseases, many other therapies with associated biomarkers are beginning to be adopted (by the UK National Health Service) or are in the development pipeline(19).
Table 1. Terminology

Therefore, the vision of personalised or precision medicine in most areas of medicine is arguably still aspirational. Precision medicine aims ultimately to tailor treatments to an individual based on molecular features (plus lifestyle and environment) of a patient and/or their disease; ideally also using companion diagnostics to determine responders and non-responders to the therapy. While the terms stratified, systems, personalised and precision (Table 1) have been used interchangeably, and in some cases fiercely debated(Reference Erikainen and Chan20), the term precision medicine is now preferred and has been more commonly used in the medical literature since 2010 (Fig. 1(a)). In calling for a new (molecular) taxonomy of disease towards precision medicine, concerns outlined by the US National Research Council were that the term personalised could be misinterpreted as implying that unique treatments can be designed for each individual, in part because it had been widely used in advertisements for commercial products(21). These concerns were echoed by the European Society for Medical Oncology in their Precision Medicine Glossary(Reference Yates, Seoane and Le Tourneau22). Additional reasons outlined by the European Society for Medical Oncology were that precision medicine better reflects the highly accurate nature of new technologies that permit base pair resolution dissection of cancer genomes; whereas personalised medicine could describe all modern oncology practice that takes into account patient factors such as personal preference, cognitive aspects and co-morbidities in addition to treatment and disease factors(Reference Yates, Seoane and Le Tourneau22).

Fig. 1. (Colour online) Recent growth in publications in the PubMed database using specified terms. (a) Number of publications using adjectives precision, personalised, systems or stratified in conjunction with medicine since 2007. Data were generated by performing a PubMed [All Fields] search with terms searched within double quotation marks, e.g. “precision medicine”. Personalised medicine was searched as: “personalised medicine” or “personalized medicine”. (b) Growth in publications in genomics, transcriptomics, proteomics and metabolomics since 2001. Genomics, proteomics and metabolomics were searched as: “genomics”[MeSH] or “genomics”[All Fields]. Transcriptomics was searched as: “gene expression profiling”[MeSH] or “transcriptomics”[All Fields].
Functional genomics
As the HGP was drawing to completion, came the goals of functional genomics; namely applying high-throughput genome-wide approaches to studying gene transcription, translation and protein–protein interactions. Along with the overuse of the suffixes -ome and -omics(Reference Lederberg and McCray23), emerged research efforts in transcriptomics, proteomics and metabolomics. There was an early recognition that ultimately if viewed together, comprehensive datasets along the entire ‘omics cascade’ would provide significant insights into the response of biological systems to genetic, environmental or disease-mediated perturbations(Reference Dettmer, Aronov and Hammock24). Initial functional genomic insights came from transcriptome profiling experiments, with early applications in the nutritional sciences including the identification of genes regulated by dietary zinc(Reference Cousins, Blanchard and Popp25,Reference Moore, Blanchard and Cousins26) . The genomic sequence information from the HGP in combination with advances in lithography led to high-density DNA arrays that made it possible to measure the levels of gene expression for tens of thousands of genes simultaneously; superseding the more laborious and technically challenging differential display approach(Reference Cousins, Blanchard and Moore27).
However, while an individual's genome and transcriptome yield insight into ‘what can happen’, critical to precision medicine are clinical biomarkers, which are most commonly proteins or metabolites and speak to ‘what is happening’(Reference Dettmer, Aronov and Hammock24). Proteins and metabolites are chemically much more complex and heterogeneous than nucleic acids; and therefore, much more challenging to isolate, identify and measure. Consequently, publications in the fields of proteomics and metabolomics have risen subsequent to, and at a lower rate than, those in genomics and transcriptomics (Fig. 1(b)). Unsurprisingly then, the human proteome, the functional compartment encoded by the genome, emerged as a next logical biological challenge to be tackled internationally after completion of the HGP(Reference Abbott28). The Human Proteome Organization was founded in 2001 in large part to promote and coordinate open access initiatives in this field(Reference Hanash and Celis29). With recognition of the critical role of small-molecule (<1500 Da) metabolites in clinical diagnostics and as pharmaceutical agents, complementary efforts in metabolomics followed in short order(Reference Wishart30).
Whereas sequencing an entire genome is now relatively inexpensive and technologically feasible by next-generation sequencing within a few hours, measuring a proteome or metabolome in its entirety is still not possible from a single experimental approach. Nonetheless, advances in MS and NMR spectroscopy, along with bioinformatics, databases and annotation, mean that we can now measure many, many more proteins and metabolites in single runs than two decades ago. Building on early tissue-specific (plasma, liver, brain), antibody and data standard development initiatives, the human proteome project was formally launched by the Human Proteome Organization in 2010(31). The work of fifty international collaborating research teams is organised by chromosome, biological processes and disease categories and has since been reported collectively annually. As of 2019, robust MS data have been reported for 89 % of the 19 823 predicted coding genes, and separate antibody-based histochemical evidence exists for the expression of 17 000 proteins(Reference Omenn, Lane and Overall32). While such cataloguing efforts are not without their detractors(Reference Stern33), the efforts of ‘discovery science’ clearly can and have fostered hypothesis-driven approaches(Reference Spanos, Maldonado and Fisher34). In the context of the human proteome project, multiple strands of research have identified biomarkers and characterised molecular mechanisms of human disease, contributing to efforts towards precision medicine(Reference Omenn, Lane and Overall32).
Systems biology
Systems biology as a discipline, although proposed as early as 1966(Reference Rosen35), became truly established in the aftermath of the HGP(Reference Ideker, Galitski and Hood36,Reference Kitano37) . Representing the antithesis of reductionism, systems biology combines molecular and computational approaches to understand highly complex interactions within, and ultimately predict the behaviour of, biological systems as a whole(Reference Moore and Weeks38,Reference Fisher, Kierzek and Plant39) . From early in its conceptualisation, both the generation and the integration of different levels of biological information (e.g. genomic, transcriptomic, proteomic, metabolomic), in order to yield predictive mathematical models, were articulated as fundamental to systems biology(Reference Ideker, Galitski and Hood36). Therefore, whereas the high-throughput datasets of genomics and proteomics provide the foundation for the ‘reconstruction’ of biological networks at the genome-scale; it is a computational simulation that yields insights into the systems structure and dynamics, and predicts biological outcomes(Reference Fisher, Kierzek and Plant39,Reference Kitano40) .
The first institute for systems biology was founded in 1999 in the USA by Leroy Hood, whose early work had made seminal contributions to the fields of genomics and proteomics through the development of high-throughput instrumentation for DNA and protein sequencing; in addition to this, he led significant sequencing efforts that contributed to the HGP(Reference Agrawal41). Undoubtedly a visionary, who viewed continued advances in high-throughput measurement technologies, databases and tools for integrating the various levels of biological information, essential to systems biology(Reference Ideker, Galitski and Hood36); Hood's institute radically brought together biologists, chemists, computer scientists, engineers, mathematicians, physicists and physicians; and has continued to pioneer new technologies (including single-cell microfluidics) and new computational platforms in the ensuing decades(Reference Hood42). Perhaps most revolutionary, however, was Hood's early vision for what he first termed ‘predictive, preventive and personalised medicine’ and later renamed ‘P4 medicine: predictive, preventive, personalised and participatory medicine’(Reference Hood, Heath and Phelps43,Reference Hood44) . Relevant to the concept of personalised nutrition discussed below, there was early recognition in the systems biology field that nutrition is a critical environmental factor that interacts with genetics (and metabolism) to determine health or disease, particularly later in life(Reference Desiere45,Reference van Ommen and Stierum46) .
From the systems biology perspective, the disease is viewed as arising from either genetically and/or environmentally perturbed networks in the affected organ. Computational modelling allows the determination of how systemic networks are changing in individual cells, tissues or organisms, dynamically influencing pathophysiology of the disease. Systems medicine and systems pharmacology, considered the subfields of systems biology underpinning precision medicine(Reference Stephanou, Fanchon and Innominato47), aim to integrate genetic, clinical and omic data into network models, representing an in silico human that can yield emergent insights (Fig. 2)(Reference Moore48). Systems pharmacology is a logical extension of physiologically-based pharmacokinetic modelling, offering methods to account for genetic variation impacting whole-cell metabolism and the regulation of key drug metabolism enzymes(Reference Maldonado, Leoncikas and Fisher49). Whereas applications in pharmacology may be aimed at predicting responders/non-responders to a drug or identifying mechanisms of action underpinning drug off-target effects; equally systems approaches may be applied to predicting the response to dietary intervention given an individual's background genetics, microbiome, life stage and/or disease state (Fig. 2)(Reference Moore and Weeks38,Reference Moore48,Reference Maldonado, Fisher and Mazzatti50) .

Fig. 2. (Colour online) Systems approaches integrate genetic, clinical and ‘omic’ data into in silico models. Simulations aim to understand network dynamics and predict the response to dietary or pharmaceutical intervention accounting for an individual's genetics, lifestyle, life stage, health and/or disease state. Reprinted with permission(Reference Moore48).
Proving that systems-level integration of genetic data with clinical and multiple omic datasets is feasible and can yield personalised predictive insights and facilitate a preventative health intervention (involving nutrition) was a landmark study published in 2012(Reference Chen, Mias and Li-Pook-Than51), led by Michael Snyder, another pioneering leader in developing systems approaches to functional genomics and proteomics(Reference Schmidt52). The study combined whole-genome sequencing with transcriptomic, proteomic, metabolomic and autoantibody profiles in blood from a single individual, Professor Snyder himself, measured sequentially over a 24-month period. Apart from the significant computational feat in terms of data integration, this work was fascinating in monitoring Snyder's dynamic response to two viral infections, as well as his onset of type 2 diabetes and response to dietary and lifestyle intervention. While Snyder's elevated risk for diabetes was predicted by genome-sequence analysis, the onset of a frank high glucose and elevated glycated haemoglobin phenotype occurred about 10 months into the study and appeared to have been triggered by infection with the respiratory syncytial virus. Choosing to implement a dramatic change in diet, exercise and ingestion of low doses of acetylsalicylic acid, over the course of the following 8 months, Snyder was able to reduce his glucose and glycated haemoglobin levels to normal(Reference Chen, Mias and Li-Pook-Than51). The work uniquely characterised molecular pathways involved in both onset and resolution of viral infections and diabetes at extraordinary depth, with unique insights provided by the combination of transcriptomic, proteomic and metabolomic profiling. Other examples of multi-omic data integration in this way that have informed cancer as well as rare and common diseases have recently been reviewed(Reference Karczewski and Snyder53).
Personalised nutrition and consumer genomics
As in medicine, the meaning of personalised in the context of nutrition has been deliberated(Reference Gibney and Walsh54–Reference Gibney56); and terminology (Table 1) continues to evolve with the more recent use of the term ‘precision’ emerging in the scientific literature in the past 5 years (Fig. 3). Analogous to the ambitions of precision medicine, the aim of personalised or precision nutrition is to tailor nutritional advice/diets to optimise health based on an individual's characteristics(Reference Ordovas, Ferguson and Tai55). For a nutritionist or clinical dietitian, these characteristics have long included anthropometry, dietary history and preferences, information on lifestyle and physical activity, along with clinical parameters and biochemical markers of nutritional status. But after the sequencing of the human genome came an era of increasing research interest in nutrigenomics and nutrigenetics (Table 1 and Fig. 3), and the accompanied vision of providing personalised dietary advice to prevent diet-related diseases based on genetic differences and the predicted response to nutrients derived from genetic profiling(Reference Muller and Kersten57,Reference Trayhurn58) . Notably, while scientists have remained largely circumspect about clinical utility and the extent to which genetic or polygenic risk scores can explain overall risk for common, multifactorial diseases (e.g. obesity, diabetes, fatty liver) or micronutrient status(Reference Torkamani, Wineinger and Topol59,Reference Dib, Elliott and Ahmadi60) ; an astonishing number of direct-to-consumer (DTC) genetic testing companies have proliferated offering personalised nutrition advice to individuals based on nutrigenetic testing via the Internet(Reference Phillips61).
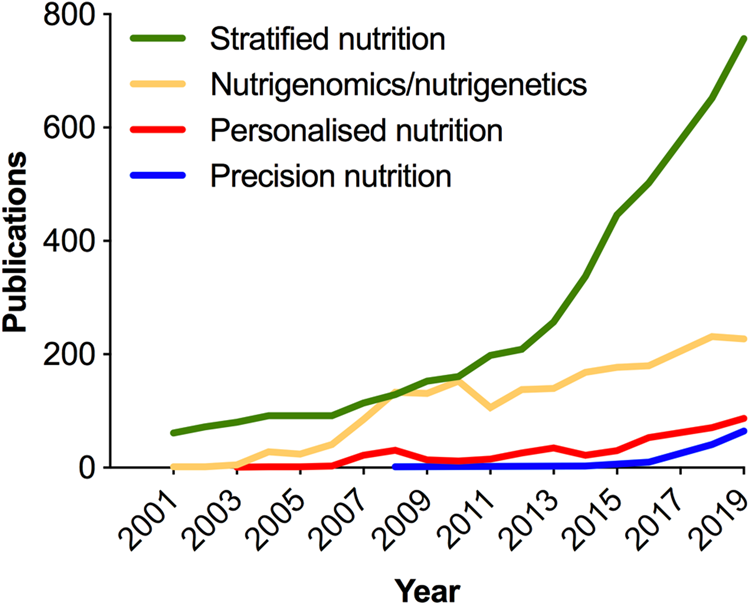
Fig. 3. (Colour online) Increase in publications in the PubMed database related to nutrigenomics and stratified, personalised or precision nutrition. In the cases of stratified, personalised and precision nutrition, terms were searched within double quotation marks, e.g. “precision nutrition”[All fields]. Personalised nutrition was searched as: “personalised nutrition” or “personalized nutrition”. Nutrigenomics/nutrigenetics was searched as: “nutrigenomics”[MeSH] or “nutrigenomics”[All Fields] or “nutrigenetics”[All Fields].
Public interest in these commercial genetic services has rapidly grown in the past 5 years. The number of genotyped consumers started rising exponentially in 2016 and surpassed 10 million worldwide at the beginning of 2018(Reference Khan and Mittelman62). The notorious, ultimately temporary, US Food and Drug Administration ban of medically-relevant testing by 23andMe in 2013 means the majority of DTC genomic tests sold to date were marketed and sold as ancestry services(Reference Torkamani, Wineinger and Topol59,Reference Khan and Mittelman62) . In addition to raising a host of ethical questions around data privacy, forensic genealogy, personal identity and race(Reference Blell and Hunter63,Reference Aldous64) , this prompted a very market-based work around the regulatory legislation for health-based genetic testing(Reference Saey65). Specifically, a crop of third-party interpretation services has arisen that will interpret raw genotyping data that are provided to consumers by many DTC ancestry genetic services without having done the testing per se (Reference Saey65,Reference Tandy-Connor, Guiltinan and Krempely66) . Separately, in a much criticised reversal, in 2017, the US Food and Drug Administration approved a 23andMe genetic health risk test of limited clinical sensitivity (limited positive and negative predictive values)(Reference Wynn and Chung67). Moreover, a significant number of companies are marketing ‘health and wellness insights’ that are largely unregulated and relate to common (nutrition-related) disease risk(Reference Phillips61,Reference Kalokairinou, Howard and Slokenberga68) . In a survey of 246 companies offering online DNA testing, done in 2016, a majority (136) offered some form of health-related testing service(Reference Phillips61). Seventy-four companies offered nutrigenetic testing, many of which also offer tailored diet services, food supplements and/or meal plans; and thirty-eight companies offered tests for athletic ability.
There are multiple scientific concerns with the personalised nutrition promises offered by DTC nutrigenetic testing companies, given the marked absence of published studies assessing either analytical or clinical/predictive validity of these tests. A merely analytical concern is the reliability of the sequence data in the first instance. A concerning study of confirmatory testing in referrals to a clinical diagnostic laboratory found 40 % of variants in a variety of genes reported in DTC raw data to be false positives(Reference Tandy-Connor, Guiltinan and Krempely66). In terms of predictive validity, the majority of genetic risk estimates returned by DTC companies are based on only a select number of genetic variants. This is in contrast to the numerous (>100) genetic loci identified by the largest (>100 000 individuals) GWAS done to date, which still only explain a fraction (20 % or less) of the heritability of common diet-related chronic diseases such as obesity and type 2 diabetes(Reference Locke, Kahali and Berndt69,Reference Fuchsberger, Flannick and Teslovich70) . Moreover, very recently, completely novel genome-wide polygenic risk scores (GPRS) have been developed for obesity, type 2 diabetes and other common diseases; facilitated by improved algorithms and very large GWAS(Reference Khera, Chaffin and Aragam71,Reference Khera, Chaffin and Wade72) . In the case of obesity, the GPRS comprised 2⋅1 million common genetic variants and significantly outperformed a score that incorporated only the 141 independent variants that had reached genome-wide levels of statistical significance in the prior GWAS(Reference Locke, Kahali and Berndt69,Reference Khera, Chaffin and Wade72) . A 13 kg gradient in weight and a 25-fold gradient in risk of severe obesity were observed in adults across GPRS deciles. Although practical considerations on how such a GPRS might be implemented and inform interventions for obesity prevention remain(Reference Torkamani and Topol73); and methodological and clinical utility questions have been raised(Reference Curtis74) about an equally novel GPRS for coronary artery disease(Reference Khera, Chaffin and Aragam71). Nonetheless, these GPRS studies call into question any DTC genetic test and personalised nutrition advice around body weight made on a handful of SNPs.
Related to nutrition status, and equally suspect in terms of predictive validity, is personalised nutrition advice from multiple companies claiming to help consumers maintain healthy levels of vitamins, antioxidants and minerals, on the basis of a handful of genetic variants. In contrast to obesity and type 2 diabetes, to date much fewer loci have been associated with the biomarkers of micronutrient status(Reference Dib, Elliott and Ahmadi60). These explain only a small fraction of variance in micronutrient status. Moreover, not all vitamins and minerals have been studied, and there are no data examining response to intake/supplementation. Perhaps even more relevant for the concept of personalised nutrition beyond the much debated ‘missing heritability’(Reference Genin75) is that both micronutrient status and the risk for many common diseases are only partially determined by genetics; with the environment playing a critical and often dominant role. Similar to the heterogeneity observed in response to pharmaceutical agents in clinical trials, human subjects are inherently variable in their responses to food and nutrient/dietary interventions(Reference Gibney56,Reference Zeevi, Korem and Zmora76,Reference Drew77) . Beyond genetics, inter-individual variation in a host of factors (sex, habitual dietary habits, physical activity, epigenetics, gut microbiome) affects an individual's absorption, distribution, metabolism and excretion of dietary compounds and metabolites(Reference de Roos and Brennan78).
Wearables and digital health
In addition to advances in multi-omic technologies, the miniaturisation of electronic devices in the past decade in particular has heralded tremendous innovation in, and adoption of, mobile technologies, sensors and wearable devices. Globally, smartphone (considered mobile computing devices) usage increased by 40 % between 2016 and 2020, and an estimated 45 % of the world's population now owns one(Reference Turner79). Worldwide revenue for the wearable tech industry was estimated at $23 billion in 2018 and is anticipated to reach $54 billion by 2023(80). The so-called wearables now permit individuals to track a multitude of parameters including diet, physical activity and sleep; and physiological measurements such as heart rate, body temperature, blood pressure, oxygen saturation and glucose levels(Reference Yetisen, Martinez-Hurtado and Unal81). Although heart rate monitors for exercise have existed since the early 80s, the first clip-on accelerometer activity tracker, the Fitbit, appeared on the market in 2007. By 2013, Fitbit (and other companies) had released a wristband tracker capable of measuring sleep as well as activity.
Since then there has been a market explosion of DTC wearables and medical devices, along with associated apps, aimed at encouraging individuals to actively participate in their own health/wellness behaviour change or disease management(Reference Yetisen, Martinez-Hurtado and Unal81,Reference Dias and Paulo Silva Cunha82) . These have included most recently smartwatches capable of taking an electrocardiogram reading with an accompanying app running a Food and Drug Administration-approved algorithm for recognition of atrial fibrillation(Reference Isakadze and Martin83). By 2015, there were more than 500 different healthcare-related wearables available facilitating real-time data collection of lifestyle and physiological measurements both by individuals and for research(Reference Li, Dunn and Salins84,Reference Witt, Kellogg and Snyder85) . In addition to the application of new technologies for dietary assessment(Reference Forster, Walsh and Gibney86), of particular relevance to personalised nutrition and the goal of prevention of diet-related diseases, has been the improvements in wearable devices for continuous glucose monitoring (CGM). In DTC fashion, data may now be released to a user's phone and sensors can now be worn for up to 2 weeks. This lengthening of sensor life has greatly facilitating recent research efforts using CGM, which have underscored the remarkable high level of variability between people in response to the same meals(Reference Zeevi, Korem and Zmora76,Reference Hall, Perelman and Breschi87) .
In a notable study for computationally driven personalised nutrition, Zeevi et al. developed a predictive algorithm for postprandial glycaemic response through profiling an 800-person Israeli cohort without diabetes who underwent CGM for 7 d, while recording food intake, activity and sleep in real-time via their mobile devices(Reference Zeevi, Korem and Zmora76). The machine learning algorithm integrated gut microbiome data derived from 16S rRNA metagenomics profiling, as well as blood parameters, anthropometrics, dietary intakes, activity and CGM data profiled over the week in the development cohort and first validated in an independent cohort of 100 individuals. The algorithm's predictions for glycaemic responses correlated significantly better to the CGM measured responses than carbohydrate counting (correlation, R = 0⋅71 v. 0⋅38) or energetic counting (R = 0⋅33) models often utilised; a result that has now been replicated in independent American populations(Reference Mendes-Soares, Raveh-Sadka and Azulay88,Reference Mendes-Soares, Raveh-Sadka and Azulay89) . Lastly, in a smaller randomised trial in twenty-six individuals, it was shown that the algorithm could accurately predict good and bad diets. In a 1-week crossover design, participants had lower glycaemic responses and favourable changes in the composition of their gut microbiomes in response to their predicted good diet in comparison to a week on the bad diet.
Although the interpretation of the high interindividual variability in glycaemic response observed by Zeevi et al. has been criticised(Reference Wolever90), multiple research studies since have also concluded that there is both high intraindividual and interindividual variation in glycaemic response to both standardised meals and mixed diets(Reference Hall, Perelman and Breschi87,Reference Matthan, Ausman and Meng91,Reference Meng, Matthan and Ausman92) ; with implications for the often debated concepts of glycaemic index and glycaemic load(Reference Meng, Matthan and Lichtenstein93,Reference Vega-Lopez, Venn and Slavin94) . Notably, the work by Hall et al. also applied a data-driven approach to CGM defining ‘glucotypes’ based on how variable the glycaemic responses were in aggregate overtime for fifty-seven healthy participants with no diagnosis of diabetes (on screening five met criteria for type 2 diabetes and fourteen had prediabetes). They show a relationship between their novel machine learning classification (low, moderate, severe) of glucose variability and clinical measures of aberrant glucose metabolism. Where severe glycaemic variability correlated with higher values for fasting glucose, oral glucose tolerance test glycated haemoglobin and the steady-state plasma glucose test for insulin resistance. Similar to the work by Zeevi et al., they also demonstrated tremendous heterogeneity in the glycaemic responses to three standardised meals of either bread and peanut butter, a protein bar or cornflakes and milk. While the expected relationship between carbohydrate/fibre content of the meals and severity of glycaemic response was observed (cornflakes conspicuously producing a ‘severe’ response for 80 % of participants), for each meal there were high and low responders in terms of blood glucose spikes. The authors show that even among their normoglycaemic participants, those classed with a ‘severe glucotype’ had glycaemic responses in prediabetic and diabetic ranges 15 and 2 % of the time. However, whether these individuals are at an increased risk for developing diabetes or other metabolic diseases requires long-term follow-up studies, as does the investigation of the utility of CGM for early-risk detection.
A critical question for public health is whether or not insights from ‘big data’ generated from wearables and multi-omic profiling can empower individuals to behavioural change. Two other recent studies, remarkable for their scope of phenotyping and big data analyses orchestrated, suggest that, at least in an intervention setting, changes with health benefits can be motivated(Reference Price, Magis and Earls95,Reference Schussler-Fiorenza Rose, Contrepois and Moneghetti96) . The first of these, the Pioneer 100 Wellness Project, was the realisation of Leroy Hood's aforementioned vision of P4 medicine(Reference Price, Magis and Earls95). Here, 108 individuals had their whole genome sequenced and were followed for a 9-month period with daily activity tracking and extensive clinical testing along with the analyses of their metabolomes, proteomes and microbiomes. Significantly, participants also received monthly behavioural coaching on ‘actionable possibilities’ based on their profiles to improve their individual health via diet, exercise, stress management, dietary supplements or doctor referral as necessary. Longitudinal improvement in a host of clinical analytes related to nutrition, diabetes, CVD and inflammation were observed. The second study was an extension of Michael Snyder's self-piloted systems approach to 109 individuals at risk for type 2 diabetes(Reference Schussler-Fiorenza Rose, Contrepois and Moneghetti96). Participants' genomes were whole exome sequenced and participants were followed prospectively with multi-omic profiling done quarterly for up to 8 years (median, 2⋅8 years) along with CGM and activity monitoring. Again, unique insights into temporal changes in molecular physiology were made along with ‘actionable health discoveries’ for participants, and 81 % reported some change in their diet and exercise habits.
Conclusions
The past two decades have brought unprecedented advances in omics, wearables and digital technologies. Undoubtedly, systems integration of multiple technologies has generated mechanistic insights and informed the evolution of precision medicine and personalised nutrition. These have prompted the recent launching of the most ambitious precision medicine cohort study to date, the All of Us Research Program, which aims to collect genetic and health data (utilising electronic health records and digital health technology), along with biospecimens for biomarker analyses, from at least one million diverse individuals in the USA(97). Nonetheless, work to date has been limited to the ground-breaking discovery studies led by a few elite research groups, and significant research and societal challenges yet need to be overcome prior to widespread adoption in clinical and public health settings(Reference Prosperi, Min and Bian98,Reference Wang and Hu99) . Considerable data integration and methodological issues in the study design must be addressed. In addition to issues around data dimensionality reduction, data storage, handling and sharing, there are complex challenges regarding study design, analytical assumptions and statistical validation(Reference Misra, Langefeld and Olivier100). Prediction modelling is suspect to algorithmic bias, black box issues, confounders and the fundamental problem of causal inference(Reference Prosperi, Min and Bian98).
In addition, pertinent ethical issues involve who can access new technologies, and how commercial companies are storing, using and/or re-mining consumer data. Substantial questions about efficacy in terms of long-term behavioural change and health outcomes remain. Related concerns are those of overdiagnosis in healthy individuals(Reference Vogt, Green and Ekstrom101), cost-benefit and impacts on health inequalities. Dietary and lifestyle choices are influenced by a broad range of socioeconomic factors including income, education, social networks and the built environment(Reference Moore and Boesch102). Tackling diet-related disease requires close scrutiny of the social determinants of food environments and population-wide, public health policies aimed at reducing health inequalities(Reference Moore and Fielding103). Ultimately, financial investment in the future of precision medicine and digital health must be balanced with limited resources available for public health initiatives.
Acknowledgements
The author would like to dedicate this manuscript to Professor Robert J. Cousins who was at the forefront of early applications of functional genomics in the Nutritional Sciences(Reference Cousins104), and fostered her early fascination in nutrigenomics. He has remained a source of scientific inspiration and mentorship in the decades since. The author is also grateful to Professor John Blundell for critical reading of the manuscript in its final stages. Finally, the author would like to thank her students past, present and future, for whom she wrote this manuscript.
Financial Support
Some of the work reviewed here from J. B. M. was made possible through funding from the UK Biotechnology and Biological Sciences Research Council, including a studentship grant (BB/J014451/1 for Dr Elaina Maldonado) and the project grant (BB/I008195/1). The support of both the University of Surrey and the University of Leeds is also acknowledged.
Conflict of Interest
None.
Authorship
The author had sole responsibility for all aspects of preparation of this manuscript.