INTRODUCTION
Cases of campylobacteriosis in New Zealand have been increasing steadily in the last decade, peaking at 379 cases/100 000 people per annum in 2006 [1]. This situation has been accompanied by a considerable amount of media attention with public calls for urgent action to reduce the unacceptable burden of human disease [Reference Baker2]. Internationally rare clones of Campylobacter could be isolated during an epidemic [Reference McTavish3], but their role in the long-term occurrence of disease remained unclear. The complex epidemiology of campylobacteriosis has hampered the development of successful control programmes for this pathogen in New Zealand and elsewhere. Campylobacter spp. have been isolated from a wide range of food sources including poultry [Reference Chrystal4, Reference Snow5], red meat [Reference Wong6] and milk [Reference Gillespie7], as well as environmental sources such as wild birds [Reference French8] and contaminated water [Reference Savill9]. In addition a variety of risk factors for acquiring campylobacteriosis, such as consumption of offal and dog-ownership have previously been identified [Reference Gillespie10, Reference Stafford11].
The ecological situation in New Zealand is unique: there is extensive agricultural usage of land and the islands are geographically remote, resulting in human, animal and pathogen populations that are relatively isolated. The country has among the highest enteric infectious disease rates in industrialized countries [Reference Lake12], and the high ratio of food-producing animals to humans, and frequent use of rural water supplies in New Zealand have been proposed as underlying causes [Reference Crump, Murdoch and Baker13]. The poultry supply is also different from many other developed countries, in that suppliers are almost entirely focused on the domestic market and for biosecurity reasons no raw poultry products are imported into the country. Despite little movement of animals and animal products into the country as a result of tight biosecurity measures, New Zealand is, however, exposed to a relatively large number of international travellers [14].
Previous studies have indicated that in New Zealand [Reference Devane15], as in other countries [Reference Oporto16], the species Campylobacter jejuni is responsible for the majority (82·6%) of human campylobacteriosis cases. C. jejuni differs in its epidemiology from other Campylobacter spp., as illustrated in a case-case comparison for C. jejuni and C. coli [Reference Gillespie17]. There is evidence that these differences also apply to different strains [Reference McCarthy18], which may affect inferences made at the species level such as the estimation of risk factors and population attributable risk. In addition, commonly used typing schemes for Campylobacter spp., such as serotyping, have struggled to provide sufficient resolution for detailed epidemiological studies based on the differences between strains. The increasing availability of genotyping methods allows for the study of transmission patterns and risk factors at the strain level, and this is changing the way epidemiologists study infectious diseases [Reference Murray19]. Multilocus sequence typing (MLST) has emerged as a genotyping technique for Campylobacter [Reference Dingle20]. It has revealed differences in the epidemiology of individual genotypes [Reference Sopwith21] and allows for the identification of source-associated strains [Reference Mullner22, Reference Sheppard23]. Based on the sequence of seven, relatively conserved, housekeeping genes, this approach has several properties, such as efficiency, accuracy, reproducibility and the opportunity to share data via an openly accessible database. These properties make it suitable as a typing tool for epidemiological investigations of genetically diverse pathogens [Reference Sullivan, Diggle and Clarke24, Reference Urwin and Maiden25]. In addition MLST has informed recently developed epidemiological and population-genetic source attribution models such as the Hald and island models [Reference Mullner26]. These models aim to apportion human cases to disease sources, and hence estimate the relative importance of each source. They are therefore a valuable tool for decision-making in food safety. Furthermore, the results from the genetic analysis performed by the asymmetric island model [Reference Wilson27] can be used to assign pathogen strains to likely sources for further molecular epidemiological analysis.
In response to the high burden of human disease in New Zealand a long-term sentinel surveillance site was established in the Manawatu region to better understand key determinants of human campylobacteriosis by combining longitudinal epidemiological surveillance and genotyping data. In this paper we investigate the epidemiology of C. jejuni infection at the genotype level using data from this site. Patterns of human cases are described and spatial and multivariable analyses are used to identify risk factors for source-associated strains. We compare the molecular epidemiology of C. jejuni in New Zealand with findings from overseas, and discuss the implications of our results for the prevention and control of this important zoonosis.
MATERIAL AND METHODS
Study population
The study was conducted within the Manawatu Health District of New Zealand's North Island. The population at the 2006 census consisted of 155 072 people [28] living in a mixture of rural and urban dwellings, including both costal and inland areas. The main urban centre is the city of Palmerston North, which has a population of about 75 000 people.
Case definition
A case was defined as a laboratory-confirmed sporadic C. jejuni case in the Manawatu Health District between 1 March 2005 and 29 February 2008. Outbreak-related cases, except the index case, were excluded. Campylobacteriosis is a notifiable disease under the Health Act 1956 in New Zealand. Data from New Zealand's national notifiable disease database EpiSurv [29] were obtained for cross-validation with notified case numbers in the study region. EpiSurv is operated by the Institute of Environmental Science and Research Ltd (ESR) on behalf of the Ministry of Health. General practitioners and public health units are required to report notified cases of campylobacteriosis to ESR for nationwide disease surveillance.
Case ascertainment
Cases were prospectively recruited via the regional diagnostic laboratory, which tests all human pathological samples from the region. Samples were tested with a commercial enzyme immunoassay that detects a Campylobacter-specific antigen, shared by C. jejuni and C. coli (ProSpecT; Remel, USA). Positive faecal swabs (Amies Charcoal transport swabs; Copan, Italy) were sent to the Hopkirk Research Institute for isolation of presumptive Campylobacter. In brief, swabs were cultured on modified cefoperazone charcoal desoxycholate agar plates (Fort Richard, NZ) and in Bolton Broth (Lab M, UK) and incubated at 42°C in a microaerobic atmosphere for 2 days. A single colony resembling Campylobacter spp. was subcultured to Columbia horse blood agar (Fort Richard) and incubated microaerobically at 42°C for 2 days before DNA preparations were made. Isolates were confirmed as C. jejuni by polymerase chain reaction. If multiple samples were collected from a single patient, only one isolate was selected.
Genotyping
After speciation, MLST of C. jejuni isolates was performed to assign isolates to a sequence type (ST) using seven housekeeping genes: aspA (aspartase A), glnA (glutamine synthase), gltA (citrate synthase), glyA (serine hydroxymethyltransferase), pgm (phosphoglucomutase), tkt (transketolase) and uncA (ATP synthase α-subunit) based on the method outlined by Dingle et al. [Reference Dingle20].
Epidemiological surveillance
Three years of human data were acquired; these were initially collected using routine public health service methods which were then superseded by a sentinel surveillance approach with data collection targets. Case information between February 2005 and June 2006 was acquired using both mailed questionnaires and telephone interviews. To enhance the quality of the surveillance data gathered, from 1 July 2006 notified cases of campylobacteriosis were contacted by telephone with a target of 95% of cases interviewed and 95% of datasets completed. The interviews were conducted using the standard structured EpiSurv case report form used for enteric diseases in New Zealand [Reference Lake, Whyte and Kliem30]. The questionnaire gathered demographic variables, risk factor information for variables within the incubation period, as well as case characteristics. Investigators were trained to ensure consistency and standardization of interviews.
Data handling and statistical analysis
The epidemiological dataset was merged with the genotyping information in an Access database, which was linked into a Bionumerics database for analysis. Data were quality controlled and validated by techniques such as consistency check, range check and batch totals. R, version 2.7.0, was used for statistical analysis [31]. Distributions were compared using χ2 tests and Fisher's exact test for count data.
All STs isolated from human cases were assigned a probability that they were acquired from individual animal reservoirs using the asymmetric island model [Reference Wilson27]. Data for this assignment were collected from parallel structured studies at the sentinel surveillance site over the same time period [Reference Mullner22, Reference Mullner26, 32]. To attribute STs to their most likely source, we first computed the attribution probabilities of each ST to each source using the asymmetric island model. The distribution of the attribution probabilities of the most likely source of each ST (i.e. the maximum attribution probability among the sources for a given ST) was assessed. Based on this distribution, a cut-off point was determined to provide a reasonable balance between the number of STs assigned to one of the three sources, and the confidence as to their correct assignment. STs with an estimated probability of at least 60% of having originated from a certain source were assigned to that source, thereby creating four different groups of human cases: poultry-associated ST474, other poultry-associated STs, ruminant-associated STs and STs not associated with any of these sources.
The spatial location of each notified human case was provided at the meshblock level. These are the smallest regions defined for the New Zealand census, each containing between zero and 498 individuals, with a median size of 78. The spatial resolution was therefore much higher in urban areas. Urban and rural areas were defined following the Urban/Rural Profile Classification provided by Statistics New Zealand [33]. The risk of being a case of campylobacteriosis in each meshblock was described using a Bayesian hierarchical model with a Markov random field prior [Reference Besag, York and Mollié34]. Relative risk surfaces were prepared for human cases from the first three of the four groupings of STs produced by the asymmetric island model. STs not associated with a particular source were not analysed as there were insufficient data.
More precisely, the model is defined as follows. Y i,t represents the number of notified cases from a particular group of STs of campylobacteriosis in meshblock i and week t. We assumed that Y i,t∼ Poisson (n iλi), where n i is the usually resident population of meshblock i (obtained from the most recent census) and λi represents the expected risk of notification for this meshblock. We assumed that the logs of the λi follow a Gaussian Markov Random Field prior (also called a Gaussian intrinsic autoregression) in which the risk in each meshblock is assumed to be similar to the mean risk of the neighbouring meshblocks. More formally, we assumed the following full conditional distributions:

where n(i) is the set of meshblocks that are neighbours to meshblock i. For the hyperparameter κ we assumed the mildly informative and conjugate gamma-distributed prior: κ∼Gamma(1, 0·5) Several prior distributions were examined and this one produced the best balance between aiding convergence of the Markov chain and allowing sufficient flexibility in the model to ensure that the data could be well represented in the posterior.
In order to investigate the associations between covariate information and infection with poultry- and ruminant-associated strains, a multivariable logistic regression model was constructed. The binary response variable was whether or not the infection was with a poultry-associated strain vs. a ruminant strain. Multiple covariates, both numerical (such as age) and categorical (such as urban/rural living classification) were analysed using the defined source associations of strains and odds ratios (OR) were calculated where appropriate. Variables for inclusion in the final models were chosen by exploratory univariable analysis with a P value cut-off of 0·2. Final variables were chosen from all variables that met this criterion via a backwards elimination. The final model was of the form:

where age was treated as a continuous variable, and rural living was binary.
RESULTS
Description of cases
Examination of laboratory and surveillance data revealed that 774 (75%) of the 1036 swabs submitted between 1 March 2005 and 29 February 2008 could be considered ‘primary samples’ that originated in the Manawatu area. The remainder were duplicate samples, or samples from outside the study area. Of these 662 (86%) were culture positive and 581 (88%) were confirmed as C. jejuni, of which 502 (86%) provided complete MLST data. The remainder provided partial profiles or could not be resuscitated (Fig. 1). The comparison of demographic data in C. jejuni cases for which an isolate was genotyped and C. jejuni cases for which no isolate was genotyped revealed no significant difference between typed and non-typed cases (Table 1). The response rate to the questionnaire, applied to all notified cases in the Manawatu Health District, increased after the introduction of data collection targets in July 2006 from 58% to 97% with a 96–100% completion of case-report data fields. A significant difference could be observed for the distribution of gender (χ2=4·12, d.f.=1, P=0·04) before (n=207) and after (n=252) the changes to the interview process, with an increase in male respondents as a result of the enhanced surveillance. The age distribution of respondents was not significantly different between the two periods (χ2=4·15, d.f.=2, P=0·13).

Fig. 1. The number of ELISA positive (–––), culture positive (---) and genotyped (……) human samples from the Manawatu surveillance site, New Zealand, in each month between March 2005 and February 2008.
Table 1. Distribution of selected demographic characteristics in surveillance-linked C. jejuni cases for which a bacterial isolate was genotyped (typed) and cases for which no isolate was genotyped (non-typed), Manawatu Health District, New Zealand 2005–2008

* P value from a χ2 test.
† Sex was unknown for two cases.
‡ Sex was unknown for one case.
Incidence
A total of 971 cases from the Manawatu region were notified to the national notifiable disease database EpiSurv during the study period compared to 774 cases included in the laboratory recruited study. The number of cases reflected the number of notifications in each week with only minor aberrations (data not shown). Case numbers increased during each southern hemisphere summer, with an additional peak of cases in winter in 2006. Annual notification rates (per 100 000 population) in the region for the 3-year period were 206 for the first year, 232 for the second and 188 in the final year of the study.
Distribution and source association of genotypes
A total of 51 different MLST genotypes were detected in our study. Seven STs each accounted for more than 20 cases and amounted to a total of 66% of all cases (Table 2). The most dominant genotype was ST474 accounting for the largest proportion of human cases in most quarters, contributing a total of 154 cases (30·7%) over the study period. Other major genotypes were ST48 and ST45, which could be isolated from 8·4% and 8·2%, respectively, of human cases. Common STs, occurring in over 20 cases, were STs 53, 50, 190 and 354. A total of 23 STs were poultry-associated including STs 474, 45, 48, 190 and 354. Sixteen STs were associated with ruminant sources including STs 61, 38, 42 and 2026 (Table 2).
Table 2. Frequency and source association of human C. jejuni MLST genotypes, Manawatu Health District, New Zealand, 2005–2008

* Source association was determined by applying the island model.
Risk factor analysis
Of the 502 cases typed using MLST 459 (90·4%) could be linked to a notified case of campylobacteriosis and thus provided the dataset for the risk factor analysis. A total of ten cases associated with overseas travel were excluded, as well as one case with missing information on key risk factors, bringing the total number of cases in the dataset to 448. This included 141 ST474 cases, 209 cases with other poultry-associated STs, 70 cases with ruminant-associated STs and 28 cases which could not be assigned to a probable source. The genotype distribution between linked and unlinked cases was compared using a χ2 test and no significant differences between the two groups could be observed (χ2=58·98, d.f.=51, P=0·21). The age distributions for ruminant- and poultry-associated strains are shown in Figure 2. Ruminant-associated strains were relatively more common in cases in children aged <10 years compared to adults. In contrast the age distribution of poultry-associated strains was more even across age categories, with no obvious association with children. This difference in age profiles for ruminant- compared to poultry-associated cases was significant (χ2=6·48, d.f.=1, P=0·011). Figure 2 also shows the age distributions for both poultry- and ruminant-associated cases divided into rural and urban residency. In rural areas ruminant-associated cases were relatively more common in younger children, while in urban areas ruminant cases were much more evenly distributed across all age groups.

Fig. 2. (a) Age distribution of cases associated with poultry (, n=351) and ruminant sources (□, n=70) in the Manawatu between March 2005 and February 2008. (b) Age distribution of urban cases associated with poultry (
, n=295) and ruminant sources (□, n=46). (c) Age distribution of rural cases associated with poultry (
, n=55) and ruminant sources (□, n=24). The error bars represent 95% confidence intervals. The comparison is within group (i.e. poultry- or ruminant-associated cases) across age categories; e.g. 29% of ruminant-associated cases were aged <10 years compared to 7% in adults aged ⩾70 years.
Table 3 shows results from the multivariable case-case analysis comparing the risk factors for infection with poultry strains compared to ruminant strains. Age and location were identified as predictors. Older people were more likely to be infected with poultry strains compared to younger people; e.g. the estimated OR of being a poultry-associated rather than a ruminant-associated case for a 65-year-old adult compared to a 5-year-old child was OR=2·1 (e0·012*60). Conversely children were therefore more likely to be infected with ruminant strains compared to adults. People in rural communities were less likely to be infected with poultry strains (OR=0·39 for rural compared to urban dwellers) and therefore more likely to be infected with ruminant strains, while the opposite was evident for people living in urban communities.
Table 3. Results of a multivariate case-case risk-factor analysis for infection with poultry strains vs. ruminant strains, Manawatu region of New Zealand 2005–2008

OR, Odds ratio; CI, confidence interval.
Spatial analysis
Figure 3 shows the relative-risk surfaces for the different ST groups. Poultry-associated cases were more prevalent in urban areas than rural areas, whereas for ruminant-associated cases the reverse was evident. There was a higher risk of notification with ST474 in and around Palmerston North, and very little increased risk in the smaller urban centre of Levin. For the other poultry-associated STs, there was a smaller increase in risk near Palmerston North, but a more significant increase in risk around the urban areas of Levin and Foxton. The relative risk for ST474 shows the most spatial variation, followed by other poultry and then ruminant STs, which exhibited the least variation of the three groups.
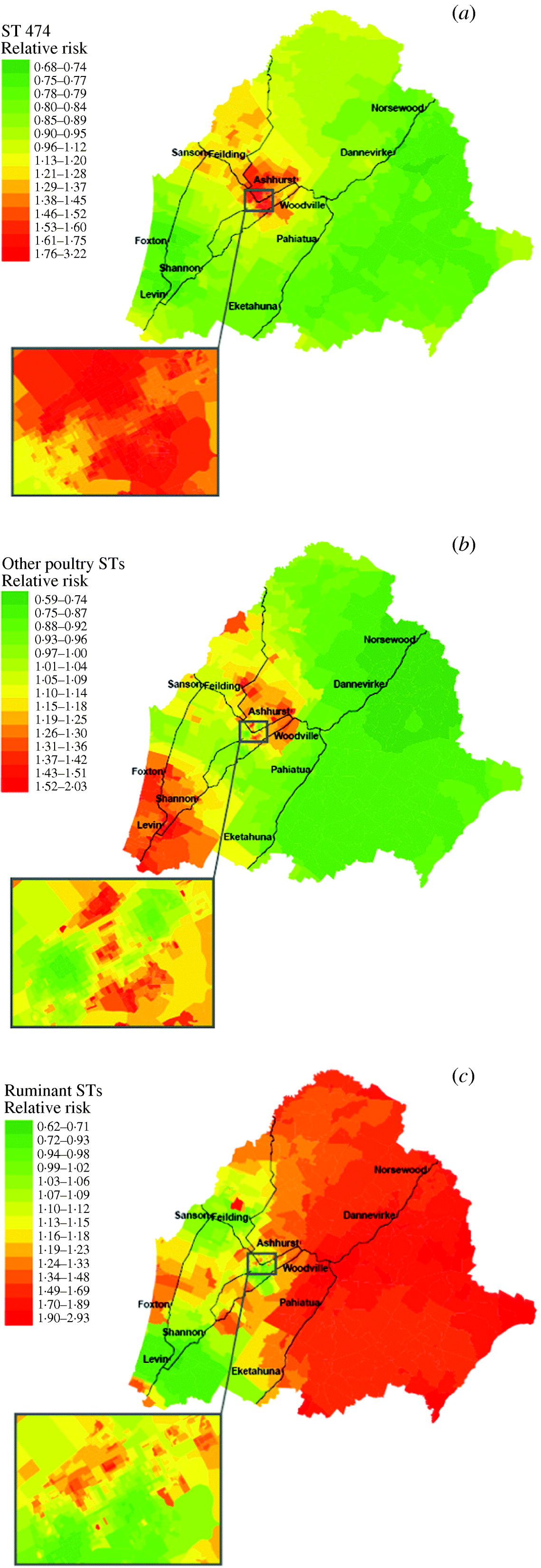
Fig. 3. (a) Relative risk surfaces of human campylobacteriosis cases of ST474, (b) other poultry-associated strains and (c) ruminant-associated strains in the Manawatu between March 2005 and February 2008. The box shows an enlargement of the city of Palmerston North.
DISCUSSION
We used data collected from a 3-year longitudinal study to explore the spatial and molecular epidemiology of C. jejuni at the genotype level, and findings from this study have influenced the development of disease control strategies for this pathogen in New Zealand [Reference Mullner26]. Through the integration of molecular and population genetic tools into epidemiological approaches new insight has been gained into the determinants of New Zealand's most important zoonosis. Our sentinel sampling strategy, gathering longitudinal data concurrent in both space and time, differs from other studies with similar objectives that have used data from different geographical regions and time periods [Reference Wilson27, Reference Strachan35]. A concentration of resources in the defined site provided a rich source of information and included advantages such as (i) standardized microbiological and epidemiological methods, (ii) high-quality data on individual cases as well as (iii) timeliness and cost-effectiveness.
An estimate of the population at risk was available in this study, allowing incidence rates of notified cases to be calculated. Manawatu Health District was an area of moderate disease rate in the study period (188–232 cases/100 000) compared to the rest of New Zealand, where in some regions and time periods more than 500 cases/100 000 population were notified [1]. From 2005 to 2007 for the whole of New Zealand notification rates ranged from 302 to 379 cases/100 000 population. Rates in our study population were above those of comparable countries such as USA (12·71/100 000), EU-25 (46·1/100 000) or Australia (111·8/100 000) [36–38]. Previous studies of the national notification system have confirmed that rates of campylobacteriosis in New Zealand are comparatively high by international comparison [Reference Baker, Sneyd and Wilson39]. The observed 2006 nationwide increase in cases during the southern hemisphere winter [Reference McTavish3] is also evident in our study as well as the expected seasonal increase in campylobacteriosis during the warmer months [Reference Nylen40].
A good correlation was observed between the number of cases statutorily notified in the Manawatu region and the number of faecal samples from primary cases submitted to the laboratory. The figures were not identical as the notified cases contained additional non-laboratory-confirmed cases associated with outbreaks; these were excluded in our case definition. This was highlighted in the data from November 2006 where the number of notifications deviated from the number of cases included in the study as a result of a major outbreak which included 26 outbreak-associated cases [Reference McTavish3]. In addition differences between notifications and study cases in each month may have been caused by a delay between laboratory confirmation and notifications of cases, as well as technical problems such as lost or unidentifiable samples, or sample submission to laboratories outside the study region. Not all cases included in the study were genotyped and the potential misclassification and differences between characteristics of patients with and without genotyped isolates present potential sources of bias. We consider, supported by a temporal analysis of the dataset, that the typed samples are representative of human cases in the region over the study period. In addition our analysis revealed no significant differences between the two groups, and we therefore consider the typed cases to be broadly representative of all notified cases. No evidence was found that cases without a linked surveillance report were different from cases with a report, and we therefore conclude that cases included in the analysis are representative of the study population.
Although we found over 50 different STs in human samples in our study, most human cases (66%) were associated with only seven different STs. These major human genotypes were generally associated with a consistent number of human cases over the time period and appear to be endemic to New Zealand. ST474 was the dominant genotype found in our study and was also the most commonly isolated genotype in the winter epidemic in 2006 in New Zealand [Reference McTavish3]. Surprisingly this ST has only rarely been reported overseas [Reference McTavish3] and besides human cases has been detected predominantly in samples from poultry in New Zealand [32]. The link between poultry and human cases of campylobacteriosis has long been identified [Reference Sheppard41] and poultry sources have recently been estimated to cause the majority of campylobacteriosis cases in New Zealand [Reference Mullner22], Scotland [Reference Sheppard23] and England [Reference Wilson27]. Although this study found a dominant clone and several other STs, such as ST2345, that are currently only found in samples collected in New Zealand, many genotypes isolated in this study can be commonly found in other countries [Reference Gormley42, Reference Sopwith43]. This includes major human pathogens such as STs 45, 48 and 53.
The spatial analysis (Fig. 3) showed that cases in urban areas were more likely to be infected with poultry-associated STs than rural areas. Conversely, cases in rural areas were more likely to be infected with ruminant-associated STs than urban areas. This supports the findings from a similar study in Scotland [Reference Strachan35] where urban and rural areas were also shown to have different epidemiological patterns. Figure 3 b shows an increased risk of poultry-associated strains around the small towns of Levin and Foxton; however, the same increase in risk was not present for ST474 cases (Fig. 3 a). This may be because ST474 is associated with one particular poultry company [Reference Mullner26] which has a relatively high market share in Palmerston North. A second company (not associated with ST474) has a processing plant near Foxton, and this may explain why there is an increased risk of non-ST474 poultry-associated cases near Levin and Foxton, either through occupational or environmental exposure or increased local market share of poultry products. The increased risk for infection with ruminants strains in rural areas (Fig. 3 c) may be associated with increased exposure to faecal matter or poorer quality drinking water in these areas [Reference Savill9, Reference Gilpin44, Reference Gilpin45]. Similarly, the observed differences in age distributions for ruminant and poultry genotypes may represent different transmission pathways, such as an increased exposure to ruminant faecal matter in young children, as well as differences in immunity between age groups [Reference Forbes46, Reference Havelaar47]. Different exposure pathways are therefore likely to exist in rural and urban communities, and this should influence the development of control strategies.
This research suggests that the high rates of campylobacteriosis in New Zealand were associated with a dominant, internationally rare clone, namely ST474, as well the presence of internationally common human genotypes such as ST45. ST474 was also the most commonly isolated genotype in the winter epidemic in 2006 in New Zealand [Reference McTavish3]. Currently, this ST has only one submission to the Campylobacter PubMLST database [Reference Dingle48] from a chicken sample in the Czech Republic, and has only been reported sporadically outside New Zealand [Reference Wilson27, Reference Best49, Reference Clark50]. If ST474 were to display phenotypic traits associated with, for example, higher virulence, this might help to explain the relatively high incidence of campylobacteriosis in New Zealand. However, there is currently only limited evidence for this: studies by Taboada and Pope indicated that some Campylobacter strains, including ST474 of New Zealand origin, may have higher virulence as measured by an invasion assay [Reference Pope51, Reference Taboada52]. Further research into pathogenicity and virulence genes of this dominant clone could reveal determinants for human infection and commensal colonization and may explain the dominance of this ST in New Zealand. The identification of ST-specific risk factors will facilitate the identification of tailor-made control programmes and by prioritizing dominant STs, more efficient control programmes can be designed.
Instead of using a network of surveillance sites this study used only one region and the importance of transmission routes is likely to vary regionally [Reference Hearnden53]. The generalization of our results from the sentinel surveillance site to the general population is supported by validation studies performed in two other regions where a similar distribution of MLST types was observed [32]. New Zealand provides a unique ecology and this is likely to have influenced the national distribution of MLST genotypes and the epidemiology of human cases [Reference Mullner26]. However, some of the results from this study are in accord with those made in a similar study in Scotland [Reference Strachan35]. These include the differences in risks of infection in rural and urban communities.
Source attribution studies from the Manawatu sentinel surveillance site [Reference Mullner22, Reference Mullner26] provided strong evidence that poultry was the leading cause of human campylobacteriosis in New Zealand, causing an estimated 54–80% of cases. In contrast, environmental sources played a relatively minor role. This is supported by the dramatic decline in human notified cases in 2008 to a 16-year low, which is a probably a consequence of poultry industry-specific interventions that were implemented based on these findings. The analysis presented here complements those results by improving our knowledge of risk factors and transmission pathways to implement more refined control strategies; for example to protect young children from disease through exposure to farm animal faecal matter in rural settings in addition to reducing transmission through poultry products in urban and rural areas.
In conclusion, the combination of molecular and epidemiological tools in a sentinel surveillance site has improved our understanding of the epidemiology of campylobacteriosis in New Zealand. The analysis of risk factors associated with MLST groupings has revealed disease characteristics that will influence the design and implementation of control measures. The results from this study underline the importance of studying Campylobacter spp. beyond the strain level. Extending this work in space and time by, for example, including data from other sites where risk factors may differ and integrating temporal components into the analysis could further improve our understanding of the commonly observed regionality and seasonality of Campylobacter infections [Reference Hearnden53].
ACKNOWLEDGEMENTS
The authors are grateful to all members of the Hopkirk Molecular Epidemiology Team, Environmental Science and Research (ESR), MidCentral Health, Public Health Services, NZFSA (Science Group), and MedLab Central (in particular Lynn Rogers) for their contributions. This work was supported by the New Zealand Food Safety Authority through their Postgraduate PhD Programme: ‘Estimating the contribution of different food sources to the burden of human campylobacteriosis in the Manawatu’. In addition financial support was provided through the New Zealand Food Safety Authority project ‘Enhancing Surveillance of Potentially Foodborne Enteric Diseases in New Zealand: Human Campylobacteriosis in the Manawatu’ (FDI/236/2005).
DECLARATION OF INTEREST
None.