Introduction
Paleobiological research practices are evolving. Advances in computational power, modeling, and databases have equipped paleobiologists with new tools to analyze the fossil record. These advances have given rise to analytical paleobiology as a research topic within paleontology. Analytical paleobiology comprises paleobiological research that uses analytical (primarily quantitative) methods, including database-driven analyses, meta-analyses, and primary data analyses (Signor and Gilinsky Reference Signor and Gilinsky1991). Although analytical methods have long been used in paleontology, analytical paleobiology crystallized in the 1970s and 1980s following pivotal computational work that examined past biodiversity dynamics (e.g., Valentine Reference Valentine1969; Raup Reference Raup1972; Raup et al. Reference Raup, Gould, Schopf and Simberloff1973; Sepkoski et al. Reference Sepkoski, Bambach, Raup and Valentine1981; Raup and Sepkoski Reference Raup and Sepkoski1982). Since then, it has matured both by adapting methods from other disciplines and by developing new methods specific to analyzing the fossil record (Raup Reference Raup1991; Liow and Nichols Reference Liow and Nichols2010; Silvestro et al. Reference Silvestro, Salamin and Schnitzler2014; Alroy Reference Alroy2020; Warnock et al. Reference Warnock, Heath and Stadler2020). Analytical paleobiology has now grown to touch most subfields within paleontology. For example, analytical tools have been used to document macroevolutionary patterns, evaluate the causes and consequences of ecosystem change, and predict biotic responses to the current biodiversity and climate crises (Condamine et al. Reference Condamine, Rolland and Morlon2013; Finnegan et al. Reference Finnegan, Anderson, Harnik, Simpson, Tittensor, Byrnes, Finkel, Lindberg, Liow, Lockwood, Lotze, McClain, McGuire, O'Dea and Pandolfi2015; Muscente et al. Reference Muscente, Prabhu, Zhong, Eleish, Meyer, Fox, Hazen and Knoll2018; Yasuhara et al. Reference Yasuhara, Huang, Hull, Rillo, Condamine, Tittensor, Kučera, Costello, Finnegan, O'Dea, Hong, Bonebrake, McKenzie, Doi, Wei, Kubota and Saupe2020). The demand for workshops on these topics, such as the Analytical Paleobiology Workshop (https://www.cnidaria.nat.uni-erlangen.de/shortcourse/index.html) and Paleontological Society Short Courses at the Geological Society of America annual meeting (https://www.paleosoc.org/short-courses), indicates that this research frontier is set to grow.
Although analytical paleobiology has been firmly established as a research topic, it continues to face challenges related to data analysis, synthesis, and accessibility. Some of these challenges are long-standing (Seddon et al. Reference Seddon, Mackay, Baker, Birks, Breman, Buck, Ellis, Froyd, Gill, Gillson, Johnson, Jones, Juggins, Macias-Fauria, Mills, Morris, Nogués-Bravo, Punyasena, Roland, Tanentzap, Willis, Aberhan, van Asperen, Austin, Battarbee, Bhagwat, Belanger, Bennett, Birks, Bronk Ramsey, Brooks, de Bruyn, Butler, Chambers, Clarke, Davies, Dearing, Ezard, Feurdean, Flower, Gell, Hausmann, Hogan, Hopkins, Jeffers, Korhola, Marchant, Kiefer, Lamentowicz, Larocque-Tobler, López-Merino, Liow, McGowan, Miller, Montoya, Morton, Nogué, Onoufriou, Boush, Rodriguez-Sanchez, Rose, Sayer, Shaw, Payne, Simpson, Sohar, Whitehouse, Williams and Witkowski2014), while others have been recently illuminated or even amplified by analytical advances (Raja et al. Reference Raja, Dunne, Matiwane, Khan, Nätscher, Ghilardi and Chattopadhyay2022). In response, many paleobiologists—particularly early-career researchers—have advocated for more collaborative, interdisciplinary, and open science. Their willingness to embrace new research practices has already begun to permeate the broader paleontological community. However, the guidelines and community buy-in that are needed to standardize these practices are still developing. As both the challenges that face analytical paleobiology and our capacity to tackle them evolve, it can be productive to monitor progress and reflect on how this research topic might continue to mature.
As one of the most recent cohorts to graduate from the Analytical Paleobiology Workshop (2019), we present this synthetic survey to signpost obstacles in analytical paleobiology from an early-career perspective and map them onto emerging solutions. We outline four interconnected challenges (Table 1), highlight recent progress, and collate a list of tools that have pushed analytical paleobiology in new directions (Supplementary Tables 1, 2). By surveying a wide range of topics, we aim to link disparate advances and provide readers with entry points for engagement with each challenge, while directing them to comprehensive discourse on each. We also echo calls for more consistent and equitable approaches to data production, synthesis, and sharing within analytical paleobiology.
Table 1. Summary of four challenges facing analytical paleobiology. Key advances are highlighted under each challenge.
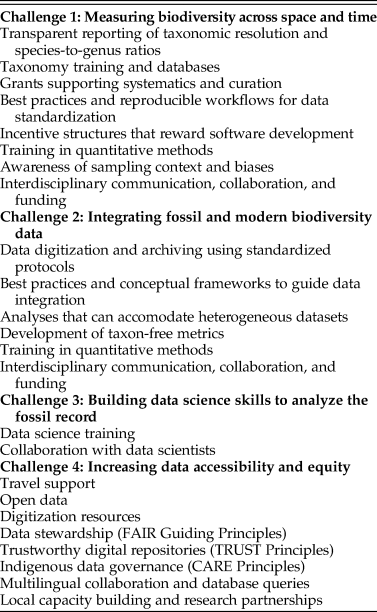
Challenge 1: Measuring Biodiversity across Space and Time
The fossil record provides an invaluable but imperfect time capsule to explore how and why biodiversity has changed over Earth's history. Early studies of deep-time biodiversity interpreted the fossil record at face value, but these interpretations are now widely documented to be confounded by a combination of geological, taphonomic, and sampling biases (Raup Reference Raup1972, Reference Raup1976; Sepkoski et al. Reference Sepkoski, Bambach, Raup and Valentine1981; Benton Reference Benton1995; Smith and McGowan Reference Smith and McGowan2011; Walker et al. Reference Walker, Dunhill and Benton2020). These biases can distort biodiversity estimates and hinder meaningful comparisons of fossil assemblages across space and time (Close et al. Reference Close, Benson, Alroy, Carrano, Cleary, Dunne, Mannion, Uhen and Butler2020a; Benson et al. Reference Benson, Butler, Close, Saupe and Rabosky2021). In recent years, quantitative methods have accrued to alleviate some of these limitations, improving our ability to quantify true biodiversity patterns (Supplementary Table 2). However, researchers now face the challenge of creating transparent, reproducible workflows to navigate this landscape of resources as they prepare their raw data for analysis (Fig. 1). Here, we focus on four aspects of this workflow: taxonomic resolution, sampling standardization, spatial standardization, and time series analysis.
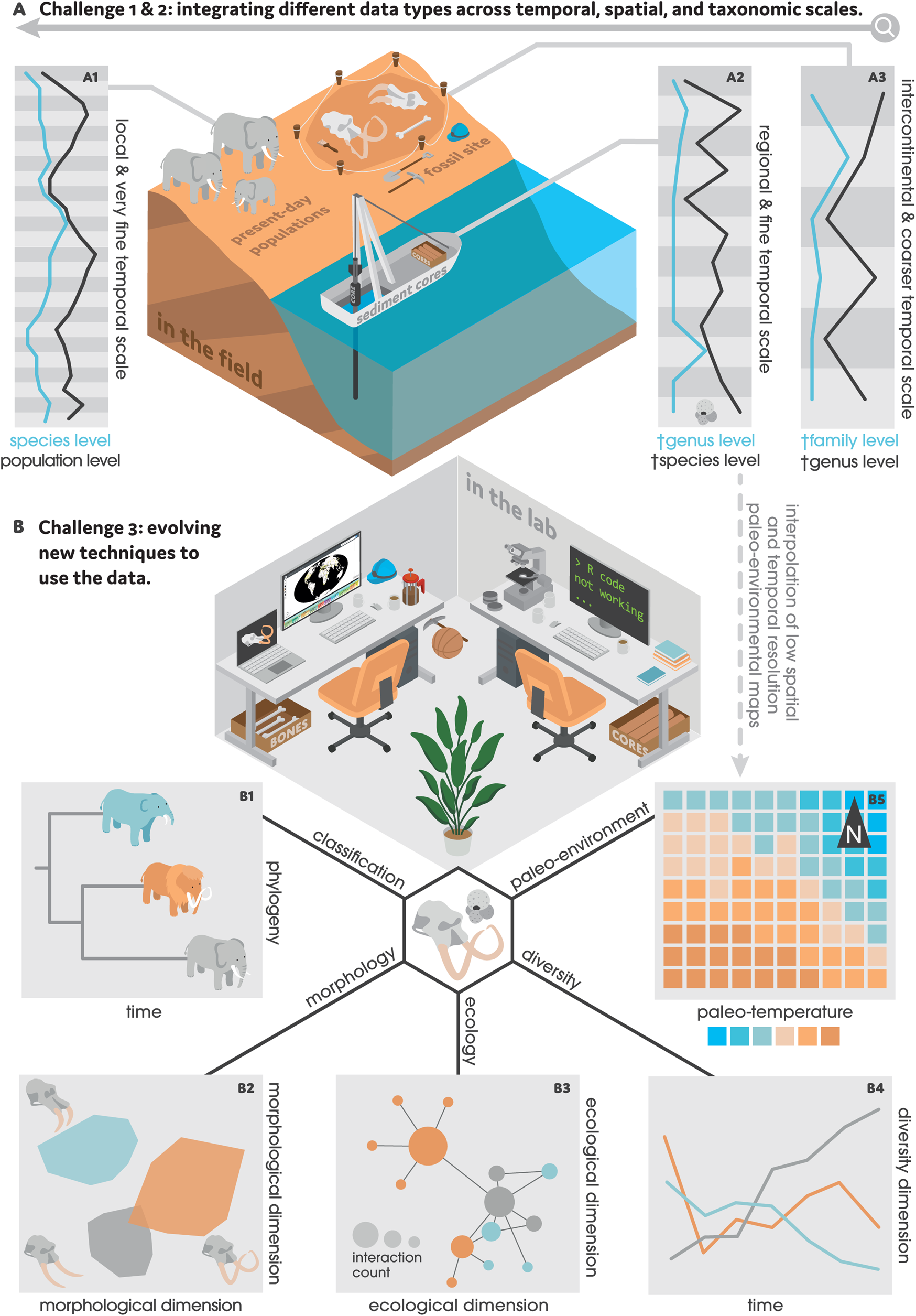
Figure 1. A, the interpretation and integration of different data types pose two major challenges in analytical paleobiology given their contrasting properties and scales. Moving from fine to coarse: A1, real-time monitoring data—indicated here by elephants—often having a very fine temporal (days, months), spatial (localities, sites), and taxonomic (populations, species) resolution; A2, microfossil data—often recovered from marine sediment cores and represented here by a Globigerina foraminifer fossil—having a fine temporal (thousands of years), spatial (basins), and taxonomic (species, genera) resolution; and A3, macrofossil data—indicated here by fossil remains from mammoth and Deinotherium—having a coarser temporal (millions of years), spatial (continents, worldwide), and taxonomic (genera, families) resolution. Microfossil, pollen, and geological data can also produce interpolated paleoenvironmental maps with low temporal (stages, periods) and spatial (km2) resolution (B5). B, to overcome these challenges, paleobiologists are developing quantitative approaches that use computer programming languages, software, and online databases. The scope of these analyses is vast, including but not limited to: B1, reconstructing phylogenetic relationships; B2, visualizing morphological differences among taxa; B3, quantifying biotic interactions (e.g., using ecological networks); B4, calculating diversity dynamics; and B5, pairing paleoenvironmental patterns with taxon occurrences to model ecological niches through time.
Estimates of taxonomic diversity are influenced by the resolution at which specimens are identified. Deep-time biodiversity patterns have long been quantified using counts of higher taxa, such as families (Sepkoski Reference Sepkoski1981; Labandeira and Sepkoski Reference Labandeira and Sepkoski1993) or genera (Sepkoski Reference Sepkoski1997; Alroy et al. Reference Alroy, Aberhan, Bottjer, Foote, Fürsich, Harries, Hendy, Holland, Ivany, Kiessling, Kosnik, Marshall, McGowan, Miller, Olszewski, Patzkowsky, Peters, Villier, Wagner, Bonuso, Borkow, Brenneis, Clapham, Fall, Ferguson, Hanson, Krug, Layou, Leckey, Nürnberg, Powers, Sessa, Simpson, Tomašových and Visaggi2008; Cleary et al. Reference Cleary, Benson, Evans and Barrett2018). Genera are often preferred, because they are typically easier to identify, more robust to stratigraphic binning, and more taxonomically stable than fossil species (Allmon Reference Allmon1992; Foote Reference Foote2000), such that they are considered to be a good substitute for biodiversity (Jablonski and Finarelli Reference Jablonski and Finarelli2009). However, genera are not perfect proxies for species, which are more directly shaped by evolutionary and ecological processes (Hendricks et al. Reference Hendricks, Saupe, Myers, Hermsen and Allmon2014). Nor are they immediately comparable with ecological data, which are often collected at the species level and are increasingly delineated using genetics (Pinzón et al. Reference Pinzón, Sampayo, Cox, Chauka, Chen, Voolstra and LaJeunesse2013; Zamani et al. Reference Zamani, Fric, Gante, Hopkins, Orfinger, Scherz, Bartoňová and Pos2022) (Fig. 1A). Authors have therefore called for greater transparency when analyzing genus-level patterns (e.g., justifying the use of genera as well as reporting species-to-genus ratios) and discussing their implications for species (Hendricks et al. Reference Hendricks, Saupe, Myers, Hermsen and Allmon2014). At the same time, the taxonomic work that underpins specimen identification remains chronically undervalued (Zeppelini et al. Reference Zeppelini, Dal Molin, Lamas, Sarmiento, Rheims, Fernandes, Lima, Silva, Carvalho-Filho, Kováč, Montoya-Lerma, Moldovan, Souza-Dias, Demite, Feitosa, Boyer, Weiner and Rodrigues2021; Gorneau et al. Reference Gorneau, Ausich, Bertolino, Bik, Daly, Demissew, Donoso, Folk, Freire-Fierro, Ghazanfar, Grace, Hu, Kulkarni, Lichter-Marck, Lohmann, Malumbres-Olarte, Muasya, Pérez-González, Singh, Siniscalchi, Specht, Stigall, Tank, Walker, Wright, Zamani and Esposito2022; although see Costello et al. Reference Costello, Wilson and Houlding2013). To preserve taxonomic knowledge, efforts could be made to invest in taxonomy courses (e.g., Smithsonian Training in Tropical Taxonomy), grants that fund curation and systematics (e.g., Paleontological Society Arthur James Boucot Research Grants), and taxonomy databases (Costello et al. Reference Costello, Wilson and Houlding2013; Fawcett et al. Reference Fawcett, Agosti, Cole and Wright2022; Grenié et al. Reference Grenié, Berti, Carvajal‐Quintero, Dädlow, Sagouis and Winter2023). Investments in systematics might, in turn, encourage stronger connections between genus- and species-level analyses when studying biodiversity through time.
Biodiversity estimates are also sensitive to sampling. In the last two decades, numerous quantitative methods have been developed to compare numbers of taxa (taxonomic richness) among assemblages while accounting for variation in sampling. Yet there is still no one-size-fits-all approach, leaving researchers to weigh the trade-offs between different methods (Close et al. Reference Close, Evers, Alroy and Butler2018; Alroy Reference Alroy2020; Roswell et al. Reference Roswell, Dushoff and Winfree2021) or use multiple complementary methods (e.g., Allen et al. Reference Allen, Wignall, Hill, Saupe and Dunhill2020). Richness estimators are a popular sampling standardization method (Alroy Reference Alroy2020). One example is shareholder quorum subsampling (Alroy et al. Reference Alroy, Aberhan, Bottjer, Foote, Fürsich, Harries, Hendy, Holland, Ivany, Kiessling, Kosnik, Marshall, McGowan, Miller, Olszewski, Patzkowsky, Peters, Villier, Wagner, Bonuso, Borkow, Brenneis, Clapham, Fall, Ferguson, Hanson, Krug, Layou, Leckey, Nürnberg, Powers, Sessa, Simpson, Tomašových and Visaggi2008; Alroy Reference Alroy2010a,Reference Alroyb,Reference Alroyc), which standardizes samples based on a measure of sample completeness, or coverage. This approach is mathematically similar to coverage-based rarefaction, which is commonly used in ecology to standardize samples when measuring species diversity (Chao and Jost Reference Chao and Jost2012; Chao et al. Reference Chao, Kubota, Zelený, Chiu, Li, Kusumoto, Yasuhara, Thorn, Wei, Costello and Colwell2020, Reference Chao, Henderson, Chiu, Moyes, Hu, Dornelas and Magurran2021; Roswell et al. Reference Roswell, Dushoff and Winfree2021). Other popular methods focus on macroevolutionary rates (e.g., origination and extinction). These range from relatively straightforward equations (Kocsis et al. Reference Kocsis, Reddin, Alroy and Kiessling2019) to more complex Bayesian frameworks (PyRate; Silvestro et al. Reference Silvestro, Salamin and Schnitzler2014) and models that incorporate phylogenetic information (fossilized birth–death process; Heath et al. Reference Heath, Huelsenbeck and Stadler2014; Warnock et al. Reference Warnock, Heath and Stadler2020). Ecological methods, such as capture–mark–recapture (Liow and Nichols Reference Liow and Nichols2010), can also be used to infer biodiversity dynamics from incomplete samples but have not been as widely applied in paleobiology. The diversity of available methods underscores the complexity of measuring biodiversity but also presents an opportunity to establish best practices that fine-tune their usage. As consensus forms, paleobiologists and ecologists could collaborate to consolidate sampling standardization methods across disciplines (Challenge 2).
Although sampling standardization corrects for differences in sample completeness, it does not consider the geographic distribution of samples. Biodiversity patterns in the fossil record have traditionally been interpreted at global scales, yet these inferences are affected by the fossil record's spatial structure (Bush and Bambach Reference Bush and Bambach2004; Vilhena and Smith Reference Vilhena and Smith2013; Close et al. Reference Close, Benson, Saupe, Clapham and Butler2020b). If spatial variation in sampling is not addressed, apparent changes in biodiversity might reflect heterogeneity in depositional, environmental, or climatic conditions rather than genuine patterns (Shaw et al. Reference Shaw, Briggs and Hull2020; Benson et al. Reference Benson, Butler, Close, Saupe and Rabosky2021). Additionally, global analyses can mask local- or regional-scale variation in biodiversity (Benson et al. Reference Benson, Butler, Close, Saupe and Rabosky2021). Researchers are increasingly using spatially explicit approaches to track biodiversity changes at nested spatial scales (Cantalapiedra et al. Reference Cantalapiedra, Domingo and Domingo2018; Womack et al. Reference Womack, Crampton and Hannah2021). A variety of procedures have been developed in recent years to account for the spatial distribution of samples. Some are relatively simple metrics, such as the convex-hull area (Close et al. Reference Close, Benson, Upchurch and Butler2017) and number of occupied equal-area grid cells (Womack et al. Reference Womack, Crampton and Hannah2021). Others are more complex, such as kernel density estimators (Chiarenza et al. Reference Chiarenza, Mannion, Lunt, Farnsworth, Jones, Kelland and Allison2019), summed minimum spanning tree length (Jones et al. Reference Jones, Dean, Mannion, Farnsworth and Allison2021; Womack et al. Reference Womack, Crampton and Hannah2021), and spatial subsampling procedures (Antell et al. Reference Antell, Kiessling, Aberhan and Saupe2020; Close et al. Reference Close, Benson, Saupe, Clapham and Butler2020b; Flannery-Sutherland et al. Reference Flannery-Sutherland, Silvestro and Benton2022). Some of the newer statistical approaches have been released with reproducible code or as R packages to allow updates from community members, providing an example of how methods in analytical paleobiology might mature (Challenge 3). Next steps could include efforts to establish incentive structures for contributing to this codebase, guidelines that compare methods, and workflows that link these packages.
Many paleobiological studies aim to quantify biodiversity through time, yet such analyses are complicated by variation in the fossil record's temporal resolution and quality (Fig. 1A). Because stratigraphic sequences are irregularly arranged in time and variably time-averaged, many common approaches to time series analysis (such as autoregressive integrated moving average, or ARIMA, models) cannot be readily applied (Kidwell and Holland Reference Kidwell and Holland2002; Yasuhara et al. Reference Yasuhara, Tittensor, Hillebrand and Worm2017; Simpson Reference Simpson2018; Fraser et al. Reference Fraser, Soul, Tóth, Balk, Eronen, Pineda-Munoz, Shupinski, Villaseñor, Barr, Behrensmeyer, Du, Faith, Gotelli, Graves, Jukar, Looy, Miller, Potts and Lyons2021). Additionally, biodiversity dynamics can be scale dependent (Levin Reference Levin1992; McKinney and Drake Reference McKinney and Drake2001; Lewandowska et al. Reference Lewandowska, Jonkers, Auel, Freund, Hagen, Kucera and Hillebrand2020; Yasuhara et al. Reference Yasuhara, Huang, Hull, Rillo, Condamine, Tittensor, Kučera, Costello, Finnegan, O'Dea, Hong, Bonebrake, McKenzie, Doi, Wei, Kubota and Saupe2020) or can interact over different scales to yield emergent patterns (Mathes et al. Reference Mathes, van Dijk, Kiessling and Steinbauer2021). Recent efforts to analyze biodiversity trends have been aided by advances in geochronology and age–depth modeling that provide more robust age control as well as models of depositional processes (Tomašových and Kidwell Reference Tomašových and Kidwell2010; Kidwell Reference Kidwell2015; Tomašových et al. Reference Tomašových, Kidwell and Barber2016; Hohmann Reference Hohmann2021; McKay et al. Reference McKay, Emile-Geay and Khider2021). Progress has also been made by implementing analyses that can accommodate observations from different types of stratigraphic sequences while accounting for age-model uncertainty. In particular, generalized additive models (Simpson Reference Simpson2018), causal analyses like convergent cross mapping (Hannisdal and Liow Reference Hannisdal and Liow2018; Runge et al. Reference Runge, Bathiany, Bollt, Camps-Valls, Coumou, Deyle, Glymour, Kretschmer, Mahecha, Muñoz-Marí, van Nes, Peters, Quax, Reichstein, Scheffer, Schölkopf, Spirtes, Sugihara, Sun, Zhang and Zscheischler2019; Doi et al. Reference Doi, Yasuhara and Ushio2021), multivariate rate-of-change analyses (Mottl et al. Reference Mottl, Grytnes, Seddon, Steinbauer, Bhatta, Felde, Flantua and Birks2021), and machine learning methods (Karpatne et al. Reference Karpatne, Ebert-Uphoff, Ravela, Babaie and Kumar2019) are changing research norms from describing temporal change to estimating statistical trends and making causal inferences among paleobiological time series. These approaches are still gaining momentum but will likely become more mainstream as they are incorporated into stratigraphic paleobiology and paleoecology training programs (Birks et al. Reference Birks, Lotter, Juggins and Smol2012; Patzkowsky and Holland Reference Patzkowsky and Holland2012; Holland and Loughney Reference Holland and Loughney2021).
As we highlighted earlier, paleobiological data often require extensive cleaning and standardization before they can be meaningfully analyzed. Open-source tools are being developed to streamline this workflow (e.g., Jones et al. Reference Jones, Gearty, Allen, Eichenseer, Dean, Galván, Kouvari, Godoy, Nicholl, Buffan, Dillon, Flannery-Sutherland and Chiarenza2022), typically in the R programming environment (Supplementary Table 2). Moving forward, this ecosystem of tools might encourage more reproducible data processing workflows within analytical paleobiology (Challenge 3). Nevertheless, quantitative methods cannot mitigate all biases, particularly those influencing the extent of the sampled fossil record. For example, variation in the preservational potential or environmental types represented by samples elude simple statistical corrections (Purnell et al. Reference Purnell, Donoghue, Gabbott, McNamara, Murdock and Sansom2018; Walker et al. Reference Walker, Dunhill and Benton2020; Benson et al. Reference Benson, Butler, Close, Saupe and Rabosky2021; de Celis et al. Reference de Celis, Narváez, Arcucci and Ortega2021). Socioeconomic disparities can also exacerbate taphonomic or geological biases by fueling differences in sampling effort across countries (Amano and Sutherland Reference Amano and Sutherland2013; Guerra et al. Reference Guerra, Heintz-Buschart, Sikorski, Chatzinotas, Guerrero-Ramírez, Cesarz, Beaumelle, Rillig, Maestre, Delgado-Baquerizo, Buscot, Overmann, Patoine, Phillips, Winter, Wubet, Küsel, Bardgett, Cameron, Cowan, Grebenc, Marín, Orgiazzi, Singh, Wall and Eisenhauer2020; Moudrý and Devillers Reference Moudrý and Devillers2020; Raja et al. Reference Raja, Dunne, Matiwane, Khan, Nätscher, Ghilardi and Chattopadhyay2022) (Challenge 4). Although quantitative methods can help illuminate the potential severity of these biases, they cannot fill sampling gaps. As such, understanding the context in which samples were collected and communicating how they were interpreted will remain critical aspects of analytical paleobiology.
Challenge 2: Integrating Fossil and Modern Biodiversity Data
Studies that link data from ancient and modern ecosystems offer holistic insight into processes spanning long timescales. For example, time series of taxon occurrences and environmental conditions in the fossil record can complement real-time monitoring to disentangle drivers of community assembly (Lyons et al. Reference Lyons, Amatangelo, Behrensmeyer, Bercovici, Blois, Davis, DiMichele, Du, Eronen, Tyler Faith, Graves, Jud, Labandeira, Looy, McGill, Miller, Patterson, Pineda-Munoz, Potts, Riddle, Terry, Tóth, Ulrich, Villaseñor, Wing, Anderson, Anderson, Waller and Gotelli2016), assess extinction risk (Raja et al. Reference Raja, Lauchstedt, Pandolfi, Kim, Budd and Kiessling2021), evaluate how ecosystems respond to disturbances (Buma et al. Reference Buma, Harvey, Gavin, Kelly, Loboda, McNeil, Marlon, Meddens, Morris, Raffa, Shuman, Smithwick and McLauchlan2019; Tomašových et al. Reference Tomašových, Albano, Fuksi, Gallmetzer, Haselmair, Kowalewski, Nawrot, Nerlović, Scarponi and Zuschin2020; Dillon et al. Reference Dillon, McCauley, Morales-Saldaña, Leonard, Zhao and O'Dea2021), and inform conservation decisions (Dietl et al. Reference Dietl, Kidwell, Brenner, Burney, Flessa, Jackson and Koch2015; Kiessling et al. Reference Kiessling, Raja, Roden, Turvey and Saupe2019). However, despite becoming more intertwined over the last decade, paleontology and ecology continue to progress as separate disciplines (Willis and Birks Reference Willis and Birks2006; Goodenough and Webb Reference Goodenough and Webb2022). Here, we outline four obstacles that impede the synthesis of paleobiological and ecological data, although these extend to other multiproxy work.
A first obstacle is data acquisition. Recent years have seen advances in data archiving as well as funding for projects that aggregate fossil and modern biodiversity data. Databases and museum collections, especially when digitized (Allmon et al. Reference Allmon, Dietl, Hendricks, Ross, Rosenberg and Clary2018), have promoted data discovery (Supplementary Table 1). In turn, application programming interfaces and web interfaces have facilitated data downloads. Examples include the paleobioDB R package, which extracts data from the Paleobiology Database (Varela et al. Reference Varela, González-Hernández, Sgarbi, Marshall, Uhen, Peters and McClennen2015), and the EarthLife Consortium (https://earthlifeconsortium.org), which queries the Paleobiology Database, Neotoma Paleoecology Database, and Strategic Environmental Archaeology Database (Uhen et al. Reference Uhen, Buckland, Goring, Jenkins and Williams2021). As these tools have gained traction, there have been calls to standardize archiving and formatting protocols to increase database interoperability (Guralnick et al. Reference Guralnick, Hill and Lane2007; Morrison et al. Reference Morrison, Sillett, Funk, Ghalambor and Rick2017; König et al. Reference König, Weigelt, Schrader, Taylor, Kattge and Kreft2019; Wüest et al. Reference Wüest, Zimmermann, Zurell, Alexander, Fritz, Hof, Kreft, Normand, Cabral, Szekely, Thuiller, Wikelski and Karger2020; Heberling et al. Reference Heberling, Miller, Noesgaard, Weingart and Schigel2021; Nieto-Lugilde et al. Reference Nieto-Lugilde, Blois, Bonet-García, Giesecke, Gil-Romera and Seddon2021; Huang et al. Reference Huang, Yasuhara, Horne, Perrier, Smith and Brandão2022) as well as maintain interdisciplinary funding structures (e.g., Past Global Changes, https://pastglobalchanges.org) to ensure their future accessibility (Challenge 4).
A second obstacle stems from the practical aspects of integrating paleobiological and ecological data. Integrative analyses involve combining datasets with different units, scales, resolutions, biases, and uncertainties (e.g., paleoclimate proxies aligned with taxon occurrences; Fig. 1). These disparate data properties can hinder their inclusion in statistical models, which typically require consistent inputs that meet certain conditions (Yasuhara et al. Reference Yasuhara, Tittensor, Hillebrand and Worm2017; Su and Croft Reference Su, Croft, Croft, Su and Simpson2018). In recent years, data synthesis has been streamlined by efforts to: (1) develop analyses that can accommodate heterogeneous datasets (Challenge 3); (2) calibrate complementary methods (Vellend et al. Reference Vellend, Brown, Kharouba, McCune and Myers-Smith2013; Buma et al. Reference Buma, Harvey, Gavin, Kelly, Loboda, McNeil, Marlon, Meddens, Morris, Raffa, Shuman, Smithwick and McLauchlan2019); (3) standardize data harmonization protocols (König et al. Reference König, Weigelt, Schrader, Taylor, Kattge and Kreft2019; Rapacciuolo and Blois Reference Rapacciuolo and Blois2019; Nieto-Lugilde et al. Reference Nieto-Lugilde, Blois, Bonet-García, Giesecke, Gil-Romera and Seddon2021); and (4) support interdisciplinary work (Ferretti et al. Reference Ferretti, Crowder, Micheli, Blight, Kittinger, McClenachan, Gedan and Blight2014). As integrative analyses become more common, best practices could be formalized to describe data properties, processing workflows, and boundaries of inference (e.g., Bennington et al. Reference Bennington, Dimichele, Badgley, Bambach, Barrett, Behrensmeyer, Bobe, Burnham, Daeschler, Dam, Eronen, Erwin, Finnegan, Holland, Hunt, Jablonski, Jackson, Jacobs, Kidwell, Koch, Kowalewski, Labandeira, Looy, Lyons, Novack-Gottshall, Potts, Roopnarine, Stromberg, Sues, Wagner, Wilf and Wing2009; McClenachan et al. Reference McClenachan, Cooper, McKenzie and Drew2015; Wilke et al. Reference Wilke, Wagner, Van Bocxlaer, Albrecht, Ariztegui, Delicado, Francke, Harzhauser, Hauffe, Holtvoeth, Just, Leng, Levkov, Penkman, Sadori, Skinner, Stelbrink, Vogel, Wesselingh and Wonik2016; Lendemer and Coyle Reference Lendemer and Coyle2021). One potential path forward is through frameworks that guide the practice of integration and provide conceptual scaffolding for new analytical techniques (Price and Schmitz Reference Price and Schmitz2016; Kliskey et al. Reference Kliskey, Alessa, Wandersee, Williams, Trammell, Powell, Grunblatt and Wipfli2017; Rapacciuolo and Blois Reference Rapacciuolo and Blois2019; Napier and Chipman Reference Napier and Chipman2022).
Conceptual barriers to data integration pose a third obstacle. These barriers often arise from differences between discipline histories, research goals, or methods (Szabó and Hédl Reference Szabó and Hédl2011; Sievanen et al. Reference Sievanen, Campbell and Leslie2012; Yasuhara et al. Reference Yasuhara, Tittensor, Hillebrand and Worm2017). Process-, function-, or trait-based metrics offer a potential workaround. These metrics can help align datasets over multiple scales and identify common currencies that are grounded in ecological or evolutionary theory (Eronen et al. Reference Eronen, Polly, Fred, Damuth, Frank, Mosbrugger, Scheidegger, Stenseth and Fortelius2010; Ezard et al. Reference Ezard, Aze, Pearson and Purvis2011; Mouillot et al. Reference Mouillot, Graham, Villéger, Mason and Bellwood2013; Wolkovich et al. Reference Wolkovich, Cook, McLauchlan and Davies2014; Yasuhara et al. Reference Yasuhara, Doi, Wei, Danovaro and Myhre2016; Pimiento et al. Reference Pimiento, Griffin, Clements, Silvestro, Varela, Uhen and Jaramillo2017, Reference Pimiento, Leprieur, Silvestro, Lefcheck, Albouy, Rasher, Davis, Svenning and Griffin2020; Spalding and Hull Reference Spalding and Hull2021). This paradigm moves away from conventional attempts to explore an ecological or evolutionary process within the bounds of a single discipline, instead encouraging interaction among researchers who approach the same process from different angles. For example, resilience concepts from the ecological literature are already being applied to the fossil record (Davies et al. Reference Davies, Streeter, Lawson, Roucoux and Hiles2018; Scarponi et al. Reference Scarponi, Nawrot, Azzarone, Pellegrini, Gamberi, Trincardi and Kowalewski2022). Moving forward, we echo existing calls to improve interdisciplinary communication (Benda et al. Reference Benda, Poff, Tague, Palmer, Pizzuto, Cooper, Stanley and Moglen2002; Boulton et al. Reference Boulton, Panizzon and Prior2005; Eigenbrode et al. Reference Eigenbrode, O'Rourke, Wulfhorst, Althoff, Goldberg, Merrill, Morse, Nielsen-Pincus, Stephens, Winowiecki and Bosque-Pérez2007), which could help design meaningful metrics that are comparable between fossil and modern datasets.
Finally, the paleontological and ecological communities remain siloed despite their complementarity. They ask similar questions but use different terminology and tools over different timescales (Rull Reference Rull2010). Interdisciplinary networks, conferences, departments, journals, and training programs can facilitate cross talk between these disciplines. Many examples already exist that provide blueprints for future partnerships. These include the Oceans Past Initiative (https://oceanspast.org), Conservation Paleobiology Network (https://conservationpaleorcn.org), Crossing the Palaeontological-Ecological Gap meeting (https://www.cpegberlin.com) and journal issue (Dunhill and Liow Reference Dunhill and Liow2018), and the PaleoSynthesis Project (https://www.paleosynthesis.nat.fau.de). Collectively, such efforts could increase institutional support for interdisciplinary research and gradually change the culture of interdisciplinarity (Ferretti et al. Reference Ferretti, Crowder, Micheli, Blight, Kittinger, McClenachan, Gedan and Blight2014; Price and Schmitz Reference Price and Schmitz2016; Yasuhara et al. Reference Yasuhara, Tittensor, Hillebrand and Worm2017). We could also learn from other interdisciplinary work such as social-ecological systems research, which links insights across the natural and social sciences (Schoon and van der Leeuw Reference Schoon and van der Leeuw2015). Ultimately, the high buy-in from early-career researchers in these initiatives bodes well for their longevity and impact.
Challenge 3: Building Data Science Skills to Analyze the Fossil Record
Paleobiology is embracing “big data.” Not only are there more ways to collect high-resolution data (Olsen and Westneat Reference Olsen and Westneat2015; del Carmen Gomez Cabrera et al. Reference del Carmen Gomez Cabrera, Young, Roff, Staples, Ortiz, Pandolfi and Cooper2019; Goswami et al. Reference Goswami, Watanabe, Felice, Bardua, Fabre and Polly2019) and automate analyses using machine learning (Peters et al. Reference Peters, Zhang, Livny and Ré2014; Hsiang et al. Reference Hsiang, Nelson, Elder, Sibert, Kahanamoku, Burke, Kelly, Liu and Hull2018, Reference Hsiang, Brombacher, Rillo, Mleneck-Vautravers, Conn, Lordsmith, Jentzen, Henehan, Metcalfe, Fenton, Wade, Fox, Meilland, Davis, Baranowski, Groeneveld, Edgar, Movellan, Aze, Dowsett, Miller, Rios and Hull2019; Kopperud et al. Reference Kopperud, Lidgard and Liow2019; Muñoz and Price Reference Muñoz and Price2019; Beaufort et al. Reference Beaufort, Bolton, Sarr, Suchéras-Marx, Rosenthal, Donnadieu, Barbarin, Bova, Cornuault, Gally, Gray, Mazur and Tetard2022) but also new opportunities to tap into online databases (Alroy Reference Alroy2003; Brewer et al. Reference Brewer, Jackson and Williams2012) (Fig. 1B). These advances have contributed to the volume, velocity, and variety of datasets that characterize big data (LaDeau et al. Reference LaDeau, Han, Rosi-Marshall and Weathers2017). However, with this accumulating information (Supplementary Table 1) comes the need for more awareness of quantitative tools (Supplementary Table 2) and best practices for data analysis. Data science training programs paired with proactive efforts to collaborate with environmental data scientists could aid the transition toward more quantitative research.
There is a growing need for paleobiologists to learn statistical and coding skills. These skills are needed to analyze large heterogeneous datasets, implement reproducible coding practices (Nosek et al. Reference Nosek, Alter, Banks, Borsboom, Bowman, Breckler, Buck, Chambers, Chin, Christensen, Contestabile, Dafoe, Eich, Freese, Glennerster, Goroff, Green, Hesse, Humphreys, Ishiyama, Karlan, Kraut, Lupia, Mabry, Madon, Malhotra, Mayo-Wilson, McNutt, Miguel, Paluck, Simonsohn, Soderberg, Spellman, Turitto, VandenBos, Vazire, Wagenmakers, Wilson and Yarkoni2015; Lowndes et al. Reference Lowndes, Best, Scarborough, Afflerbach, Frazier, O'Hara, Jiang and Halpern2017), and streamline analytical workflows (Wilson et al. Reference Wilson, Bryan, Cranston, Kitzes, Nederbragt and Teal2017; Bryan Reference Bryan2018) (Challenges 1 and 2). Training could take the form of community-based discussions (Lowndes et al. Reference Lowndes, Froehlich, Horst, Jayasundara, Pinsky, Stier, Therkildsen and Wood2019) and meetups (e.g., TidyTuesday), formal courses (e.g., Software Carpentry, https://software-carpentry.org), or independent instruction through coding tutorials (e.g., Coding Club, https://ourcodingclub.github.io/course.html). Additionally, data science topics could continue to be incorporated into paleobiology degree programs or taught as stand-alone analytical paleobiology courses. These training opportunities would provide a foundation for paleobiologists to use existing quantitative methods and create new software to analyze the fossil record.
As more paleobiologists run analyses in R, Python, and other coding languages, they could benefit from engagement with data scientists as well as with other disciplines that interface with data science, such as ecology and environmental science. Building computational skills might seem daunting, but there is no need to reinvent the wheel. Tools and infrastructure already exist (Sandve et al. Reference Sandve, Nekrutenko, Taylor and Hovig2013; Michener Reference Michener2015; Hart et al. Reference Hart, Barmby, LeBauer, Michonneau, Mount, Mulrooney, Poisot, Woo, Zimmerman and Hollister2016; Lowndes et al. Reference Lowndes, Best, Scarborough, Afflerbach, Frazier, O'Hara, Jiang and Halpern2017; Wilson et al. Reference Wilson, Bryan, Cranston, Kitzes, Nederbragt and Teal2017; Filazzola and Lortie Reference Filazzola and Lortie2022) that can be adapted to paleobiology (e.g., Barido-Sottani et al. Reference Barido-Sottani, Saupe, Smiley, Soul, Wright and Warnock2020). Working groups at synthesis centers such as the National Center for Ecological Analysis and Synthesis (which produced the Paleobiology Database) and online communities like LinkedEarth (https://linked.earth) have already begun to foster data-driven collaborations in paleontology, foreshadowing how quantitative research agendas might progress.
Challenge 4: Increasing Data Accessibility and Equity
Paleobiological data and computing resources are more accessible now than ever, but access to them is not equitable among researchers. Many financial, technological, institutional, and socioeconomic factors determine who participates in research as well as how paleobiological data are collected, interpreted, and shared (Núñez et al. Reference Núñez, Rivera and Hallmark2020; Valenzuela-Toro and Viglino Reference Valenzuela-Toro and Viglino2021) (Fig. 2). Advancing equity in the context of analytical paleobiology entails acknowledging that access to analytical resources is unequal and allocating them in relation to researchers’ needs to achieve fairer outcomes (CSSP 2019). Here, we discuss barriers pertaining to the access of paleobiological data and resources. These are by no means exhaustive but represent several broadscale challenges for which solutions have been proposed.
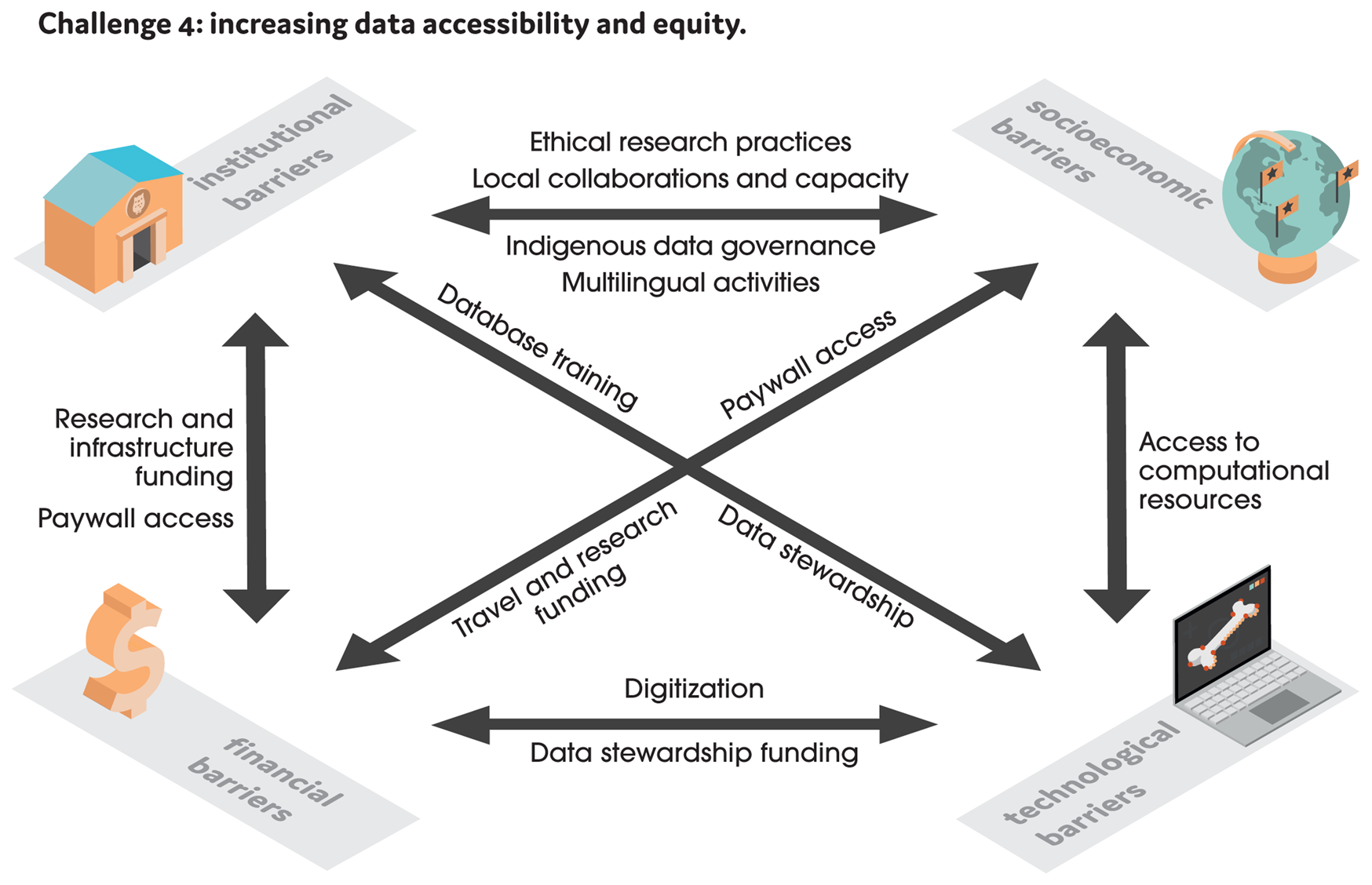
Figure 2. We identify four main barriers that hinder data accessibility and equity in analytical paleobiology: institutional (relating to museums, universities, and other research institutions), socioeconomic, technological, and financial. The arrows show relationships between these barriers and highlight where solutions are being applied.
Fossil specimens and their associated morphological, geographic, and stratigraphic information underpin research in analytical paleobiology. Data collection often involves visiting museums or gathering digital data from publications and repositories. However, these data are not always accessible. Visiting museums to study specimens can be logistically, financially, or politically infeasible—or even impossible. Travel grants (e.g., John W. Wells Grants-in-Aid of Research Program at the Paleontological Research Institution) can help offset transportation costs, but they cannot alleviate visa issues or other travel restrictions. Likewise, data underlying publications might be buried in supplementary files or locked behind paywalls or might lack consistent metadata or formatting—if they are even made available. As such, emphasis could be placed on finding alternative ways to make paleobiological data more open, particularly for researchers who historically have had less access.
One major step forward is digitization. For example, many museums have committed to digitizing their collections (Nelson and Ellis Reference Nelson and Ellis2019; Bakker et al. Reference Bakker, Antonelli, Clarke, Cook, Edwards, Ericson, Faurby, Ferrand, Gelang, Gillespie, Irestedt, Lundin, Larsson, Matos-Maraví, Müller, von Proschwitz, Roderick, Schliep, Wahlberg, Wiedenhoeft and Källersjö2020; Hedrick et al. Reference Hedrick, Heberling, Meineke, Turner, Grassa, Park, Kennedy, Clarke, Cook, Blackburn, Edwards and Davis2020; Sandramo et al. Reference Sandramo, Nicosia, Cianciullo, Muatinte and Guissamulo2021). However, only a fraction of these “dark data” have been mobilized given the substantial time, money, and effort required (Nelson et al. Reference Nelson, Paul, Riccardi and Mast2012; Paterson et al. Reference Paterson, Albuquerque, Blagoderov, Brooks, Cafferty, Cane, Carter, Chainey, Crowther, Douglas, Durant, Duffell, Hine, Honey, Huertas, Howard, Huxley, Kitching, Ledger, McLaughlin, Martin, Mazzetta, Penn, Perera, Sadka, Scialabba, Self, Siebert, Sleep, Toloni and Wing2016; Marshall et al. Reference Marshall, Finnegan, Clites, Holroyd, Bonuso, Cortez, Davis, Dietl, Druckenmiller, Eng, Garcia, Estes-Smargiassi, Hendy, Hollis, Little, Nesbitt, Roopnarine, Skibinski, Vendetti and White2018). If paleobiology continues to value digital data, financial and logistical support could be expanded for online databases and museum digitization efforts as well as resources for researchers to access those data.
Open-data practices do not end with digitization, however, as digital assets must also be maintained. In 2016, the FAIR Guiding Principles (Findability, Accessibility, Interoperability, and Reusability) for scientific data management and stewardship were published to enhance data discovery and reuse (Wilkinson et al. Reference Wilkinson, Dumontier, Aalbersberg, Appleton, Axton, Baak, Blomberg, Boiten, da Silva Santos, Bourne, Bouwman, Brookes, Clark, Crosas, Dillo, Dumon, Edmunds, Evelo, Finkers, Gonzalez-Beltran, Gray, Groth, Goble, Grethe, Heringa, ’t Hoen, Hooft, Kuhn, Kok, Kok, Lusher, Martone, Mons, Packer, Persson, Rocca-Serra, Roos, van Schaik, Sansone, Schultes, Sengstag, Slater, Strawn, Swertz, Thompson, van der Lei, van Mulligen, Velterop, Waagmeester, Wittenburg, Wolstencroft, Zhao and Mons2016). Additionally, the TRUST Principles (Transparency, Responsibility, User focus, Sustainability and Technology) were developed to demonstrate the trustworthiness of digital repositories (Lin et al. Reference Lin, Crabtree, Dillo, Downs, Edmunds, Giaretta, De Giusti, L'Hours, Hugo, Jenkyns, Khodiyar, Martone, Mokrane, Navale, Petters, Sierman, Sokolova, Stockhause and Westbrook2020). Although the biological sciences have embraced these principles, paleontology still lags behind (Stuart et al. Reference Stuart, Baynes, Hrynaszkiewicz, Allin, Penny, Lucraft and Astell2018; Kinkade and Shepherd Reference Kinkade and Shepherd2021). To encourage better data management practices, paleontological journals could require authors to archive their data, metadata, and code in centralized online repositories instead of only in supplementary files (Kaufman and PAGES 2k Special-Issue Editorial Team Reference Kaufman2018). Unique dataset identifiers could, in turn, be adopted to track data reuse and credit the authors (Pierce et al. Reference Pierce, Dev, Statham and Bierer2019). Normalizing these practices begins with data stewardship training to highlight resources (e.g., https://fairsharing.org) and community standards (e.g., Biodiversity Information Standards, https://www.tdwg.org) when managing paleobiological data (Koch et al. Reference Koch, Glover, Zambri, Thomas, Benito and Yang2018; Seltmann et al. Reference Seltmann, Lafia, Paul, James, Bloom, Rios, Ellis, Farrell, Utrup, Yost, Davis, Emery, Motz, Kimmig, Shirey, Sandall, Park, Tyrrell, Thackurdeen, Collins, O'Leary, Prestridge, Evelyn and Nyberg2018; Stall et al. Reference Stall, Yarmey, Boehm, Cousijn, Cruse, Cutcher-Gershenfeld, Dasler, de Waard, Duerr, Elger, Fenner, Glaves, Hanson, Hausman, Heber, Hills, Hoebelheinrich, Hou, Kinkade, Koskela, Martin, Lehnert, Murphy, Nosek, Parsons, Petters, Plante, Robinson, Samors, Servilla, Ulrich, Witt and Wyborn2018; Krimmel et al. Reference Krimmel, Karim, Little, Walker, Burkhalter, Byrd, Millhouse and Utrup2021).
As analytical paleobiology moves toward a future of open data, concerns regarding data ownership, representation, and control have been rekindled, particularly in relation to Indigenous communities and lands (Kukutai and Taylor Reference Kukutai, Taylor, Kukutai and Taylor2016; Jennings et al. Reference Jennings, David-Chavez, Martinez, Lone Bear Rodriguez and Rainie2018; Rainie et al. Reference Rainie, Kukutai, Walter, Figueroa-Rodriguez, Walker, Axelsson, Davies, Walker, Rubinstein and Perini2019; McCartney et al. Reference McCartney, Anderson, Liggins, Hudson, Anderson, TeAika, Geary, Cook-Deegan, Patel and Phillippy2022). In response, the CARE Principles of Indigenous Data Governance (Collective Benefit, Authority to Control, Responsibility, and Ethics) were created to complement the FAIR Guiding Principles and promote the ethical use and reuse of Indigenous data (Carroll et al. Reference Carroll, Garba, Figueroa-Rodríguez, Holbrook, Lovett, Materechera, Parsons, Raseroka, Rodriguez-Lonebear, Rowe, Sara, Walker, Anderson and Hudson2020, Reference Carroll, Herczog, Hudson, Russell and Stall2021). Methods for implementing the FAIR Guiding Principles and CARE Principles in tandem (Rainie et al. Reference Rainie, Kukutai, Walter, Figueroa-Rodriguez, Walker, Axelsson, Davies, Walker, Rubinstein and Perini2019; Carroll et al. Reference Carroll, Garba, Figueroa-Rodríguez, Holbrook, Lovett, Materechera, Parsons, Raseroka, Rodriguez-Lonebear, Rowe, Sara, Walker, Anderson and Hudson2020, Reference Carroll, Herczog, Hudson, Russell and Stall2021) should be incorporated into analytical paleobiology courses to train researchers how to work with Indigenous data and partners without perpetuating entrenched power imbalances (Liboiron Reference Liboiron2021; Monarrez et al. Reference Monarrez, Zimmt, Clement, Gearty, Jacisin, Jenkins, Kusnerik, Poust, Robson, Sclafani, Stilson, Tennakoon and Thompson2021).
Another dimension of access pertains to the language used to communicate information. Studies in analytical paleobiology rely heavily on information published in English (Raja et al. Reference Raja, Dunne, Matiwane, Khan, Nätscher, Ghilardi and Chattopadhyay2022). Although having a shared language of science can facilitate global collaboration, it also selectively excludes voices (Tardy Reference Tardy2004). For example, non-English publications are frequently omitted from data compilations, which might bias results from literature reviews (Amano et al. Reference Amano, González-Varo and Sutherland2016, Reference Amano, Rios Rojas, Boum II, Calvo and Misra2021; Nuñez and Amano Reference Nuñez and Amano2021; Raja et al. Reference Raja, Dunne, Matiwane, Khan, Nätscher, Ghilardi and Chattopadhyay2022) and meta-analyses (Konno et al. Reference Konno, Akasaka, Koshida, Katayama, Osada, Spake and Amano2020). To help alleviate language biases, researchers could conduct literature searches and disseminate their findings in multiple languages, advocate for translation or English proofing services at journals, and be considerate of non-native English speakers (Márquez and Porras Reference Márquez and Porras2020; Ramírez-Castañeda Reference Ramírez-Castañeda2020; Amano et al. Reference Amano, Rios Rojas, Boum II, Calvo and Misra2021; Gaynor et al. Reference Gaynor, Azevedo, Boyajian, Brun, Budden, Cole, Csik, DeCesaro, Do-Linh, Dudney, Galaz García, Leonard, Lyon, Marks, Parish, Phillips, Scarborough, Smith, Thompson, Vargas Poulsen and Fong2022; Steigerwald et al. Reference Steigerwald, Ramírez-Castañeda, Brandt, Báldi, Shapiro, Bowker and Tarvin2022). Creating space for multilingual collaborations in analytical paleobiology would welcome knowledge, perspectives, and skills that might otherwise be overlooked due to language barriers.
Paleontology's history has left an indelible imprint on how research in the field is conducted today, contextualizing the challenges we highlight throughout this article. Knowledge production in analytical paleobiology, like other natural sciences, depends in part on socioeconomic factors such as wealth, education, and political stability, as well as colonial legacy (Boakes et al. Reference Boakes, McGowan, Fuller, Chang-qing, Clark, O'Connor and Mace2010; Amano and Sutherland Reference Amano and Sutherland2013; Hughes et al. Reference Hughes, Orr, Ma, Costello, Waller, Provoost, Yang, Zhu and Qiao2021; Monarrez et al. Reference Monarrez, Zimmt, Clement, Gearty, Jacisin, Jenkins, Kusnerik, Poust, Robson, Sclafani, Stilson, Tennakoon and Thompson2021; Trisos et al. Reference Trisos, Auerbach and Katti2021; Raja et al. Reference Raja, Dunne, Matiwane, Khan, Nätscher, Ghilardi and Chattopadhyay2022). Consequently, sampling effort is not equally distributed across the world. For example, 97% of fossil occurrence data recorded in the Paleobiology Database over the last 30 years was generated by higher-income countries, particularly those in western Europe and North America (Raja et al. Reference Raja, Dunne, Matiwane, Khan, Nätscher, Ghilardi and Chattopadhyay2022). These socioeconomic factors intensify other geographic biases in the fossil record and warp biodiversity estimates (Challenge 1). As such, efforts to obtain a representative view of biodiversity across space and time are not disconnected from efforts to advance equity, inclusion, and ethics in analytical paleobiology. Recent publications have spotlighted actions that individuals and institutions should take to change research norms, urging our community to not only reflect on its past but forge a new path forward (Cronin et al. Reference Cronin, Alonzo, Adamczak, Baker, Beltran, Borker, Favilla, Gatins, Goetz, Hack, Harenčár, Howard, Kustra, Maguiña, Martinez-Estevez, Mehta, Parker, Reid, Roberts, Shirazi, Tatom-Naecker, Voss, Willis-Norton, Vadakan, Valenzuela-Toro and Zavaleta2021; Liboiron Reference Liboiron2021; Theodor et al. Reference Theodor, Lewis E and Rayfield J2021; Cisneros et al. Reference Cisneros, Raja, Ghilardi, Dunne, Pinheiro, Regalado Fernández, Sales, Rodríguez-de la Rosa, Miranda-Martínez, González-Mora, Bantim, de Lima and Pardo2022; Dunne et al. Reference Dunne, Raja, Stewens and Zaw2022; Mohammed et al. Reference Mohammed, Turner, Fowler, Pateman, Nieves-Colón, Fanovich, Cooke, Dávalos, Fitzpatrick, Giovas, Stokowski, Wrean, Kemp, LeFebvre and Mychajliw2022; Raja et al. Reference Raja, Dunne, Matiwane, Khan, Nätscher, Ghilardi and Chattopadhyay2022).
Conclusion
Analytical paleobiology has grown in available data, computational power, and community interest over the last half century. Notably, progress in quantitative methods, conceptual frameworks, interdisciplinary partnerships, and data stewardship has contributed to more open and reproducible paleobiological research. These advances have expanded our ability to account for biases in the fossil record, accommodate different data types in models, integrate insights across disciplines, and pursue innovative research questions. Early-career researchers in particular, despite being precarious in terms of employment and career prospects, are embracing these evolving research practices. However, there is still a need to increase their acceptance among the broader paleontological community, establish best practices, and dismantle systemic inequities in how paleobiological data have historically been generated, shared, and accessed. Fortunately, we are not alone in facing these issues, and we can learn a great deal from solutions proposed by other disciplines. Great opportunity lies in both individual and institutional action to transform the future of how we study the past.
Acknowledgments
We thank the Analytical Paleobiology Workshop organizing committee, who indirectly catalyzed this paper by bringing us together as the Class of 2019. We also thank our wonderful instructors, whose teaching and insight shaped our perspectives on the four challenges we present. We thank G. Mathes, N. Raja, and Á. Kocsis for their invaluable feedback, and K. Anderson for their insight into museum collections. We also thank W. Kiessling, M. Yasuhara, and an anonymous reviewer whose detailed comments greatly improved the article. Finally, we thank the University of California for covering the publication fees. E. M. Dillon was supported by a University of California Santa Barbara Chancellor's Fellowship. E. M. Dunne was supported by a Leverhulme Research Project Grant (RPG-2019-365). A.I. was supported by the Austrian Science Fund (FWF; P31592-B25). M.K. was supported by a Royal Society of Science Grant (RGF\EA\180318). S.V.R. was supported by the University of Calgary Faculty of Graduate Studies Eyes High Doctoral Recruitment Scholarship. This paper was composed during the COVID-19 pandemic, and the authors wish to acknowledge the widespread and profound political, economic, and personal effects that this event has had, and continues to have, on the early-career researcher community.
Declaration of Competing Interest
The authors declare no competing interest.
Data Availability Statement
Supplementary Tables are available from the Zenodo Digital Repository: https://doi.org/10.5281/zenodo.7340036.