Introduction
Forest ecosystems harbour approximately two-thirds of terrestrial biodiversity, yet few forests have escaped anthropogenic impacts (FAO 2006). Neotropical forests harbour high levels of biodiversity but are threatened by vegetation loss and degradation (Laurance et al. Reference Laurance, Clements, Sloan, O’Connell, Mueller and Goosem2014). Cultivated areas and human settlements and the construction of roads and other infrastructure result in habitat loss and fragmentation for many species and contribute to high extinction rates globally (Barnosky et al. Reference Barnosky, Matzke, Tomiya, Wogan, Swartz and Quental2011). Protected areas are key to species conservation; however, they are insufficient for the long-term conservation of some species, especially those with large home ranges (Gardner et al. Reference Gardner, Barlow, Chazdon, Ewers, Harvey, Peres and Sodhi2009).
Medium- and large-sized mammals (>1 kg body mass) play important roles in maintaining ecosystem functions; they act as landscape engineers, shaping the structure and composition of ecosystems and communities (Bakker et al. Reference Bakker, Gill, Johnson, Vera, Sandom, Asner and Svenning2016, Morris & Letnic Reference Morris and Letnic2017). However, many of these mammals have suffered population declines or extirpation due to overhunting and habitat loss and degradation (Ceballos et al. Reference Ceballos, Ehrlich and Dirzo2017, Vynne et al. Reference Vynne, Gosling, Maney, Dinerstein, Lee, Burgess and Svenning2022). Many formerly remote areas are now accessible through roads, which can increase hunting pressure, and are affected by exotic species such as dogs and domestic livestock (Cullen et al. Reference Cullen, Bodmer and Pádua2000, Laurance et al. Reference Laurance, Croes, Tchignoumba, Lahm, Alonso, Lee and Ondzeano2006, Michalski & Peres Reference Michalski and Peres2007).
The human footprint index (HFI) captures anthropogenic impacts on biodiversity and has been mapped at global (Sanderson et al. Reference Sanderson, Jaiteh, Levy, Redford, Wannebo and Woolmer2002, Venter et al. Reference Venter, Sanderson, Magrach, Allan, Beher and Jones2016) and at regional scales (Leu et al. Reference Leu, Hanser and Knick2008, Woolmer et al. Reference Woolmer, Trombulak, Ray, Doran, Anderson and Baldwin2008). The HFI is defined through certain geographical indicators, such as human population density, human settlements, roads, agricultural lands and electrical infrastructure, which are summed to calculate the HFI (Sanderson et al. Reference Sanderson, Jaiteh, Levy, Redford, Wannebo and Woolmer2002). Where the HFI is higher, anthropogenic impact is also higher and native wildlife species experience greater threats to survival or reproduction (Martinuzzi et al. Reference Martinuzzi, Radeloff, Pastur, Rosas, Lizarraga and Politi2021). Areas with HFI values equal to 0 are defined as wilderness areas, which are likely to contain the best-conserved environments with intact or nearly intact biological communities (Sanderson et al. Reference Sanderson, Jaiteh, Levy, Redford, Wannebo and Woolmer2002, Vynne et al. Reference Vynne, Gosling, Maney, Dinerstein, Lee, Burgess and Svenning2022). Wilderness areas are important for biodiversity conservation, climate stabilization and the provision of essential goods and services for human well-being (Watson et al. Reference Watson, Evans, Venter, Williams, Tulloch, Stewart and Lindenmayer2018, Grantham et al. Reference Grantham, Duncan, Evans, Jones, Beyer and Schuster2020). In summary, wilderness areas maintain ecosystem functionality, in part by supporting complete medium- and large-sized mammal assemblages (Estes et al. Reference Estes, Terborgh, Brashares, Power, Berger, Bond and Wardle2011, González-Maya et al. Reference González-Maya, Martínez-Meyer, Medellín and Ceballos2017).
Although global assessments using the HFI have been conducted at 1-km scale resolution, global-scale geographical information may be insufficient in terms of accuracy, resolution and detail at finer scales (Sanderson et al. Reference Sanderson, Jaiteh, Levy, Redford, Wannebo and Woolmer2002), where information at a finer resolution might be needed to apply human footprint measures at regional, national or local scales. Recently, the global HFI has been improved with a higher resolution (300 m), resulting in substantial improvements to the spatial interpretation of landscape transformation (Sanderson et al. Reference Sanderson, Fisher, Robinson, Sampson, Duncan and Royte2022). Additionally, regional HFIs developed at higher resolution (90 m) allowed for the inclusion of more anthropogenic variables and revealed a level of spatial heterogeneity in landscape transformation that was masked in the global analysis (Leu et al. Reference Leu, Hanser and Knick2008, Woolmer et al. Reference Woolmer, Trombulak, Ray, Doran, Anderson and Baldwin2008). Greater detail in human footprint analysis makes it particularly applicable to conservation planning at local or regional scales, at which most plans are implemented. Recently, an ecoregional-scale human footprint map was developed for the forests of Argentina at 100-m resolution to identify the most intact forest in the country (Martinuzzi et al. Reference Martinuzzi, Radeloff, Pastur, Rosas, Lizarraga and Politi2021).
The Southern Yungas are forests distributed along the eastern slopes of the Andes in north-western Argentina and southern Bolivia (Politi & Rivera Reference Politi and Rivera2019). The Southern Yungas are considered a global biodiversity hotspot that is under increasing anthropogenic threat (Myers et al. Reference Myers, Mittermeier, Mittermeier, da Fonseca and Kent2000). Approximately 30% of the original area of the Southern Yungas of Argentina has been converted to other land uses (Politi & Rivera Reference Politi and Rivera2019). The most widespread human activities in remnant forests are logging and cattle raising, and many rural communities use multiple forest resources (e.g., firewood, subsistence hunting), facilitated by logging or oil roads (Politi & Rivera Reference Politi and Rivera2019). In Argentina, the Southern Yungas harbours 157 species of mammals, of which 36 species are medium- or large-sized mammals, including several that are categorized as globally or nationally threatened (i.e., Panthera onca, Tayassu pecari, Tapirus terrestris, Myrmecophaga tridactyla) due to notable contractions of their historical distributions (SAREM 2019). Although much remains to be discovered about the anthropogenic impacts on mammals in the Southern Yungas, early work has shown that native mammal species richness might decrease with elevation (Di Bitetti et al. Reference Di Bitetti, Albanesi, Foguet, De Angelo and Brown2013), that tapirs persist on private land with greater probability near to than farther from national parks (Rivera et al. Reference Rivera, Martinuzzi, Politi, Bardavid, De Bustos, Chalukian and Pidgeon2021) and that high cattle abundance is associated with low abundance of large native herbivores (Cuyckens et al. Reference Cuyckens, Gonzalez Baffa Trasci, Perovic and Malizia2022).
Monitoring programmes are essential to detecting and quantifying population trends and assessing the influences of various threats on wild populations (Ficetola et al. Reference Ficetola, Romano, Salvidio and Sindaco2018). Monitoring populations is important not only because countries contracting to the Convention on Biological Diversity are obligated to do so, but also because if they are not monitored they are often marginalized in decision-making processes (Boutin Reference Boutin, Haughland, Schieck, Herbers and Bayne2009, Perino et al. Reference Perino, Pereira, Felipe-Lucia, Kim, Kühl and Marselle2022). The sensitivity or tolerance of medium- and large-sized mammals to human pressure probably varies with species, and therefore detecting changes over time to the probability of habitat use of these species in relation to the human footprint is valuable for determining at what point it might be necessary to mitigate negative human impacts (Fisher & Burton Reference Fisher and Burton2018, Shackelford et al. Reference Shackelford, Standish, Ripple and Starzomski2018, Toews et al. Reference Toews, Juanes and Burton2018). In the Southern Yungas, there is no monitoring of changes in forest condition, and the establishment of an early warning programme to detect population changes of medium- and large-sized mammals in wilderness and in areas experiencing anthropogenic impacts is urgently needed (Clements et al. Reference Clements, Kerley, Cumming, De Vos and Cook2019). Here, our goals are: (1) to assess how medium- and large-sized mammals respond to anthropogenic threats as measured by the HFI; and (2) to identify mammal species that could serve to help us monitor human impacts in the Southern Yungas of Argentina.
Materials and methods
Study area
Our study area includes the Southern Yungas of the provinces of Salta and Jujuy (Argentina; Fig. 1); it is bounded by the border with Bolivia to the north and by El Rey National Park to the south. We used the human footprint map generated for forests of Argentina at a 100-m resolution (Martinuzzi et al. Reference Martinuzzi, Radeloff, Pastur, Rosas, Lizarraga and Politi2021), which integrates human settlements (urban and rural settlements), transportation (roads and trails), energy infrastructure (oil and gas wells and pipelines) and land use (exotic tree plantations and deforestation). The human footprint map uses an influence score and a decay function to represent declining human influence with increasing distance, and it was calibrated based on local forest studies to better represent local conditions (see Martinuzzi et al. Reference Martinuzzi, Radeloff, Pastur, Rosas, Lizarraga and Politi2021). For example, data on the rural settlements locally known as puestos (one or a few houses clustered around an artificial water source for livestock), and caserios (clusters of a few rural houses plus a first-aid post and a small school) are not included in global maps but are regionally very important. The values in the human footprint map were obtained through Theobald’s fuzzy algebraic sum of each variable of human influence that ranges from 0 (i.e., minimum human influence, or wilderness) to 1 (maximum human influence; Martinuzzi et al. Reference Martinuzzi, Radeloff, Pastur, Rosas, Lizarraga and Politi2021). The total study area comprises c. 3.1 million ha of forest with a mean HFI value of 0.14 (range = 0–0.88), of which 1.7 million ha (54%) are wilderness (i.e., HFI value equal to 0), and the remaining 1.4 million ha (46%) are under human influence (i.e., HFI value > 0; Fig. 1).

Figure 1. Human footprint map for the Southern Yungas of the provinces of Salta and Jujuy (Argentina; Martinuzzi et al. Reference Martinuzzi, Radeloff, Pastur, Rosas, Lizarraga and Politi2021) and locations of camera traps (white circles). The left box shows the relative location of the main box in Argentina and the green shading shows the portion of the Southern Yungas that was included as the study area.
Camera trapping of medium- and large-sized mammals
Camera traps are a reliable, non-invasive and low-cost sampling tool for monitoring medium- and large-sized mammals (Burton et al. Reference Burton, Neilson, Moreira, Ladle, Steenweg and Fisher2015, Steenweg et al. Reference Steenweg, Whittington, Hebblewhite, Forshner, Johnston and Petersen2016). We placed 165 camera traps within private properties where we obtained permits to work. Within these properties, camera traps were set in continuous forests across different levels of HFI values up to 0.6, as greater values represent areas where forests have been completely transformed to other land uses (e.g., cities) and our objective was to assess mammal species in forested areas (Fig. S1). We attached camera traps to a tree at a height of 30 cm from the ground, spaced 1.62 ± 0.51 km apart and more than 100 m from crops or roads. We programmed the camera traps to take photographs during the day and night and remain active for at least 30 days. We set camera traps in groups of 10–20 camera traps at a station and then moved to another station; each station was sampled only once during May–October in 2016, 2017, 2018 or 2019, the months coinciding with the dry season in the Southern Yungas, when the properties could be accessed (TEAM Network 2011). We processed image data from camera traps using the program Wild.ID (TEAM Network 2011) and identified individuals present in the photographs to species level. We excluded records of the Dasypodidae family (armadillos) due to the complexity of taxonomic identification through photographs. In addition, we excluded records of exotic species such as cattle, dogs and horses as our objective was to determine the presence of native mammal species.
Data analysis
We constructed detection histories to determine the encounter history for each species with the independent photographs (i.e., >60 min between photographs of the same species; Sollmann Reference Sollmann2018). We calculated naïve occupancy as the sum of camera traps where each species was detected over the total number of cameras placed (MacKenzie et al. Reference MacKenzie, Nichols, Royle, Pollock, Bailey and Hines2017). Occupancy models were constructed for species with a naïve occupancy ≥0.07 (14 of 25 species) because a low number of detections results in poor occupancy model output (Einoder et al. Reference Einoder, Southwell, Lahoz-Monfort, Gillespie and Wintle2018).
We used single-species, single-season occupancy models and a sampling occasion defined as a 5-consecutive-day period of operation for each camera (MacKenzie et al. Reference MacKenzie, Nichols, Royle, Pollock, Bailey and Hines2017). The occupancy models were composed of two sub-models, one for probability of habitat use (Ψ) and one for detection (p). The detection sub-model describes the observational process used to estimate the probability of detection for each sampling occasion and camera, conditional on the presence of the species and following a Bernoulli probability distribution (MacKenzie et al. Reference MacKenzie, Nichols, Royle, Pollock, Bailey and Hines2017), whereas the probability of habitat use sub-model describes the ecological process of the probability of habitat use at each camera, also following a Bernoulli distribution. In the probability of habitat use sub-models, we used the mean value of the HFI calculated within 500 m of each camera trap as the explanatory variable to avoid overlap of values between camera traps and because this is the average home range of the medium- and large-sized mammals of interest (Maffei et al. Reference Maffei, Cuellar and Noss2002, Rovero et al. Reference Rovero, Zimmermann, Berzi and Meek2013). The variable ‘effort’ was included in the detection models and represents the total number of days for which each camera trap was active. Both variables (i.e., HFI and effort) were standardized by subtracting the mean and dividing by the standard deviation (Zipkin et al. Reference Zipkin, Andrew Royle, Dawson and Bates2010). To select the best occupancy models, we followed a secondary candidate model selection strategy (Morin et al. Reference Morin, Yackulic, Diffendorfer, Lesmeister, Nielsen, Reid and Schauber2020), in which the Akaike information criterion corrected for small samples (AICc) was used to rank the possible candidate models. For each species, the best model was that for which the difference in AICc (ΔAICc) was <2. In the case that more than one model was retained (ΔAICc < 2), we used the averaged model to define the best model. To run the analyses, we used the ‘camtrapR’ (Niedballa et al. Reference Niedballa, Courtiol, Sollmann, Mathai and Wong2020) and ‘unmarked’ (Fiske & Chandler Reference Fiske and Chandler2011) packages in R version 4.3 (R Computing Team 2023). The results from this study should be interpreted as probability of habitat use and not occupancy probability as the spacing of camera traps might be smaller than the home ranges of some of the species, therefore not ensuring spatial independence (MacKenzie & Nichols Reference MacKenzie and Nichols2004).
To assess the goodness of fit of the models for each species, we used the 1000 parametric bootstrap approach to compare the sampling distribution (Fiske & Chandler Reference Fiske and Chandler2011). In addition, the dispersion parameter (c-hat) was calculated as the ratio of the observed χ2 statistic value over the mean of the simulated distribution. The entire fitting procedure of the single-species occupancy models was performed with a maximum likelihood approach using the ‘unmarked’ package (Fiske & Chandler, Reference Fiske and Chandler2011) in R (R Computing Team 2023). We evaluated the autocorrelation of the residuals of the best models with a spatial correlogram using the ‘ncf’ package (Bjornstad Reference Bjornstad2016) because this allows for the generation of correlograms using a non-parametric significance test by means of Monte Carlo simulations (Bjornstad Reference Bjornstad2016).
Results
With 6043 camera trap-nights across all cameras, we obtained a total of 2156 independent records of 25 species that belong to 12 families of medium- and large-sized mammals (Fig. 2). Five species (common tapeti Sylvilagus brasiliensis, grey brocket deer Mazama gouazoubira, Central American agouti Dasyprocta punctata, lowland tapir Tapirus terrestris and collared peccary Pecari tajacu) accounted for 80% of the records (Fig. 2). We produced 56 single-species, single-season models for 14 medium- and large-sized mammal species (Table S1). No convergence of models was found for the giant anteater (Myrmecophaga tridactyla; Table S1). For all but one species (margay Leopardus wiedii), the selected models included the HFI as a covariate (Table S1). We found an association trend between the detection probability and sampling effort for 11 species, and this association was only significant for the crab-eating raccoon (Procyon cancrivorus) and the Central American agouti (Fig. 3).

Figure 2. Number of independent records and proportion of camera traps with records of medium- and large-sized mammal species in the Southern Yungas of Salta and Jujuy provinces (Argentina).
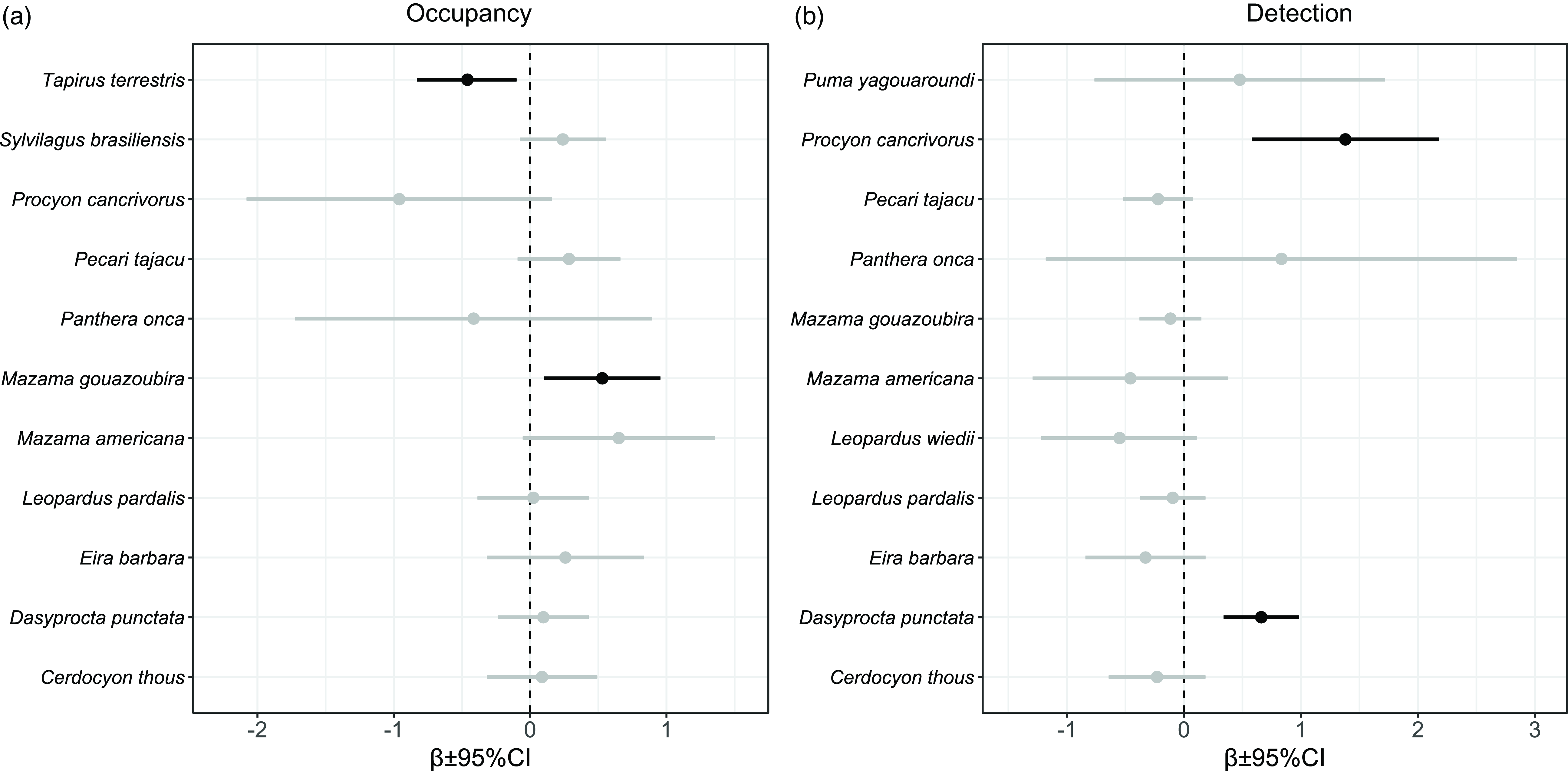
Figure 3. β parameter estimate and 95% confidence intervals (CIs) of (a) probability of habitat use (occupancy) and (b) detection models for medium- and large-sized mammal species in the Southern Yungas of Salta and Jujuy provinces (Argentina). Black lines indicate statistically significant β parameter estimates (p < 0.05) and grey lines indicate non-statistically significant β parameter estimates (p > 0.05). We did not include here the probability of habitat use of jaguarundi (Puma yagouaroundi) because the values exceeded the graph boundaries (β = 4.96; 95% CI = –4.95 to 14.88).
We found a significant negative association between the occupancy of lowland tapir and the HFI (Figs. 3 & 4 & Table S2). The best model for lowland tapir included only the HFI as an explanatory variable (Table S1); it had a good fit (Fig. S2), and there was no evidence of autocorrelation in the residuals (Fig. S3). For grey brocket deer, the models indicated a significant positive association of occupancy with the HFI (Figs. 3 & 4 & Table S2). For grey brocket deer, the final model was averaged between the global model and the HFI model (Table S1); this had a good fit (Fig. S2), and there was no evidence of autocorrelation in the residuals (Fig. S3).
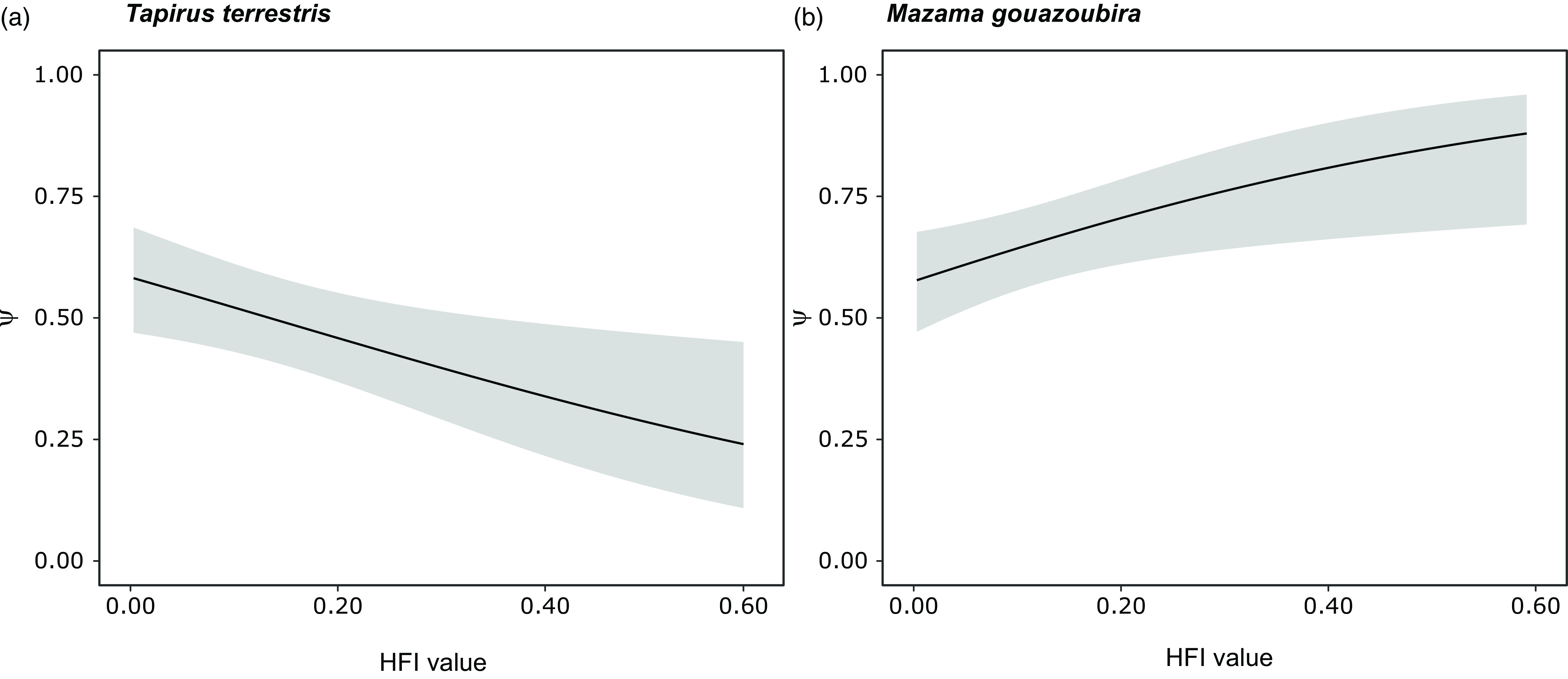
Figure 4. Probability of habitat use (Ψ; black line: mean; grey shading: ±95% confidence intervals) related to the human footprint index (HFI) values for (a) lowland tapir (Tapirus terrestris) and (b) grey brocket deer (Mazama gouazoubira) in the Southern Yungas of Salta and Jujuy provinces (Argentina).
Other species associated with the HFI that are worth highlighting yet were not statistically significant were the crab-eating raccoon, which showed a negative trend, and the common tapeti, the collared peccary and the red brocket deer (Mazama americana), which showed positive trends in their occupancy as the HFI increased (Fig. 3). Based on the estimates from the best-ranked models, mean occupancy ranged from ψ = 0.03 (range = 0.01–0.17) for the crab-eating raccoon to ψ = 0.79 (range = 0.67–0.87) for the grey brocket deer (Table 1).
Table 1. Probability of habitat use expressed as mean, minimum (Min) and maximum (Max) ranges for medium- and large-sized mammal species in the Southern Yungas of Salta and Jujuy provinces (Argentina).
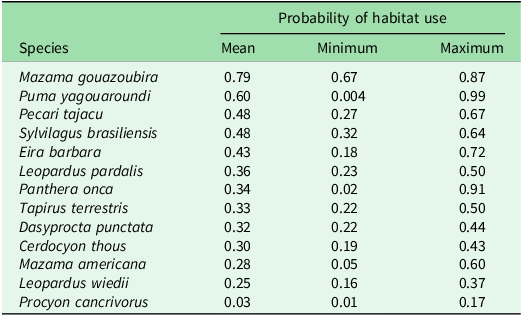
Discussion
We identified two mammal species (i.e., lowland tapir and grey brocket deer) that would be suitable for monitoring programmes in the Southern Yungas because they are easily identifiable, they are frequently detected in camera traps and their probability of habitat use shows a significant association with the HFI (MacKenzie & Nichols Reference MacKenzie and Nichols2004). The lowland tapir is strongly associated with wilderness areas, and monitoring the probability of habitat use of this species through camera traps could provide an early warning system of increasing levels of human impact. An increase in human impact can negatively affect the probability of habitat use of this species, with cascading effects on the ecosystem and interactions among species of the entire mammal assemblage, as is the case for other species and ecoregions (Galetti et al. Reference Galetti, Giacomini, Bueno, Bernardo, Marques and Bovendorp2009). We expected to find this positive association for lowland tapir with wilderness areas due to the species’ sensitivity to anthropogenic impacts (Rivera et al. Reference Rivera, Martinuzzi, Politi, Bardavid, De Bustos, Chalukian and Pidgeon2021). Wilderness areas are more remote from roads, infrastructure, crop edges and human settlements, making access by hunters and resource extraction more difficult (Martinuzzi et al. Reference Martinuzzi, Olah, Rivera, Politi, Silveira and Pastur2023). In addition, lowland tapir has been shown to be one of the first species to disappear in landscapes with high levels of transformation and fragmentation (Boron et al. Reference Boron, Deere, Xofis, Link, Quiñones-Guerrero, Payan and Tzanopoulos2019). The lowland tapir is a globally and nationally threatened species, so monitoring its occupancy is further justified (Flesher & Medici Reference Flesher and Medici2022).
On the other hand, monitoring the probability of habitat use of the grey brocket deer could indicate changes in forest conditions due to increased human impact because we found this probability to be associated with areas of higher anthropogenic impact. This deer species has a high degree of tolerance to human disturbance and high ecological plasticity, and it is found in a wide range of environmental conditions, even on agricultural lands (Ferreguetti et al. Reference Ferreguetti, Tomás and Bergallo2015, Rodrigues et al. Reference Rodrigues, Kays, Parsons, Versiani, Paolino and Pasqualotto2017). The grey brocket deer and the other species that showed a similar trend (i.e., common tapeti, collared peccary and red brocket deer) are dependent on forests, and the positive association of these species with higher human impact values might lead to negative outcomes in landscapes severely depleted of forest (Bianchi et al. Reference Bianchi, Jenkins, Lesmeister, Gouvea, Cesário, Fornitano and Gompper2021). Therefore, we call for caution when using these results and stress that we are not stating that these species can tolerate areas with no forest (HFI > 0.6), but rather that these species have high adaptive plasticity to disturbed forests and can inhabit highly modified forest landscapes (relatively high road and settlement density; Bianchi et al. Reference Bianchi, Jenkins, Lesmeister, Gouvea, Cesário, Fornitano and Gompper2021). The observed association of the grey brocket deer with human impact may be related to changes in forest structure, exclusion of predators (e.g., jaguar avoidance of human presence may lead to a predator-free environment) or increases in the availability of food resources (e.g., crops; Pérez & Pacheco Reference Pérez and Pacheco2006), which should be explored in future studies.
Our work points to the HFI, developed at a finer resolution, being a useful tool for monitoring anthropogenic impacts on medium- and large-sized mammals in the Southern Yungas of Salta and Jujuy provinces. The HFI used in this analysis (Martinuzzi et al. Reference Martinuzzi, Radeloff, Pastur, Rosas, Lizarraga and Politi2021) incorporated layers of locally relevant variables not available in global-scale human footprint maps at 1-km resolution (WCS & CIESIN 2005) such as puestos, caserios and different types of energy infrastructure, allowing for a more detailed grain of analysis for the study area (100-m resolution) and calibration of the intensity scores and distance of influence that better reflect the local conditions (Leu et al. Reference Leu, Hanser and Knick2008, Woolmer et al. Reference Woolmer, Trombulak, Ray, Doran, Anderson and Baldwin2008). However, the global-scale human footprint map has recently been updated with a ninefold improvement in spatial resolution (300 m) over previous efforts (Sanderson et al. Reference Sanderson, Fisher, Robinson, Sampson, Duncan and Royte2022), which allows for similar results to be obtained using the same datasets as those applied at an ecoregional scale (Table S3). In this sense, the human footprint map used in this study and the updated global human footprint (300-m resolution) provide proxies for other human activities that are widespread in the Southern Yungas, as in most Neotropical forests, but are difficult to detect and map, such as poaching, because roads, puestos and caserios provide access for hunters (Sanderson et al. Reference Sanderson, Jaiteh, Levy, Redford, Wannebo and Woolmer2002, Peres & Lake Reference Peres and Lake2003, Peres & Palacios Reference Peres and Palacios2007). Poaching affects medium- and large-sized game mammals and can lead to local extinction of prized species (Fa et al. Reference Fa, Peres and Meeuwig2002, Di Bitetti et al. Reference Di Bitetti, Paviolo, Ferrari, De Angelo and Di Blanco2008, Barboza et al. Reference Barboza, Lopes, Souto, Fernandes-Ferreira and Alves2016). We did not find a negative association between the most prized game species in the Southern Yungas (e.g., red brocket deer, grey brocket deer and collared peccary) and increasing HFI values. This could either indicate that the poaching level is low or that the high proportion of wilderness areas can buffer the negative effects of poaching by acting as population sources for these species (Peres Reference Peres2001, Peres & Palacios Reference Peres and Palacios2007).
We recorded 80% of the 32 medium- and large-sized mammal species (excluding the four species of armadillos) known to occur in the Southern Yungas (SAREM 2019). The seven species that were not recorded in this study are arboreal, semi-aquatic or associated with other types of environment (SAREM 2019). This suggests that the Southern Yungas of Argentina have not experienced the extirpation of species as has been observed for other nearby Neotropical ecoregions (e.g., Atlantic Forest; Bogoni et al. Reference Bogoni, Pires, Graipel, Peroni and Peres2018). Therefore, we highlight that there are still opportunities to develop conservation strategies that minimize threats to mammal species in the Southern Yungas. However, conservation strategies should not be delayed because local extirpations have already occurred in some parts of the study area; for example, that of the jaguar (Panthera onca) in the Serranía de Santa Bárbara (Perovic et al. Reference Perovic, de Bustos, Rivera, Arguedas Mora and Lizárraga2015).
Avoiding the defaunation that has already occurred in other Neotropical ecoregions requires that wilderness areas are preserved (Bogoni et al. Reference Bogoni, Peres and Ferraz2020). Consequently, it is important to conserve an adequate amount of intact native forest and keep human impacts in these areas at low levels, especially through the creation of protected areas, sustainable land management plans and corridors to maintain connectivity between protected areas (Bogoni et al. Reference Bogoni, Pires, Graipel, Peroni and Peres2018). Avoiding the expansion of anthropogenic impacts into wilderness areas is fundamental to prevent species range contractions, declining abundances and cascading effects on communities and ecosystems (Erb et al. Reference Erb, McShea and Guralnick2012, Venier et al. Reference Venier, Thompson, Fleming, Malcolm, Aubin and Trofymow2014). The Southern Yungas forest in Argentina still contains large tracts of wilderness and provides suitable habitat for many species that elsewhere are experiencing negative population trends (Martinuzzi et al. Reference Martinuzzi, Rivera, Politi, Bateman, Ruiz de los Llanos and Lizarraga2018, Politi & Rivera Reference Politi and Rivera2019). However, anthropogenic pressures are increasing in the Southern Yungas, and some species of medium- and large-sized mammals are sensitive to human impacts, such as the lowland tapir, and can show population declines that may make them prone to rapid extirpation (Woinarski et al. Reference Woinarski, Garnett, Legge and Lindenmayer2017). We showed here that the HFI, when applied at finer (100-m or 300-m) resolutions, is useful for monitoring anthropogenic threats to medium- and large-sized mammals. Specifically, we propose that monitoring the occupancy of the lowland tapir and the grey brocket deer could serve as an early warning system for tracking cumulative anthropogenic impacts on native forest (Toews et al. Reference Toews, Juanes and Burton2018).
Supplementary material
To view supplementary material for this article, please visit https://doi.org/10.1017/S037689292400002X.
Acknowledgements
We thank the private landowners who authorized us to place camera traps on their properties, the numerous field assistants and the provinces of Jujuy and Salta for the permits to conduct this research. We are grateful to Fundación CEBio (www.cebio.org.ar), which provided the necessary support to carry out this work. The authors would like to thank the editor, Nicholas Polunin, the associate editor and two anonymous referees who reviewed an earlier version of this manuscript and provided valuable suggestions and comments.
Financial support
This work received financial support from University of Jujuy B/064 2018, the National Agency for the Promotion of Science and Technology PICT 2020 SERIE A-03040, PICT-2021-I-A00708, the National Research Council CONICET PIP 100954, the Ministry of Science, Technology and Innovation Interinstitutional Projects on Strategic Issues PITES 03, the National Aeronautics and Space Administration (NASA) Biodiversity and Ecological Forecasting Program 80NSSC19K0183, Rufford Small Grants and the Whitley Fund for Nature.
Competing interests
The authors declare none.
Ethical standards
None.