The associations between weight change and CVD risk factors are well established. Specifically, a greater change in weight is associated with greater changes in BP, the serum cholesterol level, and the plasma glucose level (Ashley & Kannel, Reference Ashley and Kannel1974; Kannel et al., Reference Kannel, D’Agostino and Cobb1996). Although previous studies have provided some evidence of genetic and environmental influences on individual differences in current weight and CVD risk factors such as BP, glucose levels, lipid profiles (Elder et al., Reference Elder, Lichtenstein, Pittas, Roberts, Fuss, Greenberg and Neale2009; Gluckman & Hanson, Reference Gluckman and Hanson2008; Heller et al., Reference Heller, de Faire, Pedersen, Dahlen and McClearn1993; Iliadou et al., Reference Iliadou, Lichtenstein, de Faire and Pedersen2001), and genetic effects on rate of weight change and body mass index (BMI) change (Austin et al., Reference Austin, Friedlander, Newman, Edwards, Mayer-Davis and King1997; Hjelmborg et al., Reference Hjelmborg, Fagnani, Silventoinen, McGue, Korkeila, Christensen and Kaprio2008), the contribution of genes and the environment to the changes in these phenotypes has been scarcely investigated (Friedlander et al., Reference Friedlander, Meiner, Sharon, Siscovick and Miserez2009; Golla et al., Reference Golla, Strauch, Dietter and Baur2003; Herbeth et al., Reference Herbeth, Samara, Ndiaye, Marteau, Berrahmoune, Siest and Visvikis-Siest2010; Rice et al., Reference Rice, Perusse, Bouchard and Rao1999). Given the importance of optimal weight management and reduction in CVD risk factors for public health, a better understanding of the genetic and environmental contributions towards the observed changes in these phenotypes would provide insights into possible prevention strategies for CVD.
Since a within-pair comparison of MZ twins would preclude the genetic component, any observed relationships between within-pair weight change and changes in CVD risk factors would presumably be attributable to non-genetic factors. The aim of this study was to estimate the MZ twin similarity of changes in weight and CVD risk factors such as BP, the glucose level, the TC level, the TG level, the HDL level, and the LDL level and to assess the non-genetic contribution to the associations between the change in weight and changes in CVD risk factors among Korean MZ twins.
Subjects and Methods
Subjects and Study Design
The study subjects were participants in the Healthy Twin Study, which has been a part of the Korean Genomic Epidemiologic Study since 2005 (Sung et al., Reference Sung, Cho, Lee, Ha, Choi, Choi and Song2006). The zygosity of twins was determined by a questionnaire, resulting in a greater than 90% accuracy, and genetic analysis using 16 short tandem repeat markers (15 autosomal-markers and one sex-determining marker; AmpFlSTR Identifier Kit; Perkin Elmer, Waltham, MA, USA; Song et al., Reference Song, Lee, Lee, Lee, Lee, Hong and Sung2010). A total of 3,077 individuals (1,217 men and 1,860 women; 1,062 MZ and 243 dizygotic twin individuals, five undetermined twin individuals, and 1,767 non-twin family members) completed the baseline questionnaire and provided weight and height measurements. The participants, including twins and their family members, were invited by phone and mail in the follow-up examination. The participation rate was 44.8% for twins and their family members and 53.0% for MZ twins. There were no significant differences in BMI, BP, FBS (glucose), and lipid profiles at baseline between MZ twin individuals who participated in the follow-up examination and MZ twin individuals who did not participate.
Of these participants, 194 MZ twin pairs (116 men, 272 women; age 38.5 ± 6.8 years) who had complete data for repeat measurements of body weight and CVD risk factors (assessed at an average of 2.5 ± 0.9 years after the initial visit) and had no medical history of diabetes mellitus, hypertension, dyslipidemia, or CVD, were included in the analyses.
All participants provided written informed consent. The Institutional Review Board of each participating institution approved all study procedures. None of the authors has a conflict of interest.
Clinical and Biochemical Measurements
Weight and height measurements were taken with the subjects wearing indoor clothing and without shoes. Body weight and height were measured using a digital balance (Tanita Co., Seoul, South Korea) and a stadiometer (Samwha Co., Seoul, South Korea). BMI was calculated as the weight in kg divided by the height (in m) squared. BP was measured manually using a standard mercury sphygmomanometer with the participant in a sitting position. All procedures for the assessment of body weight and BP measurements were standardized across centers, by developing a standard protocol and training research coordinators and research assistants. All measurements were obtained in duplicate for each participant, and the average value of the two measurements was used for the analysis. A blood sample was drawn from each study subject after a 12-hour overnight fast and was shipped on the same day to a designated central laboratory institute that was accredited by the Korean Association of Quality Assurance for Clinical Laboratories. Serum concentrations of glucose (Hexokinase enzymatic assay), TC (enzymatic assay), HDL (enzymatic or homogeneous assay), TG (enzymatic assay), and LDL (enzymatic assay) were measured in fresh sera on a Siemens Advia 1650 (Erlangen, Germany) or Hitachi 7600-210/7180 biochemistry analyzer (Tokyo, Japan) using commercially available kits. A self-administered questionnaire was used to collect information about smoking, alcohol consumption, physical activity, and socioeconomic status (educational attainment, monthly income, and marital status). The cumulative lifetime amount of cigarette smoking (pack-years) was calculated by multiplying the smoking duration (years) by the mean number of packs smoked per day. Weekly consumption of alcohol (g/week) was calculated as the product of the number of days on which alcohol was consumed per week and the mean amount of alcohol consumption at each drinking occasion. Physical activity was assessed using the Korean version of the International Physical Activity Questionnaire (Craig et al., Reference Craig, Marshall, Sjostrom, Bauman, Booth, Ainsworth and Oja2003).
Statistical Analyses
The changes in weight, BMI, and CVD risk factors were calculated by subtracting the baseline values from the values at follow-up. The paired t-test or McNemar's test were used to compare anthropometric measurements and the CVD risk factors at baseline and follow-up, and changes of those phenotypes, lifestyle-related variables at baseline, and socioeconomic status-related variables at baseline between twins (one with a higher weight change and the co-twin with a lower weight change). The correlations between within-pair difference in weight change and within-pair differences in changes of CVD risk factors were performed. Since the data were paired, the relationships between the change in weight and the change in CVD risk factors were examined using a generalized linear model. In this model, the change in weight was classified as common (mean of twin pairs) weight change (between-pair regression coefficient) or individual-specific (differences within-pairs) weight change (within-pair regression coefficient; Carlin et al., Reference Carlin, Gurrin, Sterne, Morley and Dwyer2005) and adjusted for within-pair and between-pair differences in weight at baseline and within-pair differences in smoking and alcohol consumption at baseline. These analyses were performed using SPSS Statistics Version 19 (IBM, Armonk, NY, USA). All hypotheses were tested bidirectionally with an alpha level set at 0.05.
Variance component analysis was applied to assess the relative contributions of genetics and the environment to changes in weight and CVD risk factors from baseline until the follow-up visit. Heritability estimates of these phenotypes were defined by the proportion of phenotypic variance explained by additive genetic effects after adjustment for covariates (age and sex). To prevent biased MZ twin similarity due to outlier values, the kurtosis was adjusted by assuming a multivariate t distribution. The common environmental variance component was defined by the proportion of the total phenotype variance that is due to the non-genetic familial variance (e.g., common to twins).
To ascertain evidence of genetic and environmental relationships shared between changes in weight and CVD risk factors, we conducted bivariate analysis using Sequential Oligogenic Linkage Analysis Routines, version 4.2.0, which allows classification of the phenotypic correlations into genetic (ρG) and environmental correlations (ρE). If ρG or ρE is significantly different from zero, then significant genetic or environmental effects shared between the two traits are respectively suggested (Blangero et al., Reference Blangero, Williams and Almasy2001).
Results
Table 1 shows the within-pair comparisons of weight and CVD risk factors at baseline and follow-up and the changes in these phenotypes according to weight change. The twins who had a higher change in weight were more likely to have higher changes in systolic blood pressure, DBP, TG, TC, and LDL compared to their co-twins who had a lower change in weight (p < .05). The significance of within-pair differences in glucose level changes was borderline (p = .056). On the other hand, there were no significant within-pair differences in lifestyle and socioeconomic status-related variables.
TABLE 1 Within-Pair Comparison of Changes in Cardiovascular Disease Risk Factors According to Weight Change in 194 Monozygotic Twin Pairs

SBP = systolic blood pressure, DBP = diastolic blood pressure, TG = triglycerides, TC = total cholesterol, HDL = high-density lipoprotein cholesterol, LDL = low-density lipoprotein cholesterol, Hx = history, PA = physical activity, d = day. Change in value was calculated as value at follow-up minus baseline value.
aPaired t-test for comparison between pairs with higher and lower weight changes:*p <.05, †comparison of baseline values, ‡comparison of follow-up values, §comparison of change in values. bMcNemar test for comparison between pairs with higher and lower weight changes.
The MZ twin similarity for weight and CVD risk factors at baseline and those for the changes are shown in Table 2. The within-pair correlations at baseline, which ranged from 0.44 to 0.89, were higher than those for the changes, which ranged from 0.05 to 0.42. Likewise, heritability estimates for the changes were lower as compared to those at baseline, although all the estimates were significant. In bivariate analyses, we found significant environmental correlations shared between the change in weight and changes in CVD risk factors, except for glucose, whereas genetic correlations shared between the phenotype pairs were not significant (Table 3). In contrast, the common environmental variance was not significant for any of the phenotypes at baseline and for the changes.
TABLE 2 Within-Pair Correlations and Heritability Estimates for Cardiovascular Disease Risk Factors in 194 Monozygotic Twin Pairs

h2 = Heritability estimated after adjustments for age and sex, SBP = systolic blood pressure, DBP = diastolic blood pressure, TG = triglycerides, TC = total cholesterol, HDL = high-density lipoprotein cholesterol, LDL = low-density lipoprotein cholesterol, PA = physical activity.
*p <.05,**p <.001.
TABLE 3 Bivariate Correlations Between Weight Change and Changes in Cardiovascular Disease Risk Factors in 194 Monozygotic Twin Pairs
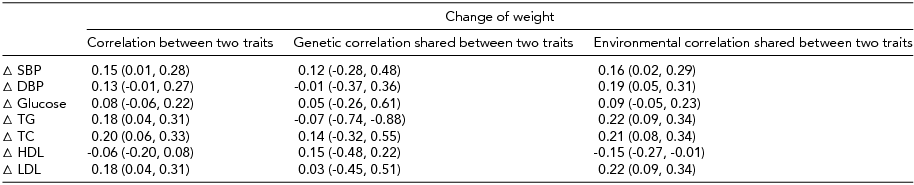
Δ = change, SBP = systolic blood pressure, DBP = diastolic blood pressure, TG = triglycerides, TC = total cholesterol, HDL = high-density lipoprotein cholesterol, LDL = low-density lipoprotein cholesterol.
Figure 1 shows the relationships between within-pair difference in weight change and within-pair differences in changes of CVD risk factors. Within-pair differences in weight change were significantly correlated with within-pair differences in changes of TC and TG, but not significantly correlated with within-pair differences in changes of BP, FBS, HDL, and LDL.

FIGURE 1 Scatter diagrams of the within-pair difference in weight change versus within-pair differences in changes of cardiovascular risk factors. Note: SBP = systolic blood pressure, TC = total cholesterol. Rho, Pearson correlation coefficient; *p < .05
The results of co-twin control analyses are presented in Table 4. After adjusting for baseline weight, smoking, and alcohol consumption and common weight change, DBP, TG, TC, and LDL significantly increased by 1.6 mmHg, 0.09 mmol/L, 0.10 mmol/L, and 0.09 mmol/L, respectively, per 1 kg increase in within-pair differences in weight change. None of the changes in CVD risk factors was independently associated with common weight change (mean of the weight changes in twin pairs). The within-pair difference in weight at baseline was only associated with the change in the glucose level after adjusting for the change in weight, common weight at baseline, and smoking and alcohol consumption at baseline.
TABLE 4 Associations Between Weight Change, Baseline Weight, and Changes in Cardiovascular Risk Factors in 194 Monozygotic Twin Pairs

BB = between-pair regression coefficient, Bw = within-pair regression coefficient, Δ = change; SBP = systolic blood pressure, DBP = diastolic blood pressure, TG = triglycerides, TC = total cholesterol, HDL = high-density lipoprotein cholesterol, LDL = low-density lipoprotein cholesterol.
Generalized linear model after adjusting for †within- and between-pair differences in weight and within-pair differences in alcohol consumption and smoking (pack-years); ‡within- and between-pair differences in weight change and within-pair differences in alcohol consumption and smoking (pack-years).
*p < .05.
Discussion
Changes in weight have been shown to be mirrored by changes in CVD risk factors (Ashley & Kannel, 1974; Kannel et al., 1996). The most plausible pathogenic mechanisms driving these associations appear to be insulin resistance and chronic inflammation that are induced by weight change. Insulin resistance is related to the activity of lipoprotein lipase and sodium reabsorption that cause changes in lipid metabolism and BP (Kannel et al., Reference Kannel, D’Agostino and Cobb1996; Reaven, Reference Reaven1988). In addition, the inflammatory state that is related to cytokine and acute-phase protein secretion by adipocytes may be causal in the progression of insulin resistance and related disorders (Calabrò et al., Reference Calabrò, Golia, Maddaloni, Malvezzi, Casillo, Marotta and Golino2009; Shoelson et al., Reference Shoelson, Lee and Goldfine2006). However, it is not clear whether genetic or non-genetic effects that are common to both weight change and changes in CVD risk factors could explain this underlying mechanism. In this study of MZ twins, we aimed to assess the contribution of non-genetic factors to the association between weight change and changes in CVD risk factors by eliminating genetic influences and environmental influences common to both twins (Vaag & Poulsen, Reference Vaag and Poulsen2007). The results of our analyses indicate that there was a significant non-genetic influence on the associations between weight change and changes in DBP, TG, TC, and LDL, after adjusting for potential confounders, including baseline weight, smoking, and alcohol consumption. Bivariate analyses also support our conclusions that the associations between these phenotypes were not influenced by genetics, but rather by environmental factors. Thus, the non-genetic effects that could be shared effects not related to genes can make within-pair difference in MZ twins. Despite the fact that we did not demonstrate any specific non-genetic mechanisms, we found that non-genetic mechanisms might mediate the association between the change in weight and changes in CVD risk factors.
We found that MZ twin similarity measured by within-pair correlations and heritability estimates for all phenotypes at baseline were moderate to high, whereas that for changes in these phenotypes was found to be small to moderate. Together, these results suggest that genetic effects may be dependent on age (Rice et al., Reference Rice, Perusse, Bouchard and Rao1999) and that MZ similarity for the changes in the phenotypes may vary over time. The observed MZ similarity pattern of phenotypes at the baseline in our study was comparable to those of previous twin studies (Elder et al., Reference Elder, Lichtenstein, Pittas, Roberts, Fuss, Greenberg and Neale2009; Heller et al., Reference Heller, de Faire, Pedersen, Dahlen and McClearn1993; Iliadou et al., Reference Iliadou, Lichtenstein, de Faire and Pedersen2001) With regard to changes in these phenotypes, comparable data on these factors are also reported in twin and family studies. In three family studies, the heritability estimates for long-term changes in BMI and weight were 0.36 (Friedlander et al., Reference Friedlander, Meiner, Sharon, Siscovick and Miserez2009; Rice et al., Reference Rice, Perusse, Bouchard and Rao1999) and 0.15 (Golla et al., Reference Golla, Strauch, Dietter and Baur2003) respectively, while the genetic effects on rate of change in BMI ranged between 0.58 and 0.68 for Finnish male and female twins (Hjelmborg et al., Reference Hjelmborg, Fagnani, Silventoinen, McGue, Korkeila, Christensen and Kaprio2008) and ranged from 0.57 to 0.86 over decades in cohorts of the Kaiser Permanente Women Twins Study (Austin et al., Reference Austin, Friedlander, Newman, Edwards, Mayer-Davis and King1997). In another family study, the heritability estimates for 5-year changes in metabolic syndrome components were not significant, whereas there was a significant environmental effects shared between the components of metabolic syndrome (Herbeth et al., Reference Herbeth, Samara, Ndiaye, Marteau, Berrahmoune, Siest and Visvikis-Siest2010). In yet another family study, the heritability estimate for BP change was not significant (Golla et al., Reference Golla, Strauch, Dietter and Baur2003). Heritability for the same trait may vary between populations where the relative importance of genetic variance and environmental effects shared between traits differs. Thus, the heritability estimates are generally higher, relative to environmental influence, for populations with more diverse genetic backgrounds (Herbeth et al., Reference Herbeth, Samara, Ndiaye, Marteau, Berrahmoune, Siest and Visvikis-Siest2010; Rice, Reference Rice2008). It is likely that the inconsistency in the heritability estimates for the changes in phenotypes between studies is related to differences in genetic and environmental variance. For example, differences in study design (twins, nuclear families, and extended families), study population characteristics (age, sex, lifestyle, and presence of chronic disease), adjustment for covariates, and analytic methods for heritability estimates may explain, at least in part, the differences in results that are reported in the literature (Herbeth et al., Reference Herbeth, Samara, Ndiaye, Marteau, Berrahmoune, Siest and Visvikis-Siest2010).
This study has several strengths and limitations that should be taken into account. Ideally, the baseline and follow-up examinations of twins should be conducted in the same day and at same research centers. Although the biochemical tests were conducted in one central qualified laboratory center and anthropometric measurements were performed by trained research assistants, the participants were recruited in three centers at baseline and two centers at follow-up. In addition, the twins were not always studied on the same day in the same center. Therefore, this systemic error could influence on the results. Second, we did not take into account additional non-genetic factors such as a sharing of living environment, medications related to weight change, and frequency of dieting. Third, potential violations of model assumptions that could influence the outcomes of interest, such as non-additive factors, gene-gene interactions, and gene-environment interactions, may distort the validity of the estimates (Herbeth et al., Reference Herbeth, Samara, Ndiaye, Marteau, Berrahmoune, Siest and Visvikis-Siest2010; Rice, Reference Rice2008). Lastly, we should consider the limitations related to MZ twin studies, such as the impossible assessment of the effects of both shared environment and gene-environment interaction at the same time. In addition, the results derived from twin studies may not be generalizable because twins are not a random sample of the general population. Nevertheless, as most similar studies were conducted in the Caucasian population, the findings of the present study, which included Korean MZ twins, are unique.
In conclusion, MZ twin similarity of changes in weight and CVD risk factors were small to moderate and non-genetic effects are likely responsible for the associations between the change in weight and changes in DBP, TG, TC, and LDL. These findings confirm the importance of modifiable factors such as lifestyle and environment in preventing weight gain and minimizing CVD risk factors.
Acknowledgments
This research was supported by the Basic Science Research Program through the National Research Foundation of Korea (NRF) funded by the Ministry of Education (NRF-2013R1A1A2057608). The views expressed in this article are those of the authors and not any funding body.