Introduction
Subthreshold symptoms of emotional disorders (such as mood and anxiety disorders) occurring during developmental years have been shown to graduate to full syndromic disorders in early adulthood,Reference Shankman, Lewinsohn, Klein, Small, Seeley and Altman1 but how and when this occurs, and more importantly what drives this process, is unknown. What is known, is that 50% of the projected lifetime risk of developing a mental disorder is accumulated by the age of 14.Reference Jones2 This means that in order to fully understand emotional disorders and meaningfully implement early intervention strategies, a deeper and more sophisticated appreciation of their emergence and the neurobiological processes that underpin their gestation in younger years, is urgently needed.
Adolescence
Adolescence is a period prone to the development of diverse forms of psychopathology related to the dysregulation of emotion. It is also the time when one's sense of self changes profoundly.Reference Bradley, Colcombe, Henderson, Alonso, Milham and Gabbay3 Interestingly, the two are linked and the regulation of emotion has been found to be modulated by the extent to which a stimulus has self-referential significance.Reference Herbert, Pauli and Herbert4 This is consistent with the fact that the prefrontal cortex, which is implicated in both self-referential processing and regulation of emotion, undergoes marked development during adolescence,Reference Yurgelun-Todd5 and frontolimbic network dysfunction is thought to be key to the inception of emotional disorders.Reference Mayberg6 With this in mind, in our earlier studyReference Das, Coulston, Bargh, Tanious, Phan and Calhoun7 we identified adolescents vulnerable to emotional disorders using family history and the Child and Adolescent PsychProfiler (CAPP) profiler, which uses a liberal threshold and takes into account a range of disorders. This is useful because at this time subclinical symptoms of different disorders appear.
Participants identified by CAPP as vulnerable or non-vulnerable to emotion disorders displayed significant differences in emotional symptoms such as depression, anxiety and dysregulation of emotion and therefore they were divided into groups with (ES+) and without (ES−) emotional symptoms. We used a functional magnetic resonance imaging (fMRI) task that necessitated emotion processing to examine whether neural activity and functional connectivity within frontolimbic networks differed between the ES+ and ES− groups. It was found that trait anxiety in adolescence may be as a result of impaired activity and connectivity within the frontolimbic network. Recently the investigation of the brain's intrinsic networks using resting-state fMRI, which obviates task confounds, has come to the fore and is proving useful providing novel insights into the neural substrates of major psychiatric and neurological disorders.Reference Menon8, Reference Calhoun, Sui, Kiehl, Turner, Allen and Pearlson9
Functional connectivity
Studies are investigating functional connectivity within and between networks to better understand the neural underpinnings of psychiatric illnesses. It has been suggested that during adolescence only the functional connectivity between networks (FNC) undergoes modification, and it is this that determines to a large degree optimal cognitive functioning.Reference Marek, Hwang, Foran, Hallquist and Luna10 Therefore, in order to piece together the emergence of emotional symptoms in adolescence it might be important to investigate the nature of FNC involved in emotion regulation and self-referential processing. Networks encompassing lateral and medial prefrontal brain regions are primarily involved in regulation of emotionReference Allen, Erhardt, Damaraju, Gruner, Segall and Silva11 and the default mode network (DMN), which consists of interconnected functional hubs and subsystems that in different configurations give rise to diverse sets of functions including self-referential processing.Reference Mak, Minuzzi, MacQueen, Hall, Kennedy and Milev12 Any attentional networks encompassing inferior or superior parietal lobule or temporoparietal junctionReference Viviani13 also play an important role in emotion regulation process.Reference Wadlinger and Isaacowitz14 Very recent research suggests that instead of static FNC, dynamic FNC (dFNC), which assumes FNC evolves over time, is a highly replicable and powerful tool that should be employed to understand network integration.Reference Abrol, Damaraju, Miller, Stephen, Claus and Mayer15 Intriguingly, although in practice, emotional symptoms often appear as a prodrome to future emotional disorders, to date, few if any neuroimaging studies have systematically examined the emergence of emotional dysfunction in adolescence, in particular using dFNC.
Therefore, in this study, using dFNC, we investigated the possible neural mechanisms that underpin the emergence of subsyndromal emotional symptoms in females during their adolescent years. We hypothesised that girls with subsyndromal emotional symptoms (ES+), compared to those without (ES−), will display a significantly differential pattern of FNC among key networks involved in emotion regulation and self-referential processing, and that these substantive differences will be linked meaningfully with clinical symptomatology. Thus providing important insights into their development.
Method
Participants
In total 88 adolescent girls (mean age 15 years) participated in this follow-up study. They were all recruited from the same girl's school in Sydney. At the first time point (T 1) all participants underwent neuroimaging and on the same day they completed a series of self-report questionnaires in the CADE clinic (www.cadeclinic.com). After 2 years (T 2) girls were followed-up at which time they only completed the clinical questionnaires they had completed at T 1 (i.e. there was no follow-up scan). Participants with a history of significant neurological illness (for example traumatic head injury, loss of consciousness, epilepsy or other neurological events), developmental disability or any medical/physical condition that precluded them from neuroimaging were excluded.
Written informed consent was obtained from the parents/guardians. The study was approved by the hospital Human Research Ethics Committee and the principal of the school. Participants were identified as ES+ or ES− according to the presence or absence of emotional symptoms, respectively. Participants qualified as ES+ if they surpassed the cut-off score for any mental disorder according to the CAPP,Reference Langsford, Houghton and Douglas16 or had a history of a mental health issue for which they had received psychological support or treatment.
Questionnaires
Participants completed a series of questionnaires measuring depression, anxiety and emotion regulation. Depression was measured using the Children's Depression Inventory (CDI),Reference Kovacs17 state (SA) and trait (TA) anxiety were measured using the State-Trait Anxiety Inventory (STAI)Reference Spielberger18 and emotion regulation using the Difficulties in Emotional Regulation Scale (DERS).Reference Gratz and Roemer19
MRI data acquisition
Imaging data-sets (structural and functional) were acquired on a University of Sydney 3 T Siemens Magnetom Trio Scanner based at the Advanced Research and Clinical High-field Imaging (ARCHI) facility. A T 2*-weighted gradient-echo echo-planar imaging (EPI) sequence (29 axial slices, slice thickness 4 mm with 1 mm gap, repeat time (TR) = 2000 ms, echo time (TE) = 35 ms, flip angle 70°, 64 × 64 matrix) was used to acquire 155 whole-brain volumes of functional data. This volume of data has previously been shown to capture different connectivity states robustly.Reference Abrol, Damaraju, Miller, Stephen, Claus and Mayer15, Reference Calhoun, Miller, Pearlson and Adali20–Reference Miller, Yaesoubi, Turner, Mathalon, Preda and Pearlson22
A high-resolution T 1-weighted structural image was also acquired for precise localisation of brain activity using a magnetisation prepared rapid gradient-echo (MPRAGE) sequence (TR = 1570 ms, TE = 3.22 ms, flip 15°, matrix 256 × 256, 192 slices). Participants were instructed to keep their eyes open during the functional scan and stare passively at a foveally presented fixation cross, as this is suggested to facilitate network delineation more so than eyes-closed conditionsReference Van Dijk, Hedden, Venkataraman, Evans, Lazar and Buckner23 and mitigates against participants falling asleep. Head motion during scanning was limited by restraining the head using foam pads inserted on each side. All participants were awake and alert at the start and conclusion of scanning.
fMRI data analysis
Data analyses using dFNC have been conducted in keeping with procedures outlined in studies investigating the brain's FNC with this methodology.Reference Damaraju, Allen, Belger, Ford, McEwen and Mathalon21
Pre-processing of data
Pre-processing used a combination of toolboxes (AFNI, https://afni.nimh.nih.gov; SPM, https://www.fil.ion.ucl.ac.uk/spm; and GIFT, http://mialab.mrn.org/software/gift). Each participant's functional and structural images were first inspected visually for scanner artefacts and gross anatomical abnormalities, and then re-oriented so that the origin of the image lay within 3 cm of the anterior commissure. The initial five images were discarded to remove longitudinal equilibration effects. A rigid body motion correction was performed using the INRIAlign – a motion correction algorithm that is unbiased by local signal changes. This was followed by slice time correction, using the middle slice as the reference frame, to account for timing differences in slice acquisition. Then fMRI data were despiked using AFNI's 3dDespike algorithm that mitigates the impact of outliers. These images were then spatially normalised to a common stereotactic space using the Montreal Neurological Institute EPI template and spatially smoothed with a Gaussian kernel of 8 mm3 full-width-at-half-maximum. Following spatial normalisation, the data (originally acquired at 3.75 × 3.75 × 5 mm3) were slightly subsampled to 3 × 3 × 3 mm3, resulting in 53 × 63 × 46 voxels. Finally, prior to performing group independent component analysis (ICA), the mean intensity was removed from the time course of each voxel.
Group ICA
Group spatial ICA, as implemented in the GIFT software was used to identify 100 components from the pre-processed fMRI data of all participants, and the number of components were chosen following previous literature investigating dFNC.Reference Damaraju, Allen, Belger, Ford, McEwen and Mathalon21, Reference Nomi, Farrant, Damaraju, Rachakonda, Calhoun and Uddin24 Spatial ICA decomposes the participant data into linear mixtures of spatially independent components that exhibit a unique time course profile. This was achieved using two data reduction steps. First a participant-specific data-reduction step was used to reduce 150 time-point data and then participant data were concatenated across time and a group data Principal Component Analysis (PCA) step was used to reduce data further. One hundred independent components were obtained from the group PCA-reduced matrix using the infomax algorithm.Reference Bell and Sejnowski25 To ensure stability of estimation this algorithm was repeated 20 times in ICASSO (http://research.ics.tkk.fi/ica/icasso/) and the most central run was selected and analysed further. Participant-specific spatial maps and time courses were obtained using the spatiotemporal regression back reconstruction approach.Reference Calhoun, Sui, Kiehl, Turner, Allen and Pearlson9, Reference Erhardt, Rachakonda, Bedrick, Allen, Adali and Calhoun26
Post-ICA processing
To identify peak activation clusters within a spatial component, all participants' maps for that particular component were entered into a random-effect analysis model (one-sample t-test in SPM). Brain regions were considered to be within each network if they met a height threshold of P<0.05 corrected for multiple comparisons using a family-wise error and an extent threshold of 50 voxels.
Networks for analysis were chosen on the basis of three conditions. First a component's peak activation clusters should fall on grey matter and it should show low spatial overlap with known vascular, ventricular, susceptibility and edge regions corresponding to head motion. Second, a component should show more spectral power in the low frequency range (i.e. the ratio of integral spectral power below 0.10 Hz to power between 0.15 Hz and 0.25 Hz should be greater than 5) and finally it should represent either DMN or any regulatory frontal networks showing primary activity in the lateral and medial prefrontal cortex or any attentional networks encompassing inferior or superior parietal lobule or temporoparietal junction.
To remove remaining noise sources including scanner drift and movement-related artefacts the participant specific time courses from the selected components were detrended, and orthogonalised with respect to estimated participant motion parameters. The impact of movement ‘spikes’ on subsequent FNC measures was reduced by despiking the time courses.Reference Damaraju, Allen, Belger, Ford, McEwen and Mathalon21
dFNC
To understand the dynamic nature of FNC, we used the temporal dFNC toolbox within the GIFT software. To compute dFNC between ICA time courses, a sliding window approach was adopted where the window segment was tapered by convolving a rectangle (width 20, TRs = 40 s) with a Gaussian (σ = 3 TRs), and advancing 1 TR at each step,Reference Du, Pearlson, Yu, He, Lin and Sui27 which resulted in W = 128 windows. The chosen window length 40 s (2 s × 20), has been suggested to be suitable for capturing dynamics in FNC.Reference Du, Fryer, Fu, Lin, Sui and Chen28 Covariance between components was estimated following the procedure as previously outlined.Reference Damaraju, Allen, Belger, Ford, McEwen and Mathalon21 Finally, the covariance matrices for each window were concatenated to form a component × component × window array to represent the changes in covariance (correlation) between networks (components) as a function of time.
Using Matlab's implementation of k-means clustering with the squared Euclidean distance, 500 iterates and 150 replicates dFNC windows were partitioned into four clusters. The centroids of these clusters can be treated as a small set of prototype connectivity ‘states’ (these can be thought of as average patterns that participants tend to return to during the course of the experiment).Reference Calhoun, Miller, Pearlson and Adali20, Reference Miller, Yaesoubi, Turner, Mathalon, Preda and Pearlson22 The optimal number of centroid states was estimated using the elbow criterion, defined as the ratio of within-cluster to between-cluster distances. A k of 4 was obtained using this method in a search window of k from 2 to 10.Reference Damaraju, Allen, Belger, Ford, McEwen and Mathalon21 To examine the structure of dFNC states across groups, we evaluated group-level dFNC states.
Differences between groups in dFNC
In each state differences between groups (ES+ and ES−) in dFNC was investigated using two sample t-tests and results were corrected for multiple comparisons using the false discovery rate (P<0.05).
Differences between groups in dFNC who remained ES− and ES+ at both time points
Given that some conversions from ES+ to ES− and vice versa were observed between the two time points, we also investigated for differences in dFNC between the groups in those who remained ES+ and ES− at both time points (T 1 and T 2).
Contribution of differences in dFNC to the future subclinical symptoms in all participants
In order to understand whether significant differences in dFNC between ES+ and ES− groups (identified using all participants) contribute to future symptoms (measured at T 2) of emotion dysregulation (using DERS), anxiety (using STAI) and depression (using CDI) several stepwise regression analyses were performed where changes in symptoms between two different time points were entered as dependent variable and symptom at T 1 and differences in dFNC were entered as independent variables.
Results
Participants
Out of 88, 71 participants completed the questionnaires at the second time point (T 2) and therefore the final analyses included 71 adolescents (27 in the ES+ group and 44 in the ES− group). Demographics and raw subclinical symptoms scores at two time points (T 1 and T 2) for each group have been presented in supplementary Table 1 available at https://doi.org/10.1192/bjp.2019.10.
There was no significant difference in age between the ES+ and ES− groups but clinical symptoms differed (Table 1). As can be seen from the table the ES+ group had consistently displayed significantly greater levels of psychopathology with greater mood and anxiety symptoms, and lower emotion regulation compared with the ES− group.
Table 1 Differences between groups (with (ES)+ and without (ES−) emotional symptoms) in age and clinical symptoms at two time points (T 1 and T 2)
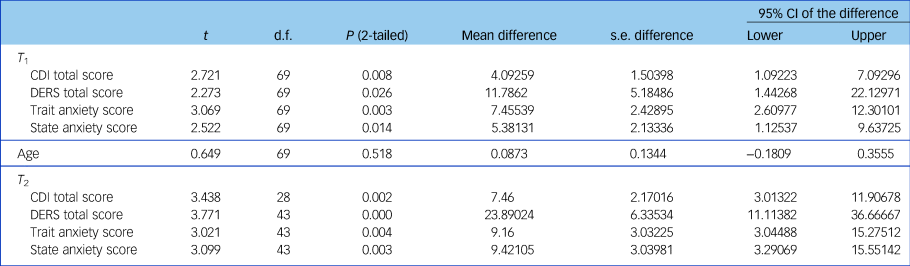
CDI, Children's Depression Inventory; DERS, Difficulties in Emotional Regulation Scale.
Networks of interests
From a total of 100 components, 28 were chosen as our networks of interest (Fig. 1) among which 7 components represented DMNs, 7 components represented frontal networks and 14 components represented attentional networks.Reference Allen, Erhardt, Damaraju, Gruner, Segall and Silva11 The cluster stability/quality were very high (Iq>0.9) for 25 components and high (Iq>0.85) for 3 components. Spatial maps of these networks are shown in Fig. 1 and primary regions within each network are provided in the supplementary Table 2.

Fig. 1 Spatial maps (displayed at the three most informative slices) of 28 independent components (ICs) that have been chosen as our networks of interest.
Differences between groups in dFNC
Significant differences between groups in dFNC were observed only in state 2 (Fig. 2). Coupling among four networks differed between groups. Among these four networks two represented DMNs (independent component (IC)17 and IC31). From now on IC17 and IC31 will be referred to as the pDMN and the aDMN, respectively, as they contain midline posterior and frontal cortices of the DMN. The other two networks represented lateral prefrontal networks (LPFNs; IC52 and IC59). Similarly, IC52 and IC59 will be referred to as the left LPFN (lLPFN) and right LPF network (rLPFN) as they encompass left and right lateral prefrontal cortices, respectively.

Fig. 2 Differences in dynamic functional network connectivity (dFNC) between groups with (ES+) and without (ES−) emotional symptoms.
Three sets of coupling (lLPFN with pDMN, aDMN and rLPFN) differed between ES− and ES+ groups. Coupling of the lLPFN with rLPFN was positive (coupling strength 0.1543) in the ES+ group but it was negative (coupling strength −0.0696) in the ES− group. This pattern was reversed for the coupling of lLPFN with pDMN and aDMN, which means coupling was positive in the ES− group and negative in the ES+ group. In the ES+ group coupling strength of the lLPFN with pDMN was −0.0503 and with aDMN it was −0.0622, whereas in the ES− group it was 0.01286 and 0.1044, respectively (schematically presented in Fig. 3).
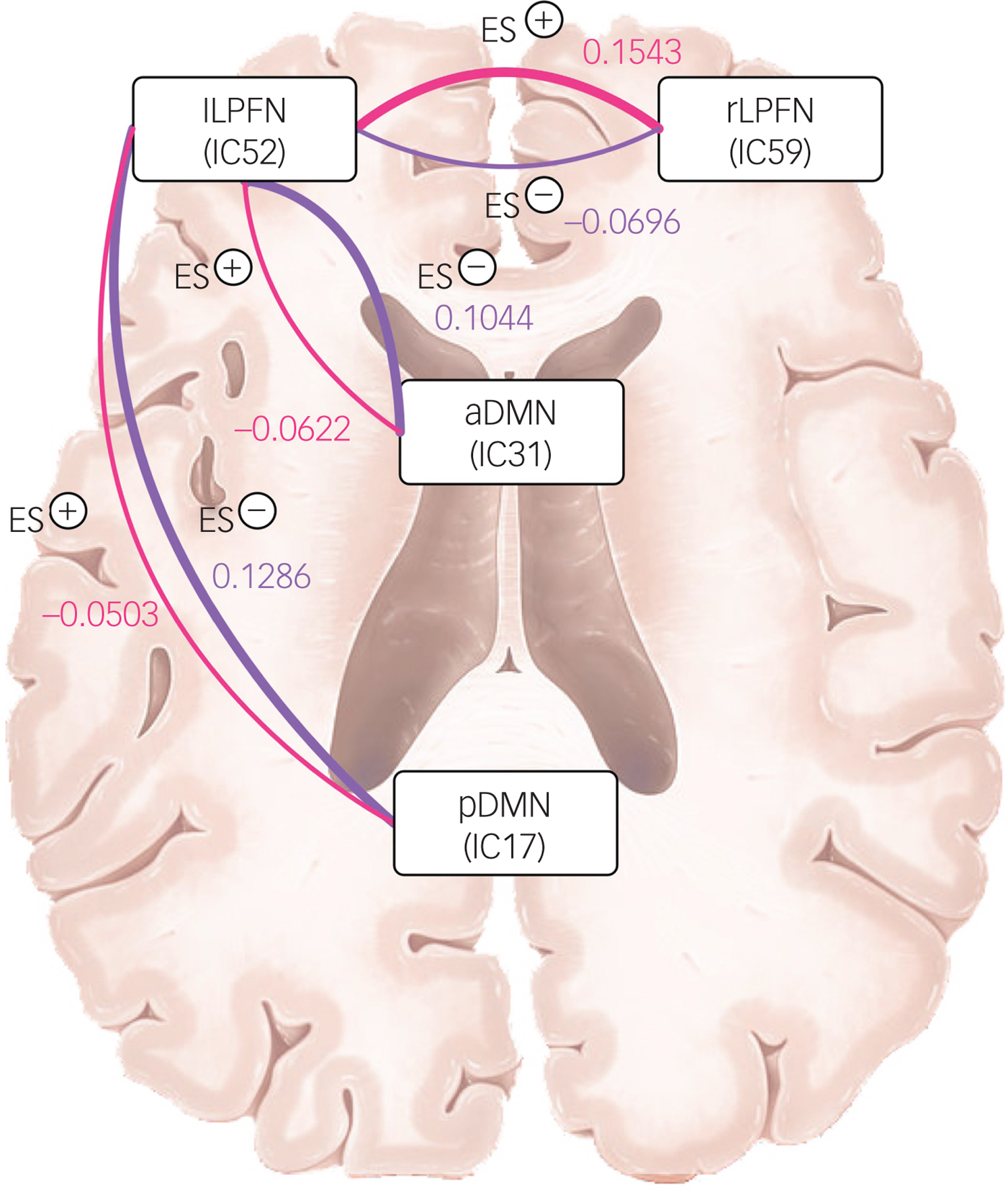
Fig. 3 Schematic of coupling strength differences between groups with (ES+) and without (ES−) emotional symptoms.
Differences between groups in dFNC who remained ES− and ES+ at both time points
There were 20 participants who remained in the ES+ group and 25 participants who remained in the ES− group across both time points. However, the three sets of coupling identified in the previous analysis (lLPFN with pDMN, aDMN and rLPFN) remained significantly different between the two groups and the nature of this coupling, in each group, remained the same as observed in the total sample.
Contribution of differences in dFNC to the future subclinical symptoms in all participants
The functional connectivity between the two lateral prefrontal networks (lLPFN-rLPFN) contributed to the changes (T 2 –T 1) in CDI and State Anxiety (SA). Whereas the CDI score at T 1 by itself explained 13.8% variance (adjusted), along with the coupling lLPFN-rLPFN it explained 21.9% variance (adjusted). Similarly, SA at T 1 explained 21% variance (adjusted), whereas along with the coupling lLPFN-rLPFN it explained 24.8% variance (adjusted).
Discussion
In recent years, dynamic functional connectivity analysis has shown increasing potential for interrogating the neural substrates of mental disorders.Reference Calhoun, Miller, Pearlson and Adali20 Therefore, employing this technique, the present study investigated whether the emergence of emotional symptoms in adolescence is linked to abnormal functional connectivity among networks involved in emotion regulation and self-referential processing. Our results revealed that increased interaction of the lLPFN with its counterpart, the rLPFN, along with decreased interaction with the anterior and posterior DMNs, is associated with the emergence of emotional symptoms in female adolescents.
Our ability to pursue goals effectively is critical for maintaining long-term health and well-being. Goal pursuit can be defined as ‘responses intentionally performed to bring about or maintain desired state’ or ‘responses intentionally performed to control or prevent an undesired state’ and these are often referred to as approach and avoidance goal pursuit, respectively.Reference Spielberg, Heller and Miller29 In practice to attain a desired outcome it has been proposed that careful regulation of both approach and avoidance systems is needed, and that such modulation is contingent on the strategies an individual chooses to employ that may depend on their personal goals.Reference Strauman, Detloff, Sestokas, Smith, Goetz and Rivera30 Ideal goals, such as hopes and aspirations are pursued via the promotion system through ‘making good things happen’, whereas ought goals such as obligations or responsibilities are pursued via the prevention system through keeping bad things from happening.
Neuroimaging research suggests that approach and avoidance actions stem from the left and right lateral prefrontal cortices, respectivelyReference Spielberg, Heller and Miller29 and the regions activated in response to ideal and ought goal priming broadly map onto the cortical midline structures of the DMN associated with self-referential processing.Reference Strauman, Detloff, Sestokas, Smith, Goetz and Rivera30 It has also been suggested that the anterior medial prefrontal cortex is involved in the evaluation and appraisal of self-referential stimuli, whereas the posterior medial cortex is thought to drive the temporal integration of new self-referential information into one's emotional and autobiographical self.Reference Northoff and Bermpohl31 Therefore, the DMN is able to construct mental simulations concerning possible future events by combining past experience with incoming stimuli, and thereby it is in a position to influence regulatory behaviour.
In our study, we found a differential pattern of functional connectivity between these networks in the ES+ and ES− groups. This differential pattern of functional connectivity perhaps impairs the evaluation of goals and strategies used to pursue them and thereby leads to a failure to achieve desired outcomes and that may lead to depression.
In our study, the functional connectivity between two LPFNs not only differed between groups with and without emotional symptoms but has also been found to predict future levels of depression and state anxiety in adolescent girls. It has been proposed that deficits in cognitive control putatively associated with depression affect emotion regulation in critical ways, thereby setting the stage for maintained negative affect and diminished levels of positive affect.Reference Joormann and Vanderlind32 Our finding of differences between groups (ES+ and ES−) in cognitive control networks and their association with depression suggest that this may underlie dysregulation of emotion in adolescent girls and may lead to full-blown emotional disorder in adulthood.
In conclusion, the findings from this study point to an intriguing possibility, namely that of impairment in the integration of approach–avoidance behaviours with self-referential thought processing, which potentially plays an important role in the emergence of emotional symptoms in female adolescents.
Limitations
Although findings from this study provide new insights into the emergence of emotional symptoms in adolescence it has several limitations of note. First, this study has used a broad measure to divide the participants into two groups. A positive result in CAPP indicates that a participant meets screening criteria for one or more of a range of possible disorders within DSM-IV-TR. However, this broad grouping was employed because non-specific emotional symptoms in adolescence can manifest as a range of disorders in early adulthood.Reference Shankman, Lewinsohn, Klein, Small, Seeley and Altman1 Second, although it has been suggested that these symptoms can lead to full-blown disorder, in the absence of a long-term longitudinal study that extends into adulthood this cannot be stated conclusively because engagement of these regions may change further with age, and only time will tell whether a full-blown disorder materialises.Reference McRae, Gross, Weber, Robertson, Sokol-Hessner and Ray33 Nevertheless, there does appear to be consistency of the groupings over time and extant research certainly points to the gradual development of mood and anxiety disorders emanating from a milieu of such emotional symptoms.
Third, our findings cannot be generalised to male adolescents although the investigation of females in this study does have the advantage of minimising the potential confound of gender differences in resting-state FNCReference Filippi, Valsasina, Misci, Falini, Comi and Rocca34 and also targets the population most prone to developing mood and anxiety-emotional symptoms. Fourth and finally, there may be additional networks that we have not considered in this particular study that play an equally important role in the emergence and formulation of emotional symptoms in adolescents. Clearly, all of these points require further investigation and findings from our own study also need to be replicated. For example, they should be extended through studies that span the age spectrum, both prior to and beyond the ages of the girls in this study, and to be truly generalisable need to be replicated in boys.
Clinical implications
The findings from our study are not only interesting because they inform future research but because they point to important treatment implications. The possibility that functional brain changes can be identified in adolescents prior to them developing disorders is of immense significance both diagnostically, and for identifying potential targets for treatment and determining the timing of specific interventions. However, in order to understand how emotional symptoms and emotional dysregulation in adolescence can lead to depression in adulthood, further longitudinal research is needed.
Funding
This research is supported by an NHMRC Program Grant (APP1073041) awarded to G.S.M., P.D. and R.A.B.
Supplementary material
Supplementary material is available online at https://doi.org/10.1192/bjp.2019.10.
eLetters
No eLetters have been published for this article.