Long-standing concern exists about the potential influence of financial interests on medical decision-making (e.g. Reference Hillman, Joseph and MagelHillman et al, 1990; Reference Rennie and FlanaginRennie & Flanagin, 1992). Especially vigorous discussion has centred on the conduct and reporting of pharmacoeconomic research (e.g. Reference Hillman, Eisenberg and PaulyHillman et al, 1991; Reference Udrarhelyi, Graham and RaiUdrarhelyi et al, 1992; Reference Gulati and BitranGulati & Bitran, 1995; Reference Siegel, Weinstein and RussellSiegel et al, 1996; Reference NeumannNeumann, 1998; Reference Hill, Mitchell and HenryHill et al, 2000; Reference Jones and CockrumJones & Cockrum, 2000; Reference Neumann, Stone and ChapmanNeumann et al, 2000b). However, there has been little quantitative study of potential bias in pharmacoeconomic research throughout medicine. Reported studies have reached mixed conclusions (e.g. Reference Sacristan, Bolanos and SotoSacristan et al, 1997; Reference Azimi and WelchAzimi & Welch, 1998; Reference Friedberg, Saffran and StinsonFriedberg et al, 1999; Reference Neumann, Sandberg and BellNeumann et al, 2000a), perhaps in part because with one exception (Reference Friedberg, Saffran and StinsonFriedberg et al, 1999) they investigated several drugs and in some cases included medical devices. We are unaware of any study focused on psychiatric medication.
We studied associations between sponsorship and study design with quantitative outcome in pharmacoeconomic studies by examining the test case of antidepressants. We asked the following primary questions. First, is there an association between industry v. non-industry sponsorship of studies and quantitative conclusions? Second, among industry-sponsored studies and between industry-sponsored v. non-industry-sponsored studies, is there an association between study design and quantitative conclusions?
METHOD
We chose antidepressants licensed in the UK or the USA as our test case because of their large market share and the number of pharmacoeconomic studies. Antidepressants rank in the top three drug classes world-wide in terms of sales dollars. Their growth in sales ranks them among the top five drug classes worldwide (IMS Health, 2001). Additionally, these antidepressants are the subject of multiple cost-outcome studies reporting quantitative results.
Study sample
To locate reports of pharmacoeconomic studies of antidepressant drugs we used the Cochrane Library, Medline and Health-STAR databases supplemented by manual searches based on the references cited in the studies located through the databases. We searched for all articles between 1987 - the year the first 'newer' antidepressant, fluoxetine, received US Food and Drug Administration (FDA) approval - and April 2001. The search terms we used in Medline and HealthSTAR were COST-BENEFIT ANALYSIS or COST SAVINGS or DRUG COSTS or COST-EFFECTIVENESS (text word) and ANTIDEPRESSIVE AGENTS or ANTIDEPRESSANT (text word). The search term we used in the Cochrane Library was ANTIDEPRESSIVE AGENTS. We identified 46 articles (Jonsson & Bebbington, Reference Jonsson, Bebbington, Jonsson and Rosenbaum1993, Reference Jonsson and Bebbington1994; Reference Hatziandreu, Brown and RevickiHatziandreu et al, 1994; Reference Le Pen, Levy and RavilyLe Pen et al, 1994; Reference McFarlandMcFarland, 1994; Sclar et al, Reference Sclar, Robison and Skaer1994, Reference Sclar, Robison and Skaer1995, Reference Sclar, Skaer and Robison1998, Reference Sclar, Skaer and Robison1999; Reference StewartStewart, 1994; Reference Anton and RevickiAnton & Revicki, 1995; Einarson et al, Reference Einarson, Arikian and Sweeney1995, Reference Einarson, Addis and Iskedjian1997; Reference Lapierre, Bentkover and SchainbaumLapierre et al, 1995; Reference Nuijten, Hardens and SoutreNuijten et al, 1995; Revicki et al, Reference Revicki, Brown and Palmer1995, Reference Revicki, Brown and Keller1997; Reference Skaer, Sclar and RobisonSkaer et al, 1995; Reference Bentkover and FeighnerBentkover & Feighner, 1996; Reference Forder, Kavanagh and FenyoForder et al, 1996; Hylan et al, Reference Hylan, Kotsanos and Andersen1996, Reference Hylan, Crown and Meneades1998; Reference Montgomery, Brown and ClarkMontgomery et al, 1996; Reference Smith and SherrillSmith & Sherrill, 1996; Croghan et al, Reference Croghan, Lair and Engelhart1997, Reference Croghan, Kniesner and Melfi2000; Reference Melton, Kirkwood and FarrarMelton et al, 1997; Reference Obenchain, Melfi and CroghanObenchain et al, 1997; Reference Woods and RizzoWoods & Rizzo, 1997; Reference Boyer, Danion and BisserbeBoyer et al, 1998; Canadian Coordinating Office for Health Technology Assessment, 1998; Reference Crown, Hylan and MeneadesCrown et al, 1998; Reference Simon and FishmanSimon & Fishman, 1998; Reference Thompson, Hylan and McMullenThompson et al, 1998; Brown et al, Reference Brown, Nimmerrichter and Guest1999a,Reference Brown, van Loon and Guestb; Reference Griffiths, Sullivan and FrankGriffiths et al, 1999; Reference Nurnberg, Hensley and ThompsonNurnberg et al, 1999; Reference Russell, Berndt and MiceliRussell et al, 1999; Reference Simon, Heilegenstein and RevickiSimon et al, 1999; Reference Borghi and GuestBorghi & Guest, 2000; Reference Sullivan, Griffiths and FrankSullivan et al, 2000; Reference Casciano, Doyle and ArikianCasciano et al, 2001; Reference Doyle, Casciano and ArikianDoyle et al, 2001; Reference Poret, Neslusan and RicciPoret et al, 2001; Reference Wan, Crown and BerndtWan et al, 2002). We excluded two studies (Reference Boyer, Danion and BisserbeBoyer et al, 1998; Reference Simon, Heilegenstein and RevickiSimon et al, 1999) because they were randomised trials, unlike all the other studies, which were modelling studies or analyses of administrative databases. The remaining articles represent 45 separate studies. Two articles report the results of one study (Jonsson & Bebbington, Reference Jonsson, Bebbington, Jonsson and Rosenbaum1993, Reference Jonsson and Bebbington1994). Two articles report two studies each, one in-patient, one out-patient (Einarson et al, Reference Einarson, Arikian and Sweeney1995, Reference Einarson, Addis and Iskedjian1997). Two articles reported slight variations on two studies, one in-patient and one out-patient (Reference Casciano, Doyle and ArikianCasciano et al, 2001; Reference Doyle, Casciano and ArikianDoyle et al, 2001).
Classification of studies
For the primary analysis we categorised each study according to whether it was industry-sponsored. The study was categorised as industry-sponsored if at least one author was listed as a pharmaceutical company employee, or an acknowledgement listed pharmaceutical company support; otherwise, it was categorised as non-industry-sponsored. For secondary analyses we categorised studies authored by industry employees separately from studies only listing financial support.
Study sponsors were categorised by product into those manufacturing selective serotonin reuptake inhibitors (SSRIs: fluoxetine, sertraline, paroxetine and citalopram) or ‘atypical’ antidepressant drugs (venlafaxine, bupropion and mirtazapine).
Operationalisation of outcomes
For either of the questions posed in our study no single means of operationalising the issue of which antidepressant was favoured could be applied to all studies. Therefore, we performed separate analyses using alternative operationalisations. Specifically, for question one (the industry v. non-industry comparison), no single standard was applicable that allowed analysis of all 46 studies. Seemingly simple standards such as ‘sponsor's antidepressant favoured' could not apply: in non-industry-sponsored studies, there is no ‘sponsor's antidepressant'. In our primary depressant'. analysis of industry-sponsored v. non-industry-sponsored studies we examined whether the outcome favoured SSRIs or tricyclic antidepressants (TCAs), excluding studies sponsored by ‘atypical’ antidepressant manufacturers. To allow analysis of the latter studies, we performed an alternative analysis based on whether the outcome favoured the ‘newest antidepressant’ (‘newness’ was based upon date of FDA approval). In this analysis, studies in which the sponsor's drug was not the newest were excluded.
In addressing our second question, regarding the association of study design with bias on outcome, we examined the issue both within industry-sponsored trials and between industry-sponsored and non-industry-sponsored trials. Within the first group we looked at the association of modelling v. administrative study designs with outcome. We operationalised the outcomes and groups in two alternative ways: favouring the newest drug among all industry-sponsored studies, or favouring the sponsored drug among all industry-sponsored studies.
In examining the association of study design with outcome between industry v. non-industry sponsors, we compared the outcome patterns within modelling studies. We could not compare outcome patterns in administrative data studies given there was only one such non-industry-sponsored study. We operationalised outcomes in two alternative ways: favouring the newest drug, or favouring SSRIs v. TCAs.
Rating study outcomes
Initially two of the authors (C.B.B. and M.N.J.) independently categorised sponsorship and outcomes of each study. If their ratings were inconsistent, a third author (S.W.W.) rated the study. Initial ratings agreed in all cases but one.
Most studies contained several outcomes. However, we wished to rate a single outcome from each study and employed the following decision rules to select that outcome. First, we selected only quantitative outcomes. Second, among base case and variants, we selected the base case. Third, among outcomes adjusted for bias and unadjusted outcomes, we selected the adjusted outcome. Fourth, among outcomes for various time periods, we selected the longest period. Fifth, among multiple pharmacoeconomic indicators, we selected a single outcome on the basis of the following rules: if only cost outcomes were reported, we chose total costs over more limited costs; if cost and cost-effectiveness outcomes were reported, we chose cost-effectiveness outcomes; and if more than one type of cost-effectiveness ratio was reported, we chose incremental over average ratios. Sixth, if results were reported separately for individual countries, we selected the results for the UK and the USA.
After selecting a single outcome for each study, the researchers rated each study as favourable, neutral or unfavourable for the drug of interest, depending on the particular analysis (e.g. SSRI in the SSRI v. TCA analysis, or newest antidepressant in the newest v. older antidepressant analysis): ‘favourable’ meant that a drug's quantitative cost-effectiveness results were unequalled by any of the other drugs in the study; ‘neutral’ meant that although other drugs' results might be equal to it, none surpassed the drug of interest; and ‘unfavourable’ meant that other drugs' results did surpass the drug of interest. Raters used all available information to judge differences in outcomes among drugs. If the study reported statistical significance, raters based their judgements on statistically significant differences. If the study did not report statistical significance, raters based their judgements on the reported numerical differences. With quality-adjusted life-years (QALYs), raters judged a treatment superior if marginal cost-effectiveness was less than US$20 000 per QALY, a common applied limit (Reference Laupacis, Feeny and DetskyLaupacis et al, 1992). Subsequently, we performed a sensitivity analysis by varying the marginal threshold between $20 000 and $100 000 per QALY.
The following is an example of how raters applied the rules noted above to designate a specific study as favourable, neutral or unfavourable. In the SSRI v. tricyclic or heterocyclic antidepressant analysis of the Hatziandreu study (Reference Hatziandreu, Brown and RevickiHatziandreu et al, 1994) the preceding rules led raters to judge that the study favoured the SSRI. The study reported the base case incremental cost-effectiveness ratio to be £2172 ($3692) for each QALY gained by using the SSRI rather than the TCA. This cost per QALY gained is less than the $20 000 per QALY cut-off noted in the raters' decision rules; therefore, the study was rated as favourable for the SSRI.
In addition to the planned analyses described above, we performed two exploratory analyses: one was based on the number of industry authors and the second was based on the ordinal position of any industry authors. Neither of these analyses yielded a significant association.
We analysed the association between sponsorship and outcome using Fisher's exact test as generalised for 2×3 tables. We chose contingency table analysis rather than a meta-analytic technique because of the qualitative heterogeneity of the pharmacoeconomic outcome types across studies, which ranged from direct costs per patient, to direct costs per treatment success, to direct costs per symptom-free day, to lifetime direct costs per discounted QALY. We judged it inappropriate to transform these qualitatively disparate types of outcomes into a common effect size. We selected the 0.05 α level, two-tailed.
RESULTS
Details of the studies are listed in Tables 1, 2, 3.
Table 1 Economic outcome studies of antidepressant therapy sponsored by manufacturers of selective serotonin reuptake inhibitors
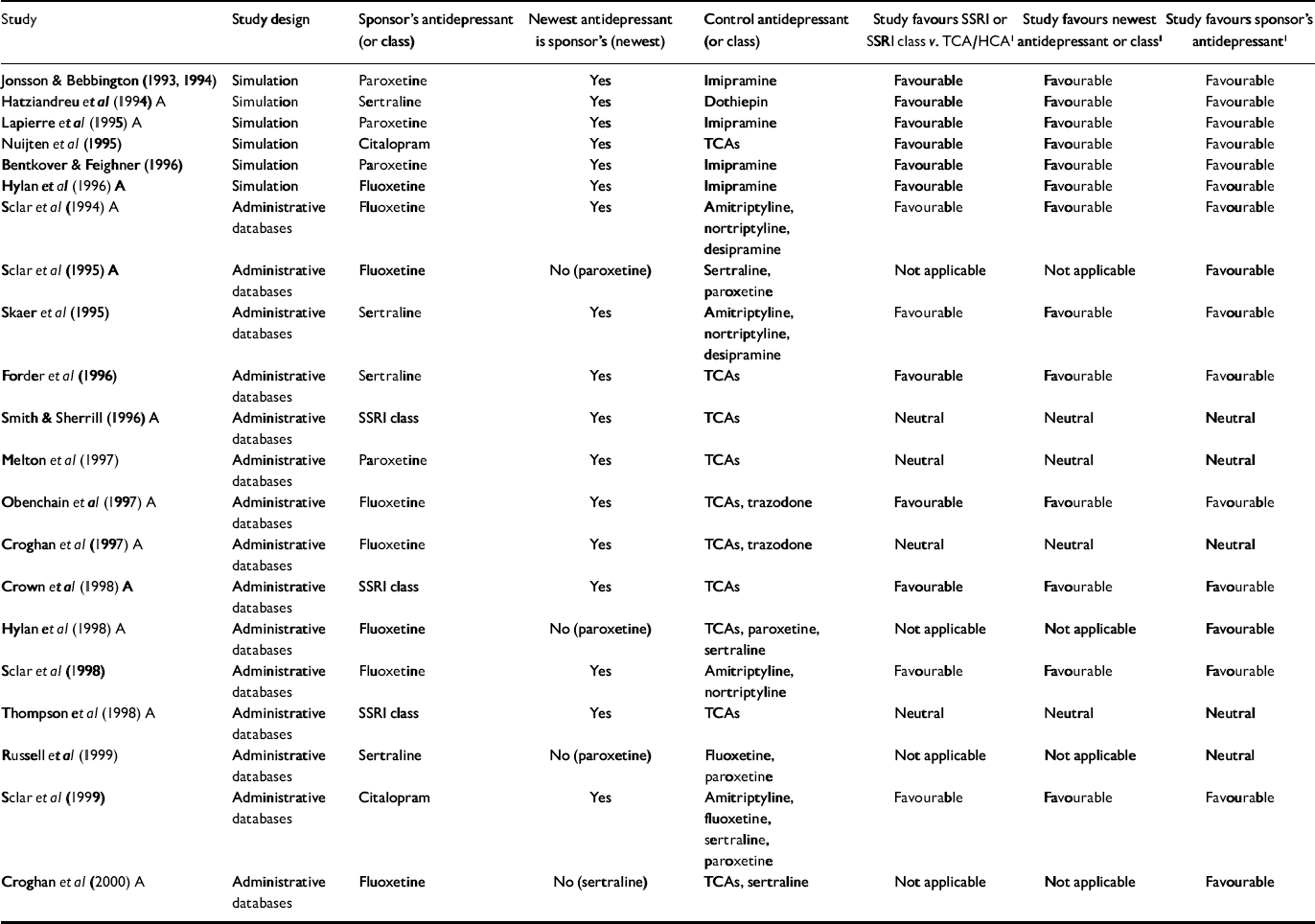
Study | Study design | Sponsor's antidepressant (or class) | Newest antidepressant is sponsor's (newest) | Control antidepressant (or class) | Study favours SSRI or SSRI class v. TCA/HCA1 | Study favours newest antidepressant or class1 | Study favours sponsor's antidepressant1 |
---|---|---|---|---|---|---|---|
Jonsson & Bebbington (Reference Jonsson, Bebbington, Jonsson and Rosenbaum1993, Reference Jonsson and Bebbington1994) | Simulation | Paroxetine | Yes | Imipramine | Favourable | Favourable | Favourable |
Hatziandreu et al (Reference Hatziandreu, Brown and Revicki1994) A | Simulation | Sertraline | Yes | Dothiepin | Favourable | Favourable | Favourable |
Lapierre et al (Reference Lapierre, Bentkover and Schainbaum1995) A | Simulation | Paroxetine | Yes | Imipramine | Favourable | Favourable | Favourable |
Nuijten et al (Reference Nuijten, Hardens and Soutre1995) | Simulation | Citalopram | Yes | TCAs | Favourable | Favourable | Favourable |
Bentkover & Feighner (Reference Bentkover and Feighner1996) | Simulation | Paroxetine | Yes | Imipramine | Favourable | Favourable | Favourable |
Hylan et al (Reference Hylan, Kotsanos and Andersen1996) A | Simulation | Fluoxetine | Yes | Imipramine | Favourable | Favourable | Favourable |
Sclar et al (Reference Sclar, Robison and Skaer1994) A | Administrative databases | Fluoxetine | Yes | Amitriptyline, nortriptyline, desipramine | Favourable | Favourable | Favourable |
Sclar et al (Reference Sclar, Robison and Skaer1995) A | Administrative databases | Fluoxetine | No (paroxetine) | Sertraline, paroxetine | Not applicable | Not applicable | Favourable |
Skaer et al (Reference Skaer, Sclar and Robison1995) | Administrative databases | Sertraline | Yes | Amitriptyline, nortriptyline, desipramine | Favourable | Favourable | Favourable |
Forder et al (Reference Forder, Kavanagh and Fenyo1996) | Administrative databases | Sertraline | Yes | TCAs | Favourable | Favourable | Favourable |
Smith & Sherrill (Reference Smith and Sherrill1996) A | Administrative databases | SSRI class | Yes | TCAs | Neutral | Neutral | Neutral |
Melton et al (Reference Melton, Kirkwood and Farrar1997) | Administrative databases | Paroxetine | Yes | TCAs | Neutral | Neutral | Neutral |
Obenchain et al (Reference Obenchain, Melfi and Croghan1997) A | Administrative databases | Fluoxetine | Yes | TCAs, trazodone | Favourable | Favourable | Favourable |
Croghan et al (Reference Croghan, Lair and Engelhart1997) A | Administrative databases | Fluoxetine | Yes | TCAs, trazodone | Neutral | Neutral | Neutral |
Crown et al (Reference Crown, Hylan and Meneades1998) A | Administrative databases | SSRI class | Yes | TCAs | Favourable | Favourable | Favourable |
Hylan et al (Reference Hylan, Crown and Meneades1998) A | Administrative databases | Fluoxetine | No (paroxetine) | TCAs, paroxetine, sertraline | Not applicable | Not applicable | Favourable |
Sclar et al (Reference Sclar, Skaer and Robison1998) | Administrative databases | Fluoxetine | Yes | Amitriptyline, nortriptyline | Favourable | Favourable | Favourable |
Thompson et al (Reference Thompson, Hylan and McMullen1998) A | Administrative databases | SSRI class | Yes | TCAs | Neutral | Neutral | Neutral |
Russell et al (Reference Russell, Berndt and Miceli1999) | Administrative databases | Sertraline | No (paroxetine) | Fluoxetine, paroxetine | Not applicable | Not applicable | Neutral |
Sclar et al (Reference Sclar, Skaer and Robison1999) | Administrative databases | Citalopram | Yes | Amitriptyline, fluoxetine, sertraline, paroxetine | Favourable | Favourable | Favourable |
Croghan et al (Reference Croghan, Kniesner and Melfi2000) A | Administrative databases | Fluoxetine | No (sertraline) | TCAs, sertraline | Not applicable | Not applicable | Favourable |
Table 2 Economic outcome studies of antidepressant therapy sponsored by manufacturers of atypical antidepressants
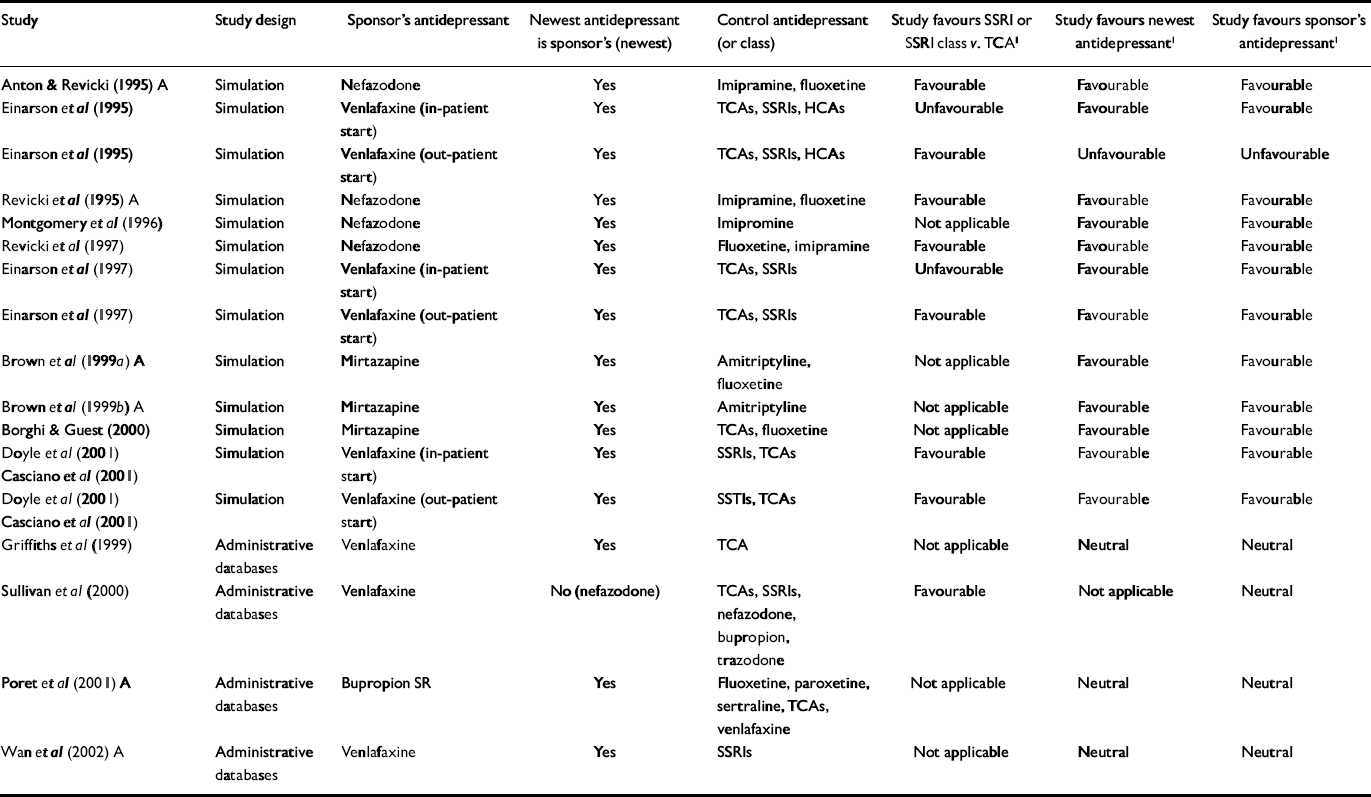
Study | Study design | Sponsor's antidepressant | Newest antidepressant is sponsor's (newest) | Control antidepressant (or class) | Study favours SSRI or SSRI class v. TCA1 | Study favours newest antidepressant1 | Study favours sponsor's antidepressant1 |
---|---|---|---|---|---|---|---|
Anton & Revicki (Reference Anton and Revicki1995) A | Simulation | Nefazodone | Yes | Imipramine, fluoxetine | Favourable | Favourable | Favourable |
Einarson et al (Reference Einarson, Arikian and Sweeney1995) | Simulation | Venlafaxine (in-patient start) | Yes | TCAs, SSRIs, HCAs | Unfavourable | Favourable | Favourable |
Einarson et al (Reference Einarson, Arikian and Sweeney1995) | Simulation | Venlafaxine (out-patient start) | Yes | TCAs, SSRIs, HCAs | Favourable | Unfavourable | Unfavourable |
Revicki et al (Reference Revicki, Brown and Palmer1995) A | Simulation | Nefazodone | Yes | Imipramine, fluoxetine | Favourable | Favourable | Favourable |
Montgomery et al (Reference Montgomery, Brown and Clark1996) | Simulation | Nefazodone | Yes | Imipromine | Not applicable | Favourable | Favourable |
Revicki et al (Reference Revicki, Brown and Keller1997) | Simulation | Nefazodone | Yes | Fluoxetine, imipramine | Favourable | Favourable | Favourable |
Einarson et al (Reference Einarson, Addis and Iskedjian1997) | Simulation | Venlafaxine (in-patient start) | Yes | TCAs, SSRIs | Unfavourable | Favourable | Favourable |
Einarson et al (Reference Einarson, Addis and Iskedjian1997) | Simulation | Venlafaxine (out-patient start) | Yes | TCAs, SSRIs | Favourable | Favourable | Favourable |
Brown et al (Reference Brown, Nimmerrichter and Guest1999a ) A | Simulation | Mirtazapine | Yes | Amitriptyline, fluoxetine | Not applicable | Favourable | Favourable |
Brown et al (Reference Brown, van Loon and Guest1999b ) A | Simulation | Mirtazapine | Yes | Amitriptyline | Not applicable | Favourable | Favourable |
Borghi & Guest (Reference Borghi and Guest2000) | Simulation | Mirtazapine | Yes | TCAs, fluoxetine | Not applicable | Favourable | Favourable |
Doyle et al (Reference Doyle, Casciano and Arikian2001) Casciano et al (Reference Casciano, Doyle and Arikian2001) | Simulation | Venlafaxine (in-patient start) | Yes | SSRIs, TCAs | Favourable | Favourable | Favourable |
Doyle et al (Reference Doyle, Casciano and Arikian2001) Casciano et al (Reference Casciano, Doyle and Arikian2001) | Simulation | Venlafaxine (out-patient start) | Yes | SSTIs, TCAs | Favourable | Favourable | Favourable |
Griffiths et al (Reference Griffiths, Sullivan and Frank1999) | Administrative databases | Venlafaxine | Yes | TCA | Not applicable | Neutral | Neutral |
Sullivan et al (Reference Sullivan, Griffiths and Frank2000) | Administrative databases | Venlafaxine | No (nefazodone) | TCAs, SSRIs, nefazodone, bupropion, trazodone | Favourable | Not applicable | Neutral |
Poret et al (Reference Poret, Neslusan and Ricci2001) A | Administrative databases | Bupropion SR | Yes | Fluoxetine, paroxetine, sertraline, TCAs, venlafaxine | Not applicable | Neutral | Neutral |
Wan et al (Reference Wan, Crown and Berndt2002) A | Administrative databases | Venlafaxine | Yes | SSRIs | Not applicable | Neutral | Neutral |
Table 3 Non-industry-sponsored economic outcome studies of antidepressant therapy
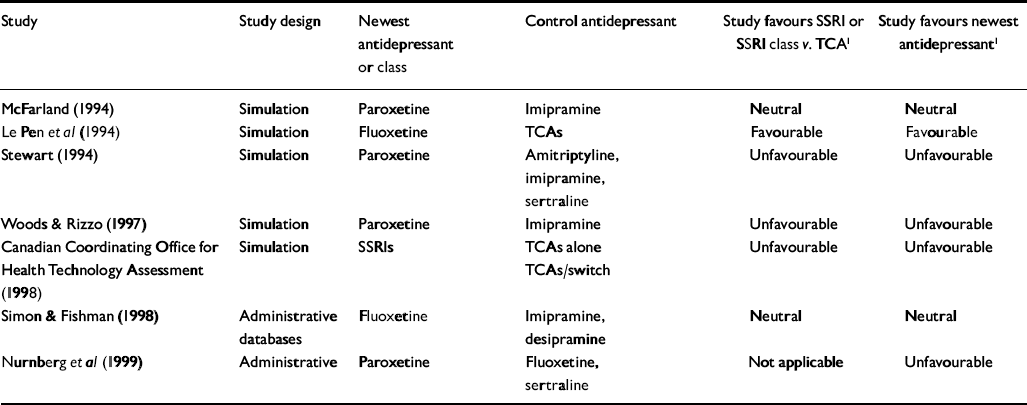
Study | Study design | Newest antidepressant or class | Control antidepressant | Study favours SSRI or SSRI class v. TCA1 | Study favours newest antidepressant1 |
---|---|---|---|---|---|
McFarland (Reference McFarland1994) | Simulation | Paroxetine | Imipramine | Neutral | Neutral |
Le Pen et al (Reference Le Pen, Levy and Ravily1994) | Simulation | Fluoxetine | TCAs | Favourable | Favourable |
Stewart (Reference Stewart1994) | Simulation | Paroxetine | Amitriptyline, imipramine, sertraline | Unfavourable | Unfavourable |
Woods & Rizzo (Reference Woods and Rizzo1997) | Simulation | Paroxetine | Imipramine | Unfavourable | Unfavourable |
Canadian Coordinating Office for Health Technology Assessment (1998) | Simulation | SSRIs | TCAs alone TCAs/switch | Unfavourable | Unfavourable |
Simon & Fishman (Reference Simon and Fishman1998) | Administrative databases | Fluoxetine | Imipramine, desipramine | Neutral | Neutral |
Nurnberg et al (Reference Nurnberg, Hensley and Thompson1999) | Administrative | Paroxetine | Fluoxetine, sertraline | Not applicable | Unfavourable |
Association of sponsorship
SSRI v. TCA analysis
For the primary analysis of industry v. non-industry sponsorship of SSRI v. TCA studies, six of seven non-industry-sponsored studies were eligible for analysis (see Table 3). Seventeen industry studies were eligible (see Tables 1 and 2).
Distribution and results for Fisher's exact test are noted in Table 4. The association between industry sponsorship and outcome favouring SSRIs v. TCAs was statistically significant. Each of the two secondary analyses contrasting studies with industry-employed authors v. non-industry-sponsored studies and contrasting studies with industry funding alone v. non-industry-sponsored studies demonstrated a statistically significant association between industry sponsorship and outcome favouring SSRIs v. TCAs, with probability values of 0.0420 and 0.0163 respectively.
Table 4 Fisher's exact test: distributions and probabilities
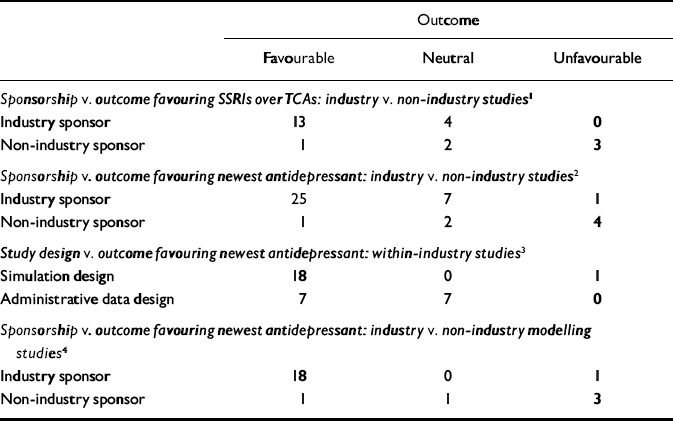
Outcome | |||
---|---|---|---|
Favourable | Neutral | Unfavourable | |
Sponsorship v. outcome favouring SSRIs over TCAs: industry v. non-industry studies 1 | |||
Industry sponsor | 13 | 4 | 0 |
Non-industry sponsor | 1 | 2 | 3 |
Sponsorship v. outcome favouring newest antidepressant: industry v. non-industry studies 2 | |||
Industry sponsor | 25 | 7 | 1 |
Non-industry sponsor | 1 | 2 | 4 |
Study design v. outcome favouring newest antidepressant: within-industry studies 3 | |||
Simulation design | 18 | 0 | 1 |
Administrative data design | 7 | 7 | 0 |
Sponsorship v. outcome favouring newest antidepressant: industry v. non-industry modelling studies 4 | |||
Industry sponsor | 18 | 0 | 1 |
Non-industry sponsor | 1 | 1 | 3 |
New v. old antidepressant analysis
All non-industry-sponsored studies were eligible (see Table 3). Thirty-three industry-sponsored studies (see Tables 1 and 2) were eligible. Distribution and results of Fisher's exact test are noted in Table 4. The association between industry sponsorship and outcome favouring the newest antidepressant was statistically significant. Each of the two secondary analyses contrasting studies with industry-employed authors v. non-industry-sponsored studies and contrasting studies with industry funding alone v. non-industry-sponsored studies demonstrated a statistically significant association between industry sponsorship and outcome favouring the newest antidepressant, with probability values of 0.0047 and 0.0018 respectively.
Association between study design and sponsorship bias
Question 1
Within industry-sponsored studies, is there a difference in tendency to favour the sponsor's drug over a competitor's drug or drug class, based on type of study design? For the principal analysis, ‘favouring the sponsor's drug or drug class’ was defined based on favouring the newest drug among all manufacturer-sponsored studies. Thirty-three industry-sponsored studies were eligible (see Tables 1 and 2). Distribution and results of the Fisher's exact test are noted in Table 4. The association between modelling v. administrative study design and outcome favouring the newest drug was statistically significant.
For our alternative analysis based on whether the sponsor's drug or drug class won, regardless of whether it was newest, all 38 industry-sponsored studies were eligible (see Tables 1 and 2). This analysis yielded a probability value of 0.0011, consistent with the results in the primary analysis.
Question 2
Between industry-sponsored and non-industry-sponsored modelling design studies, is there a difference in outcome patterns? For the principal analysis of this question we examined the patterns of favouring the newest drug. Nineteen industry studies (see Tables 1 and 2) and five non-industry studies (see Table 3) were eligible. The distribution and results of the Fisher's exact test are noted in Table 4. The association between industry v. non-industry sponsorship of modelling studies and outcome favouring the newest drug was statistically significant. Each of the two secondary analyses contrasting studies with industry-employed authors v. non-industry-sponsored studies and contrasting studies with industry funding alone v. non-industry-sponsored studies demonstrated a statistically significant association between industry sponsorship and outcome favouring the newest antidepressant in modelling studies, with probability values of 0.0010 and 0.0100 respectively.
In an alternative analysis we examined the patterns of favouring SSRIs v. favouring TCAs in modelling studies. We performed this analysis with the five eligible non-industry-sponsored studies (see Table 3) contrasted first with all twelve eligible industry-sponsored modelling studies (see Tables 1 and 2) that included SSRI v. TCA comparisons, and then with the six eligible modelling studies sponsored by SSRI manufacturers (see Table 1) that included SSRI v. TCA comparisons. The results of the Fisher's exact test in the two cases were 0.0139 and 0.0151 respectively, indicating that the tendency for industry-sponsored simulations to favour SSRIs more often than non-industry-sponsored studies is unlikely to be due to chance.
The sensitivity analysis varying the marginal cost-effectiveness threshold from $20 000 to $100 000 per QALY did not change any of the results reported above.
DISCUSSION
Our analyses show that, regardless of how the question was operationalised, for each of our study questions there was greater than chance association between study sponsorship and outcome. Among industry-sponsored v. non-industry-sponsored studies, industry-sponsored studies more frequently reported results favourable to the industry sponsor than did non-industry-sponsored studies. This was true whether industry sponsorship was defined as industry authorship, industry financial support alone, or both. Among industry studies, modelling studies were more likely to report results favourable to the sponsor than administrative data studies. Between industry-sponsored and non-industry-sponsored modelling design studies, industry studies were more likely to report results favourable to industry.
Consistency with prior studies
Our overall finding of sponsorship bias is consistent with prior studies in that all three prior fully reported studies found some association between study sponsorship and outcomes (Reference Azimi and WelchAzimi & Welch, 1998; Reference Friedberg, Saffran and StinsonFriedberg et al, 1999; Reference Neumann, Sandberg and BellNeumann et al, 2000a ). However, any detailed comparison between our study and previous studies is necessarily limited given that the previous studies mixed drugs, devices and other health interventions, and mixed various classes of medicines (Reference Azimi and WelchAzimi & Welch, 1998; Reference Neumann, Sandberg and BellNeumann et al, 2000a ); focused on qualitative conclusions (Reference Friedberg, Saffran and StinsonFriedberg et al, 1999); and used various definitions to select the specific study outcomes to be analysed (Reference Azimi and WelchAzimi & Welch, 1998; Reference Friedberg, Saffran and StinsonFriedberg et al, 1999; Reference Neumann, Sandberg and BellNeumann et al, 2000a ). The study by Friedberg et al (Reference Friedberg, Saffran and Stinson1999) of oncology drugs is perhaps most comparable with our current study, given their focus on a single pharmaceutical class and their categorisation of study conclusions as favourable, neutral or unfavourable, although they focused on qualitative rather than quantitative conclusions. Like our study, that of Friedberg et al did find an association between study conclusion and funding source.
Support for concern about modelling studies
In addition to supporting the general concern about sponsorship bias in pharmacoeconomic studies, our findings support the more specific concerns that have been raised about the potential for bias in modelling studies (Reference LuceLuce, 1995; Reference O'BrienO'Brien, 1996; Reference SheldonSheldon, 1996; Reference Maynard and CooksonMaynard & Cookson, 1998; Reference McCabe and DixonMcCabe & Dixon, 2000). Such support stems from the combination of our two findings regarding study design: among industry studies, modelling studies are more favourable to the sponsor than administrative studies, and in a comparison of industry-sponsored and non-industry-sponsored modelling studies, studies sponsored by industry are significantly more favourable to industry.
Limitations of our study
Our study has clear limitations. Randomised pharmacoeconomic trials could not be compared on the basis of sponsorship because there were only two such trials in this area. Relatively few non-industry-sponsored studies were available. We examined only one class of medications; analyses of other classes of medications should be conducted.
Bias v. accuracy
Although we have demonstrated several associations between study sponsorship and outcome, these associations do not suggest which (if either) side presents a more accurate estimate of relative pharmacoeconomic outcome. Both industry-supported and non-industry-supported researchers may be subject to forces that could potentially bias their work (Reference Yee and HillmanYee & Hillman, 1997; Reference DrummondDrummond, 1998; Reference Rennie and LuftRennie & Luft, 2000). Additionally, journal editorial processes can result in a biased sample of studies being published. It has been observed that journals tend to publish studies with ‘positive’ rather than ‘negative’ results (Reference Freemantle and MasonFreemantle & Mason, 1997).
Causes of bias
Many ideas have been offered to explain how sponsorship could result in biased reported outcomes (Reference Udrarhelyi, Graham and RaiUdrarhelyi et al, 1992; Reference Freemantle and MasonFreemantle & Mason, 1997; Reference DrummondDrummond, 1998; Reference CookCook, 1999; Reference Neumann, Sandberg and BellNeumann et al, 2000a ; Reference Rennie and LuftRennie & Luft, 2000). Industry, motivated to enhance sales of its products, might only pursue studies on products and select comparators that would yield favourable results. They might select biased populations within administrative data-sets, overtly or subtly influence analytical methods or models, or veto submission for publication of studies yielding unfavourable results. Non-industry-sponsored researchers might bias the studies submitted for publication in similar ways, although perhaps from different motivations such as controlling formulary costs, personal or academic rivalries, or career promotion.
We are unable to pinpoint the causes of bias among the reports analysed here. Examination of the individual studies does not reveal a common element that differ differentiates industry-sponsored from non-industry-sponsored studies; rather, the methodological limitations in the studies vary widely. These limitations have been discussed extensively elsewhere (Reference Hotopf, Lewis and NormandHotopf et al, 1996; Woods & Baker, Reference Woods and Baker1997, Reference Woods, Baker, Davis, Coyle and Charney2002). However, at least two suggested causes seem unlikely. First, some commentators have noted the potential role of selection bias - i.e. the tendency of researchers not to submit and of journals not to publish small studies or studies with negative statistical outcomes (Reference Freemantle and MasonFreemantle & Mason, 1997; Reference NeumannNeumann, 1998). This would help to explain how an overall preponderance of statistically positive studies could exist even if there were true uncertainty about alternative medications (Reference Djulbegovic, Lacevic and CantorDjulbegovic et al, 2000). The difference we have shown between industry-sponsored and non-industry-sponsored studies suggests that submission or editorial selection bias based on statistical significance alone does not adequately explain the bias in the present case. Second, it has been suggested that a particular sponsor weeds out weak alternatives among its drugs in early preliminary processes; therefore, drugs that reach the stage of being marketed are strong competitors and likely to yield analyses that favour the sponsor's drug (Reference GagnonGagnon, 2000). However, these same strong competitors performed less well in non-industry-sponsored studies, as shown clearly in our analysis of outcomes favouring either SSRIs or TCAs. Moreover, it should be noted that in the 18 studies with head-to-head comparisons among such strong competitors, the sponsor's drug lost only once (Reference Einarson, Arikian and SweeneyEinarson et al, 1995).
Bias in efficacy v. pharmacoeconomic studies
It is not possible to comment about whether the bias revealed in the current study of pharmacoeconomic reports of antidepressants is any greater or less than the sponsorship bias that may exist in efficacy studies of antidepressants. There is no published report on sponsorship bias in efficacy studies in any medication category within psychiatry. The only published report devoted to such quantitative analysis of psychiatric medications is a letter reviewing efficacy studies of any psychiatric medication in one journal over a 1-year period (Reference MandelkernMandelkern, 1999). This author reported a tally for industry-supported studies of 16 favourable to the manufacturer's drug and none unfavourable, and for unsupported studies 10 favourable and 6 unfavourable, concluding that there was a correlation between source of support and efficacy outcome. In other areas of medicine, bias has been demonstrated repeatedly in efficacy studies (Reference DavidsonDavidson, 1986; Reference Rochon, Gurwitz and SimmsRochon et al, 1994; Reference Stelfox, Chua and O'RourkeStelfox et al, 1998; Reference Djulbegovic, Lacevic and CantorDjulbegovic et al, 2000). A study of sponsorship bias in efficacy trials of antidepressants would provide a useful comparison for our study.
It is important for pharmacoeconomic studies to attempt to give estimates that are as accurate and uninfluenced by bias as possible, given the large and growing number of health care dollars spent on medications. Pharmaceutical sales for North America were reported to be US$153 billion in 2000, representing a 14% growth over the previous year (IMS Health, 2001). Owing to the importance of cost constraint in medicine the volume of pharmacoeconomic research has been growing (Reference DetskyDetsky, 1994) and is linked to governmental purchasing decisions in some jurisdictions (Canadian Coordinating Office for Health Technology Assessment, 1994; Ontario Ministry of Health, 1994; Australian Government, 1995). However as we noted previously, financial and other incentives create strong motives for bias. Our results for antidepressants suggest that actual bias related to sponsorship appears to exist, although whether or how the bias and specific motives are related cannot be determined. Until the mechanisms producing the bias are better understood, interpretation of results from pharmacoeconomic studies should take sponsorship into account.
Clinical Implications and Limitations
CLINICAL IMPLICATIONS
-
▪ Evaluation of pharmacoeconomic studies of antidepressants for treatment of major depression should take into account the source of study funding and of authorship.
-
▪ Evaluation of modelling studies in this area may warrant additional scrutiny.
-
▪ Evaluation of pharmacoeconomic studies should be informed by a thorough understanding of their methodology.
LIMITATIONS
-
▪ Randomised pharmacoeconomic trials could not be compared because of their scarcity.
-
▪ Few non-industry-sponsored trials were available.
-
▪ Only one class of medication was examined.
Acknowledgements
Consultancies, research grants or unrestricted grants were received from. Pfizer, Bristol-Myers Squibb, Eli Lilly & Co. and Forest Laboratories. The. work was supported in part by PHS KO8 MH-01718 (C.B.B.), NARSAD R03051 (C.B.B.), Robert Wood Johnson Foundation (M.L.C.), Substance Abuse and Mental. Health Services Administration (M.L.C.), Meadows Foundation (M.L.C.), Houston. Endowment (M.L.C.), Hogg Foundation (M.L.C.), Texas Department of Mental. Health and Mental Retardation (M.L.C.) and PHS MH-54446 (S.W.W.).
eLetters
No eLetters have been published for this article.