Suicide is one of the largest health burdens in industrialised nations. Reference Hawton and van Heeringen1 The Organisation for Economic Co-operation and Development 2 reports that suicide mortality rates in Austria are constantly above the European average. In the period 2005–2009 the mean Austrian suicide rate was 15.7 per 100 000 people showing a distinctive gender gap with a suicide rate ratio of 3.3:1 in favour of women. Suicide is a complex phenomenon affected by a variety of interwoven risk and protective factors (i.e. sociodemographic characteristics, access to health services and environmental factors). Reference Kapusta, Mossaheb, Etzersdorfer, Hlavin, Thau and Willeit3,Reference Hawton, Casanas, Haw and Saunders4 The complexity of suicide epidemiology is amplified because of considerable risk variations between nations and within countries. 2,Reference Helbich, Leitner and Kapusta5,Reference Gunnell, Wheeler, Chang, Thomas, Sterne and Dorling6,Reference Balint, Dome, Daroczid, Gonda and Rihmer7 Mental disorders, especially depression and bipolar disorders, are well-known risk factors for suicide, increasing mortality by up to 20 times. Reference Harris and Barraclough8 Because of its mood-stabilising effects and suicide preventive properties, lithium is used for acute and prophylactic treatments in psychiatry. Reference Müller-Oerlinghausen and Lewitzka9,Reference Bschor10 Meta-analyses of clinical trials have found that suicide risk among people affected by mood disorders is significantly reduced when they are treated with lithium. Reference Cipriani, Pretty, Hawton and Geddes11,Reference Baldessarini, Tondo, Davis, Pompili, Goodwin and Hennen12 A cohort study in Denmark concluded that lithium treatment diminishes suicide risk independently of a person's age and gender, even though the suicide risk is approximately 10 times higher for patients taking lithium than the general population. Reference Kessing, Søndergård, Kvist and Andersen13 However, whereas the effects of therapeutic dosages of lithium are well understood there is a lack of knowledge on the effects of natural lithium intake.
Lithium, as a natural trace element, is eluded from rock and soil and accumulates in groundwater. These natural doses are significantly lower than those used in therapeutic treatment. Reference Schrauzer and Shrestha14 It is assumed that long-term exposure to even minor lithium levels might act as a suicide preventive. However, scientific evidence is far from conclusive and contradictory empirical results have been reported for different regions. Among one of the first studies reporting a negative effect of natural lithium in drinking water on suicide was conducted in Texas, USA. Reference Schrauzer and Shrestha14 After adjusting for risk factors, a more recent study in this area confirmed that lithium in public water is negatively but weakly related to suicide mortality. Reference Blüml, Regier, Hlavin, Rockett, König and Vyssoki15 In the Oita prefecture in Japan, lithium levels in tap water were found to diminish suicide risk significantly, Reference Ohgami, Terao, Shiotsuki, Ishii and Iwata16 even though methodological flaws have been highlighted. Reference Chandra and Babu17,Reference Huthwaite and Stanley18 Sugawara et al Reference Sugawara, Yasui-Furukori, Ishii, Iwata and Terao19 reported gender differences with only a significant negative association between lithium in drinking water and suicide mortality among Japanese women. These studies sparked interest in Europe. For the East of England and prefectures in Greece neither significant associations between suicide and lithium in public water supply nor gender differences were found. Reference Kabacs, Memon, Obinwa, Stochl and Perez20,Reference Giotakos, Nisianakis, Tsouvelas and Giakalou21 In contrast, in Austria the natural lithium–suicide association was confirmed, although statistical significance was limited to eastern regions. Reference Helbich, Leitner and Kapusta5
Previous studies have assumed that the lithium in drinking water originated from natural sources alone (the natural lithium hypothesis). They have not considered whether lithium prescribed to patients may have accumulate via waste water in groundwater aquifers after urinary excretion and interplay with natural lithium. Reference Concha, Broberg, Grandér, Cardozo, Palm and Vahter22 Strong evidence exists that pharmaceuticals in waste water give rise to public health problems such as antibiotics causing resistant bacteria in waste water. Reference Huang, Hu, Lu, Li, Tang, Lu and Wei23,Reference Rodríguez, Navarro-Villoslada, Benito-Peña, Marazuela and Moreno-Bondi24 Pharmaceuticals, such as carbamazepine and ibuprofen, and also biphosphenol A have been detected in Canadian drinking water, Reference Kleywegt, Pileggi, Yang, Hao, Zhao and Rocks25 and acetaminophen caffeine, carbamazepine, codeine have been found in drinking water in California. Reference Fram and Belitz26 There is some evidence that the regions with high prescriptions of pharmaceuticals, such as in Los Angeles, have higher levels of pharmaceuticals in groundwater than other regions in California. Reference Fram and Belitz26 In the USA, levels of the common antidepressant fluoxetine were measurable in 4.3% of all investigated groundwater sites. Reference Barnes, Kolpin, Furlong, Zaugg, Meyer and Barber27 Nentwig Reference Nentwig28 confirmed that the effects of fluoxetine and carbamazepine concentrations in sediment and water systems were strong enough to affect health. Therefore, it is assumed that lithium may accumulate in drinking water reservoirs and modify its natural occurrence and thus may be responsible for a non-random distribution of lithium in drinking water across regions. Although the findings discussed above have contributed significantly to our knowledge about the epidemiology of suicide, no research known to the authors deals with the relationship between prescribed lithium-based pharmaceuticals, natural lithium levels in drinking water and suicide mortality. In addition, gender differences are rarely considered separately, as higher and lower suicide rates tend to cluster spatially. Our study addresses these research gaps and focuses on the following research questions: (a) are lithium levels in drinking water associated with lithium prescriptions; (b) is there statistical evidence that any association found between suicide and lithium levels in drinking water is a function of lithium prescription rates operating as a moderating effect; and (c) are there gender-specific variations concerning both lithium-based variables (i.e. drinking water and lithium prescriptions) while controlling for other risk factors? To examine whether the natural lithium hypothesis holds true or whether the lithium levels in drinking water may result from the accumulation of lithium prescriptions in waste water (lithium prescription hypothesis), we generated the following hypotheses. First, districts with a high lithium level in drinking water also have high lithium prescription rates and both lithium sources are inversely associated with suicide rates, even when risk and protective factors are adjusted for. Second, natural lithium levels in drinking water have a suicide protective effect, even when adjusted for lithium prescriptions. Besides being vital for health prevention strategies, testing the lithium prescription hypothesis is of importance to medicine, public health and environmental science.
Method
Study area
The empirical analysis was conducted in Austria using an ecological study design. Austria represents an excellent case study to explore the potential interaction between prescriptions rates and lithium in drinking water on suicide mortality. This is because of (a) its unique topography leading to different geological bedrocks and thus different natural lithium levels, (b) its alpine areas with reduced health service accessibility and (c) knowledge about the existence of essential confounding risk factors, therefore reducing the risk of an omitted variable bias. The study is based on 99 administrative territorial units (districts) from which suicide cases were available. In order to link the suicide numbers with prescription data available for each sanitation district, a few administrative districts were carefully aggregated to match the sanitation districts (n = 90).
Data
The suicide data were collected from the Austrian mortality database, maintained by Statistics Austria (http://www.statistik.at/web_en/), on a case level. Suicide data comprise all registered suicides across all ages (with the youngest in the 5–9 age group and the oldest in the 95+ age group) within the 5-year period from 2005 to 2009. In accordance with ICD-10 29 the data include the following causes of death: poisoning (X60–X69), hanging (X70), drowning (X71), shooting (X72–X74), jumping (X80) and other less frequent causes of death (X75–X79, X81–X84). As suicide mortality is a rare event, the annual data were pooled over time to reduce the random fluctuations of suicides within a district. Moreover, because the raw number of suicides per district depends on the underlying population at risk, age and gender-adjusted standardised suicide mortality ratios (SMRs) were calculated. On average, the gender ratio in Austria is approximately 51% women and 49% men. The population counts extracted from the census of 2001 (Statistics Austria) serve as a baseline. The SMRs reflect the ratio between observed and expected suicides within each district. Mapping of the total SMR (SMRt) and gender-specific SMRs for males and females (SMRm, SMRf, respectively) in Fig. 1 indicate spatial heterogeneity in risk. Southern areas are faced with a more distinct risk compared with northeastern areas.

Fig. 1 Spatial distribution of the total and gender-specific suicide rates.
Suicide mortality ratios (a) total, (b) males and (c) females. Dark grey lines delimit federal states; light grey lines define sanitation district boundaries.
Besides suicide data, two other data-sets of prime interest were obtained, namely the lithium content in drinking water and lithium pharmaceuticals sales. Data about the former were provided by AQA GmbH (http://www.aqa-online.com) and came from 6460 water samples from across Austria. Reference Kapusta, Mossaheb, Etzersdorfer, Hlavin, Thau and Willeit3 To merge the individual lithium measurements with the district-level data, the mean lithium concentration per district was computed and log transformed to a Gaussian-like distribution (logLITH). On average the lithium content was 0.010 mg/L with a standard deviation of 0.010 mg/L. The lowest lithium level was 0.003 mg/L, which corresponds to the minimum measurement accuracy of the spectrometry. Figure 2(a) shows a geographically clear spatial variation, following an east–west trend. Eastern regions have higher lithium levels (>0.016 mg/l) than western parts (0.004 mg/l).

Fig. 2 Spatial distribution of lithium-based variables.
(a) Logged natural lithium in drinking water and (b) logged lithium prescriptions. Dark grey lines delimit federal states; light grey lines define sanitation district boundaries.
Data on lithium pharmaceuticals sales in pharmacies were obtained from IMS Health (http://www.goeg.at/en). The number of prescriptions were based on sold units (i.e. packages, tablets) for the two lithium-based medical products, namely Quilonorm and Neurolepsin (lithium-carbonate), used in psychiatric treatment in 2010 and 2011 and these were converted to the daily defined dose (DDD). As depicted in Fig. 2(b), logged lithium DDDs sold per km2 (logPHARM) increased with urbanisation and is exceptionally high in the (provincial) capital cities including Vienna (1360.2 DDD per km2), Graz (1286.2 DDD per km2) and Linz (894.8 DDD per km2). The nationwide average is approximately 85 DDD per km2 with a standard deviation of 85.2.
The selection of the considered controls was based upon data availability and suggestions from the literature. Reference Hawton, Casanas, Haw and Saunders4 To account for known risk factors, the most complete data on the proportion of Roman Catholics (ROMCAT), population density (POPDENS) and average income per capita (INCOME) were obtained from Statistics Austria for the year 2001. Additional data on the density of psychiatrists per 10 000 population for the year 2007 (PSYC), the number of general practitioners for 2007 (GP) (both provided by the Austrian Medical Chamber), and the density of psychotherapists per 10 000 people for the year 2005 (PT) were obtained from the Austrian Institute of Health to account for possible protective effects of the local psychosocial services. Average unemployment rates for the period 2005–2008 per district (UNEMPL) were collected from the Austrian Public Employment Service. Finally, in order to take spatial heterogeneity in suicide risks into account, Reference Helbich, Leitner and Kapusta5 the 99 districts from nine federal states were aggregated into four larger regions (west – Vorarlberg, Tyrol; south – Carinthia, Styria; central – Salzburg, Upper Austria; and east – Lower Austria, Vienna, Burgenland) and considered as regional dummy variables (REG) in the analysis.
Statistical analysis
Conditional plots were utilised to explore the associations between the two lithium-based variables conditioned on certain ranges of the SMRs. Because of the exceptionally high lithium prescription densities in capital cities, as well as peculiarities within their water supplies separate conditional plots with and without capital cities were drawn. Supportive bivariate Spearman's ρ correlation coefficients were calculated to investigate the nature and magnitude of associations between variables. As indicated by prior studies, Reference Gunnell, Wheeler, Chang, Thomas, Sterne and Dorling6,Reference Balint, Dome, Daroczid, Gonda and Rihmer7 SMRs do not exhibit a spatially random distribution, which has serious consequences for multivariate non-spatial analysis (i.e. ordinary least squares regression) assuming that observations are independent of each other. To quantify the extent to which a district value is similar to the adjacent district values, Moran's I statistic was calculated. Reference Helbich, Leitner and Kapusta5 Positive values smaller or equal to +1 refer to positive spatial autocorrelation (i.e. similar values are spatially nearby), whereas negative values larger or equal to − 1 indicate negative spatial autocorrelation (i.e. dissimilar values are nearby). A Moran's I statistic of slightly below 0 confirms spatial independence. Significance was tested based on pseudo P-values obtained from 9999 permutations against the null hypothesis of spatial randomness providing the foundation for spatial regression modelling.
To control for potential confounding factors, multivariate spatial Bayesian hierarchical models were implemented. Reference Gunnell, Wheeler, Chang, Thomas, Sterne and Dorling6 This suite of regression models provides a powerful approach to model spatial autocorrelation based on unmodelled risk effects, actual spatial patters in SMRs, etc. Spatial correlations were accounted for at a second hierarchical level by means of spatial random effects, such as the conditional autoregressive (CAR) prior distribution. Supported by a simulation study, Reference Lee30 this research applies CAR priors after Leroux (for a discussion see Lee Reference Lee30 ) permitting locally differentiated smoothing. On that basis, the random effects were set up with independent and diffuse Gaussian priors and the model estimation utilised Markov chain Monte Carlo (MCMC) simulations. As the number of confounders was large, some may feature multicollinearity. Since the impact of individual confounders is of secondary interests, principal component analysis (PCA) was employed to obtain a reduced set of latent variables (principal components) that are uncorrelated and depict the overall risk factor variability.
Results
Bivariate relationships between total and gender-specific SMRs, as well as logLITH and logPHARM were investigated using Spearman correlations (Table 1). LogLITH is, as expected, consistently negatively related with all three SMRs. Similarly, with the exception of SMRf, logPHARM is statistically significantly negatively associated with SMRt and SMRm at least at the 0.05 level, meaning that areas with high prescription rates face lower suicide mortality. Moreover, there is a tendency (P<0.100) that logLITH is positively associated with logPHARM.
Table 1 Spearman correlation coefficients
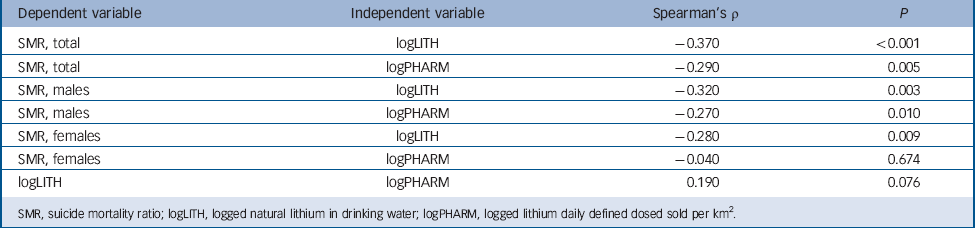
Dependent variable | Independent variable | Spearman's ρ | P |
---|---|---|---|
SMR, total | logLITH | −0.370 | <0.001 |
SMR, total | logPHARM | −0.290 | 0.005 |
SMR, males | logLITH | −0.320 | 0.003 |
SMR, males | logPHARM | −0.270 | 0.010 |
SMR, females | logLITH | −0.280 | 0.009 |
SMR, females | logPHARM | −0.040 | 0.674 |
logLITH | logPHARM | 0.190 | 0.076 |
SMR, suicide mortality ratio; logLITH, logged natural lithium in drinking water; logPHARM, logged l ithium daily defined dosed sold per km2.
To further explore the association between the lithium-based variables for different ranges of SMRs, conditional plots are shown in Fig. 3. Capital cities have high lithium prescription densities and function as leverage points dominating the regression line. In general, scatter plots indicate that logLITH and logPHARM were positively associated across different ranges of SMRs and gender. As logPHARM is much more pronounced in cities and thus distorts associations, it is mandatory to control for that issue. In this regard, population density seems to be a rational proxy variable. Figure 3(b), (d) and (f) show a slight tendency that in higher suicide risk areas the logLITH–logPHARM association is more positive than in lower risk areas. However, focusing on a specific SMR range reduces the sample size and results should be interpreted with caution.

Fig. 3 Conditional plots.
Solid lines refer to a linear regression and dotted lines to a locally weighted polynomial regression. Suicide mortality ratios (a) total, (b) total, without capital cities; (c) males, (d) males, without capital cities; (e) females and (f) females, without capital cities. Note (a), (c) and (e) are based on all data whereas (b), (d) and (f) do not consider data from eight provincial capital cities and Vienna. logPHARM, logged lithium daily defined dose sold per km2; logLITH, logged natural lithium in drinking water.
Furthermore, to evaluate the spatial patterns of each variable, the Moran's I statistic with the five nearest neighbours were investigated ensuring that each district has a similar number of neighbours. Table 2 lists the results. The Moran's I statistic is highly significant for SMRt and the SMRm, indicating that similar values are located nearby. This finding is critical for subsequent analysis calling for a spatially explicit regression. Furthermore, Spearman correlations point to multicollinearity between some continuous risk factors requiring PCA to establish some uncorrelated principal components. The first three principal components, explaining in total approximately 84% of the overall variance, were selected for further analysis. Principal component (PC)1 explains 58% of the variance, PC2 15%, and PC3 11%. In brief, the variables logPOPDENS, logINCOME, logPSYC, logPT and logGP load moderate negatively on PC1, whereas logUNEMPL loads lower negatively. In contrast, logROMCAT has a positive loading. LogUNEMPL possesses a negative loading on PC2, whereas other variables show minor positive or negative loadings. PC3 is mainly shaped by logGP and logROMCAT, which both having a strong positive loading. Even though more difficult to interpret, these three PCs are used subsequently to adjust for confounding factors in the regressions.
Table 2 Moran's I results

Moran's I | P | |
---|---|---|
SMR, total | 0.414 | <0.001 |
SMR, males | 0.386 | <0.001 |
SMR, females | 0.067 | 0.104 |
logLITH | 0.539 | <0.001 |
logPHARM | 0.083 | 0.059 |
SMR, suicide mortality ratio; logLITH, logged natural lithium in drinking water; logPHARM, logged lithium daily defined dose sold per km2.
To account for unmodelled residual patterns not explained by the three principal components, spatial Bayesian hierarchical models were estimated. In total, six models were run, namely two each for total, male and female. The modelling strategy comprises the following two models with rising complexity. First, individual effects of logLITH and logPHARM on SMRs were tested, while adjusting for a variety of risk factors included as principal components. These models are referred to as ‘model 1’. Second, the moderating effect of logPHARM on logLITH was tested by means of extending the previous model with a logLITH–logPHARM interaction term, representing ‘model 2’. The deviance information criterion (DIC) serves for model comparison. A lower DIC score refers to a better fit. All models were set up on 100 000 MCMC samples, with 20 000 samples discarded during the burn-in period. To incorporate the geography, the models used a neighbourhood matrix based on the five nearest neighbours. Sensitivity runs, with a varying number of nearest neighbours, confirmed the robustness of the model specifications. All DIC scores suggest that models of type 1 rule out type 2 models. Residual independence was confirmed by the Moran's I statistics, supporting the conclusion that spatial autocorrelations were captured appropriately. The posterior distribution of the spatial correlation (ρ) results in a moderate spatial correlation even after controlling for risk factors. Tables 3 and 4 summarise the results of these six models, including the estimated model parameters (i.e. the posterior medians) and the 95% credible intervals (similar to confidence intervals). It should be noted that if the 95% credible interval of a variable includes zero, it can be concluded that its effect is of minor relevance.
Table 3 Results of the estimated models for suicide mortality ratio, total

Model 1: without interaction effect | Model 2: with interaction effect | |||||||
---|---|---|---|---|---|---|---|---|
Credible interval | Credible interval | |||||||
Median | 2.5% | 97.5% | P | Median | 2.5% | 97.5% | P | |
Intercept | 0.483 a | 0.144 | 0.827 | 0.441 | −0.212 | 1.113 | ||
logLITH | −0.080 a | −0.136 | −0.024 | −0.088 | −0.214 | 0.041 | ||
logPHARM | 0.015 | −0.032 | 0.062 | 0.029 | −0.176 | 0.226 | ||
logLITH × logPHARM | 0.003 | −0.038 | 0.042 | |||||
PC1 | 0.035 a | 0.004 | 0.065 | 0.035 a | 0.004 | 0.065 | ||
PC2 | 0.022 | −0.009 | 0.052 | 0.021 | −0.009 | 0.052 | ||
PC3 | 0.052 a | 0.018 | 0.085 | 0.052 a | 0.019 | 0.084 | ||
RegEAST | −0.188 a | −0.322 | −0.063 | −0.190 a | −0.328 | −0.066 | ||
RegMID | −0.149 a | −0.248 | −0.042 | −0.149 a | −0.250 | −0.039 | ||
RegWEST | −0.147 a | −0.250 | −0.038 | −0.147 a | −0.247 | −0.036 | ||
ρ | 0.370 a | 0.040 | 0.860 | 0.390 a | 0.050 | 0.870 | ||
DIC | −190 | −186 | ||||||
Moran's I errors | −0.019 | 0.537 | −0.013 | 0.488 |
logLITH, logged natural lithium in drinking water; logPHARM, logged lithium daily defined dose sold per km2; PC1, first principal component; PC2, second principal component; PC3, third principal component; Reg, regional dummy variable; MID, central area of Austria; DIC, deviance information criterion.
a. There is strong evidence that this variable is relevant.
Table 4 Results of the estimated models for gender-specific suicide mortality ratios

Model 1: without interaction effect | Model 2: with interaction effect | |||||||
---|---|---|---|---|---|---|---|---|
Credible interval | Credible interval | |||||||
Median | 2.5% | 97.5% | P | Median | 2.5% | 97.5% | P | |
Males | ||||||||
Intercept | 0.416 a | 0.024 | 0.826 | 0.674 | −0.089 | 1.472 | ||
logLITH | −0.092 a | −0.157 | −0.026 | −0.039 | −0.188 | 0.118 | ||
logPHARM | 0.026 | −0.029 | 0.082 | −0.065 | −0.311 | 0.168 | ||
logLITH × logPHARM | −0.019 | −0.068 | 0.028 | |||||
PC1 | 0.049 a | 0.012 | 0.084 | 0.050 a | 0.015 | 0.086 | ||
PC2 | 0.017 | −0.020 | 0.053 | 0.016 | −0.022 | 0.052 | ||
PC3 | 0.051 a | 0.012 | 0.090 | 0.051 a | 0.012 | 0.089 | ||
RegEAST | −0.221 a | −0.361 | −0.086 | −0.223 a | −0.368 | −0.086 | ||
RegMID | −0.161 a | −0.273 | −0.041 | −0.166 a | −0.277 | −0.042 | ||
RegWEST | −0.119 | −0.235 | 0.001 | −0.118 | −0.232 | 0.000 | ||
ρ | 0.360 a | 0.030 | 0.860 | 0.370 a | 0.030 | 0.880 | ||
DIC | −146 | −130 | ||||||
Moran's I errors | −0.016 | 0.517 | −0.020 | 0.530 | ||||
Females | ||||||||
Intercept | 0.613 | −0.009 | 1.254 | −0.110 | −1.360 | 1.156 | ||
logLITH | −0.044 | −0.148 | 0.059 | −0.191 | −0.432 | 0.052 | ||
logPHARM | −0.006 | −0.097 | 0.080 | 0.243 | −0.145 | 0.635 | ||
logLITH × logPHARM | 0.051 | −0.026 | 0.129 | |||||
PC1 | −0.009 | −0.064 | 0.045 | −0.011 | −0.066 | 0.043 | ||
PC2 | 0.029 | −0.031 | 0.090 | 0.031 | −0.029 | 0.092 | ||
PC3 | 0.060 | −0.003 | 0.122 | 0.061 | −0.002 | 0.124 | ||
RegEAST | −0.066 | −0.264 | 0.132 | −0.068 | −0.266 | 0.128 | ||
RegMID | −0.106 | −0.273 | 0.064 | −0.102 | −0.275 | 0.068 | ||
RegWEST | −0.245 a | −0.421 | −0.075 | −0.248 a | −0.417 | −0.076 | ||
ρ | 0.350 a | 0.010 | 0.880 | 0.370 a | 0.020 | 0.890 | ||
DIC | −19 | −13 | ||||||
Moran's I errors | −0.119 | 0.977 | −0.107 | 0.962 |
logLITH, logged natura llithium in drinking water; logPHARM, logged lithium daily defined dose sold per km2; PC1, first principal component; PC2, second principal component; PC3, third principal component; Reg, regional dummy variable; MID, central area of Austria; DIC, deviance information criterion.
a. There is strong evidence that this variable is relevant.
Model 1 provides considerable evidence that logLITH has the expected negative influence on suicide. This holds true for the total suicide ratio and for males. This means that higher natural lithium levels decrease the suicide risk. This inverse association is in line with previous studies. Reference Kapusta, Mossaheb, Etzersdorfer, Hlavin, Thau and Willeit3,Reference Blüml, Regier, Hlavin, Rockett, König and Vyssoki15 Even though the sign of the coefficient for females matches the results for SMRt and SMRm, statistical significance was not reached. However, for prescription rates (logPHARM), a contradictory result in comparison with the bivariate correlations was found. With the exception of the SMRf model, showing the anticipated negative sign, the models for SMRt and SMRm of type 1 have positive logPHARM effects, which seem counterintuitive. However, neither for SMRf nor for SMRt and SMRm do the models support that these predictors are of relevance, as the 95% credible intervals include zero. In a similar study in France, regional prescription rates were negatively associated with male suicide. Reference Bellanger, Jourdai and Batt-Moillo31
Models extended by logLITH–logPHARM moderating effects indicate that interactions were unrelated. This was also reflected in the weaker DIC scores. Furthermore, consideration of a lithium interaction effect in the three extended models 2 absorbed the significance of the originally important contribution of natural lithium, suggesting that logPHARM does not moderate the logLITH effect on suicide. Considerable gender differences were noticeable in the principal components controlling for potential confounders. Whereas the first and third principal components were relevant in modelling SMRt and SMRm, this conclusion was not supported by the SMRf model. Not surprisingly this confirms the widely recognised fact of gender-specific risk factors as well as a generally lower female suicide risk. Reference Hawton, Casanas, Haw and Saunders4 As indicated by regional dummies, evidence exists that suicide rates vary within Austria at a region-wide scale. Compared with southern areas, i.e. Carinthia and Styria, the other regions have a considerably lower suicide risk. This conclusion is valid throughout the models. In addition, small-scale suicide spill-over effects beyond regions were present, efficiently captured by all models. The ρ parameter indicates that complete suicide patterns cannot be explained by confounders alone. This mirrors previous findings Reference Gunnell, Wheeler, Chang, Thomas, Sterne and Dorling6,Reference Balint, Dome, Daroczid, Gonda and Rihmer7 emphasising that geography is a crucial factor in explaining suicide patterns.
Finally, the estimated suicide risks, represented as the posterior distributions of the fitted risk patterns of the best performing models (model 1), are illustrated in Fig. 4. Although a general comparison suggests similar risk patterns, a detailed inspection indicates striking differences across space and between genders. This impression is also confirmed by a correlation analysis between risk surfaces. A high correlation exists between the total risk and the risk for males with a Spearman's ρ of 0.970 (P<0.001). However, the total risk is lower when correlated with the female risk (ρ = 0.730, P<0.001) and the correlation between male and female is even lower (ρ = 0.610), although highly significant (P<0.001). It is apparent that suicide risk varies significantly across Austria. All risk surfaces show a rough trend of elevated risks from the north to the south with a more pronounced overall risk in the federal state of Styria, which has risk values above 1. Independently of this risk pattern, the Styrian district Voitsberg has, in particular, a distinctly elevated suicide risk. For that district the total risk is 1.142, which is even more pronounced for males (1.144). The north-eastern areas, including parts of Lower Austria, Vienna and northern Burgenland, have a reduced risk. For example, the total risk ranges from 0.476 to approximately 0.700. Remarkably, the estimated female risk surface has a comparable pattern but the magnitude of the risk does not exceed the critical value of 1 across the study area.

Fig. 4 Estimated suicide risk.
Suicide mortality ratios (a) total, (b) males and (c) females. Dark grey lines delimit federal states; light grey lines define sanitation district boundaries.
Discussion
Main findings
The objective of this study was to test the hypothesis whether pharmaceutical lithium prescriptions accumulating in groundwater together with natural lithium in drinking water jointly affect suicide mortality. Utilising spatial Bayesian hierarchical models we looked for this potential co-association in 99 districts in Austria. Although correlation analyses confirm that both natural lithium in drinking water and prescription rates are inversely associated with suicide and both lithium sources are weakly and positively associated, these conclusions are reversed, as soon as risk factors are adjusted for. This may also explain why prior studies Reference Ohgami, Terao, Shiotsuki, Ishii and Iwata16,Reference Kabacs, Memon, Obinwa, Stochl and Perez20,Reference Giotakos, Nisianakis, Tsouvelas and Giakalou21 using a bivariate research design have reported non-significant results regarding suicide and natural lithium levels. More importantly, the models in our study do not support the lithium prescription hypothesis. Both, for total and gender-specific suicide mortalities no evidence was found that lithium-based pharmaceuticals either directly or via accumulation in drinking water diminish the risk of death by suicide. Neither the individual effects nor the lithium interaction effect turned out to be significant when adjusted for confounders, suggesting that lithium in drinking water does not seem to originate from lithium-based pharmaceutical medications (i.e. Quilonorm, Neurolepsin) used in psychiatric treatments. This conclusion is independent of SMR stratification and consistent throughout the models providing additional support that suicide and lithium levels in drinking water are not a function of lithium prescription rates across Austria. However, natural lithium levels in drinking water still provide an explanation for the spatial distribution of suicide mortality. For both the total suicide rates and the male-specific rates the suicide-protective property of natural lithium is confirmed, with a stronger negative effect for males. This outcome corroborates previous studies Reference Kapusta, Mossaheb, Etzersdorfer, Hlavin, Thau and Willeit3,Reference Schrauzer and Shrestha14,Reference Blüml, Regier, Hlavin, Rockett, König and Vyssoki15 and emphasises the validity of the natural lithium hypothesis stating that lithium in drinking water likely originates from natural sources.
Our results also verify that suicide risk varies considerably across Austria, as indicated by the regional dummy variables that were consistently significant, even after carefully modelling suicide spill-over effects from adjacent districts through a spatial Bayesian model formulation. This supports findings from Hungary that showed that suicides are geographically agglomerated. Reference Balint, Dome, Daroczid, Gonda and Rihmer7 This also supports recent efforts towards a spatial turn in health science, where spatially explicit modelling approaches are receiving increasing attention. Reference Gunnell, Wheeler, Chang, Thomas, Sterne and Dorling6,Reference Helbich, Blüml, Leitner and Kapusta32 Compared with women, men are at greater risk of dying by suicide; Reference Hawton and van Heeringen1 this risk is evidently increased in the southern regions of Austria. In contrast, lowest risk exists in the north-east of Austria. This implies that suicide prevention strategies and programmes must reflect gender dissimilarities and local circumstances in risk patterns by providing specific localised health intervention strategies, such as reducing suicide risk factors and improving protective factors related to mental health well-being.
Strengths and limitations
By extending previous ecological lithium–suicide studies with the additional of a rational alternative lithium source, namely lithium prescription rates, our study adds to the literature. Besides controlling for a rich set of socioeconomic risk factors and confounding effects of access to health services, a key strength of the study is the larger sample size (of 99 districts) compared with most previous studies. Another major strength is the statistically sound analysis design, explicitly accounting for local suicide spill-over effects while simultaneously controlling for regional heterogeneity in suicide risk. However, some limitations can be identified. In contrast to cohort studies on an individual level, Reference Termorshuizen, Wierdsma, Visser, Drukker, Sytema and Laan33 the present models are cross-sectional and based on ecological regression. Thus, the district-related findings do not allow us to draw conclusions about individuals. Even though the utilised risk and protective factors successfully absorb confounding effects, a few variables could be further stratified by age and/or gender, which might have an impact on the model. Finally, lithium intake via nutrition is not taken into account, which might also contribute to daily lithium exposure. Reference Chandra and Babu17
Despite these reservations, the statistically significant inversely related association between lithium in drinking water and suicide support the validity of the natural lithium hypothesis. No evidence for lithium-based pharmaceutical medication effects on suicide could be confirmed, either as a direct effect or as a moderating effect via natural lithium in drinking water. It is likely, that in comparison with other psychopharmacological prescriptions such as antidepressants, Reference Kapusta, Niederkrotenthaler, Etzersdorfer, Voracek, Dervic and Jandl-Jager34 lithium prescriptions have only a minimal relieving effect on the nations burden of mental disease. Therefore spatial analysis of antidepressant prescriptions on suicide rates, such as that presented by Gibbons et al, Reference Gibbons, Hur, Bhaumik and Mann35 should be considered for further research.
Funding
This research was funded by the Austrian Science Fund (FWF) by grant P25474-B24 and through the Doctoral College GIScience (DK W 1237-N23).
Acknowledgements
We thank Jakob Klein for his support in data preparation and thank IMS Health, the worldwide information and service leader for the pharmaceutical and health industry, for the data on lithium prescriptions.
eLetters
No eLetters have been published for this article.