The ideal economic evaluation of a mental health service would allow the comparison of the cost-effectiveness of different mental health services with services in other clinical areas, such as cancer. In this way, decision makers could be informed about where the greatest net benefits could be obtained. The technique of cost-utility analysis was developed to allow such comparisons both within and across disease categories. In this framework costs are measured in monetary terms and outcomes are measured in a generic common unit such as quality-adjusted life-years (QALYs). A common convention is to define a value-for-money threshold (for example, $50 000/QALY) and to recommend funding of a service if its cost/QALY is below this threshold. Although the principle behind the technique of cost-utility analysis is attractive, the techniques that have been developed to assess generic health outcomes restrict comparability between studies. This means that the validity of comparability of cost-effectiveness between different services and interventions is diminished.
There are a large number of instruments used for evaluating the efficacy/effectiveness of mental health interventions, as different instruments are used for the assessment of different disorders and different aspects of disorders. Reference Ishak, Burt and Sederer1 Because of their specialisation none of these instruments can be considered a ‘gold standard’ in all contexts. Furthermore, the economic evaluation of new or existing services using a cost-utility framework generally requires a generic quality of life instrument capable of determining QALYs. There are a number of multi-attribute utility instruments (MAUIs) that measure health-related quality of life but, uniquely, have a ‘utility’ algorithm that converts people’s responses to a single utility score measured on a 0-1 scale, where 0 denotes death and 1 denotes the best health outcome measured by the instrument. The utility scores produced by these instruments in principle measure the strength of people’s preference for the health state. To obtain QALYs, the utility of a health state is multiplied by the length of time spent in the particular health state.
The most commonly used MAUI for evaluating both mental and physical disorders is the EuroQoL-five dimension (EQ-5D). Reference Brooks2 Its advantage is its brevity and simplicity. However, its sensitivity to the health states associated with mental disorders, particularly more severe disorders, has been questioned. Reference Brazier3 The main reason for this is the relatively crude measurement of psychosocial domains of quality of life (QoL) that are particularly affected by mental health problems. By contrast, the most recently developed MAUI instrument, the Assessment of Quality of Life - Eight Dimension Scale (AQoL-8D) was initially developed specifically to achieve sensitivity to the dimensions of QoL that are important to people with mental health problems. Reference Richardson, Khan and Iezzi4 This is the only MAUI that has been developed for this purpose. The construction of the AQoL-8D included people receiving specialised mental health services and particularly people with moderate to severe mental health problems, including psychotic disorders and severe depression.
Although a sensitive MAUI instrument would ideally be included in all mental health economic evaluations, this is not common practice, in part because of limitations in the number of instruments that can be simultaneously administered. A solution to this problem, which has been approved in the UK by the National Institute of Health and Care Excellence, is to estimate utility values indirectly by converting responses to disease-specific questionnaires into estimated health state utilities using a statistically derived conversion formula. Reference Brazier3 Within this context, the current study has two aims. The first is to compare the sensitivity of five MAUIs (the AQoL-8D, the EQ-5D-5L, the Short Form 6D (SF-6D), Reference Brazier, Roberts and Deverill5 the Health Utilities Index Mark 3 (HUI3) Reference Horsman, Furlong, Feeny and Torrance6 and the 15D Reference Sintonen7 ) with that of routinely used mental health outcome instruments, the Kessler Psychological Distress Scale (K10) Reference Kessler, Barker, Colpe, Epstein, Gfroerer and Hiripi8 and the Depression Anxiety Stress Scales (DASS-21) Reference Lovibond and Lovibond9 in people with self-reported depression. These instruments are described below. The second aim is to map the K10 and/or DASS-21 scores into utility values for each of the five MAUIs (a process called ‘cross-walk’ conversions) and to determine which instrument provides the best fit for the K10 and DASS-21.
Method
Data were obtained from the world’s largest multi-instrument comparison study. Reference Richardson, Iezzi and Maxwell10 This study used online methods to recruit people from six countries (Australia, UK, USA, Canada, Norway and Germany). An online panel company (CINT Pty Ltd) was engaged to send the link to the survey to people on their database until predetermined quotas of patients and demographically representative public respondents were achieved. Professional translations into German and Norwegian were used and validated by German and Norwegian project investigators. The study was approved by the Monash University Human Research Ethics Committee (Project numbers: CF11/1758-2011000974 and CF11/3192-2011001748). Figure 1 depicts the administration of the questionnaire.
Responses were subject to a set of stringent edit procedures based upon a comparison of duplicated or similar questions. Results were initially deleted when an individual’s (recorded) completion time fell below 20 min, which was judged to be the minimum time in which the 230 questions could be answered. The other edit procedures were largely concerned with inconsistent ratings between the questionnaires. For example, the EQ-5D mobility question was duplicated in the survey and anyone with a response that varied by more than ±1 difference was eliminated. Edit procedures, the questionnaire and its administration are described in detail in Richardson et al. Reference Richardson, Iezzi, Khan and Maxwell11
All respondents completed the five MAUIs (EQ-5D-5L, HUI3, SF-36, 15D and the AQoL-8D) along with the Quality of Wellbeing (QWB) scale, Reference Seiber, Groessl, David, Ganiats and Kaplan12 three subjective well-being scales and three ‘other’ instruments (including a self time-trade-off (TTO) exercise). The respondents were asked for current diagnoses and severity of any illnesses from the following list: arthritis, asthma, cancer, depression, diabetes, hearing loss and heart disease. There was also a ‘no disease’ category that was defined as ‘healthy public’. Patients were then asked to select their most serious illness and were assigned to this disease-specific stream. The disease-specific instruments included in the study were selected based on judgements of an expert panel who were asked to select commonly used outcome instruments for each disease category. The two instruments selected for completion by people with self-reported depression were the DASS-21 and the K10. These instruments are commonly used in the Australian context in particular, both in research projects and routine service delivery (the K10). The total sample for the multi-instrument comparison study was 8022 people, of whom 917 reported that they had a current diagnosis of depression.

Fig. 1 Administration of multi-instrument comparison online questionnaires. Reference Richardson, Iezzi and Maxwell10
Instrument description
AQoL-8D
The AQoL-8D was originally developed to achieve sensitivity not only in health states affected by physical disorders, but also those affected by mental disorders. The AQoL instruments were designed to assess the impact of ill health on a person’s life through the assessment of increased handicap arising from a disease. The AQoL-8D instrument contains 35 items in eight dimensions and was derived using psychometric methods for achieving content validity. Reference Richardson, Sinha, Iezzi and Khan13 Three of the dimensions (independent living, pain, senses) make up a physical super-dimension; the other five (mental health, happiness, coping, relationships and self-worth) a mental super-dimension. The size of the instrument means that it can define billions of health states. The valuation exercise for the AQoL-8D included 322 people with mental health disorders and 306 members of the general public and used the TTO valuation procedure. Reference Richardson, Sinha, Iezzi and Khan13
EQ-5D-5L
The five-item, five-level EQ-5D was used in the current study. Note that this version of the EQ-5D is sometimes referred to as the EQ-5D-5L but for simplicity we continue to refer to it as the EQ-5D. The five items of the EQ-5D include mobility, self-care, usual activities, pain/discomfort and anxiety/depression. The UK weights, which are the most widely used, employed the TTO preference techniques from a survey of 2997 members of the UK population. The original three response level instrument measured 243 health states but in 2009 the response levels were increased to five to increase reliability and sensitivity and potentially reduce ceiling effects. Reference Rabin, Oemar, Oppe and Janssen14 The revised instrument describes 3125 health states. The UK crosswalk weights for the five-level EQ-5D have been used in the current context. Reference Rabin, Oemar, Oppe and Janssen14
SF-6D
The SF-6D used in this study is the version derived from the SF-36. Reference Brazier, Roberts and Deverill5 The SF-36 was originally developed to be a general health status instrument and it covers eight dimensions that comprise two summary scores for physical and mental health. The SF-6D is composed of six multi-level dimensions (physical functioning, role limitation, social functioning, pain, mental health and vitality) and describes 18 000 health states. Reference Kharroubi, O'Hagan and Brazier15 The utility values were obtained by using the standard gamble to evaluate preferences of 249 health states with six observations from 611 UK participants. A recently developed non-parametric Bayesian approach has achieved greater predictive power for the utility algorithm and this algorithm was used in the current study. Reference Brazier, Ratcliffe, Salomon and Tsuchiya16
HUI3
The descriptive symptom of the HUI3 is a modification of the HUI2 and includes the domains of vision, hearing, speech, ambulation, dexterity, emotion, cognition and pain. The instrument defines 972 000 health states. Reference Horsman, Furlong, Feeny and Torrance6 Importantly, the HUI3 is largely a ‘within-the-skin’ based questionnaire as it contains very few psychosocial domains. The utility weights associated with the HUI3 were obtained by a representative sample of adult Canadians who used the visual analogue scale (VAS) in the valuation exercise. However a sample of health states were also valued using the standard gamble technique so that the VAS values could be transformed into standard gamble values.
15D
The 15D has 15 dimensions (questions) covering mobility, vision, hearing, breathing, sleeping, eating, speech, elimination, usual activities, mental function, discomfort and symptoms, depression, distress, vitality and sexual activity. Reference Sintonen7 As each dimension has either four or five levels, the 15D is able to define billions of health states. Reference Horsman, Furlong, Feeny and Torrance6 The valuation of the instrument was based on five random samples of the Finnish general population (500 people in each survey) using a variant of the VAS. Reference Horsman, Furlong, Feeny and Torrance6
DASS-21
The DASS-21 is a shorter version of the DASS-42. The instrument consists of a set of three self-reported scales designed to assess the severity of the core symptoms of depression, anxiety and stress. It is based on dimensional concepts of psychological morbidity. The depression subscale measures dysphoria, hopelessness, devaluation of life, self-deprecation, lack of interest, anhedonia and inertia. The anxiety scale measures physiologic effects, situational anxiety and subjective experience of anxiety. The stress subscale measures difficulty relaxing, nervous arousal, agitation/upset, irritability/overreactivity and impatience.
K10
The K10 is a short measure of non-specific psychological distress based on questions about nervousness, agitation, psychological fatigue and depression. It includes ten items with a five-level response scale. The scale was originally developed to discriminate between those who have a serious mental disorder and those who do not. The published severity cut-offs for both the DASS-21 Reference Lovibond and Lovibond9 and the K10 Reference Kessler, Barker, Colpe, Epstein, Gfroerer and Hiripi8 were used in the current study.
Analyses
The first aim of the paper was to assess the sensitivity of the MAUIs to depression severity, the psychometric approach for the assessment of construct validity was used. Reference Shelley and Cohen17,Reference Fitzpatrick, Davey, Buxton and Jones18 In the current context this means that higher levels of disease severity, as measured by the two established instruments (the K10 and the DASS-21), are expected to result in lower levels of utility as measured by the MAUIs. Mean scores for each of the MAUIs were calculated for each level of severity as measured by the disease-specific instruments and tested for differences using the non-parametric Kruskal-Wallis test since utilities were not normally distributed. Pearson correlation coefficients were also determined to enable the strength of associations between the instruments; a correlation greater than 0.5 was considered a strong association, between 0.3 and 0.49 a moderate association and less than 0.3 weak. Reference Byford19 Effect size calculations using Cohen’s d statistic were also determined and presented in online Table DS1.
The second aim of the study was to develop crosswalk transformation algorithms from the K10 and/or DASS-21 to the five MAUIs. To achieve this, a transfer to utility regression (TTU) technique Reference Mortimer, Segal and Sturm20,Reference Mortimer and Segal21 was used. In the TTU approach, a data-set containing responses to both instruments from the same individual is used to estimate the transformation algorithm that can then be applied for other studies. For example, to transform the DASS-21 subscale scores into AQoL-8D scores, the AQoL-8D utility score was regressed upon three DASS-21 subscales (depression, anxiety and stress), and also higher order (i.e. squared terms) of the DASS-21 subscales scores to test for non-linear effects. Three key models used in the transformation analysis are specified in the following equations:



where MAUI is AQoL-8D/EQ-5D/SF-6D/HUI3/15D utility, α is a constant, DASS-21D, DASS-21A and DASS-21S are the depression, anxiety and stress subscales of the DASS-21 instrument, K10 is the raw summary score of the K10 instrument, i indicates the order of score, β, γ, δ, and φ are the coefficients to be estimated, n = 2.
To incorporate potential country heterogeneity, the equations also included dummy variables for each country and interaction terms between country dummies and mental health scores. Similarly dummy variables were included for age (since age was recorded as a categorical variable, i.e. 18-24, 25-34,…, 65+) and gender. A stepwise regression technique with forward selection was used to choose the ‘best’ combination of predictors. Reference Rabe-Hesketh and Everitt22 The presence of comorbidities were not included in the equations as this would reduce the usability of the algorithms since most studies would be unlikely to collect information on comorbidities in the same way as this information was collected in the multi-instrument comparison study.
Two statistical methods - the ordinary least squares (OLS) and the generalised linear model (GLM) were used to estimate the models. The OLS has been found to be the most widely adopted in the transformation literature. Reference Mortimer and Segal21,Reference Brazier, Yang, Tsuchiya and Rowen23 The GLM allows for the non-normal distribution of dependent variables (i.e. for skewed utility scores). Reference Fox24 Among different combinations of family (for example, Gaussian, inverse Gaussian, binomial, gamma) and link (for example, identity, log, logit, cloglog, log-log, log-complement, power) functions for the GLM estimator, the Gaussian family with log link was chosen as the most appropriate based on the goodness-of-fit results (detailed results not reported but available from the authors on request). Several other econometric techniques have recently been adopted in the transformation literature, including the Tobit estimator, the censored least absolute deviations (CLAD) estimator, and the two-part model. One of their key benefits is the ability to take into account sample censoring of the dependent variable (i.e. a high proportion of respondents reporting a utility of 1). Reference Cameron and Trivedi25 However, they are not used in this analysis because sample censoring is not a major issue. A utility of 1 was found in: 0% of the AQoL-8D responses; 1.96% of the EQ-5D; 0.22% of the SF-6D; 1.09% of the HUI3; and 0.65% of the 15D. Except for the descriptive statistics and the figures, which were undertaken in IBM SPSS Statistics 19.0, all other statistical analyses were performed in Stata version 12.1.
Results
Sample description
Table 1 summarises the demographic characteristics of the sample used in the analysis. The majority of the 917 people who identified as having a depressive disorder in the multi-instrument comparison study were women aged between 25 and 54. Similar edit procedures in the six countries resulted in a similar number of results removed from the study and a similar number of respondents from each country. Only 6% of the sample was removed after the first edit procedure. There was no evidence of country-related differences in the quality of the responses. There were no missing data as the programme did not permit respondents to proceed until questions were completed.
Table 1 Sample characteristics (n = 917)
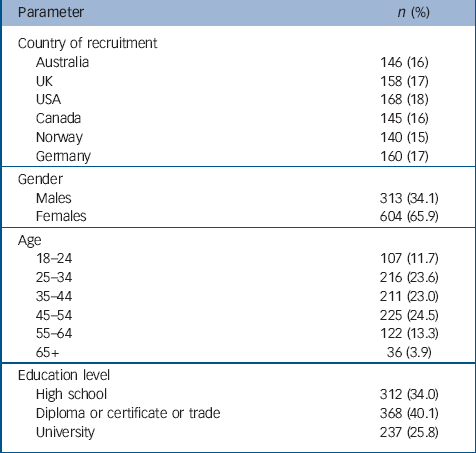
Parameter | n (%) |
---|---|
Country of recruitment | |
Australia | 146 (16) |
UK | 158 (17) |
USA | 168 (18) |
Canada | 145 (16) |
Norway | 140 (15) |
Germany | 160 (17) |
Gender | |
Males | 313 (34.1) |
Females | 604 (65.9) |
Age | |
18-24 | 107 (11.7) |
25-34 | 216 (23.6) |
35-44 | 211 (23.0) |
45-54 | 225 (24.5) |
55-64 | 122 (13.3) |
65+ | 36 (3.9) |
Education level | |
High school | 312 (34.0) |
Diploma or certificate or trade | 368 (40.1) |
University | 237 (25.8) |
As shown in Table 2, all the MAUIs discriminated between the levels of depression and psychological severity as measured by the DASS-21 and the K10. However, the range of scores varies between categories. For example the difference in utility from mild depression/anxiety to severe depression anxiety (measured on the K10) is 0.3 for the HU13, 0.2 for the AQoL-8D and the EQ-5D and 0.1 for the SF-6D and the 15D. The maximum utility on all questionnaires was 1.0 for both the depression sample and the ‘healthy public sample’ but the minimum ranged from –0.33 (HUI3) to 0.31 (15D) for the depression sample and –0.34 (HUI3) to 0.4 (SF-6D) for the healthy public. Online Table DS1 also reports effect sizes for differences between the adjacent severity levels on the DASS-21, K10 and each of the MAUIs. The magnitude of the effect sizes are as expected, with greater effect sizes observed for larger differences in severity.
Table 3 reports the correlation between the various instruments. All of the utility instruments display good to strong correlations with the K10 and the DASS-21. The results indicate that mapping from both the K10 and the DASS-21 to all MAUIs is appropriate.
Transformations
The goodness-of-fit results for different models are reported in online Table DS2. The predicted mean utilities in columns 1 to 3, are identical (from the OLS estimator) or very close (from the GLS estimator) to the observed (original) mean utility. However, the predicted utilities tend to over-/underpredict the lowest/highest value of the observed utilities for all of the instruments. This is not uncommon in transformation analysis (for example, Brazier et al Reference Brazier, Yang, Tsuchiya and Rowen23 ).
The pair-wise correlations between predicted and original utility range between 0.576 and 0.777 (column 4, online Table DS2). Consistently higher correlation coefficients can be observed in Panel A, which report the transformations from mental health scores to AQoL-8D utilities. The MAE, RMSE and R-squared reported in this study are within the range of previous published studies. Reference Brazier, Yang, Tsuchiya and Rowen23 The combined goodness-of-fit measures imply that the transformation of mental health measures into SF-6D, 15D and AQoL-8D utilities achieves greater accuracy than the transformations into EQ-5D and HUI3 utilities. Including both the K10 and DASS-21 scores improved the model goodness of fit.
The transformation equations corresponding to the goodness-of-fit result are summarised in Table 4. The country and interaction terms between country dummy and mental health scores are statistically significant implying differences in the statistical relationships in different countries. Whereas the interaction terms lead to better prediction within individual countries they create some ambiguity in the comparison between countries. For this reason alternative equations are provided in online Table DS3, which omits these terms. Gender was also found to be significant in two transformation algorithms (from mental health scores to SF-6D/HUI3). Observed v. predicted utilities for selected models are presented in Fig. 2, which indicates that the predicted range of scores is more dispersed for the EQ-5D and the HUI3 than for the AQoL-8D and the SF-6D.
Discussion
Main findings
The results of this study have demonstrated that the EQ-5D, SF-6D, HUI3, 15D and the AQoL-8D all discriminate well between the severity levels of the DASS-21 and the K10 and that transformations between the instruments result in relatively good prediction of utilities from the two depression instruments. The findings of the current study are consistent with results from other research where moderate correlations have been observed between disease-specific measures of depression and the EQ-5D and the SF-6D. Reference Peasgood, Brazier and Papaioannou26 This suggests that MAUIs have reasonably good construct validity and that they can adequately reflect depression severity.
Table 2 Multi-attribute utility instrument utilities according to severity levels on the Depression Anxiety Stress Scales (DASS-21) and the Kessler Psychological Distress Scale (K10)

n | AQoL-8D | EQ-5D | SF-6D | HUI3 | 15D | |
---|---|---|---|---|---|---|
Healthy public: mean (s.d.) | 1760 | 0.83 (0.14) | 0.88 (0.13) | 0.80 (0.11) | 0.88 (0.14) | 0.94 (0.06) |
range | 0.12 to 1.00 | –0.11 to 1.00 | 0.40 to 1.00 | –0.34 to 1.00 | 0.25 to 1.00 | |
Study sample: mean (s.d.) | 917 | 0.45 (0.18) | 0.59 (0.25) | 0.60 (0.11) | 0.53 (0.30) | 0.76 (0.13) |
range | 0.10 to 1.00 | –0.30 to 1.00 | 0.30 to 1.00 | –0.33 to 1.00 | 0.31 to 1.00 | |
DASS-21 stress categories, mean (s.d.)Footnote a | ||||||
Normal (score 0-14) | 337 | 0.56 (0.18) | 0.70 (0.18) | 0.66 (0.10) | 0.67 (0.24) | 0.83 (0.10) |
Mild (score 15-18) | 124 | 0.46 (0.16) | 0.62 (0.23) | 0.60 (0.09) | 0.55 (0.27) | 0.77 (0.12) |
Moderate (score 19-25) | 164 | 0.41 (0.15) | 0.59 (0.21) | 0.59 (0.08) | 0.52 (0.26) | 0.74 (0.11) |
Severe (score 26-33) | 192 | 0.35 (0.12) | 0.49 (0.26) | 0.56 (0.09) | 0.42 (0.29) | 0.70 (0.12) |
Extremely severe (score 34+) | 100 | 0.31 (0.14) | 0.35 (0.29) | 0.51 (0.10) | 0.29 (0.32) | 0.65 (0.14) |
DASS-21 depression categories, mean (s.d.)Footnote a | ||||||
Normal (score 0-9) | 159 | 0.66 (0.16) | 0.76 (0.13) | 0.70 (0.11) | 0.78 (0.19) | 0.86 (0.09) |
Mild (score 10-13) | 101 | 0.54 (0.16) | 0.67 (0.19) | 0.66 (0.09) | 0.64 (0.24) | 0.81 (0.09) |
Moderate (score 14-20) | 200 | 0.49 (0.14) | 0.63 (0.20) | 0.61 (0.08) | 0.63 (0.23) | 0.78 (0.11) |
Severe (score 21-27) | 150 | 0.41 (0.11) | 0.60 (0.23) | 0.59 (0.07) | 0.51 (0.26) | 0.76 (0.10) |
Extremely severe (score 28+) | 307 | 0.31 (0.12) | 0.43 (0.27) | 0.53 (0.09) | 0.32 (0.28) | 0.67 (0.13) |
DASS-21 anxiety categories, mean (s.d.)Footnote a | ||||||
Normal (score 0-7) | 311 | 0.57 (0.18) | 0.71 (0.17) | 0.66 (0.11) | 0.68 (0.23) | 0.84 (0.09) |
Mild (score 8-9) | 78 | 0.50 (0.16) | 0.65 (0.20) | 0.63 (0.08) | 0.62 (0.27) | 0.80 (0.11) |
Moderate (score 10-15) | 177 | 0.44 (0.15) | 0.61 (0.22) | 0.60 (0.08) | 0.54 (0.27) | 0.76 (0.11) |
Severe (score 16-19) | 99 | 0.39 (0.13) | 0.54 (0.23) | 0.57 (0.07) | 0.47 (0.26) | 0.72 (0.11) |
Extremely severe (score 20+) | 252 | 0.32 (0.14) | 0.41 (0.27) | 0.53 (0.10) | 0.34 (0.31) | 0.67 (0.13) |
K10 category, mean (s.d.)Footnote a | ||||||
Likely to be well (score 10-19) | 130 | 0.71 (0.14) | 0.78 (0.13) | 0.73 (0.10) | 0.80 (0.16) | 0.88 (0.08) |
Mild depression/anxiety (score 20-24) | 152 | 0.55 (0.13) | 0.70 (0.16) | 0.64 (0.08) | 0.69 (0.20) | 0.82 (0.09) |
Moderate depression/anxiety (score 25-29) | 172 | 0.45 (0.12) | 0.66 (0.18) | 0.61 (0.07) | 0.61 (0.23) | 0.79 (0.09) |
Severe depression/anxiety (score 30-50) | 463 | 0.35 (0.13) | 0.47 (0.27) | 0.55 (0.09) | 0.38 (0.29) | 0.69 (0.12) |
AQoL-8D, Assessment of Quality of Life - Eight Dimension Scale; EQ-5D-5L, EuroQoL-five dimension; SF-6D, Short Form 6D; HUI3, Health Utilities Index Mark 3.
a. Correlation is significant at the <0.001 level (Kruskal-Wallis test).
Table 3 Comparison of correlation coefficient for Assessment of Quality of Life - Eight Dimension Scale (AQoL-8D), EuroQoL-five dimension (EQ-5D); Short Form 6D (SF-6D), Health Utilities Index Mark 3 (HUI3), Depression Anxiety Stress Scales (DASS-21) and the Kessler Psychological Distress Scale (K10)

AQoL-8D | EQ-5D | SF-6D | HUI3 | 15D | |
---|---|---|---|---|---|
DASS-21 (Stress) | 0.526Footnote ** | 0.454Footnote ** | 0.467Footnote ** | 0.435Footnote ** | 0.487Footnote ** |
DASS-21 (Depression) | 0.697Footnote ** | 0.512Footnote ** | 0.583Footnote ** | 0.593Footnote ** | 0.547Footnote ** |
DASS-21 (Anxiety) | 0.550Footnote ** | 0.524Footnote ** | 0.518Footnote ** | 0.474Footnote ** | 0.563Footnote ** |
K10 | 0.734Footnote ** | 0.586Footnote ** | 0.672Footnote ** | 0.625Footnote ** | 0.618Footnote ** |
** P<0.01 level (2-tailed).
In terms of the goodness-of-fit statistics used to evaluate crosswalk transformations, the best results were generally achieved by the AQoL-8D, which also gave the highest correlation with the disease-specific instruments (except 15D on DASS-21 - anxiety). The result reflects the larger number of mental health related items and dimensions in the AQoL-8D than in the other instruments. The ‘opportunity cost’ of the larger questionnaire is the greater completion time for the AQoL-8D. For online respondents this averaged 5.5 min compared with less than 1 min for the EQ-5D. The relatively poorer fit by the more widely used EQ-5D and HUI3 is attributable in large part to the distribution of the instrument’s scores. Although the length of the AQoL-8D may be a deterrent to its use in economic evaluation, this must be balanced against the potential greater sensitivity of this instrument to mental health QoL dimensions.
The suitability of each MAUI questionnaire for a study will depend upon the context of the study, its sample size and the importance of a high completion rate. Current studies have successfully used AQoL-8D, achieving a high completion rate. Reference O'Neil, Berk, Itsiopoulos, Castle, Opie and Pizzinga27 Additionally, like the SF-6D, the AQoL-8D allows a missing item in six of the dimensions and two missing items in the two longer dimensions (with the response values interpolated). Nevertheless, in surveys in which questionnaire length is severely constrained or no missing data are permissible other MAUIs may be more suitable than the AQoL-8D.
Both the EQ-5D and HUI3 instruments have negative values with large absolute magnitudes that result in a significant difference between predicted and actual utilities. In addition, the distributions of both of these instruments are not continuous and display significant insensitivity near full health. With the EQ-5D the second highest health state has a utility of 0.88 (0.12 below full health). The study employed the EQ-5D-5L weights obtained from a crosswalk from the EQ-5D-3L. Subsequent studies that obtain weights directly for the five-level instruments may mitigate these results.
A recent review of crosswalk studies between MAUIs and other measures found that the explanatory power of studies ranged from an R 2 of 0.17 to 0.71, with the majority between 0.4 to 0.5. Reference Brazier, Yang, Tsuchiya and Rowen23 Crosswalk studies involving depression have, in particular, resulted in a poor goodness of fit. For example, although Byford Reference Byford19 did not report a crosswalk conversion this study compared the three-level EQ-5D to three commonly used outcome scales for adolescent depression: namely the Health of the National Outcome scale for Children and Adolescents (HoNOSCA); Reference Gowers, Harrington, Whitton, Lelliott, Beevor and Wing28 the Moods and Feelings Questionnaire (MFQ); Reference Angold, Costello and Messer29 and, the Children’s Depression Rating Scale (CDRS). Reference Poznanski, Grossman, Buchsbaum, Banegas, Freeman and Gibbons30 The largest correlation was with the MFQ (0.353) and the lowest was with the CGAS (0.161). By these standards the correlations and crosswalk between the two disease-specific measures and most of the MAUIs in this study perform well.
Table 4 Transformation equations from Depression Anxiety Stress Scales (DASS-21) and/or the Kessler Psychological Distress Scale (K10) to multi-attribute utility instrumentsFootnote a

Instruments | Transformation equations |
---|---|
Panel A - AQoL-8D | |
DASS-21 | AQoL-8D = 0.7503867–1.516705×DASS-21D–0.6564706×DASS-21A + 1.408039×DASS-21D2 + 0.9375113×DASS-21A2 |
K10 | AQoL-8D = exp(0.204665–3.617134×K10 + 0.0537131×NO) |
DASS-21 and K10 | AQoL-8D = 1.07836–0.500666×DASS-21D–2.612654×K10 + 2.607031×K102 |
Panel B - EQ-5D | |
DASS-21 | EQ-5D = 0.7848984–0.8450922×DASS-21A–1.496702×DASS-21D2 |
K10 | EQ-5D = 0.8644649–2.926161×K102–0.0387056×GE |
DASS-21 and K10 | EQ-5D = 0.8584133–0.4632134×DASS-21A–0.6400611×DASS-21D2–1.87376×K102 |
Panel C - SF-6D | |
DASS-21 | SF-6D = exp(–0.3207009–0.6598678×DASS-21D–0.5083976×DASS-21A + 0.7193344×DASS-21A2CA + 0.0230103×Male) |
K10 | SF-6D = exp(–0.0059462–2.165542×K10 + 1.319628×K102) |
DASS-21 and K10 | SF-6D = exp(–0.0384405–0.1992122×DASS-21D–0.170769×DASS-21A–1.84622×K10 + 1.357882×K102) |
Panel D - HUI3 | |
DASS-21 | HUI3 = 0.8556249–1.281214×DASS-21D–0.4450898×DASS-21A + 0.4352249×DASS-21SNO–1.257456×DASS-21A2US |
K10 | HUI3 = 1.034354–1.125104×K10–1.805111×K102 + 0.0862676×NO–0.0559438×Male |
DASS-21 and K10 | HUI3 = 0.9211197–0.7308938×DASS-21D + 0.46132×DASS-21SNO–1.165022×DASS-21A2US–2.461662×K102–0.0388832×Male |
Panel E - 15D | |
DASS-21 | 15D = exp(–0.0991446 - 0.4607568×DASS-21D - 0.5705034×DASS-21A - 0.1311059×DASS-21SCA–1.455366×DASS-21A2US) |
K10 | 15D = 1.028788–0.9143356×K10–0.2114242×K102CA |
DASS-21 and K10 | 15D = 0.9844646–0.1479519×DASS-21D–0.2595526×DASS-21A–0.9423584×DASS-21A2US–0.5235016×K10–0.2439458×K102CA |
DASS-21D, DASS-21 depression score; DASS-21A, DASS-21 anxiety score; DASS-21S, DASS-21 stress score; K10, K10 score; CA/GE/NO/US: country dummies for Canada/Germany/Norway/USA; Male: dummy for male; exp, exponential function; ×, interaction term.
a. The DASS-21 and K10 scores included in the regression model were calculated as original scores divided by 100.
Limitations
Despite the encouraging results presented in the current study there are some limitations. First, although the sample size was large, the survey required self-report of diseases. As such the presence of depression was not corroborated by clinical diagnostic criteria. However, since 83% of individuals in the sample were classified as mild to severely depressed on the depression subscale of the DASS-21 it is likely that these individuals did in fact have clinically relevant depression. Second, representativeness of the sample is imperfect. The current study included slightly more women as a proportion of the total sample compared with community-based prevalence surveys (such as the National Survey of Mental Health and Wellbeing in Australia). 31 The age profile of the study sample was similar to what would be expected from community-based prevalence surveys (for example Australian Bureau of Statistics 31 ) although there were fewer participants in the younger age-group (18-24) than what would be expected from community-based surveys. The survey was also a cross-sectional design so a comparison of the responsiveness of the instruments to change over time could not be assessed. However, generally speaking, the average utilities reported by respondents in the current study are within the vicinity found in the limited cost-utility studies using MAUIs reported in the literature. For example, the range of utility levels (measured using the EQ-5D) reported in a study investigating selective serotonin reuptake inhibitors for depression in primary care Reference Serrano-Blanco, Pinto-Meza, Suarez, Penarrubia and Haro32 are similar to those found in the current study (for example the baseline EQ-5D utilities were in the range of utilities reported for people with moderate to severe depression in the current study). It is still the case that very few studies investigating interventions targeting depression include MAUIs within the batteries of outcome measures, so it is difficult to comment on the results of the current study in relationship to other studies in depression that have used these measures.
Another important issue is the extent to which country-specific differences hinder the use of instruments that have been developed and valued only in one country. For example the AQoL-8D utilities reflect the preferences of an Australian sample and these may differ from preferences in other countries. However, the significance of this is unknown. Differences between MAUIs are dominated by differences in the descriptive systems, modelling techniques and choice of scaling instrument. The residual effect of differing national preferences has not been demonstrated. Previous research suggests that differences in country-specific scoring algorithms for the same MAUI may not be quantitatively large, although differences do exist. Reference Viney, Norman, King, Cronin, Street and Knox33 The problem of representative preferences is, however, more general. Even a perfectly representative cross-section of a national population may not capture preferences of a particular sociodemographic or disease subgroup in the same country. It would, nevertheless, be desirable for future research to test the validity of the AQoL-8D in other populations.
Finally, although this study presents a technique for deriving utility from surveys that may have not included an MAUI, it is important to appreciate that this is a second best solution to the inclusion of such an instrument in a study. Predicted utilities cannot create or estimate content that is not in the disease-specific instrument: they can only transform the content of these instruments to a second best estimate. Furthermore, although the current study has ensured internal validity of the mapping algorithms, external validation is still required, although the size of the sample and the international context of the study helps to mitigate any large threats to external validity.

Fig. 2 Scatter plots of observed and predicted utilities (from chosen models with both Depression Anxiety Stress Scales (DASS-21) and Kessler Psychological Distress Scale (K10) included as key predictors).
(a) Assessment of Quality of Life - Eight Dimension Scale (AQoL-8D); (b) EuroQoL-five dimension (EQ-5D); (c) Short Form 6D (SF-6D); (d) Health Utilities Index Mark 3 (HUI3); (e) 15D. OLS, ordinary least squares; GLM, generalised linear model.
Implications
All five MAUIs assessed in the current study appear to discriminate between severity levels on both the K10 and the DASS-21, and assigning utility values from the MAUIs to routinely collected outcome measures such as the K10 and the DASS-21 can be undertaken. This allows mental health service researchers to determine service cost-effectiveness using a cost-utility framework in services or interventions where utility measures are not collected.
Acknowledgements
Cathrine Mihalopoulos is currently funded by an NHMRC Early Career Fellowship (1035887).
eLetters
No eLetters have been published for this article.