Severe mental illness typically causes significant functional impairment and consequent poor physical health.1,Reference Reilly, Olier, Planner, Doran, Reeves and Ashcroft2 Excess mortality among people with severe mental illness is as much as 2–3 times higher than in the general population, with multiple interacting causes.Reference Hert, Correll, Bobes, Cetkovich-Bakmas, Cohen and Asai3 These include much higher rates of preventable chronic disease, such as diabetes and cardiovascular disease.Reference Hert, Correll, Bobes, Cetkovich-Bakmas, Cohen and Asai3–Reference Hjorthøj, Stürup, McGrath and Nordentoft5 Premature death from non-communicable disease is up to 60% more likely in people with severe mental illness.Reference Vigo, Thornicroft and Atun6 Life expectancy with severe mental illness is 10–20 years shorter in high-income countries and 30 years shorter in low-income countries.Reference Walker, McGee and Druss7–Reference Fekadu, Medhin, Kebede, Alem, Cleare and Prince9 Recent discussions have suggested that people with severe mental illness should be prioritised for COVID-19 vaccination, given their increased risk of severe infection and COVID-19-related morbidity and mortality.Reference Mazereel, Van Assche, Detraux and De Hert10
Schizophrenia, bipolar disorder and major depressive disorder are all major contributors to the global burden of disease.Reference James, Abate, Abate, Abay, Abbafati and Abbasi11 These three conditions are identified as severe mental illness in this review. All three conditions are associated with the pattern of excess mortality described above.Reference De Hert, Dekker, Wood, Kahl, Holt and Möller12–14 Quality of life is also severely diminished in individuals affected by each of these conditionsReference Evans, Banerjee, Leese and Huxley15 and each is associated with substantial functional impairment.Reference Harvey, Heaton, Carpenter, Green, Gold and Schoenbaum16–Reference Rosa, Reinares, Michalak, Bonnin, Sole and Franco19 From an economic perspective, each of these mental disorders also carries substantial lifetime costs, borne by both individuals and health systems.Reference Seabury, Axeen, Pauley, Tysinger, Schlosser and Hernandez20 Although major depressive disorder is not always classified as a severe mental illness,Reference Evans, Banerjee, Leese and Huxley15 we include it in this review to capture severe depression that leads to psychiatric hospital admission.
Clinical trials of new interventions for these conditions are generally short term, and therefore do not measure the full scale of lifetime patient outcomes. Long-term evidence is necessary to inform decisions of which interventions should be implemented within healthcare systems such as the National Health Service (NHS).21 Economic models that estimate lifetime health and cost outcomes for individual patients are vital to understanding the long-term value of new interventions for severe mental illness.Reference Knapp and Wong22 We therefore examine patient-level economic models for the three conditions described.
Challenges in economic modelling in mental health are well described,Reference Shearer and Byford23 in particular those due to the short time horizon of clinical trials in mental healthReference Ginnelly and Manca24 and the wide scope of potential economic effects of mental disorders – including productivity losses and greater lifetime use of healthcare resources for the individual directly affected, as well as spill-over effects on economic outcomes for a patient's family and their wider community.Reference Knapp and Wong22 In severe mental illness, a recent systematic review of economic models assessing antipsychotic medication for schizophrenia found 90% of models to have ‘very serious’ quality limitations based on National Institute for Health and Care Excellence (NICE) checklist appraisal.Reference Jin, Tappenden, Robinson, Achilla, Aceituno and Byford25,26 There is concern that poor-quality economic evidence may be similarly widespread in bipolar disorder and major depressive disorder.Reference Abdul Pari, Simon, Wolstenholme, Geddes and Goodwin27,Reference Kolovos, Bosmans, Riper, Chevreul, Coupé and van Tulder28 Poor-quality economic modelling may lead to inefficient allocation of healthcare resources, misestimating the health and cost effects of alternative interventions.Reference Tappenden and Chilcott29 With increasing financial commitment and focus on improving outcomes in mental health,30 clinical commissioners urgently need accurate evidence on cost-effective care in severe mental illness.
Informing national treatment guidelines
In the UK, NICE has separate guidelines for psychosis, encompassing schizophrenia and bipolar disorder,31 and for major depressive disorder.32 In psychosis, guidelines recommend the use of psychological therapies, such as cognitive–behavioural therapy (CBT) and family intervention, alongside pharmacological treatment.31 Further treatments are under development, such as the use of virtual reality therapy to help patients overcome anxious avoidance of everyday social situations.Reference Freeman, Yu, Kabir, Martin, Craven and Leal33 However, the long-term effectiveness and cost-effectiveness of new and existing treatments remain poorly understood, especially in the context of varying real-world adherence to treatment.Reference Nosé, Barbui and Tansella34,Reference David35
Studies available to inform NICE guidelines for psychosis are largely characterised by short follow-up periods (up to 6 months) and small samples (an average of 79 participants per study).Reference Gastaldon, Mosler, Toner, Tedeschi, Bird and Barbui36 NICE explicitly identifies this as a key limitation in their psychosis guidance – as data for several parameters, including relapse and treatment discontinuation probabilities, require extrapolation to a lifetime horizon to capture the long-term impact of treatment on patient outcomes and costs.31 Psychosis is a severe and often enduring mental health problem, with most patients experiencing multiple episodes or persistent symptoms.31,Reference Knapp, Chisholm, Leese, Amaddeo, Tansella and Schene37,Reference Lehman38 However, in the absence of robust long-term evidence, it is not possible to confirm whether any extrapolation of short-term data either over- or underestimates the cost-effectiveness of different psychosis treatments.31
Short follow-up in clinical studies similarly affects NICE guidelines for major depressive disorder. The bespoke economic model constructed to inform current guidelines for pharmacological interventions in depression had a time horizon of only 14 months, limited by short study follow-up.32 The NICE guidance explicitly noted the variable methodological quality of the economic evaluation studies that were available to inform its policy-making.32 As in psychosis, economic models of major depressive disorder must have capacity to estimate the impact of new interventions over the expected duration of the long-term disease course. Major depressive disorder can be a chronic condition with a high risk of recurrence over a patient's lifetime.Reference Melartin, Rytsala, Leskela, Lestela-Mielonen, Sokero and Isometsa39 In a large prospective observational study in The Netherlands, nearly 20% of patients had a single major depressive episode lasting longer than 24 months.Reference Spijker, De Graaf, Bijl, Beekman, Ormel and Nolen40 Economic modelling over a short time horizon does not give a fair reflection of a new intervention's value to the health system during each patient's lifetime.
The present review
The aim of this systematic review is to summarise health economic models of schizophrenia, bipolar disorder and major depressive disorder and their potential to extrapolate short-term studies informing the long-term value of interventions for severe mental illness. We undertook this review to inform the extrapolation of the gameChange trial,Reference Freeman, Yu, Kabir, Martin, Craven and Leal33 to provide recommendations for the broader research community to help identify patient-level models suitable for extrapolating short-term trials in severe mental illness, and to help improve the quality of future patient-level models in this area.
We focus on models designed to simulate individual patients (patient-level models) as they capture variation in presentation that leads to highly individualised patient experiences and outcomes in severe mental illness, which cannot readily be subgrouped.Reference Russo, Levine, Demjaha, Di Forti, Bonaccorso and Fearon41,Reference Harrow, Grossman, Jobe and Herbener42 Patient-level models are distinct from cohort models in explicitly calculating the expected costs and benefits for each individual patient, rather than estimating average outcomes across a patient group.Reference Davis, Stevenson, Tappenden and Wailoo43 Compared with cohort model approaches, patient-level model structures are better able to represent complex interactions between patient characteristics and an evolving disease historyReference Karnon, Stahl, Brennan, Caro, Mar and Möller44 and to capture non-linear relationships between individual risk factors and modelled outcomes.Reference Davis, Stevenson, Tappenden and Wailoo43 By more closely representing variation in disease course driven by individual patient histories and characteristics as seen in severe mental illness, patient-level models can generate more accurate estimates of health and cost outcomes in the overall population.Reference Brennan, Chick and Davies45
Method
The protocol for the literature review was registered in the PROSPERO international prospective register of systematic reviews (registration number CRD42020158243). Using OVID, we searched MEDLINE, Embase and PsycINFO for health economic models of psychosis, schizophrenia, bipolar disorder and major depressive disorder published between 1986 and 26 August 2020 (the date of extraction). Preferred Reporting Items for Systematic Reviews and Meta-Analyses (PRISMA 2009) guidelinesReference Moher, Liberati, Tetzlaff and Altman46 were followed and the checklist is reported in supplementary Appendix 1, available at https://doi.org/10.1192/bjp.2021.121. Search terms are reported in supplementary Appendix 2.
We used a two-stage approach to identify patient-level models in the review. First, we identified previous reviews of economic models for psychosis, schizophrenia, bipolar disorder and major depressive disorder. To achieve this, two reviewers screened titles and abstracts of identified records for reviews of economic models (both patient-level and non-patient-level models). Full-text records were requested for the reviews identified. Two reviewers extracted details of patient-level models reported in each review, alongside the databases searched and time periods covered by each review. Second, we updated the identified reviews by searching for all economic models published since the last date covered by the reviews.
The inclusion criteria used to identify relevant studies were as follows:
(a) studies with decision models of disease progression (models estimating risk factor progression) that reported health economic outcomes such as costs, (quality-adjusted) life expectancy and disease-related complications (such as psychotic or depressive episodes and treatment side-effects);
(b) studies with a model-based economic evaluation of intervention(s) in severe mental illness, such as cost–consequence, cost–utility and cost-effectiveness studies.
Searches were restricted to English language studies owing to challenges in locating and assessing non-English studies, given limited resources available to the research team, but no geographical restrictions were applied. Reference lists of identified economic models were also searched to identify any additional patient-level models missed by systematic searching. Abstracts and conference presentations reporting decision models were not included, as these did not provide sufficient information to allow critical appraisal of the models. For economic models identified across all conditions, patient-level economic models were extracted by reviewing titles and abstracts using keywords such as: ‘microsimulation’, ‘first-order Monte Carlo simulation’, ‘(Markov) patient-level’, ‘individual-level’ and ‘discrete-event simulation’.Reference Brennan, Chick and Davies45 References were managed using ENDNOTE X9.
There are several types of patient-level model.Reference Brennan, Chick and Davies45 A patient-level decision tree estimates each patient's expected health outcomes and costs without accounting for the timing of each modelled event (such as an in-patient stay or medication switch), other than the sequence in which each event occurs. However, most patient-level models do account for the timing as well as the sequence of modelled events. Patient-level Markov models simulate individual patients flowing between several health states, with transitions between states at fixed time cycles (such as each day, month or year). In discrete-event simulation models, the timing of each event is predicted precisely for each patient, so the timing of changes in each patient's health status is completely flexible, rather than occurring at fixed intervals. We include all types of patient-level model in our review.
A detailed extraction form was completed for each unique model to assess the suitability of current patient-level economic models to estimate long-term economic outcomes in severe mental illness, which is reported in supplementary Appendix 3. Clinical and health economic experts within the authorship group tailored the questions in the structured extraction to capture the key economic drivers within the disease course. If a decision model was found to be associated with multiple publications, data were extracted from the study that described the model in greatest detail, supported by other publications and relevant online documentation. Two reviewers each extracted all identified studies, with disagreements resolved by consensus.
The main outcomes analysed were:
(a) the objective of the model
(b) the model structure (modelling method, modelled states and links between states)
(c) the model's inputs and corresponding assumptions for costs and quality/length of life
(d) whether internal or external model validation/calibration was undertaken and documented.
A standardised checklist ranking a hierarchy of evidence quality was completed for each model, in which the data source used to inform a certain aspect of the model is awarded a score of 1 (highest quality) to 6 (lowest quality).Reference Cooper, Coyle, Abrams, Mugford and Sutton47 This provided a structured assessment of the quality of input data for key model parameters. Full ranking criteria for the grading of evidence sources are presented in supplementary Appendix 4.
Two reviewers completed quality checklists for each patient-level model identified. To assess model quality they used the International Society for Pharmacoeconomics and Outcomes Research (ISPOR) checklist, as published in a 2014 Good Practice Task Force Report by ISPOR, the Academy of Managed Care Pharmacy and the National Pharmaceutical Council (ISPOR-AMCP-NPC).Reference Caro, Eddy, Kan, Kaltz, Patel and Eldessouki48 This checklist aims to establish a model's credibility and relevance for decision-making, indicating any ‘fatal flaws’ that could render the model's results inaccurate or incomplete. To assess model validation processes and reporting they used the 2016 Assessment of the Validation Status of Health-Economic Decision Models (AdViSHE) checklist.Reference Vemer, Corro Ramos, van Voorn, Al and Feenstra49 The AdViSHE checklist supports structured reporting of model validation and aims to increase model transparency.
Findings from the review were synthesised narratively. This systematic review was exempt from ethics approval and consent of participants, as this study was based on previously published work.
Results
Literature search
In total, 2479 papers were identified from the three databases, of which 1481 were non-duplicates (Fig. 1); 39 review papers were identified, with the 3 most recent reviews in each condition covering patient-level models published up to December 2015.Reference Jin, Tappenden, Robinson, Achilla, Aceituno and Byford25,Reference Kolovos, Bosmans, Riper, Chevreul, Coupé and van Tulder28,Reference Mavranezouli and Lokkerbol50 Inclusion criteria for these reviews closely match this study and are detailed in full in supplementary Appendix 5. To update previous systematic reviews, 572 papers published between 1 January 2016 and 26 August 2020 were identified from the 1481 non-duplicate papers. An additional 5 patient-level economic models not covered by previous systematic reviews were identified from the 572 papers. Hence, we identified 15 unique patient-level economic models from a total of 28 studies.Reference Vera-Llonch, Delea, Richardson, Rupnow, Grogg and Oster51–65 Full detail of records assessed is provided in supplementary Appendix 6.
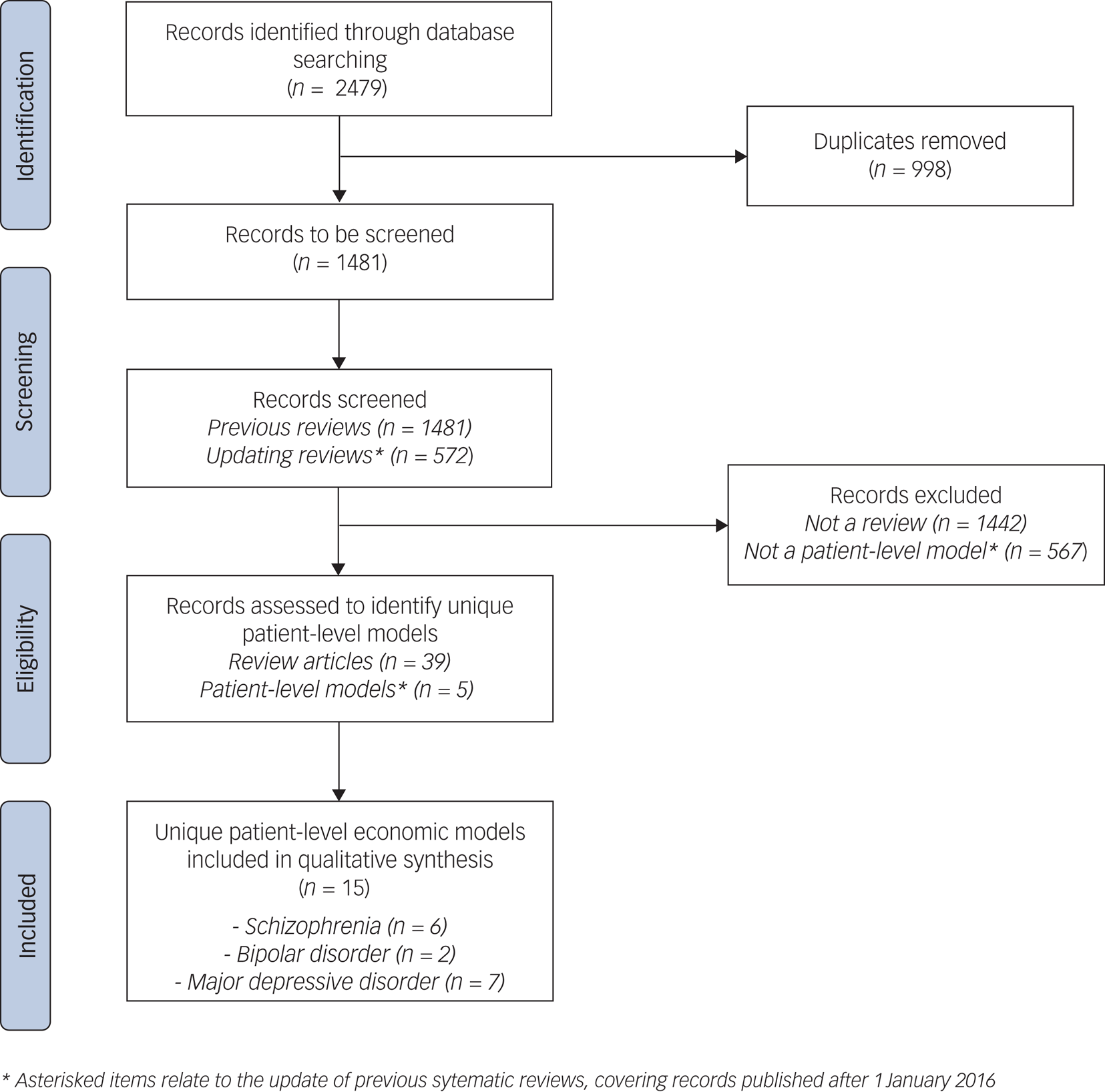
Fig. 1 Literature search – PRISMA diagram.
Description of health economic models
An overview of the 15 models is provided in Table 1. Seven patient-level models (47%) targeted major depressive disorder,Reference Sobocki, Ekman, Ågren, Jönsson and Rehnberg59–65 six (40%) targeted schizophreniaReference Vera-Llonch, Delea, Richardson, Rupnow, Grogg and Oster51–Reference Jin, Tappenden, MacCabe, Robinson and Byford56 and two models (13%) targeted bipolar I or bipolar II disorder.Reference Klok, Al Hadithy, van Schayk, Antonisse, Caro and Brouwers57,Reference Ekman, Lindgren, Miltenburger, Meier, Locklear and Chatterton58 Five models (33%) examined a UK setting,Reference Heeg, Buskens, Knapp, van Aalst, Dries and de Haan52,Reference Jin, Tappenden, MacCabe, Robinson and Byford56,Reference Ekman, Lindgren, Miltenburger, Meier, Locklear and Chatterton58,Reference Tosh, Kearns, Brennan, Parry, Ricketts and Saxon62,Reference Vataire, Aballéa, Antonanzas, Roijen, Lam and McCrone63 four models (27%) were set in North America,Reference Heeg, Buskens, Knapp, van Aalst, Dries and de Haan52,Reference Furiak, Ascher-Svanum, Klein, Smolen, Lawson and Conley53,55,65 three models (20%) had a European settingReference Dilla, Möller, O'Donohoe, Álvarez, Sacristán and Happich54,Reference Klok, Al Hadithy, van Schayk, Antonisse, Caro and Brouwers57,Reference Sobocki, Ekman, Ågren, Jönsson and Rehnberg59 and the remaining three models were set in Asia/Oceania.Reference Prukkanone, Vos, Bertram and Lim60,Reference Saylan, Treur, Postema, Dilbaz, Savas and Heeg61,Reference Nguyen and Gordon64
Table 1 Description of the 15 included studies
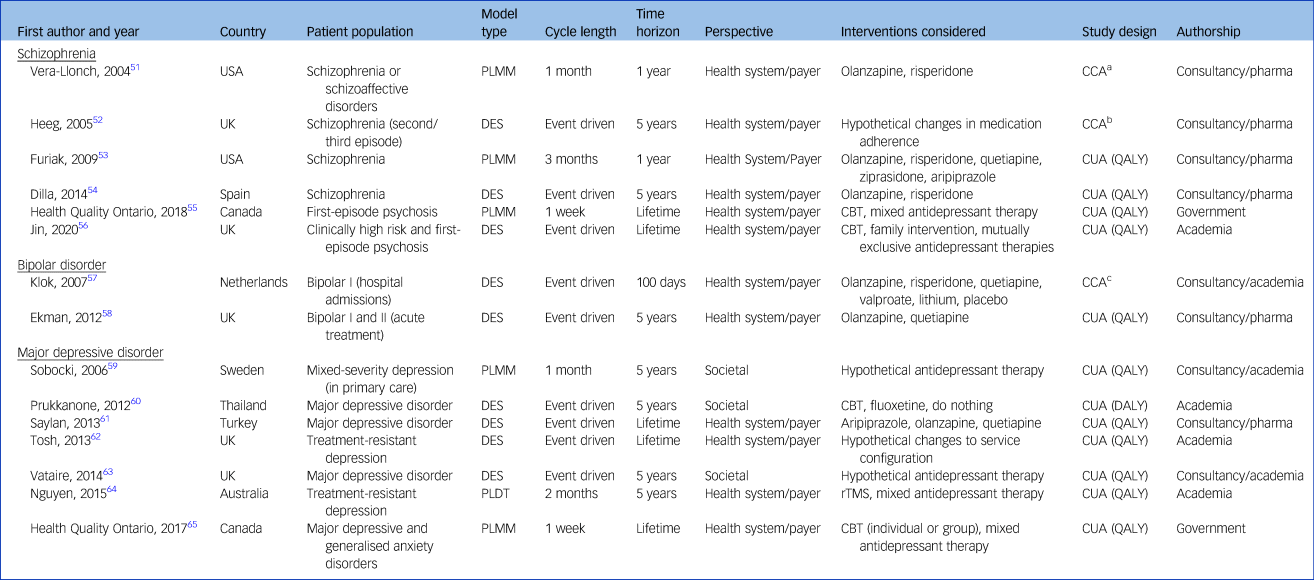
CBT, cognitive–behavioural therapy; CCA, cost–consequence analysis; CEA, Cost-effectiveness analysis; CUA, cost–utility analysis; DES, discrete-event simulation; PLDT, patient-level decision tree; PLMM, patient-level Markov model; QALY, quality-adjusted life-years; rTMS, repetitive transcranial magnetic stimulation.
a. Incidence of relapse of symptoms and side-effects, therapy discontinuation, expected costs of antipsychotic therapy, psychiatric and non-psychiatric services.
b. Cost per relapse avoided, total time in psychosis, total costs of psychosis.
c. Time to response/remission, side-effects, non-adherence, length of stay, total costs.
The majority of models (n = 11, 73%) evaluated pharmacological interventions,Reference Vera-Llonch, Delea, Richardson, Rupnow, Grogg and Oster51–Reference Dilla, Möller, O'Donohoe, Álvarez, Sacristán and Happich54,Reference Jin, Tappenden, MacCabe, Robinson and Byford56–Reference Saylan, Treur, Postema, Dilbaz, Savas and Heeg61,Reference Vataire, Aballéa, Antonanzas, Roijen, Lam and McCrone63 five models (33%) evaluated non-pharmacological individual interventions55,Reference Jin, Tappenden, MacCabe, Robinson and Byford56,Reference Prukkanone, Vos, Bertram and Lim60,Reference Nguyen and Gordon64,65 and one model (7%) examined the effect of system-level reorganisation.Reference Tosh, Kearns, Brennan, Parry, Ricketts and Saxon62 Models were generally structured as discrete-event simulations (n = 9, 60%)Reference Heeg, Buskens, Knapp, van Aalst, Dries and de Haan52,Reference Dilla, Möller, O'Donohoe, Álvarez, Sacristán and Happich54,Reference Jin, Tappenden, MacCabe, Robinson and Byford56–Reference Ekman, Lindgren, Miltenburger, Meier, Locklear and Chatterton58,Reference Prukkanone, Vos, Bertram and Lim60–Reference Vataire, Aballéa, Antonanzas, Roijen, Lam and McCrone63 or individual-level Markov models (n = 5, 33%),Reference Vera-Llonch, Delea, Richardson, Rupnow, Grogg and Oster51,Reference Furiak, Ascher-Svanum, Klein, Smolen, Lawson and Conley53,55,Reference Sobocki, Ekman, Ågren, Jönsson and Rehnberg59,65 although there was also an individual-level decision tree (n = 1, 7%).Reference Nguyen and Gordon64 The majority of models (n = 12, 80%) took a health system or payer perspectiveReference Vera-Llonch, Delea, Richardson, Rupnow, Grogg and Oster51–Reference Ekman, Lindgren, Miltenburger, Meier, Locklear and Chatterton58,Reference Saylan, Treur, Postema, Dilbaz, Savas and Heeg61,Reference Tosh, Kearns, Brennan, Parry, Ricketts and Saxon62,Reference Nguyen and Gordon64,65 and three models (20%) considered a societal perspective.Reference Sobocki, Ekman, Ågren, Jönsson and Rehnberg59,Reference Prukkanone, Vos, Bertram and Lim60,Reference Vataire, Aballéa, Antonanzas, Roijen, Lam and McCrone63
Five models (33%) considered a lifetime perspective for their analysis,55,Reference Jin, Tappenden, MacCabe, Robinson and Byford56,Reference Saylan, Treur, Postema, Dilbaz, Savas and Heeg61,Reference Tosh, Kearns, Brennan, Parry, Ricketts and Saxon62,65 with the most common time horizon considered being 5 years (n = 7, 47%).Reference Heeg, Buskens, Knapp, van Aalst, Dries and de Haan52,Reference Dilla, Möller, O'Donohoe, Álvarez, Sacristán and Happich54,Reference Ekman, Lindgren, Miltenburger, Meier, Locklear and Chatterton58–Reference Prukkanone, Vos, Bertram and Lim60,Reference Vataire, Aballéa, Antonanzas, Roijen, Lam and McCrone63,Reference Nguyen and Gordon64 Two models (13%) considered a 1-year time horizonReference Vera-Llonch, Delea, Richardson, Rupnow, Grogg and Oster51,Reference Furiak, Ascher-Svanum, Klein, Smolen, Lawson and Conley53 and one model (7%) used a time horizon of only 100 days.Reference Klok, Al Hadithy, van Schayk, Antonisse, Caro and Brouwers57
Twelve models (80%) informed cost–utility analysis (CUA) and estimated health outcomes in terms of quality-adjusted (QALY) or disability-adjusted life-years (DALY).Reference Furiak, Ascher-Svanum, Klein, Smolen, Lawson and Conley53–Reference Jin, Tappenden, MacCabe, Robinson and Byford56,Reference Ekman, Lindgren, Miltenburger, Meier, Locklear and Chatterton58–65 The remaining three models (20%) estimated health outcomes such as relapse,Reference Vera-Llonch, Delea, Richardson, Rupnow, Grogg and Oster51,Reference Heeg, Buskens, Knapp, van Aalst, Dries and de Haan52,Reference Klok, Al Hadithy, van Schayk, Antonisse, Caro and Brouwers57 side-effects,Reference Vera-Llonch, Delea, Richardson, Rupnow, Grogg and Oster51,Reference Klok, Al Hadithy, van Schayk, Antonisse, Caro and Brouwers57 treatment discontinuationReference Vera-Llonch, Delea, Richardson, Rupnow, Grogg and Oster51,Reference Klok, Al Hadithy, van Schayk, Antonisse, Caro and Brouwers57 and time in a psychotic state.Reference Heeg, Buskens, Knapp, van Aalst, Dries and de Haan52,Reference Klok, Al Hadithy, van Schayk, Antonisse, Caro and Brouwers57 These three models estimated the total disease costs and presented disaggregated results in the form of cost–consequence analyses.Reference Vera-Llonch, Delea, Richardson, Rupnow, Grogg and Oster51,Reference Heeg, Buskens, Knapp, van Aalst, Dries and de Haan52,Reference Klok, Al Hadithy, van Schayk, Antonisse, Caro and Brouwers57
Structural complexity
Table 2 summarises the features of the individual models. Simplified model structures for each study are presented in supplementary Appendix 7. All studies modelled an episodic/relapse state and a separate health state representing time not in episode. All models allowed participants to experience multiple relapses, except for the single model (7%) structured as a decision tree, which allowed only one relapse.Reference Nguyen and Gordon64
Table 2 Complexity of the 15 models
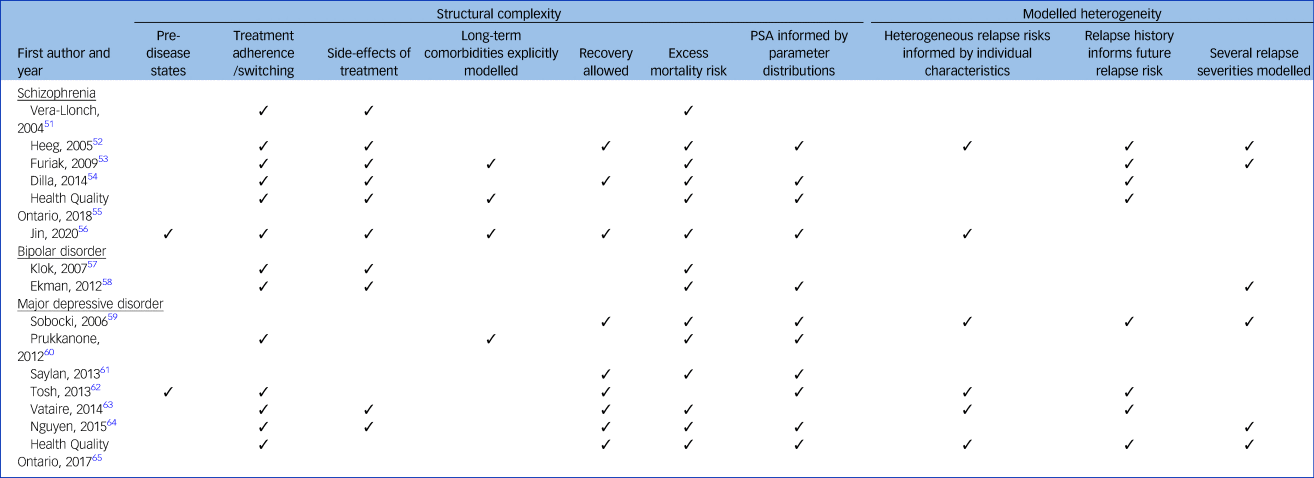
Two models (13%) explicitly modelled incident disease in otherwise healthy populations,Reference Jin, Tappenden, MacCabe, Robinson and Byford56,Reference Tosh, Kearns, Brennan, Parry, Ricketts and Saxon62 whereas the remaining models considered populations with established psychosis or major depressive disorder. All but one model (7%)Reference Tosh, Kearns, Brennan, Parry, Ricketts and Saxon62 incorporated an excess mortality risk to reflect the increased risk of death by suicide in populations with psychosis or major depressive disorder, compared with the general population. Eleven models (73%) incorporated parameter uncertainty in their modelled results, conducting a probabilistic sensitivity analysis informed by non-arbitrary parameter distributions.Reference Heeg, Buskens, Knapp, van Aalst, Dries and de Haan52,Reference Dilla, Möller, O'Donohoe, Álvarez, Sacristán and Happich54–Reference Jin, Tappenden, MacCabe, Robinson and Byford56,Reference Ekman, Lindgren, Miltenburger, Meier, Locklear and Chatterton58–Reference Tosh, Kearns, Brennan, Parry, Ricketts and Saxon62,Reference Nguyen and Gordon64,65
In six models (40%) relapse severity was modelled explicitly.Reference Heeg, Buskens, Knapp, van Aalst, Dries and de Haan52,Reference Furiak, Ascher-Svanum, Klein, Smolen, Lawson and Conley53,Reference Ekman, Lindgren, Miltenburger, Meier, Locklear and Chatterton58,Reference Sobocki, Ekman, Ågren, Jönsson and Rehnberg59,Reference Nguyen and Gordon64,65 Of these, three models (20%) categorised two types of severity: a more severe relapse requiring hospital admission and a less severe relapse with the patient remaining in the community.Reference Furiak, Ascher-Svanum, Klein, Smolen, Lawson and Conley53,Reference Nguyen and Gordon64,65 The remaining three models allowed a more continuous distribution of episode severity based on patient characteristicsReference Heeg, Buskens, Knapp, van Aalst, Dries and de Haan52,Reference Sobocki, Ekman, Ågren, Jönsson and Rehnberg59 or simply modelled relapse severity based on random chance.Reference Ekman, Lindgren, Miltenburger, Meier, Locklear and Chatterton58 The latter three models varied time to remission from relapse, so specific patients could experience a shorter or longer relapse than the cohort average. One studyReference Heeg, Buskens, Knapp, van Aalst, Dries and de Haan52 modelled time-updated disease severity scores for individual patients, which jointly determined the frequency, length and symptom severity of each episode.
Nine models (60%) distinguished between those experiencing temporary relief of symptoms (remission between episodes) and those experiencing longer-term relief of symptoms (recovery), with these states being characterised by different costs and/or health outcomes.Reference Heeg, Buskens, Knapp, van Aalst, Dries and de Haan52,Reference Dilla, Möller, O'Donohoe, Álvarez, Sacristán and Happich54,Reference Jin, Tappenden, MacCabe, Robinson and Byford56,Reference Sobocki, Ekman, Ågren, Jönsson and Rehnberg59,Reference Saylan, Treur, Postema, Dilbaz, Savas and Heeg61–65 In six models (40%), recovery was simulated by transition to a discrete ‘recovery state’.Reference Jin, Tappenden, MacCabe, Robinson and Byford56,Reference Sobocki, Ekman, Ågren, Jönsson and Rehnberg59,Reference Tosh, Kearns, Brennan, Parry, Ricketts and Saxon62–65 However, two models (13%) used a gradient of recovery (via a continuous variable or categorical variable with several levels) based on the time since last relapse and category of disease severity as determined by baseline patient characteristics.Reference Heeg, Buskens, Knapp, van Aalst, Dries and de Haan52,Reference Dilla, Möller, O'Donohoe, Álvarez, Sacristán and Happich54
Thirteen models (87%) explicitly simulated changes in treatment status by incorporating treatment switches and/or periods of non-adherence.Reference Vera-Llonch, Delea, Richardson, Rupnow, Grogg and Oster51–Reference Ekman, Lindgren, Miltenburger, Meier, Locklear and Chatterton58,Reference Prukkanone, Vos, Bertram and Lim60,Reference Tosh, Kearns, Brennan, Parry, Ricketts and Saxon62–65 Seven models (47%) considered both treatment switching and treatment discontinuation,Reference Vera-Llonch, Delea, Richardson, Rupnow, Grogg and Oster51–Reference Dilla, Möller, O'Donohoe, Álvarez, Sacristán and Happich54,Reference Jin, Tappenden, MacCabe, Robinson and Byford56–Reference Ekman, Lindgren, Miltenburger, Meier, Locklear and Chatterton58 for example low treatment adherence driving treatment switching, whereas six models (40%) incorporated only treatment switching.55,Reference Saylan, Treur, Postema, Dilbaz, Savas and Heeg61–65
Nine models (60%) predicted the impact of one or more side-effects resulting from antipsychotic treatments on patient health outcomes and costs.Reference Vera-Llonch, Delea, Richardson, Rupnow, Grogg and Oster51–Reference Ekman, Lindgren, Miltenburger, Meier, Locklear and Chatterton58,Reference Vataire, Aballéa, Antonanzas, Roijen, Lam and McCrone63,Reference Nguyen and Gordon64 However, the type of side-effects incorporated varied considerably between models. Eight models (53%) incorporated the impact of extrapyramidal symptomsReference Vera-Llonch, Delea, Richardson, Rupnow, Grogg and Oster51–Reference Ekman, Lindgren, Miltenburger, Meier, Locklear and Chatterton58 and seven models (47%) incorporated weight gain.Reference Vera-Llonch, Delea, Richardson, Rupnow, Grogg and Oster51–Reference Dilla, Möller, O'Donohoe, Álvarez, Sacristán and Happich54,Reference Jin, Tappenden, MacCabe, Robinson and Byford56–Reference Ekman, Lindgren, Miltenburger, Meier, Locklear and Chatterton58 Prolactin-related disorders,Reference Vera-Llonch, Delea, Richardson, Rupnow, Grogg and Oster51,Reference Heeg, Buskens, Knapp, van Aalst, Dries and de Haan52,55 neutropeniaReference Heeg, Buskens, Knapp, van Aalst, Dries and de Haan52,55,Reference Jin, Tappenden, MacCabe, Robinson and Byford56 and drowsinessReference Heeg, Buskens, Knapp, van Aalst, Dries and de Haan52,Reference Dilla, Möller, O'Donohoe, Álvarez, Sacristán and Happich54,Reference Vataire, Aballéa, Antonanzas, Roijen, Lam and McCrone63 were each considered in three models (20%), and tardive dyskinesiaReference Heeg, Buskens, Knapp, van Aalst, Dries and de Haan52,Reference Dilla, Möller, O'Donohoe, Álvarez, Sacristán and Happich54 and sexual dysfunctionReference Dilla, Möller, O'Donohoe, Álvarez, Sacristán and Happich54,Reference Vataire, Aballéa, Antonanzas, Roijen, Lam and McCrone63 were each considered in two models (13%). Four models (27%) considered a disparate range of other side-effects,Reference Furiak, Ascher-Svanum, Klein, Smolen, Lawson and Conley53,Reference Dilla, Möller, O'Donohoe, Álvarez, Sacristán and Happich54,Reference Vataire, Aballéa, Antonanzas, Roijen, Lam and McCrone63,Reference Nguyen and Gordon64 including insomnia, diarrhoea and post-injection syndrome.
Three models (20%) extended their modelling of medication-related side-effects,Reference Furiak, Ascher-Svanum, Klein, Smolen, Lawson and Conley53,55,Reference Jin, Tappenden, MacCabe, Robinson and Byford56 explicitly simulating a pathway from short-term transient side-effects into long-term comorbidities: diabetesReference Furiak, Ascher-Svanum, Klein, Smolen, Lawson and Conley53,55,Reference Jin, Tappenden, MacCabe, Robinson and Byford56 and cardiovascular disease.Reference Furiak, Ascher-Svanum, Klein, Smolen, Lawson and Conley53,55 The risk of developing long-term comorbidities was conditional on side-effects such as weight gain, via mediating pathways such as hyperlipidaemia and impaired glucose tolerance. In contrast, one model accounted for the long-term impact of comorbidities implicitly,Reference Prukkanone, Vos, Bertram and Lim60 with its authors applying a utility adjustment to the whole patient cohort, taking into account the incidence rate of medication-related comorbidities and their average health effect across all patients.
The medication-related side-effects incorporated by each model, and further detail of the precise modelling approach for long-term comorbidities, are presented in supplementary Appendix 8.
Incorporation of individual heterogeneity and patient history
Six patient-level models (40%) allowed the risk of a relapse to vary conditional on patient characteristicsReference Heeg, Buskens, Knapp, van Aalst, Dries and de Haan52,Reference Jin, Tappenden, MacCabe, Robinson and Byford56,Reference Sobocki, Ekman, Ågren, Jönsson and Rehnberg59,Reference Tosh, Kearns, Brennan, Parry, Ricketts and Saxon62,Reference Vataire, Aballéa, Antonanzas, Roijen, Lam and McCrone63,65 – mainly disease severity,Reference Heeg, Buskens, Knapp, van Aalst, Dries and de Haan52,Reference Jin, Tappenden, MacCabe, Robinson and Byford56,Reference Sobocki, Ekman, Ågren, Jönsson and Rehnberg59,Reference Tosh, Kearns, Brennan, Parry, Ricketts and Saxon62,Reference Vataire, Aballéa, Antonanzas, Roijen, Lam and McCrone63,65 ageReference Heeg, Buskens, Knapp, van Aalst, Dries and de Haan52,Reference Jin, Tappenden, MacCabe, Robinson and Byford56,Reference Sobocki, Ekman, Ågren, Jönsson and Rehnberg59,Reference Tosh, Kearns, Brennan, Parry, Ricketts and Saxon62,Reference Vataire, Aballéa, Antonanzas, Roijen, Lam and McCrone63 and gender.Reference Heeg, Buskens, Knapp, van Aalst, Dries and de Haan52,Reference Jin, Tappenden, MacCabe, Robinson and Byford56,Reference Sobocki, Ekman, Ågren, Jönsson and Rehnberg59,Reference Vataire, Aballéa, Antonanzas, Roijen, Lam and McCrone63 Full details of all patient characteristics considered in each model are provided in supplementary Appendix 8. The remaining nine models (60%) assumed the risk of first relapse to be equal across all individuals.Reference Vera-Llonch, Delea, Richardson, Rupnow, Grogg and Oster51,Reference Furiak, Ascher-Svanum, Klein, Smolen, Lawson and Conley53–55,Reference Klok, Al Hadithy, van Schayk, Antonisse, Caro and Brouwers57,Reference Ekman, Lindgren, Miltenburger, Meier, Locklear and Chatterton58,Reference Prukkanone, Vos, Bertram and Lim60,Reference Saylan, Treur, Postema, Dilbaz, Savas and Heeg61,Reference Nguyen and Gordon64 In eight models (53%), including five models that varied relapse risk on the basis of patient characteristics, the risk of subsequent relapse was conditional on the number of previous relapses modelled.Reference Heeg, Buskens, Knapp, van Aalst, Dries and de Haan52–55,Reference Sobocki, Ekman, Ågren, Jönsson and Rehnberg59,Reference Tosh, Kearns, Brennan, Parry, Ricketts and Saxon62,Reference Vataire, Aballéa, Antonanzas, Roijen, Lam and McCrone63,65 The risk of subsequent relapse varied in complexity. The simplest approach applied a single hazard ratio adjustment if a patient had any previous relapseReference Sobocki, Ekman, Ågren, Jönsson and Rehnberg59 and the most complex approach modelled future relapse risk as a continuous function driven by the duration of and time between previous relapses.Reference Heeg, Buskens, Knapp, van Aalst, Dries and de Haan52 In the remaining seven models (47%), the relapse risk was independent of the number of previous relapses modelled.Reference Vera-Llonch, Delea, Richardson, Rupnow, Grogg and Oster51,Reference Jin, Tappenden, MacCabe, Robinson and Byford56–Reference Ekman, Lindgren, Miltenburger, Meier, Locklear and Chatterton58,Reference Prukkanone, Vos, Bertram and Lim60,Reference Saylan, Treur, Postema, Dilbaz, Savas and Heeg61,Reference Nguyen and Gordon64
Hierarchy of evidence informing the models
The hierarchy of evidence used in the models is summarised in Fig. 2, ranging from high-quality evidence (ranked 1) to the lowest rank of evidence (rank 6). Full ranking criteria for evidence sources across all categories are presented in supplementary Appendix 4.
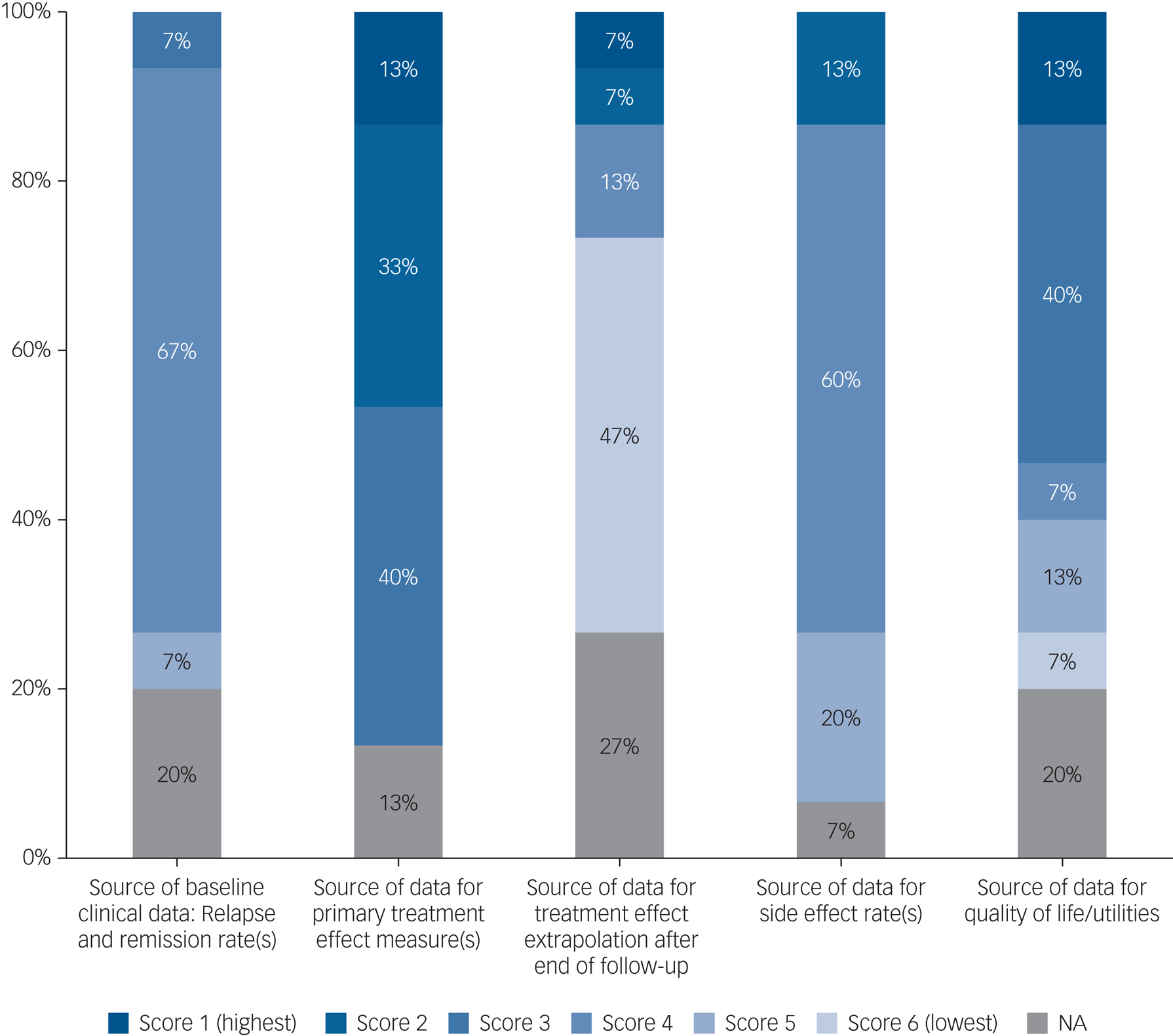
Fig. 2 Hierarchy of evidence quality. NA, not applicable.
Quality of evidence informing the models was mixed, and few models used high-quality evidence across all model elements. Evidence was particularly poor for treatment effect extrapolation, with seven models (47%) relying on expert opinion to inform treatment effect extrapolation beyond observed data to model efficacy over the whole simulated time.Reference Heeg, Buskens, Knapp, van Aalst, Dries and de Haan52–Reference Dilla, Möller, O'Donohoe, Álvarez, Sacristán and Happich54,Reference Jin, Tappenden, MacCabe, Robinson and Byford56,Reference Ekman, Lindgren, Miltenburger, Meier, Locklear and Chatterton58,Reference Prukkanone, Vos, Bertram and Lim60,Reference Nguyen and Gordon64 Often, there was no report of external expert consultation, so the opinion on the durability of the treatment effect over the time horizon was tacitly assumed by the model authors.Reference Dilla, Möller, O'Donohoe, Álvarez, Sacristán and Happich54,Reference Ekman, Lindgren, Miltenburger, Meier, Locklear and Chatterton58,Reference Prukkanone, Vos, Bertram and Lim60,Reference Nguyen and Gordon64 Two models (13%) did not attempt to extrapolate beyond their observed evidence sourcesReference Vera-Llonch, Delea, Richardson, Rupnow, Grogg and Oster51,Reference Klok, Al Hadithy, van Schayk, Antonisse, Caro and Brouwers57 and two models (13%) evaluated hypothetical treatments,Reference Tosh, Kearns, Brennan, Parry, Ricketts and Saxon62,Reference Vataire, Aballéa, Antonanzas, Roijen, Lam and McCrone63 meaning that this evidence category was not applicable to these four studies. Side-effect data were also often poorly evidenced, with studies often reporting several sources of input data with poor reporting of how each evidence source was used or how studies were combined to inform model parameters.
Model quality and validity
Models generally performed reasonably against ISPOR 2014 checklist criteria, which provide a broad overview of model quality and relevance (Fig. 3). Model design and analysis were generally adequate, with model credibility weakest in terms of data and reporting. Although the ISPOR checklist provides a high-level perspective over many diverse model attributes, only one checklist item directly scrutinises the model structure. Individual checklist items encompass multiple diverse topic areas. For example, short model time horizons are only assessed within a broad checklist item assessing the applicability of the model ‘context’. In terms of model credibility, the models performed poorly against ISPOR validation criteria, as corroborated by poor performance on the AdVISHE validation checklist (Fig. 3).
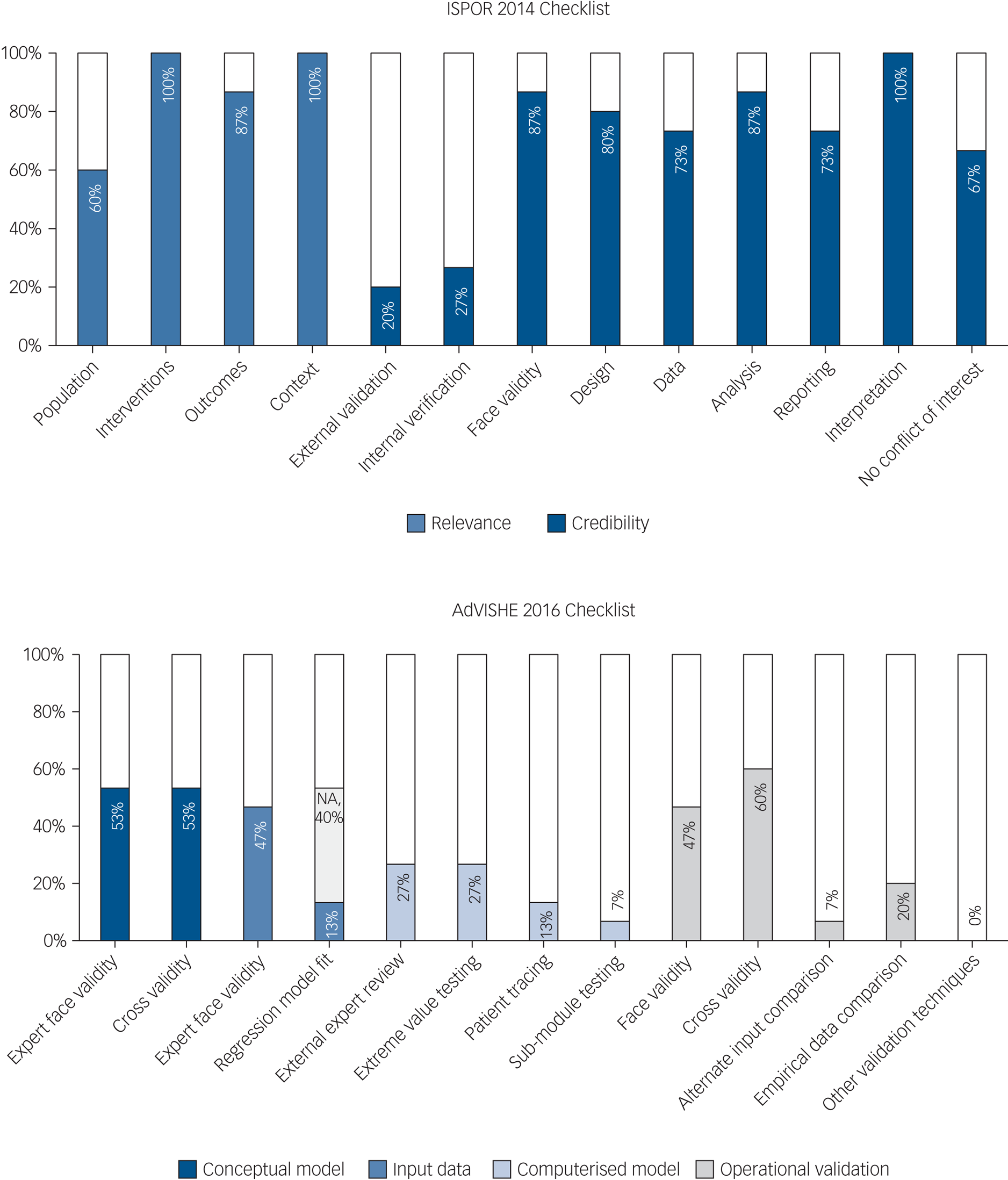
Fig. 3 Validation and quality checklists. ISPOR, International Society for Pharmacoeconomics and Outcomes; AdViSHE, Assessment of the Validation Status of Health-Economic Decision Models; NA, not applicable.
Of the 15 studies, 13 (87%) performed at least 1 of the 12 validation checks listed on the AdVISHE checklist.Reference Vera-Llonch, Delea, Richardson, Rupnow, Grogg and Oster51–Reference Jin, Tappenden, MacCabe, Robinson and Byford56,Reference Ekman, Lindgren, Miltenburger, Meier, Locklear and Chatterton58,Reference Sobocki, Ekman, Ågren, Jönsson and Rehnberg59,Reference Saylan, Treur, Postema, Dilbaz, Savas and Heeg61–65 However, model validation was generally restricted to face validity checks of model structure and the suitability of model inputs, and checks of model structure and inputs against published literature. Importantly, data and output validation was reported by only a handful of models. Two models (13%) reported validation of regression model fit,Reference Sobocki, Ekman, Ågren, Jönsson and Rehnberg59,Reference Nguyen and Gordon64 one model (7%) reported testing with alternative input dataReference Furiak, Ascher-Svanum, Klein, Smolen, Lawson and Conley53 and three models (20%) reported validation checks against empirical data.Reference Vera-Llonch, Delea, Richardson, Rupnow, Grogg and Oster51,Reference Jin, Tappenden, MacCabe, Robinson and Byford56,Reference Ekman, Lindgren, Miltenburger, Meier, Locklear and Chatterton58 Validation of the model as implemented in software (i.e. the computerised model) was particularly infrequent. Model authors rarely sought external expert model appraisal and rarely reported that basic model checks had been undertaken, such as extreme value testing or patient tracking through the computerised model. Jin et al (2020)Reference Jin, Tappenden, MacCabe, Robinson and Byford56 was an exception – providing full details of their validation procedures in supplementary material, documenting 10 of the 12 AdVISHE validation checks.
Full data for both quality checklists are presented in supplementary Appendix 9.
Discussion
Our review identified 15 unique patient-level economic models that simulated the natural history of schizophrenia, bipolar disorder and major depressive disorder. Our broad definition of severe mental illness allowed us to capture additional models that included severe depression leading to hospital admission. Antipsychotic medications can be used to treat all the conditions considered,Reference Wang and Si66,67 with common long-term comorbidities arising from specific medication-related side-effects. This is the first systematic review of economic models comparing patient-level model structures across schizophrenia, bipolar disorder and major depressive disorder, thus examining common economic modelling considerations across these patient groups.
We found considerable limitations in the quality and validity of current models. Outdated input data, lack of structural complexity and limited incorporation of patient heterogeneity are major concerns limiting models’ applicability to contemporary populations with severe mental illness. Only five models adopted the lifetime time horizon required by health technology reimbursement agencies.21 Current models therefore have limited potential to reliably extrapolate the results of short-term studies and thus inadequately inform decision makers’ assessment of the long-term value of existing and future interventions for severe mental illness.
Data quality
The data used to inform the models were generally of poor quality or were published more than 10 years ago. In psychosis, NICE treatment guidelines in England were last published in 201431 and more recent changes to the service pathway, including new waiting-time targets, have also substantially altered baseline care.68–Reference Reichert and Jacobs70 Outdated model data are a significant concern, as projections derived from models are unlikely to be relevant to current decision-making owing to shifts in best practice care over time. In several models, related model parameters such as baseline relapse/remission rates and treatment effectiveness were obtained from different patient populations, with little or no adjustment to account for varying patient characteristics between evidence sources. Several models were informed by population data from regions and countries outside the setting of the model's decision problem, raising concerns about data transferability. Furthermore, despite the majority of models estimating QALYs or DALYs, the data informing quality-of-life weights were generally of medium/poor quality.
Decision models should be based on high-quality and contemporary evidence to ensure that estimates of the scale and severity of disease burden and economic benefit of new interventions are sufficiently reliable. If good economic evidence is not available to support further investment in severe mental illness, healthcare decision makers will choose to prioritise the allocation of scarce healthcare resources in other disease areas where there is better evidence to support potential economic benefit from any additional investment. The poor quality and outdated data used in many of the identified decision models suggests that new large high-quality studies in severe mental illness are needed to construct economic models. New evidence is needed in almost all model aspects – both clinical and economic – with particular need to obtain contemporary quality-of-life data in severe mental illness using modern methods.
Model complexity
Short-term side-effects and long-term comorbidities associated with treatment
There was considerable variation in model complexity. Although most models allowed for treatment switching, there was substantial divergence in the simulation of treatment-related side-effects. Side-effects are a primary driver of antipsychotic treatment switching.Reference Roussidis, Kalkavoura, Dimelis, Theodorou, Ioannidou and Mellos71 In clinical practice, a systematic approach to medication selection may be complemented by an element of ‘trial and error’ of several medications, in response to variation in the occurrence and acceptability of specific side-effects in different patients.Reference Arango, Kapur and Kahn72,Reference Buckley and Miller73 Models therefore should incorporate all relevant side-effects resulting from multiple possible treatment choices. This is particularly important early in the disease course, when a patient's individual presentation is emerging and treatment switches are more frequent.Reference Derks, Fleischhacker, Boter, Peuskens and Kahn74 Given evidence of side-effects that are common to both first-generation ‘conventional’ and second-generation ‘atypical’ antipsychotic drug classes,Reference Leucht, Corves, Arbter, Engel, Li and Davis75 it is therefore advisable for models to consider a wide range of potential side-effects.
Most models failed to incorporate the link between short-term side-effects and long-term medication-related comorbidities. Diabetes and cardiovascular disease are common comorbidities in populations with severe mental illness, and both have distinct well-studied effects on length and quality of life, and related healthcare costs.Reference Ward and Druss76,Reference Correll, Solmi, Veronese, Bortolato, Rosson and Santonastaso77 Omitting comorbidities arising from medication side-effects may bias a comparative assessment of the long-term value of different treatments for severe mental illness. Bias is particularly likely when an intervention and comparator induce side-effects of differing magnitude. By modelling distinct pathways for patients who develop comorbidities, the health effects of medication-related comorbidities can be distinguished from health effects and costs arising directly from a mental health condition. This means that prevention and treatment strategies for comorbidities can be easily scrutinised and updated with changes to current practice, without affecting the calculation of health and cost outcomes in patients without medication-related comorbidities. However, only two models55,Reference Jin, Tappenden, MacCabe, Robinson and Byford56 identified in our review considered the impact of these long-term comorbidities over a patient's lifetime.
Patient heterogeneity
Few models incorporated all relevant aspects of patient heterogeneity and comprehensively estimated the impact of patient heterogeneity on health outcomes and costs. Only three models estimated individualised severity and frequency of relapse, based on each patient's baseline characteristics and modelled relapse history.Reference Heeg, Buskens, Knapp, van Aalst, Dries and de Haan52,Reference Sobocki, Ekman, Ågren, Jönsson and Rehnberg59,65 Failure to model interactions between patient characteristics and evolving disease history will produce inaccurate estimates of population-level health outcomes and costs.Reference Karnon, Stahl, Brennan, Caro, Mar and Möller44 For example, in psychosis a significant proportion of health service costs are driven by a subset of patients who have both a significant history of relapse and high degree of dependency on statutory social services.Reference Byford, Barber, Fiander, Marshall and Green78 By incorporating all relevant aspects of patient heterogeneity, including patient heterogeneity in excess mortality risk, models can more accurately estimate population-level outcomes. Quantification of expected outcomes for individual patients is also necessary to establish the overall value of stratified patient care.
Model validity
Beyond structural inadequacies, most models did not report rigorous validation checks to establish confidence in their results. Through the appraisal checklists, we found that authors were most likely to report cross-comparability or face validity of their model structure or results, based either on existing literature or on consultation with a clinical expert. However, few models documented more extensive validation efforts. Few models compared model predictions against empirical data, tested the robustness of model outputs using alternative input data or subjected the software underlying the model to thorough checking procedures or an external expert review. Only one model thoroughly documented most validation procedures from the 2016 AdViSHE checklist.Reference Jin, Tappenden, MacCabe, Robinson and Byford56
Nevertheless, decision makers need validated economic models to provide a foundation for credible long-term policy-making and treatment reimbursement decisions. Researchers also need evidence that economic models are sufficiently valid to extrapolate data from clinical trials. Validation efforts provide insight into model accuracy and establish the credibility of evidence generated to inform healthcare investment decisions.Reference Kent, Becker, Feenstra, Tran-Duy, Schlackow and Tew79 Economic models generate predictions regarding the stream of future costs and benefits from changes to healthcare policy. Without internal and external validation, decision makers have limited knowledge of whether an economic model produces sufficiently accurate predictions for their own setting. In this regard, additional external validation of economic models is essential to enable decision makers to be confident in models’ ability to discriminate between cost-effective and cost-ineffective health policies. Without validated models, healthcare decision makers may judge the uncertainty involved with a new investment in severe mental illness to be too great. The lack of high-quality and validated models may result in patients being denied good-value care, as depending on the model used, a range of long-term cost-effectiveness estimates could be obtained indicating different policy decisions.Reference Kim and Thompson80
Limitations
This review has some limitations. First, we included only studies published in English, and therefore models developed for non-Anglophone decision contexts, where decision makers may have different evaluation requirements are probably underrepresented. Second, we did not include cohort-level models that could be used to extrapolate short-term studies. However, given that cohort models cannot fully capture the effect of patient heterogeneity in severe mental illness, these models were of limited interest. Third, our assessment of model quality is non-context specific – we did not address model suitability for each particular decision problem being addressed, instead providing an overview of each model's performance against a general benchmark standard. Finally, our assessment of model quality is contingent on the level of detail provided in publications about the model – with publication detail potentially constrained by word-count limits.
Implications for future research and policy makers
The deficiencies of current models documented in this review across multiple dimensions can be used to inform the design of future models. In bipolar disorder and major depression, no structurally complex lifetime models with well-documented validation were identified. In schizophrenia, although Jin et al's modelReference Jin, Tappenden, MacCabe, Robinson and Byford56 was shown to be of a higher quality and more structurally complex than its peers with well-documented internal validity, additional research is needed to demonstrate its external validity and accuracy to extrapolate clinical trial data for informing healthcare decision-making.
Poor-quality economic models hinder policy makers’ ability to allocate healthcare budgets appropriately. In turn, this reduces the ability of clinicians to improve performance against NHS mental health targets while simultaneously providing cost-effective care. There is a clear need for further development of contemporary and comprehensive patient-level decision models that capture the full structural complexity of severe mental illness, in particular its relation to long-term comorbidities. High-quality contemporary evidence is needed on health-related quality of life and costs (collected in line with current best practice), as well as on long-term disease progression to inform the development of robust economic models. Following extensive internal and external validation exercises, new economic models could significantly reduce the time needed to make health policy decisions by reliably extrapolating short-term clinical trials to inform the cost-effectiveness of interventions for severe mental illness. Public research resources in severe mental illness should be coordinated to prioritise these objectives.
Supplementary material
Supplementary material is available online at https://doi.org/10.1192/bjp.2021.121.
Data availability
Data availability is not applicable to this article as no new data were created or analysed in this study.
Acknowledgements
We thank our information specialists Eli Bastin and Nia Roberts, University of Oxford, for their help in developing the search strategy and for selecting databases.
Author contributions
J.A. and J.L. designed the study. J.A. and J.-S.L conducted the literature search. J.A., J.-S.L and J.L conducted data extraction. J.A. and J.L. performed the analysis and A.T., F.W. and D.F. critically commented on the analysis results. J.A., A.T., F.W. and J.L. wrote the manuscript.
Funding
D.F. is a National Institute for Health Research (NIHR) senior investigator. F.W. is funded by a Wellcome Trust Clinical Doctoral Fellowship (102176/B/13/Z). This research was funded by the National Health Service (NHS) National Institute for Health Research (NIHR) invention for innovation (i4i) programme (Project II-C7-0117-20001). This work was also supported by NIHR Oxford Health Biomedical Research Centre funding (BRC-1215-20005). The views expressed are those of the authors and not necessarily those of the NHS, NIHR or Department of Health.
Declaration of interest
None.
eLetters
No eLetters have been published for this article.