Introduction
In the past decade, second language (L2) researchers have shown an increased interest in identifying methods suitable for investigating task effects on L2 performance (see Révész, Reference Révész, Ahmadian and Long2021a, for a review). This enhanced focus on techniques to examine task-based production has stemmed from several sources. On the theoretical front, there is a growing recognition among L2 researchers that, to test theoretical frameworks of task-based performance and learning (e.g., Skehan, Reference Skehan1998; Robinson, Reference Robinson and Robinson2001a), it is essential to provide validity evidence for all constructs invoked in them, including the independent and dependent variables and the causal processes posited to mediate links between them (Kane, Reference Kane and Brennan2006; Messick, Reference Messick1995; Norris & Ortega, Reference Norris, Ortega, Doughty and Long2003; Révész, Reference Révész2014). Tapping these various aspects of task-based models calls for a careful selection of research methods. The thorough and valid testing of task effects is also imperative from the perspective of pedagogy, as the resulting research outcomes are intended to credibly inform L2 teaching. For example, building our knowledge base about the impact of task variables on L2 performance may assist in discovering sources of task-related difficulty and in assessing the potential of manipulating tasks to generate learning opportunities assumed to foster L2 learning. Finally, in the spirit of the “methodological turn” in L2 research (Byrnes, Reference Byrnes2013, p. 825), the study of methodological issues merits attention in its own right to help increase rigor in our field, thereby raising the validity of our research.
Against this background, this study had two primary aims. First, being the first to adopt neuroimaging to study task effects on L2 spontaneous oral production, we intended to provide novel insights into the neural correlates of task-related variation in L2 oral production. Second, to advance L2 research methodology, our goal was to test the utility of a neuroimaging technique (fMRI) in examining the impact of task-related variables on L2 speech production when combined with cognitive–behavioral tools (speech analysis, expert and learner judgments). The focus of our research was task effects on silent pausing (pausing henceforth), a well-studied and common phenomenon of L2 speech. In particular, we investigated how pausing behaviors and associated neural processes might vary as a function of task complexity (i.e., the inherent cognitive demands of tasks), motivated by previous research on pausing and cognitive models of speech production and task-based performance.
Background
Models of speech production and task-based performance
Psycholinguistic models of speech production (Kormos, Reference Kormos2006, Levelt, Reference Levelt, Brown and Hagoort1999) typically see speaking as involving several different but incremental stages. The first stage, conceptualization, entails generating a preverbal plan through macro- and microplanning. During macroplanning, the speaker decides on the information to be presented and the order in which it will be expressed. As part of microplanning, they further elaborate the preverbal plan by specifying the informational perspective, including the focus, the argument structure and semantic relations, and the mood of the message. While macroplanning is assumed to be language general, microplanning is presumed to entail language-specific information, as the conceptual features to be encoded are dependent on language (e.g., tense) (DeBot, Reference De Bot1992; Kormos, Reference Kormos2006). The next stage, formulation, begins with lexical encoding, which involves pairing the conceptual specifications with the appropriate lemmas from the mental lexicon. There is an ongoing debate regarding lexical selection, depending on the theoretical assumptions of speech production. Some modular models presume that language cues are assigned to each concept of the preverbal message so that the subsequent lemma selection process is achieved with all the necessary conceptual information for identifying matching lexical entries (Kormos, Reference Kormos2006; Pouliesse & Bogaert, Reference Poulisse and Bongaerts1994). Others, however, also include an intermediary stage, the verbalizer, between conceptualization and formulation to assist with mapping conceptual features and lemmas, assuming that the preverbal message is not language-specific and the intended language for lexical entries is specified as a result of activation in the mental lexicon by the conceptual features (De Bot & Schreuder, Reference De Bot, Schreuder, Schreuder and Weltens1993). Once the appropriate lemmas have been identified, their grammatical properties are retrieved for morphosyntactic encoding, that is, building the surface structure of the message drawing on syntactic and morphological rules. Formulation ends with encoding the message into a plan of articulatory movements corresponding to the phonological representation of the message. In the final stage, articulation, the speaker executes the planned articulatory gestures to produce the overt speech. Throughout all three stages, the speaker engages in self-monitoring, checking whether the output of each stage (e.g., preverbal message, overt speech) matches their communicative intention. While the macroplanning stage of conceptualization is expected to pose similar demands on speakers with varied proficiency, lower-proficiency speakers will likely struggle more with microplanning and formulation, given their more limited L2 knowledge and partially automated processing skills (Suzuki & Kormos, Reference Suzuki and Kormos2023).
Partly drawing on models of speech production, two cognitive–interactionist frameworks, Robinson’s (Reference Robinson and Robinson2001a, Reference Robinson2011) Cognition Hypothesis and Skehan’s (Reference Skehan1998, Reference Skehan2009) Limited Capacity Model, have been proposed to theorize task effects on L2 oral performance and learning. The primary independent variable in each model is cognitive task demands, which is referred to as cognitive complexity by Skehan (Reference Skehan1998) and as task complexity by Robinson (Reference Robinson and Robinson2001a). Skehan (Reference Skehan2009) postulates that manipulating task-related features may create differential pressures on conceptualization and/or formulation processes during speech production, and the quality of the resulting linguistic performance depends on the extent to which the conceptualizer and/or formulator can deal with the cognitive demands of the task against attentional or working memory constraints. Inspired by a multiple-resources account of attention besides models of speech production, Robinson (Reference Robinson2011) posits that increasing the cognitive complexity of tasks will not only affect speech production processes predictably but also interactional patterns and the processing and retention of task-relevant input. In both frameworks, the principal dependent variable is the linguistic outcome of task-based performance described in terms of linguistic complexity, accuracy, and fluency. For fluency, both models hypothesize that when the cognitive demands of a task increase, the fluency of oral performance will decrease because L2 learners are likely to engage with controlled processing. Thus, the specific prediction for pausing, a breakdown feature of fluency (Skehan, Reference Skehan2003; Tavakoli & Skehan, Reference Tavakoli, Skehan and Ellis2005), is that more cognitively demanding tasks will lead to longer and more frequent pausing.
Based on previous empirical work on pausing, Skehan and Robinson’s prediction might be further refined by taking pause location into account. Researchers (de Jong, Reference De Jong2016; Field, Reference Field and Taylor2011; Lambert et al., Reference Lambert, Kormos and Minn2017; Suzuki & Kormos, Reference Suzuki and Kormos2023; Tavakoli et al., Reference Tavakoli, Nakatsuhara and Hunter2017, Reference Tavakoli, Nakatsuhara and A-M2020) have proposed that, depending on the location at which pauses occur, they are more or less likely to reflect certain speech production processes. In particular, there seems to be a greater likelihood that mid-clause pausing is associated with formulation processes, such as lexical encoding, syntactic, and phonological encoding, whereas end-clause pausing relates to conceptualization. Thus, following Robinson (Reference Robinson and Robinson2001a) and Skehan (Reference Skehan2009), it might be hypothesized that task manipulations that increase pressure on the conceptualizer will lead to more frequent and longer end-clause pauses. On the other hand, task demands exerting enhanced strain on the formulator will result in greater incidence and length of mid-clause pausing.
To date, only two empirical studies, Wang (Reference Wang and Skehan2014) and Lambert et al. (Reference Lambert, Kormos and Minn2017) provide information about the validity of these predictions about the relationship between task manipulations and pausing behavior, both investigating task repetition effects focusing on pause length and frequency respectively. In Wang’s (Reference Wang and Skehan2014) research, participants repeated an oral task twice, whereas Lambert et al. (Reference Lambert, Kormos and Minn2017) involved L2 learners in repeating a series of tasks six times. Both studies found that end-clause pausing decreased from the first to the second task performance, but Lambert et al. (Reference Lambert, Kormos and Minn2017) discovered no change in the incidence of end-clause pauses for further repetitions. For mid-clause pausing, neither Wang’s (Reference Wang and Skehan2014) nor Lambert et al.’s (Reference Lambert, Kormos and Minn2017) research yielded an effect for repeating a task once. Lambert et al. (Reference Lambert, Kormos and Minn2017), however, observed a reduced rate of mid-clause pauses when comparing participants’ first and third task performance. Drawing on models of speech production (Kormos, Reference Kormos2006; Levelt, Reference Levelt1989), Lambert et al. (Reference Lambert, Kormos and Minn2017) interpreted these findings as suggesting that the first repetition helped ease pressure on conceptualization processes, whereas the second task repetition assisted learners in carrying out more efficient linguistic encoding. While these conclusions seem logical, neither of these studies has provided direct evidence for the speech production processes assumed to explain the effects of task repetition on pausing patterns.
Validity considerations in assessing links between task factors and L2 performance
In general, previous research investigating the impact of task-related variables on L2 performance has dedicated relatively little attention to the causal processes that mediate the relationship between task manipulations and linguistic measures of task performance. However, as Norris and Ortega (Reference Norris, Ortega, Doughty and Long2003) highlighted and other models of validation (Kane, Reference Kane and Brennan2006; Messick, Reference Messick1995) also imply, if researchers would like to reach solid and valid conclusions about theoretical predictions regarding task effects on L2 performance, it is crucial to obtain validity evidence for measurement of every construct involved in the predictions, including the task variable in focus (independent variable), the indices of task performance used to assess the impact of the task variable (dependent variable), and the causal processes hypothesized to mediate links between the task variable studied and the performance measures (mediator).
Recently, L2 researchers have dedicated a lot of effort to aligning task-based research with this methodological recommendation. Most research attention has been allocated to identifying valid ways of selecting measures of linguistic complexity, accuracy, and fluency to assess task-based predictions (e.g., Bulté & Housen, Reference Bulté, Housen, Housen, Kuiken and Vedder2012; Housen & Kuiken, Reference Housen and Kuiken2009; Housen, Kuiken & Vedder, Reference Housen, Kuiken and Vedder2012; Norris & Ortega, Reference Norris and Ortega2009). Of particular relevance to the current research is previous validation work on measures of fluency, especially research focusing on the previously mentioned distinction between mid- and end-clause pausing. To date, most validation studies of fluency have drawn on Segalowitz’s (Reference Segalowitz2010) fluency framework, which describes fluency as including three different but interrelated subconstructs: cognitive fluency has to do with how efficiently the cognitive mechanisms underlying speech performance operate; utterance fluency refers to the observable aspects of oral performance including pausing, speed, and hesitation; and perceived fluency captures the listener’s judgments about the speaker’s cognitive fluency.
Conceptualized in terms of this framework, previous research has yielded at least three types of validity evidence that support the value of distinguishing between mid- and end-clause pausing. First, empirical studies of utterance fluency found that L2 speakers, as compared to first language (L1) speakers (de Jong, Reference De Jong2016; Duran-Karaoz & Tavakoli, Reference Duran-Karaoz and Tavakoli2020; Felker et al., Reference Felker, Klockmann and De Jong2019; Kahng, Reference Khang2014; Riazantseva, Reference Riazantseva2001; Skehan & Foster, Reference Skehan, Foster, Van Daele, Housen, Kuiken, Pierrard and Vedder2007; Tavakoli, Reference Tavakoli2011) and to more proficient L2 speakers (Duran-Karaoz & Tavakoli, Reference Duran-Karaoz and Tavakoli2020; Tavakoli et a., 2017, 2020), pause more often in the middle of clauses, but show similar patterns in terms of end-clause pausing. Second, researchers exploring the contribution of L2 cognitive fluency to L2 utterance fluency concluded that the frequency of mid-clause pausing is the strongest representative of the construct of L2 breakdown fluency (Kahng, Reference Kahng2020; Suzuki & Kormos, Reference Suzuki and Kormos2023). Finally, extant research on links between L2 perceived and utterance fluency has revealed a key role for mid-clause (but not end-clause) pausing in capturing L2 fluency (Kahng, Reference Khang2018; Saito et al., Reference Saito, Ilkan, Magne, Tran and Suzuki2018; Suzuki & Kormos, Reference Suzuki and Kormos2020; Suzuki et al., Reference Suzuki, Kormos and Uchihara2021). Overall, these results are in line with the theoretical assumption that mid-clause pausing mirrors the efficiency of L2 formulation and end-clause pauses reflect the operations of the conceptualizer (de Jong, Reference De Jong2016; Field, Reference Field and Taylor2011; Lambert et al., Reference Lambert, Kormos and Minn2017; Suzuki & Kormos, Reference Suzuki and Kormos2023; Tavakoli et al., Reference Tavakoli, Nakatsuhara and Hunter2017, Reference Tavakoli, Nakatsuhara and A-M2020). In line with these empirical observations, L2 speakers are expected to experience more difficulty with formulation due to their developing proficiency, whereas conceptualization processes are predicted to be less influenced by L2 skills. Taken together, past work on fluency suggests that adopting mid- and end-clause pausing patterns as dependent variables may enable gaining valid insights about the potential effects of task complexity on L2 performance.
Besides finding valid methods to gauge linguistic performance, a growing amount of task-based research has been concerned with the issue of supplying valid evidence for the task manipulation(s) under scrutiny (Norris, Reference Norris2010; Norris & Ortega, Reference Norris, Ortega, Doughty and Long2003; Révész, Reference Révész2014). For example, studies of task complexity more and more frequently employ independent measures of mental effort or cognitive load to gauge the validity of the task manipulation they investigate. Some researchers have used subjective methods to assess the amount of mental effort learners exerted during task performance, eliciting learner self-reports (e.g., Robinson, Reference Robinson2001b) or expert judgments of task difficulty (e.g., Révész et al., Reference Révész, Sachs and Hama2014; Révész et al., Reference Révész, Michel and Gilabert2016). A small number of studies (e.g., Lee, Reference Lee2019; Révész et al., Reference Révész, Michel and Gilabert2016; Sasayama, Reference Sasayama2016, Xu et al., Reference Xu, Zhang and Gaffney2022) have additionally used objective tools, which involved observing learners’ behaviors during task performance (e.g., dual-task methodology, eye-tracking). Notably, reflecting an increased concern with methodological issues in task-based research (e.g., Mackey, Reference Mackey2020; Norris, Reference Norris2010; Révész, Reference Révész2014, Reference Révész, Ahmadian and Long2021a, Reference Révész2021b), a few studies specifically defined their goal as to assess the usefulness of different techniques, alone or in combination, to capture the mental effort or cognitive load imposed by task demands. Like the current research, Révész et al. (Reference Révész, Michel and Gilabert2016) and Sasayama (Reference Sasayama2016) focused on oral production, triangulating data collected through the dual-task methodology and self-ratings of mental effort and task difficulty. Révész et al. (Reference Révész, Michel and Gilabert2016) additionally elicited expert judgments and Sasayama’s (Reference Sasayama2016) time estimations to assess task complexity effects on cognitive load during oral task performance. In both projects, dual-task methodology and the various subjective methods yielded converging results overall, indicating that the task versions designed to be more complex were indeed more cognitively demanding. Given the parallel results generated by the objective and subjective methods in these validation studies, it appears justified to use subjective tools to assess task difficulty, as they are easier to administer and are nonobtrusive.
To date, as compared with validation research on measures of task complexity and linguistic performance, relatively few studies have focused on methods to assess the causal processes assumed to mediate relationships between task complexity and linguistic output (Robinson, Reference Robinson and Robinson2001a; Skehan, Reference Skehan2009). Similar to related work on validating task complexity manipulations, the small amount of research available on task processes has assessed the utility of various subjective and objective methods (see Révész, Reference Révész2021b, for a review). Studies on oral production, in particular, have utilized questionnaires (Révész, Reference Révész2009; Sasayama & Norris, Reference Sasayama, Norris, Wen and Ahmadian2019), interviews (Ortega, Reference Ortega and Ellis2005; Pang & Skehan, Reference Pang, Skehan and Skehan2014), and stimulated recall protocols (Kim et al., Reference Kim, Payant and Pearson2015; Révész, Kourtali et al., Reference Révész, Kourtali and Mazgutova2017; Torres, Reference Torres2018) to obtain information about learners’ subjective experiences during task performance. To gain more objective insights, researchers have relied on dual-task methodology (Révész et al., Reference Révész, Michel and Gilabert2016; Sasayama, Reference Sasayama2016) and eye-tracking (Révész et al., Reference Révész, Sachs and Hama2014) to investigate speech production processes. More recently, neuroimaging has additionally been suggested as an objective tool that could be useful for investigating task-based processing (Révész, Reference Révész2021b). However, its utility for this purpose has not yet been evaluated. A principal aim of this study was to begin exploring the capacity of neuroimaging to provide insights into task-generated processes and to complement and extend existing insights that have been gained through behavioral methods.
Neuroimaging as a potential way to tap task-generated cognitive processes
The specific neuroimaging technique we intended to explore is functional magnetic resonance imaging (fMRI). Simply put, fMRI captures when there are increases in blood flow resulting from heightened brain activity. When a greater amount of blood is supplied to a certain area of the brain, this neural activity is detected by the fMRI scanner. In the past, researchers have predominantly employed fMRI to investigate language user’s neural activity during input processing in tightly controlled experiments. Few studies have used fMRI to investigate neural processes involved in naturalistic language use, with even fewer studies focusing on the cortical mechanisms called upon during spontaneous oral language production.
Among these studies, two previous L1 experiments are worth highlighting here, as their speech elicitation technique was similar to the one employed in our research. Based on the same dataset, Morales et al. (Reference Morales, Patel, Tamm, Pickering and Hoffman2022) and Wu et al. (Reference Wu, Morales, Patel, Pickering and Hoffman2022) set out to compare brain activation patterns during the processing and production of L1 naturalistic discourse, to identify the neural correlates of psycholinguistic characteristics of speech (e.g., coherence, lexical complexity, emotional content). In an fMRI machine, participants were asked to orally respond to common topics (e.g., describe how to make a coffee) and listen to speech samples on comparable themes. The researchers revealed that, during both production and comprehension, brain activation patterns were associated with several psycholinguistic properties of the language produced or listened to by the participants. For example, Morales et al. (Reference Morales, Patel, Tamm, Pickering and Hoffman2022) found that, in speaking and listening alike, certain areas involved in the theory of mind network (e.g., medial prefrontal cortex [mPFC], precuneus, anterior temporal, and lateral parietal cortex) showed greater activation when the discourse was less coherent. The researchers, however, also discovered modality-specific effects depending on the properties of semantic information. For instance, Wu et al. (Reference Wu, Morales, Patel, Pickering and Hoffman2022) observed that action-related content was processed in the sensory–motor area in both speech production and comprehension, but for emotional content, there was more increased activation in emotion-related areas (e.g., anterior cingulate cortex and insula) during speech production as compared with comprehension.
The neural correlates of L2 spontaneous speech production are even less explored than those involved in L1 speech. Among the few L2 studies (Jeong et al., Reference Jeong, Hashizume, Sugiura, Sassa, Yokoyama, Shiozaki and Kawashima2011; Jeong et al., Reference Jeong, Sugiura, Suzuki, Sassa, Hashizume and Kawashima2016), Jeong et al.’s (Reference Jeong, Sugiura, Suzuki, Sassa, Hashizume and Kawashima2016) study is closest in focus to this research. The purpose of this study was to identify brain activation patterns associated with communicative as compared with noncommunicative oral production. The experiment involved participants in watching short videos, in which an actor interacted with an object (e.g., played a guitar). In the communicative condition, participants were instructed to talk to the actor in the video, whereas, under the noncommunicative condition, their task was to describe the actor’s situation. The fMRI analyses compared brain activation patterns when participants produced L1 and L2 across the communicative and descriptive speech conditions, as a function of language status (L1 versus L2) and L2 oral proficiency. The researchers found that both L1 and L2 speech production enhanced activation in certain brain areas only during communicative activities. These included regions involved in the theory-of-mind system (e.g., mPFC, precuneus, posterior superior temporal sulcus [pSTS]), retrieval and integration of concepts (left angular gyrus [AG]), and semantic retrieval (e.g., left middle temporal gyrus [MTG]). Notably, the left posterior supramarginal gyrus (SMG), an area associated with the planning of speech acts, was activated only during L2 communicative production. As expected, the study also yielded L2 oral proficiency effects for brain areas related to lexical and semantic retrieval (e.g., left MTG) during L2 communicative production. In addition, during L2 production, as expected, greater activation was observed in areas associated with syntactic and phonological processing (e.g., left inferior frontal gyrus [IFG]) than during L1 speech production irrespective of condition.
In sum, previous research suggests that neuroimaging has the potential to yield insights into the type of processing in which speakers primarily engage during speech production. Specifically, it appears that certain brain areas can be linked to processes associated with conceptualization (e.g., theory-of-mind network) and other regions to linguistic encoding processes, even during short sentence production. If so, we would expect that task manipulations that pose increased demands on the conceptualizer and formulator will enhance brain activity in areas related to conceptualization and language, respectively. A principal aim of this study was to explore if, as predicted, fMRI scans are indeed sensitive to task complexity manipulations.
Research questions and hypotheses
We formed two research questions to investigate the impact of task complexity on L2 pausing behaviors and associated neural processes:
RQ1: To what extent does task complexity influence silent pause frequency and length (mid- versus end-clause) during L2 speech production?
RQ2: To what extent does task complexity influence neural processes during silent pauses (mid- versus end-clause) during L2 speech production?
Inspired by models of speech production (Kormos, Reference Kormos2006; Levelt, Reference Levelt, Brown and Hagoort1999) and task-based performance (Robinson, Reference Robinson and Robinson2001a; Skehan, Reference Skehan2009), we assumed that more complex tasks would put greater pressure on conceptualization processes, due to the increased conceptual demands they pose. In turn, we expected that the enhanced conceptual demands during more complex tasks would result in fewer attentional resources available for linguistic encoding processes, leading to increased pressure on the formulator. As mid-clause and end-clause pauses have been associated with greater engagement in formulation and conceptualization respectively (de Jong, Reference De Jong2016; Field, Reference Field and Taylor2011; Lambert et al., Reference Lambert, Kormos and Minn2017; Suzuki & Kormos, Reference Suzuki and Kormos2023; Tavakoli et al., Reference Tavakoli, Nakatsuhara and Hunter2017, Reference Tavakoli, Nakatsuhara and A-M2020), we hypothesized that these presumed task complexity effects would affect the behavioral and neural correlates of mid- and end-clause pausing as follows:
H1: More complex tasks will elicit longer and more mid-clause and end-clause pauses.
H2: During more complex tasks, there will be greater activation in conceptualization (i.e., theory-of-mind network) and language-related brain areas (e.g., left IFG) during pauses, with stronger effects for end-clause and mid-clause pauses respectively.
We also formed a methodology-related research question and hypothesis, exploring the extent to which our subjective measures of task complexity (expert judgments, speaker ratings) would yield converging results with those obtained through objective behavioral (pause length and frequency) and neuroimaging measures (brain activation):
RQ3: To what extent do subjective ratings of task complexity relate to the frequency and length of pausing and the neural correlates of pausing?
H3: Higher task complexity ratings will relate to greater pause length and frequency and greater activation in conceptualization- and language-related brain areas during pauses.
Methodology
Design
The data for this study comes from a larger dataset. The participants were 26 Japanese users of L2 English. All 26 participants carried out eight oral tasks altogether in an fMRI scanner. Each participant completed the low-complexity version of four of the tasks and the high-complexity version of the other four tasks, half of them in L2 English and the other half in L1 Japanese. Task complexity and language were counterbalanced across the participants. We could only include data for 24 L2 performances and 21 L1 performances in our analyses here; we had to exclude the rest of the data due to the poor quality of some speech recordings and excessive head movement during scanning. Immediately after carrying out a task, participants were asked to provide task difficulty and mental effort ratings on a 9-point Likert scale. Two experts also gave judgments about the anticipated difficulty and mental effort posed by the tasks. Prior to performing the experimental tasks, participants completed a background questionnaire and the listening part of the Oxford Placement Test (Allen, Reference Allan2004).
The main focus of the current study is the participants’ performance on the four tasks they carried out in their L2 and the self-ratings they provided for these (n = 96). In addition, as a subjective measure of task complexity, we also included participants’ difficulty and mental effort ratings for the four tasks they completed in their L1 (n = 84). Given the counterbalanced design, participants did not complete the same four tasks in L2 English and L1 Japanese. Nevertheless, each of the eight tasks is equally represented in the L2 and L1 datasets, with any potential task effects controlled for through counterbalancing.
Participants
All the participants were undergraduate students at a Japanese university. The mean age was 20.33 years for both the L2 and L1 performances (L2: SD = 1.43, L1: SD = 1.46) with a range of 19–24. Participants were nearly equally distributed in terms of gender (L2: 10 female, 14 male; L1: 11 female, 10 male). All L2 participants had been studying English as a first foreign language in formal school settings for an average of 9.66 years (SD = 2.82). Out of the 24 L2 participants, only three had studied abroad for one month after turning 18. The rest of the students had no study-abroad experience. The English proficiency levels of the L2 participants were in the B1–B2 bands on the Common European Framework of Reference (CEFR) scale, as determined by the listening component of the Oxford Placement Test (M = 81.08, SD = 6.43).
Instruments and Procedures
Tasks and Task Complexity Manipulations
The eight experimental tasks took the form of decision-making monologic tasks. Half of the eight tasks required participants to select four essential items from a list of eight to take with them in critical situations: having to swim to a desert island when their boat was sinking, to walk to the nearest emergency shelter after surviving an earthquake, to drive to an emergency accommodation upon receiving a flood alert, and to get to the closest camp when surviving a plane crash. The remaining four tasks required participants to select five people from a set of eight as part of further disaster situations: deciding who should receive a potentially life-saving vaccination, who should take the parachutes from a plane about to crash, who to save first from a building on fire, and who to select as government advisors in a health emergency (see the Supporting Information online for each task). Two applied linguistics experts, also experienced language teachers in the Japanese context, assisted with making the tasks and choice of items/people culturally appropriate for the participants. We also piloted the tasks with participants similar in demographics to the actual participants.
For each task, we designed simple and complex versions. In the simple versions of the tasks, the decisions among items or people were designed to be more straightforward. For example, a bottle of wine could be more easily eliminated than a bottle of water or a smoking advertiser than a doctor. Two highly experienced task-complexity researchers were asked to judge whether the task versions designed to be more complex indeed involved more complex decisions. Both experts evaluated all the task versions intended to be more complex as more cognitively demanding than their simple counterparts, resulting in 100% agreement between the two experts.
Self-rating scales
The self-rating scales evaluated participants’ perceptions of (a) the mental effort required by the task and (b) the difficulty of the task. They were instructed to judge each statement on a 9-point Likert scale immediately after carrying out a task version. The questionnaire items were presented to the participants in English for the L2 performances and in Japanese for the L1 performances. The items were worded as follows:

Data collection
The participants took part in one individual session. First, we obtained informed consent, followed by the administration of a paper-and-pencil background questionnaire (10 min) and the Oxford Placement Listening Test (15 min max). The rest of the experiment took place in the fMRI scanner. First, participants were introduced to the task instructions and experimental procedures. As part of this practice phase, they read the task instructions, listened to a sample practice task performance, carried out the practice task, and completed the task perception questionnaire. Participants were also given detailed guidance on how to reduce head movements while undergoing the fMRI scans. The practice trial within the MRI machine was also aimed at familiarizing participants with how to control head movements while speaking. To further restrict head motion, participants’ heads were secured with a combination of a foam pad and a restraint belt. Participants were encouraged to ask any questions they had regarding the procedures.
Next, participants moved on to completing the two experimental sessions, English and Japanese, inside the MRI. In each session, they carried out two simple and two complex tasks, presented in a counterbalanced order across participants. During each trial, participants had 1 minute to review the task instructions, 2 minutes to carry out their oral performance, and 10 seconds to complete the self-rating scales. Participants were asked to verbally provide their ratings. There was a 15-second rest between trials. The total time for the fMRI experiment was 834 seconds for each session. Participants’ spoken responses were captured using an MRI-compatible noise-cancelling microphone (Optoacoustics Ltd., Moshav Mazor, Israel).
Scanning was performed using a 3T Philips Achieva dStream scanner. Functional images were acquired using gradient-echo planer image sequences with the following parameters: echo time=30 ms, flip angle=80°, slice thickness=3 mm, field of view=192 mm, 64×64 matrix. Thirty-two axial slices spanning the entire brain were obtained every 2 seconds. After excluding three dummy scans performed due to the T1 saturation effect, 417 volumes were obtained for each participant and session. T1-weighted anatomical images were also acquired from each participant to serve as a reference for anatomical correlates. The following preprocessing procedures were performed using Statistical Parametric Mapping (SPM12) software (Wellcome Centre for Human Neuroimaging, London, UK) and MATLAB (MathWorks, Natick, MA, USA): adjustment of acquisition timing across slices, correction for head motion, coregistration to the anatomical image, spatial normalization using the anatomical image and the MNI template, and smoothing using a Gaussian kernel with a full width at a half maximum (FWHM) of 8 mm.
Data analyses
Behavioral analyses
All 96 L2 speech performances were transcribed and then annotated for pauses by the fourth author. Given that only a few filled pauses were identified, our further analyses focused on silent pausing. Silent pauses were identified manually, given that the audio data obtained from the fMRI scanner were noisy to allow for automatic detection of pauses. Following previous research (Goldman-Eisler, 1968), the threshold for silent pauses was defined as 250 ms. We annotated the data for clause boundaries in TextGrid files using the Praat software (Boersma & Weenink, Reference Boersma and Weenink2022). Then, we coded the pauses according to whether they appeared within or between clauses. To check reliability, we randomly selected three participants (12.5% of the data), and the first author also coded their speech samples. The intercoder agreement was high for all coding categories (pause identification: 100%, clause boundary: 99.5%, and pause location: 99.6%). Once we completed the coding, we divided the number of pauses by the total number of clauses for each task and used the resulting proportions in further analyses involving pause frequency.
fMRI analyses
In our study, we conducted a detailed fMRI analysis using SPM12, employing conventional within-participant (first-level) and between-participant (second-level) analyses. Starting at the first level, we performed a voxel-by-voxel multiple regression analysis in the time courses to estimate brain activation for each participant. We focused on the hemodynamic response during L2 task performances, hypothesizing variations in brain activity related to pause locations (mid-clause and end-clause) during simple and complex tasks. To quantify these variations, we constructed a design matrix incorporating four regressors corresponding to different task conditions: simple mid-clause (SM), simple end-clause (SE), complex mid-clause (CM), and complex end-clause (CE). These regressors were defined by the timing of silent pause onsets and duration of pauses, information derived from behavioral speech analysis for each participant. Additionally, six movement parameters (three translations and three rotations) were included as noninterest regressors. For each participant, contrast images were generated to compare the effect of pause location ([SE+CE > CM+SM] and [CM+SM > SE+CE]), task complexity ([CE+CM > SE+SM]), and their interactions ([CE -CM > SE – SM]) and ([CM- CE > [SM – SE]). These contrast images distilled the essence of brain activity differences for our specific hypotheses, including the main effects and interactions within our 2×2 factorial design.
Transitioning to the second level of analysis, we applied a one-sample t-test to these contrast images across all participants. This crucial step was aimed at identifying whether the patterns of brain activity changes we observed were consistent and significantly different from zero across the group. We employed a random effects model to conduct statistical inference on the contrasts of parameter estimates, ensuring that our findings could be generalized to the broader population. This structured approach, from individual-level analyses to group-level statistical inferences, allowed us to comprehensively investigate the neural correlates of task complexity and pause locations in L2 speech tasks.
To address the challenge of multiple comparisons inherent in fMRI data, we set a statistical threshold of p <.05, implementing whole-brain cluster size correction as recommended by Slotnick (Reference Slotnick2017). A Monte Carlo simulation with 10,000 iterations on a 64×64×32 whole-brain grid, smoothed with an 8-mm FWHM Gaussian kernel, established a voxel threshold of p <.001. This threshold was corrected to p <.05 with a cluster extent threshold of 45 voxels, ensuring the robustness of our findings. Activation peak coordinates were reported in the Montreal Neurological Institute space and identified using the automated anatomical labeling atlas in SPM12. The Marsbar toolbox (Brett et al., Reference Brett, Anton, Valabregue and Poline2002) was used to extract parameter estimates in the four conditions for each participant to illustrate the activation profile in the observed brain area.
Results
Preliminary analyses
Task complexity and self-ratings
Table 1 provides the descriptive statistics for the mental effort and task difficulty self-ratings given by participants across simple and complex task versions and L2 and L1 performances. We built a series of linear mixed-effects models, for the L1 and L2 data separately, to investigate the extent to which task complexity affected participants’ self-ratings. The fixed effect in our models was task complexity, and participants and task prompt served as random effects. The dependent variable was the self-rating of mental effort or task difficulty in the models. As shown in Table 2, task complexity did not emerge as a significant predictor of either mental effort or task difficulty self-ratings for the L2 task performances. However, the L1 data yielded a significant difference in task difficulty ratings for the simple and complex task versions. In sum, the participants did not perceive the simple and complex tasks as requiring differential mental effort or as different in difficulty when they completed them in their L2. However, they perceived the complex task versions as more difficult (but not requiring more mental effort) when completing them in their L1. Task complexity explained approximately 2% of the variance in L1 self-ratings of task difficulty.
Table 1. Ratings of mental effort and task difficulty.
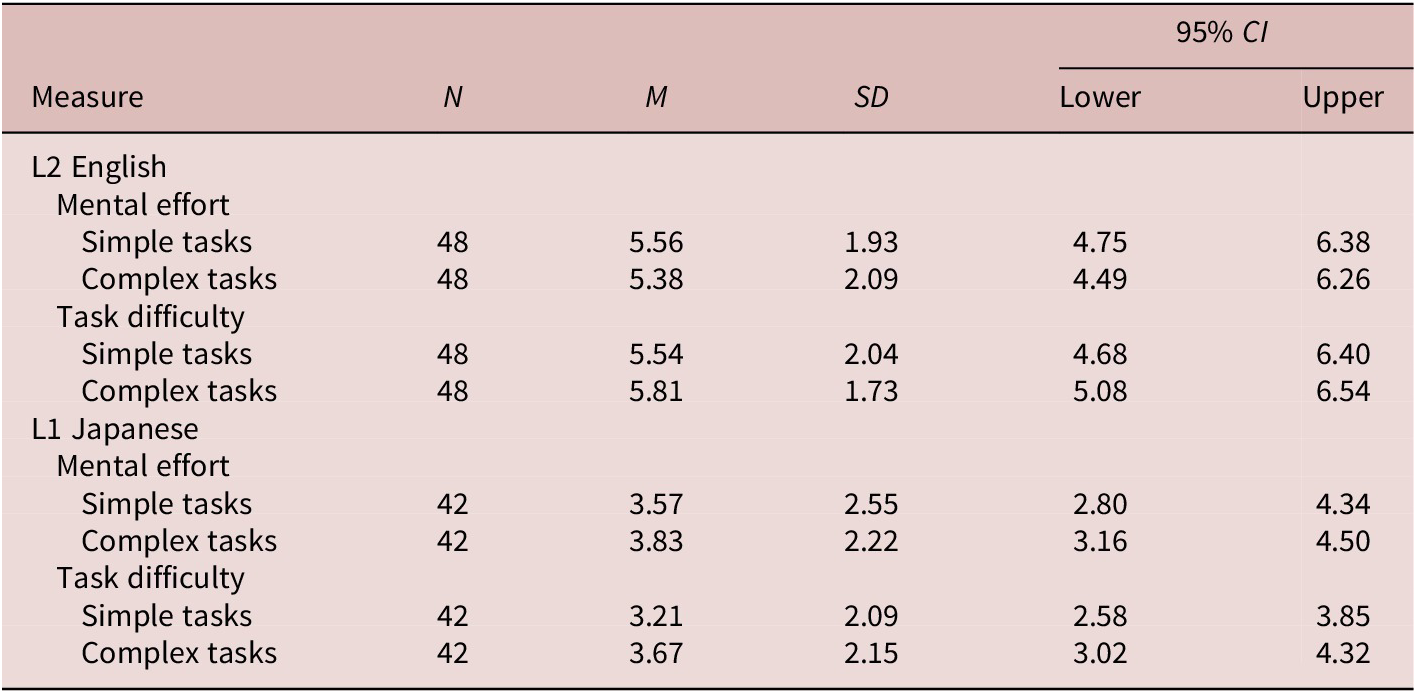
Table 2. Results for models examining the effects of task complexity on ratings of mental effort and difficulty.
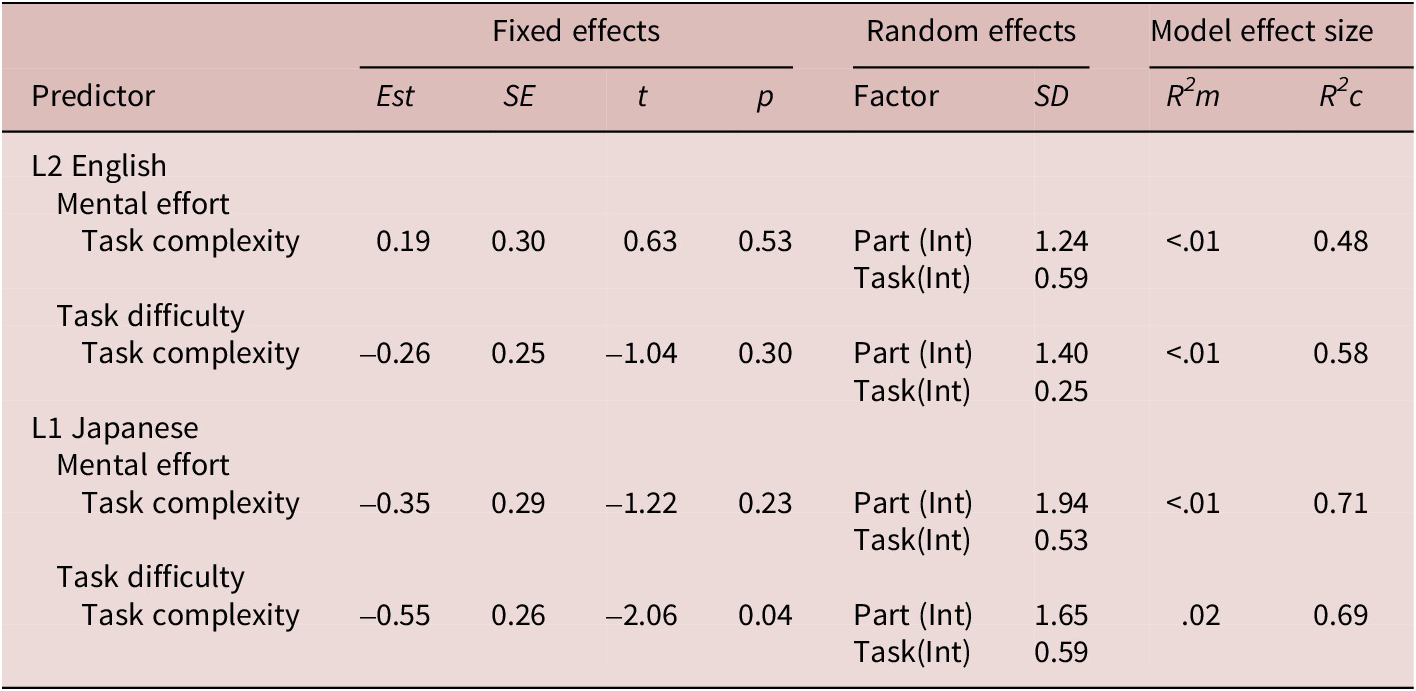
Speech length by task complexity
Table 3 provides the unpruned word count for participants’ L2 performances across the simple and complex task conditions. We constructed a linear mixed-effect model, with word count as the dependent variable, task complexity as a fixed effect, and participants and task prompt as random effects. As shown in Table 4, participants’ length of speech did not vary by the intended task complexity manipulation, accounting for less than 1% of the variance in word count.
Table 3. Word count for simple and complex tasks.

Table 4. Results for model examining the effects of task complexity on speech length.

Research question 1: effects of task complexity on pause frequency and length
Table 5 provides the descriptive statistics for the frequency and length of silent pauses by location, that is, whether the pauses were observed mid-clause or end-clause. The table also demonstrates pausing patterns across the two task complexity conditions.
Table 5. Silent pause frequency and length by task complexity and pause location.
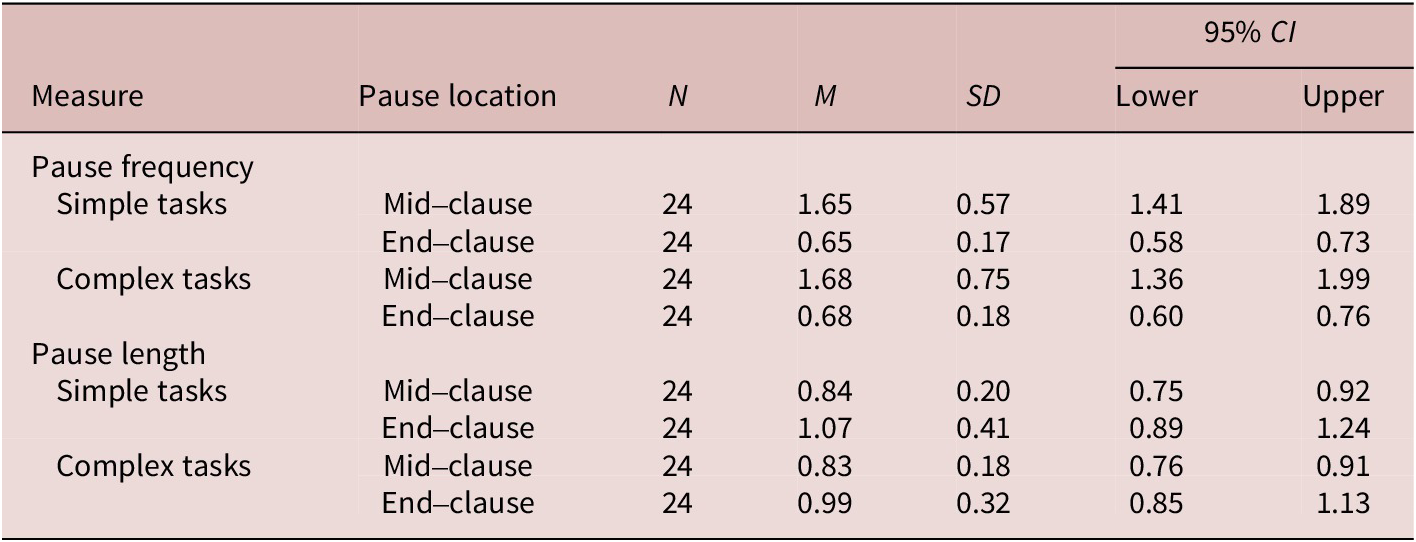
To address our first research question, we constructed a linear mixed-effects model to examine the extent to which task complexity affected pausing behaviors. The fixed effects in our models were task complexity, pause location, and their interaction; and the random effects included participants and task prompt. The dependent variable was the frequency of silent pauses. As shown in Table 6, the analysis yielded a significant effect for pause location only, with participants pausing less often but longer in end-clause than mid-clause positions. The fixed effects explained substantially more variance in the model for pause frequency than pause length, accounting for 52% and 2% of the variation respectively. This indicates that pause location, mid- versus end-clause, affected pause frequency to a greater extent than pause length.
Table 6. Results for models examining the effects of task complexity, pause location, and their interaction on pausing behaviors.
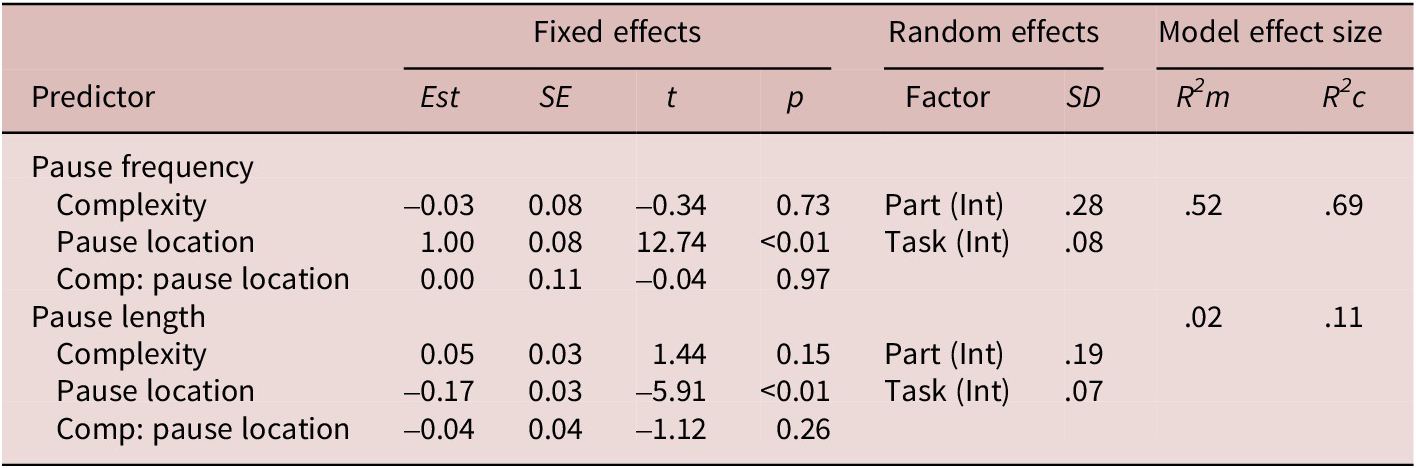
Research question 2: effects of task complexity on neural processes during pausing
Unlike our behavioral analyses, the fMRI analyses found a main effect for task complexity, but no interaction between task complexity and pause location. In complex tasks as to compared simple tasks, pauses (regardless of location) elicited greater activation in broad brain areas related to theory-of-mind activities, conceptualization, and preparation of speech. These areas included the bilateral precentral gyri, right putamen, and left cerebellum for speech planning and monitoring (Hervais-Adelman et al., Reference Hervais-Adelman, Moser-Mercer, Michel and Golestani2015; Runnqvist et al., Reference Runnqvist, Chanoine, Strijkers, Pattamadilok, Bonnard, Nazarian, Sein, Anton, Dorokhova, Belin and Alario2021; Silva et al., Reference Silva, Liu, Zhao, Levy, Scott and Chang2022); and left angular gyrus (AG), precuneus, and mPFC from the theory-of-mind network for conceptualization (Ferstl et al., Reference Ferstl, Neumann, Bogler and von Cramon2008; Morales et al., Reference Morales, Patel, Tamm, Pickering and Hoffman2022; Sassa et al., Reference Sassa, Sugiura, Jeong, Horie, Sato and Kawashima2007) (see Table 7 and Figure 1). Conversely, simple tasks did not yield higher activation for pauses than complex tasks.
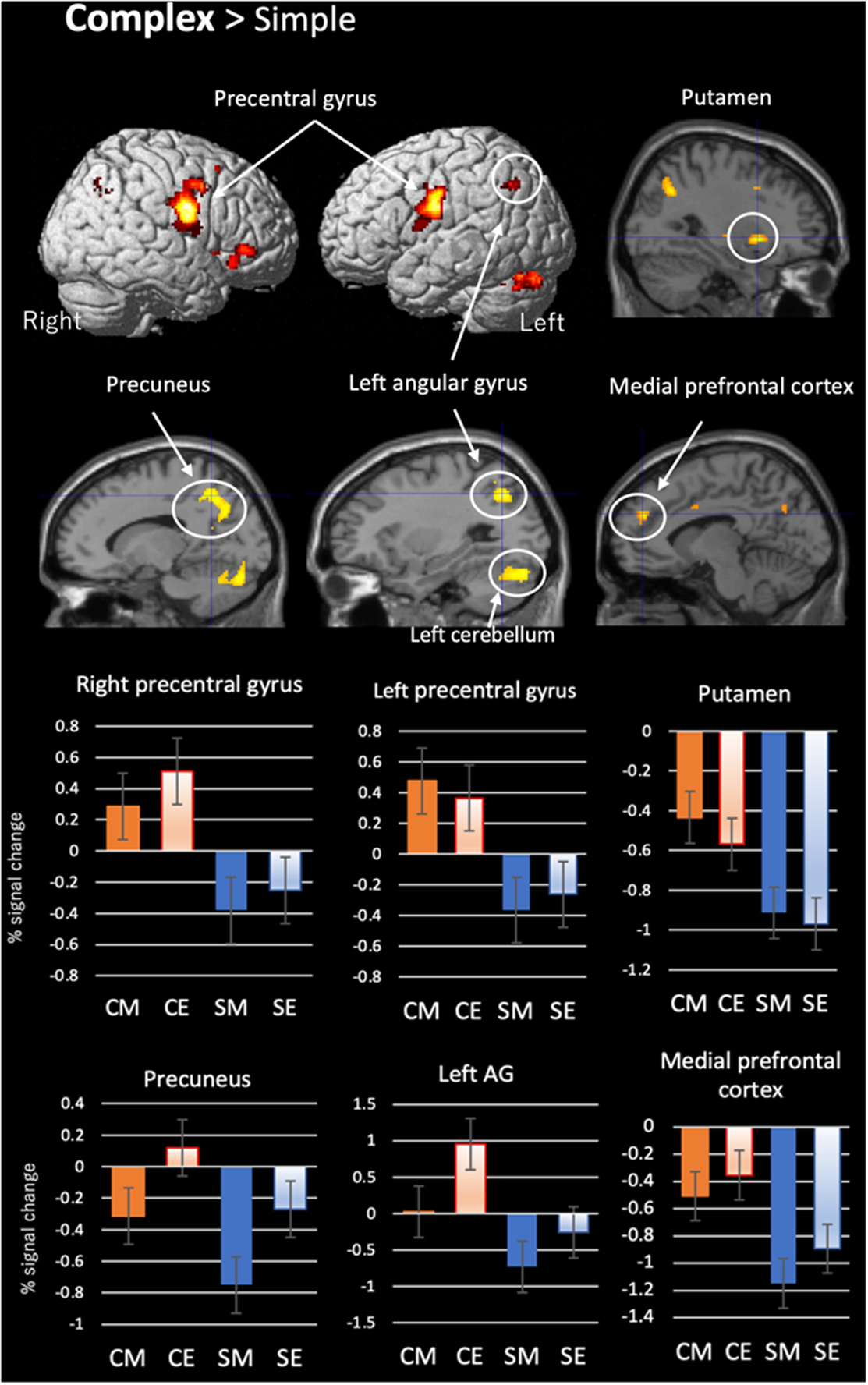
Figure 1. Brain areas showing greater activation associated with pauses during complex than simple tasks (complex > simple).
Note: The activation profile represents the mean percent signal change of each condition; CM: complex mid-clause, CE: complex end-clause, SM: simple mid-clause, SE: simple end-clause. Error bars indicate the standard error of the mean (SEM).
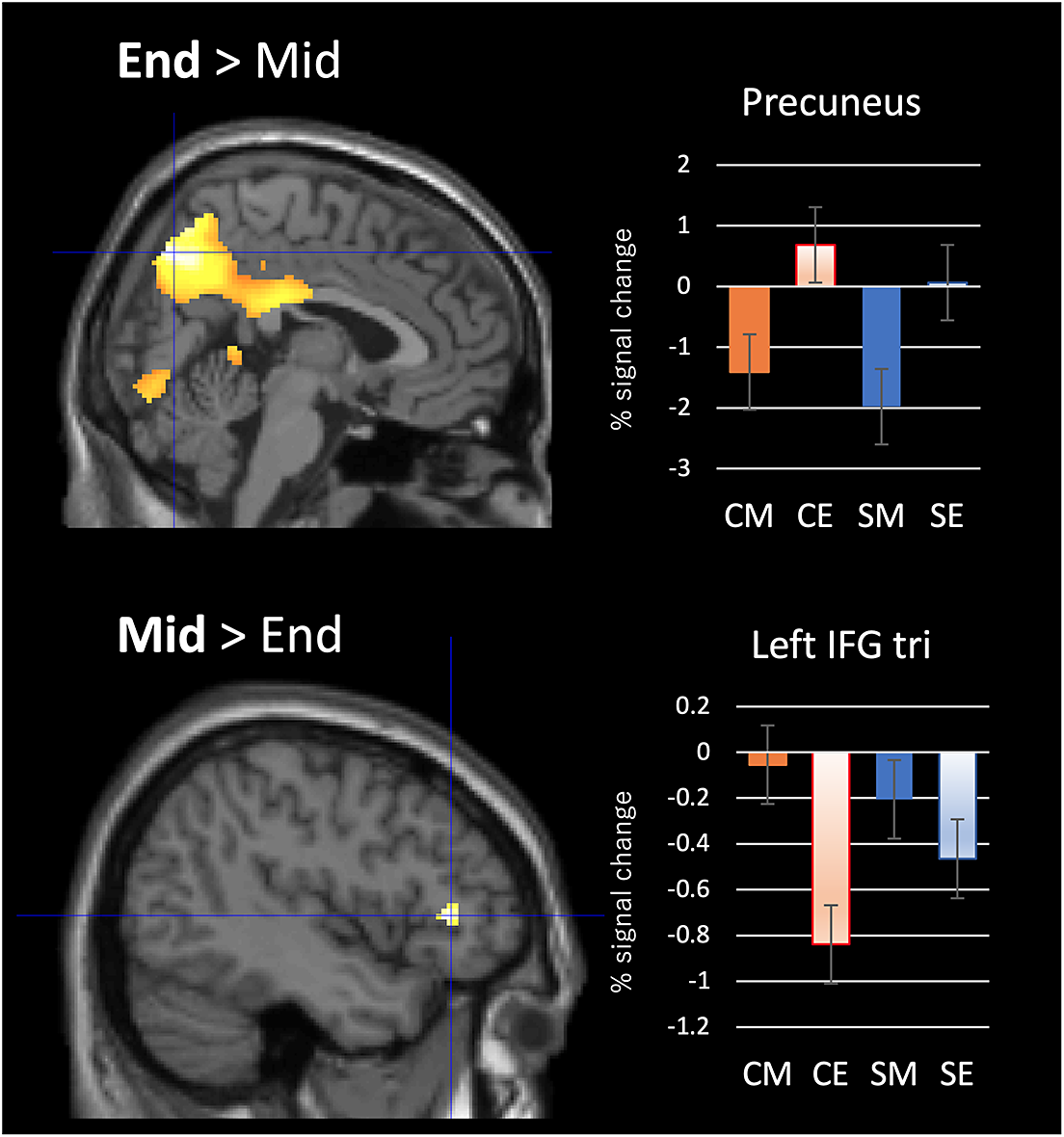
Figure 2. Brain area activation at end- and mid-clause pause locations.
Note: The activation profile represents the mean percent signal change for each condition; CM: complex mid-clause, CE: complex end-clause, SM: simple mid-clause, SE: simple end-clause. Error bars indicate SEM. Left IFG tri: left triangular part of inferior frontal gyrus.
Table 7. Brain areas showing greater activation associated with pauses during complex than simple tasks (complex > simple).
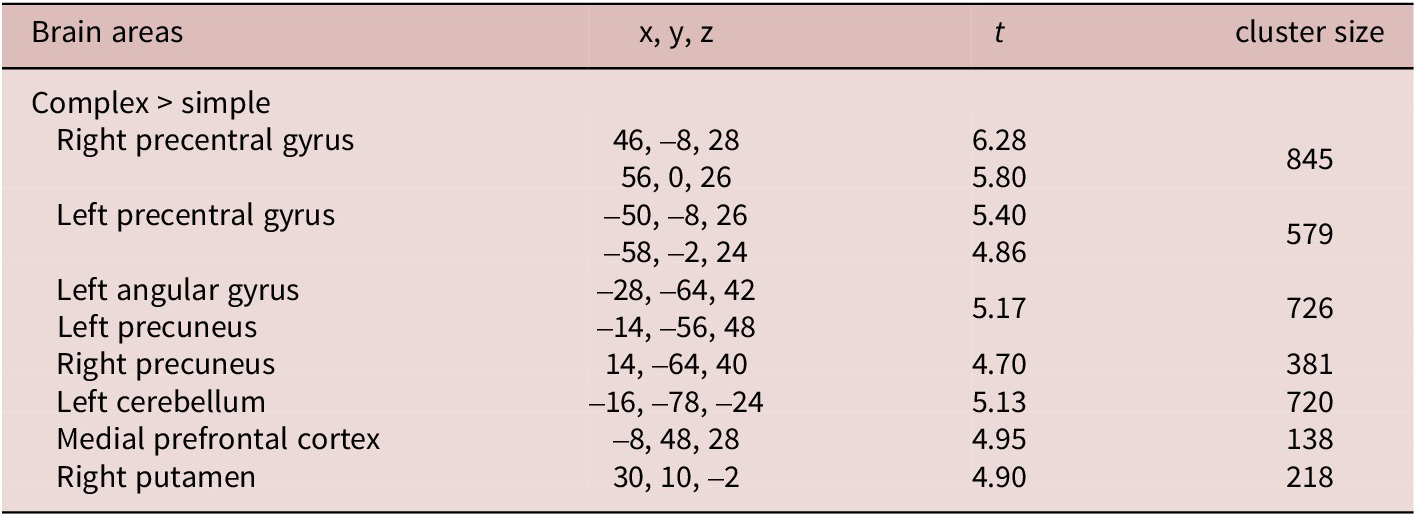
Notes. For each area, the coordinates (x, y, z) of the activation peak in MNI space, peak t-value, and size of the activated cluster in number (k) of voxels (2×2×2 mm3) are shown for all subjects (n = 24). The threshold was set at p <.05 FWE correction with the cluster level.
Similar to the behavioral results, the neuroimaging data also identified a pause location effect. As shown in Table 8, pauses at end-clause locations showed increased activation in the bilateral precuneus, extending to the posterior cingulate cortex and the left angular gyrus. These brain areas are associated with theory-of-mind activities, regulating internal thought and conceptualization (Ferstl et al., Reference Ferstl, Neumann, Bogler and von Cramon2008; Sassa et al., Reference Sassa, Sugiura, Jeong, Horie, Sato and Kawashima2007; Smallwood et al., Reference Smallwood, Gorgolewski, Golchert, Ruby, Engen, Baird, Vinski, Schooler and Margulies2013), and bilateral cerebellum, which is involved in speech planning (Runnqvist et al., Reference Runnqvist, Chanoine, Strijkers, Pattamadilok, Bonnard, Nazarian, Sein, Anton, Dorokhova, Belin and Alario2021). In contrast, mid-clause pauses, when compared to end-clauses, led to greater activation in the left triangular part of the inferior frontal gyrus (IFG), a key language area (Friederici, Reference Friederici2011), and the right insula, which is associated with motor speech control (Oh et al., Reference Oh, Duerden and Pang2014).
Table 8. Brain areas exhibiting differential activation between pauses at end- and mid-clause pause locations.
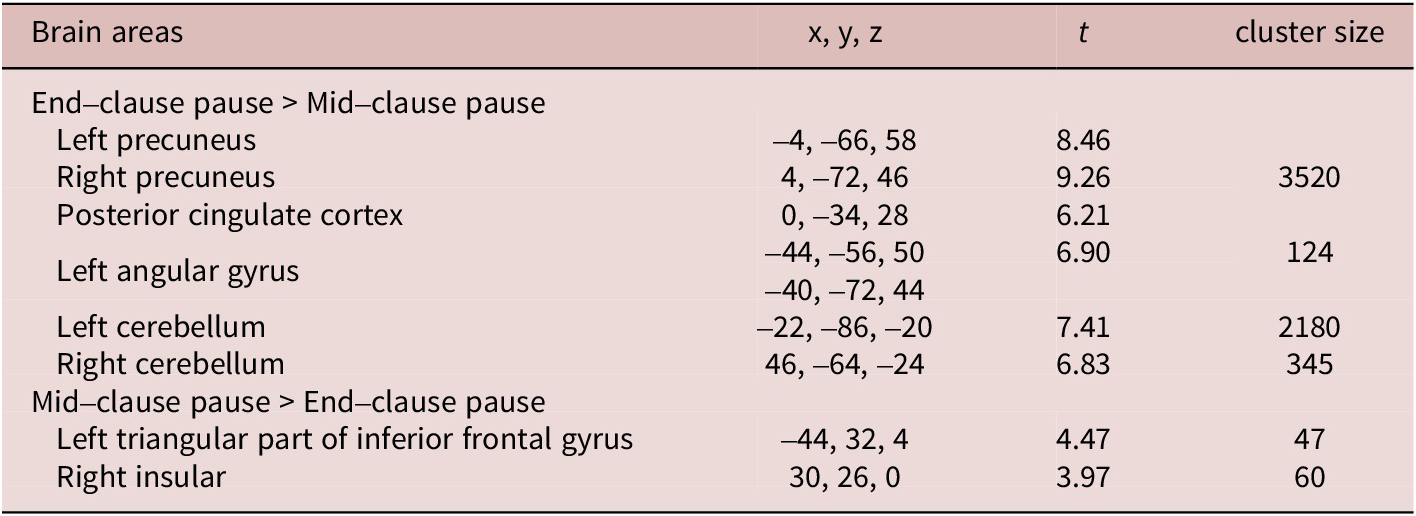
Notes. For each area, the coordinates (x, y, z) of the activation peak in MNI space, peak t-value, and size of the activated cluster in number (k) of voxels (2×2×2 mm3) are shown for all subjects (n = 24). The threshold was set at p <.05 family-wise correction (FWE) with the cluster level.
In sum, no interaction effect was detected between task complexity and pause location in the whole brain analysis, but we detected a main effect for task complexity and pause location. However, greater activation was found in the precuneus and the left angular gyrus during complex as compared with simple tasks (both mid- and end-clause locations) and at end-pause locations as compared with mid-clause locations (during both simple and complex tasks), reflecting greater cognitive demands on conceptualization.
Research question 3: relationships between self-ratings of mental effort and task difficulty, pausing patterns, and neural correlates of pausing
Relationships of mental effort and task difficulty self-ratings to pause frequency and length
To address our third research question, we constructed a series of linear mixed-effects regression models to examine the extent to which participants’ L2 ratings of mental effort and task difficulty predicted pause frequency and pause length. The fixed effects in our models were participants’ self-ratings of mental effort or difficulty, pause location, and their interaction; and the random effects included participants and task prompt. The dependent variable was the frequency or length of silent pauses.
As shown in Table 9, the models for pause frequency yielded a significant interaction between mental effort and pause location and between task difficulty and pause location. As Figure 3 illustrates, the more often participants paused within clauses, the more effortful and more difficult they perceived the task to be. The fixed effects in both models explained 55% of the variance in the frequency of pausing.
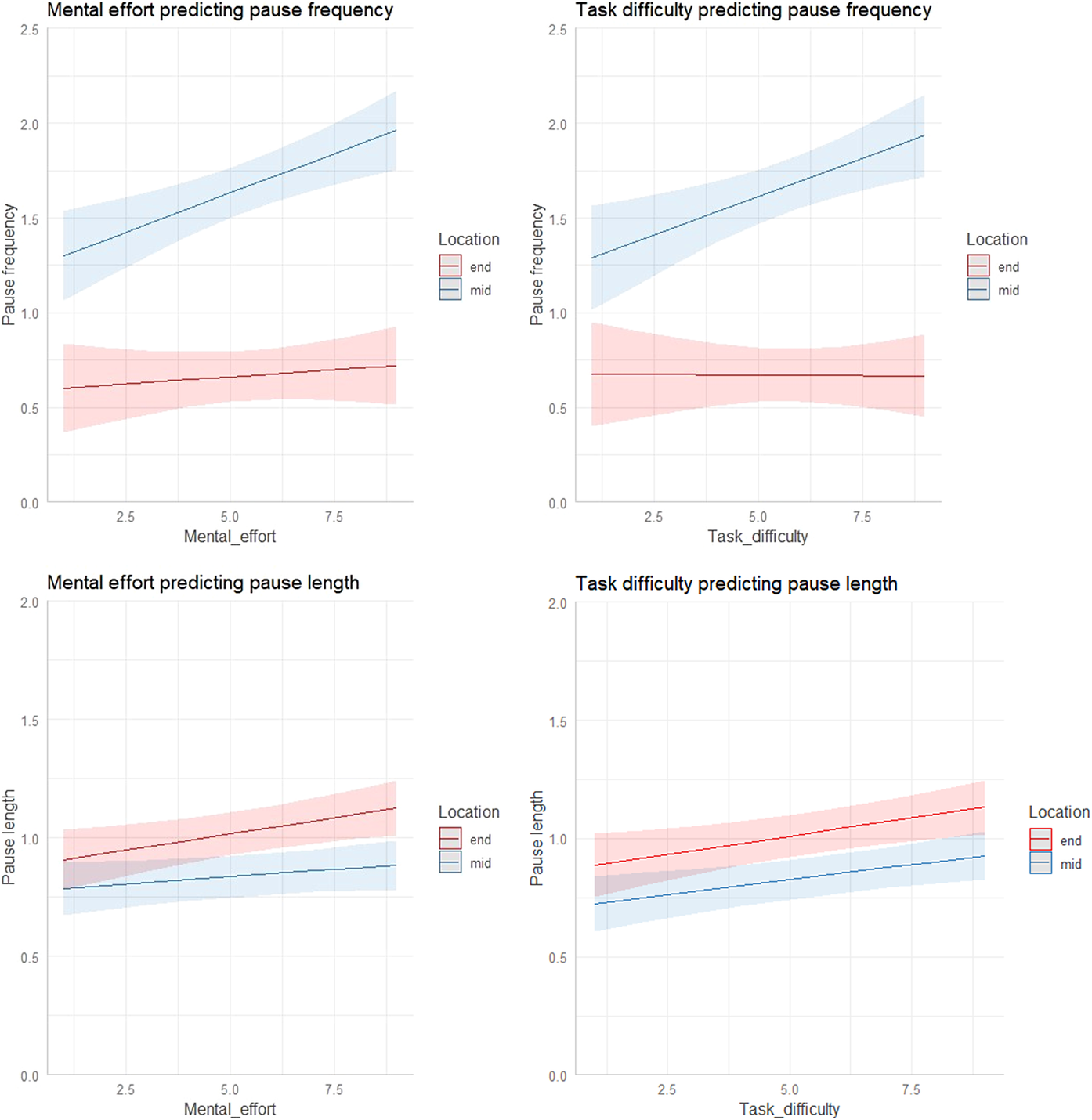
Figure 3. Self-ratings of mental effort and task difficulty predicting pausing behaviors.
Table 9. Results for models examining self-ratings, pause location, and their interaction as predictors of pausing behaviors.
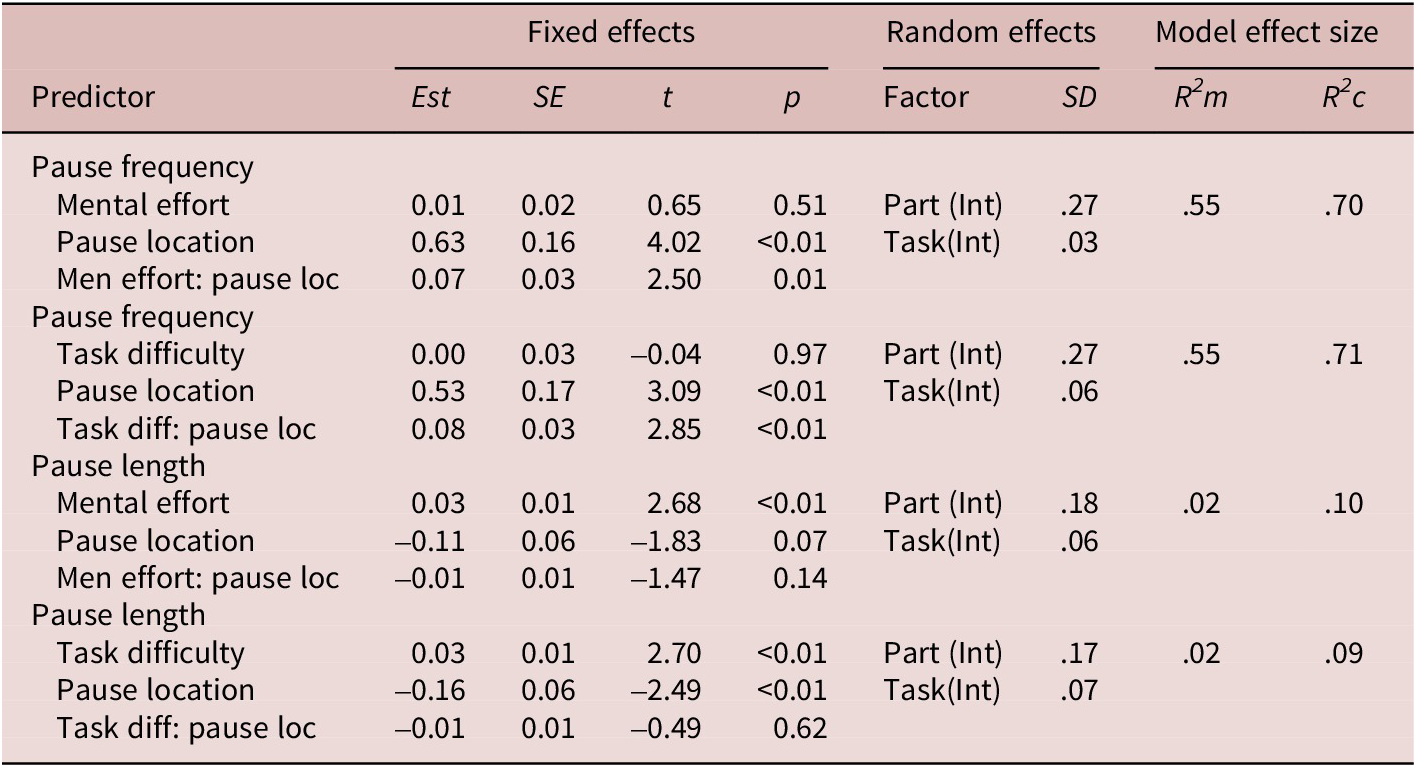
Table 9 also shows that the model involving pause length and mental effort identified mental effort as a significant predictor of pause length. Figure 3 demonstrates that participants who paused longer reported exerting greater mental effort. Similarly, the model including pause length and task difficulty found that task difficulty predicted the length of pausing. As shown in Figure 3, the longer participants paused, the more difficulty they felt the task posed. The analysis also yielded a main effect for pause location, indicating that end-clause pauses were significantly longer than mid-clause pauses during participants’ task performance.
Relationships of mental effort and task difficulty self-ratings to brain activity during pauses
To elucidate the relationship between participants’ L2 self-ratings of mental effort and task difficulty and their actual brain activity at mid-clause and end-clause pause locations, we performed a parametric modulation analysis using SPM12, separately for the mental effort or task difficulty data. In the first-level analysis, we added the mental effort/task difficulty rating for each task performance as a parametric modulator to the main regressor for mid- and end-clause locations. Then, in the second-level analysis, we tested the parametric regressors of mental/task difficulty with a one-sample t-test. While we found no significant effect for task difficulty, the left caudate nucleus (x, y, z coordinates = –4, 14, –4, t=4.90, 104 voxels) showed increased activation as perceived mental effort increased at mid-clause pause locations (see Figure 4). However, no such effect was observed at end-clause pause locations.
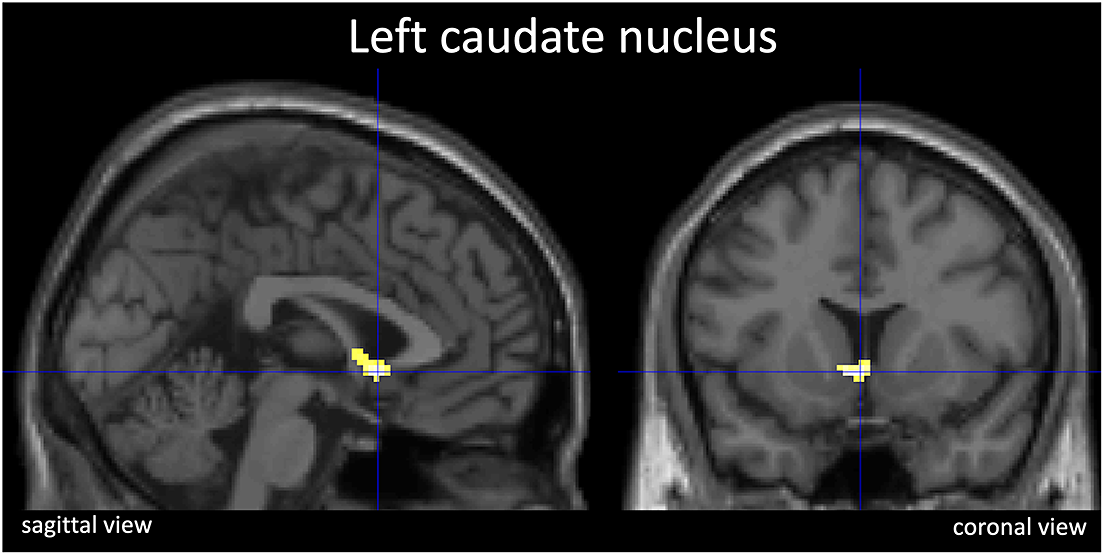
Figure 4. Perceived mental effort effect in mid-clause positions.
Discussion
Our first two research questions were concerned with the effects of task complexity on observable pausing patterns and associated neural processes. Building on models of speech production (Kormos, Reference Kormos2006; Levelt, Reference Levelt, Brown and Hagoort1999) and task-based performance (Robinson, Reference Robinson and Robinson2001a; Skehan, Reference Skehan2009), we hypothesized that increased task complexity would lead to greater pressure on the conceptualizer, given the enhanced conceptual demands more complex tasks exert. Due to the greater strain on conceptualization processes, we also anticipated that participants would have fewer attentional resources to allocate to linguistic encoding, resulting in increased pressure on the formulator. In turn, we assumed that more complex tasks would lead to an increase in pause frequency and length at both pause locations, as pausing at mid-clause and end-clause locations have been related to involvement in the formulation and conceptualization processes respectively. In parallel, we also expected that more complex tasks would activate conceptualization- and speech-related brain areas to a greater extent during pauses, with more pronounced effects observed for end-clause and mid-clause pauses respectively. Our results have provided no support for our behavioral predictions, yielding no task complexity effects for either pause length or frequency. The neural data, however, largely confirmed our hypotheses. Although we found no interaction effect between task complexity and pause location, we detected greater activation in theory-of-mind-, conceptualization-related brain areas (precuneus and angular gyrus) at end-clause positions and in speech planning and monitoring areas (bilateral precentral gyri and right putamen) at both pause locations during more complex task performance. It is also important to highlight that, while the expert judgments of task complexity and L1 difficulty ratings provided evidence in support of the validity of our task manipulation, participants’ L2 self-ratings of mental effort and task difficulty yielded no significant difference between tasks designed to be more and less complex. Interestingly, however, in addressing our third research question, we found that the more effortful and more difficult participants perceived the task to be, the more often and longer participants paused mid-clause. In line with this, the fMRI data, during mid-clause pause positions, revealed that higher mental ratings were correlated with increased activation in the left caudate nucleus. This region functions as a language control area, as indicated by Crinion et al. (Reference Crinion, Turner, Grogan, Hanakawa, Noppeney, Devlin, Aso, Urayama, Fukuyama, Stockton, Usui, Green and Price2006).
This is an intriguing set of results, yielding several points for discussion. One issue concerns the discrepancy between our behavioral and neural findings. A possible explanation for the observed task complexity effects on the neural data but the lack of those on the behavioral indices might be that the difference in complexity across the two task versions was not sufficiently large to be detected through the behavioral measures employed in the present study. Although in previous research task-related variables were found to influence pausing (Lambert et al., Reference Lambert, Kormos and Minn2017; Wang, Reference Wang and Skehan2014), our task manipulation and its impact on participants might not have been robust enough to affect observable pausing patterns. Notably, the mental effort and task difficulty ratings did not identify any impact of task complexity either, indicating an alignment between participants’ pausing behaviors and task perceptions. The fact, however, that the neural measures did yield task complexity effects affirms that, consistent with the experts’ judgments and the L1 difficulty ratings, the simple and complex task versions did actually instigate processing differences. In other words, it appears that the neural data were more sensitive to detecting the influence of task complexity than the pausing patterns we observed. A lack of convergence between our behavioral and neural measures is not a unique finding. Researchers investigating first language writing processes, for example, found that similar observable behaviors do not necessarily implicate the same brain mechanisms, that is, solely relying on behavioral-level analyses may mask processing differences (Richards et al., Reference Richards, Berninger, Yagle, Abbott and Peterson2017).
Another interesting, methods-related finding concerns the link we observed between participants’ self-ratings and the pause-related behavioral and neural data. While participants’ L2 self-ratings of mental effort and task difficulty did not yield any difference for our intended task complexity manipulation contrary to our expectation, we found that higher self-ratings of mental effort and task difficulty were associated with increased mid-clause pause length and frequency. We also found that higher self-ratings of mental effort were related to greater activation in a language-control brain area (left caudate nucleus) at mid-clause pause locations. Given that mid-clause pausing is assumed to indicate difficulty with linguistic encoding processes (de Jong, Reference De Jong2016; Field, Reference Field and Taylor2011; Lambert et al., Reference Lambert, Kormos and Minn2017; Suzuki & Kormos, Reference Suzuki and Kormos2023; Tavakoli et al., Reference Tavakoli, Nakatsuhara and Hunter2017, Reference Tavakoli, Nakatsuhara and A-M2020), these results could be interpreted as suggesting that participants largely based their self-ratings on the linguistic rather than the conceptual difficulty that they had experienced during task performance. This interpretation is also aligned with the findings for L1 self-ratings of task difficulty, which revealed that participants perceived the complex task versions as more difficult. When participants carried out the tasks in their L1, they were unlikely to experience linguistic difficulty, making it more probable that they would judge the difficulty of the tasks based on the conceptual demands they posed (Lee, Reference Lee2019).
An alternative and/or additional explanation could be that the linguistic difficulty posed by the L2 tasks was perceived by participants to be considerably larger than the issues they might have encountered conceptualizing their message. Given the context of the study, this might not be surprising; in Japan people are often exposed to disaster preparedness and response training, naturally decreasing the conceptual demands imposed by disaster-related tasks. This issue could be disentangled in future research by asking participants to provide separate task difficulty and mental effort ratings for linguistic and conceptual task demands. This more refined self-perception data could also assist with obtaining a fuller picture of perceived speech production processes during task-based work, with potential theoretical implications for task-based models and practical implications for task-based teaching.
Another potential methodological implication emerging from our study concerns the use of L1 self-ratings when establishing task complexity. In line with Lee’s (Reference Lee2019) proposal, our results suggest that, if the researchers’ aim is to establish the conceptual demands of tasks, obtaining L1 rather than L2 self-ratings of task difficulty might yield more fine-tuned results. As we discussed earlier, during L1 performance speakers are less likely to encounter difficulty with linguistic encoding, increasing the likelihood that self-ratings provide an accurate judgment of the conceptual difficulty posed by the task. This conclusion is also supported by our fMRI data, which along with the L1 self-ratings, detected an effect for task complexity, contrary to the L2 behavioral data we collected (self-ratings and linguistic performance measures).
It is also worth highlighting that our results suggest that L2 task complexity has neural correlates, providing a new type of evidence for the validity of the construct of task complexity. In particular, the greater activation we observed in areas associated with the theory-of-mind network and conceptualization during complex tasks is aligned with our intended task design manipulation that the more complex task versions would lead to greater conceptual demands. The theory-of-mind system underlines humans’ ability to understand others’ beliefs, desires, and intentions, rendering its successful use crucial for effective social interaction and verbal communication. As the participants in the current study were in imaginary rather than real-life situations, they probably needed to infer what someone would do in these critical situations, therefore making them rely on the theory-of-mind system. If so, it seems logical that the theory-of-mind network would be more involved when participants were conceptualizing their message under more complex conditions, given that these tasks were designed with the intention that participants would imagine and decide how to act in more complex critical situations. Similar to our findings, theory-of-mind-related areas were found to display higher brain activation during written text comprehension activities that require inferencing and interpretation (Ferstl et al., Reference Ferstl, Neumann, Bogler and von Cramon2008), such as reasoning about the intentions and mental states of narrative characters (Mason & Just, Reference Mason and Just2009). This finding is particularly relevant to our study, as, according to Levelt’s (Reference Levelt1989) speech production model, self-monitoring is part of one’s comprehension rather than the production system. Thus, the effect of task complexity could also be interpreted as evidence of participants’ engagement with self-monitoring, involving the theory-of-mind system through the evaluation of how the evolving message would be perceived by potential interlocutors. As discussed earlier, studies of speech production also showed greater neural activation of the theory-of-mind network when participants engaged in communication with others as compared to when they completed description activities without the need to communicate (Jeong et al., Reference Jeong, Sugiura, Suzuki, Sassa, Hashizume and Kawashima2016; Sassa et al., Reference Sassa, Sugiura, Jeong, Horie, Sato and Kawashima2007). Our study, however, is among the first to observe theory-of-mind effects on neural processes during spontaneous L2 speech production.
The results obtained here are also interesting to consider in relation to Robinson’s (Reference Robinson and Robinson2001a, Reference Robinson2011) Cognition Hypothesis and Skehan’s (Reference Skehan1998, Reference Skehan2009) Limited Capacity Model. If we take the observed differences in neural processes across the simple and complex task versions as proof that the task manipulation worked in the present study, we can conclude that the results did not confirm the predictions of the models for the dependent variable of fluency, as the amount of pausing, contrary to the prediction of the models, would not rise as the cognitive demands of the tasks increased. However, the data did provide evidence in support of the task frameworks in that the task complexity manipulations, the independent variable in the models, affected speech production processes, a presumed causal variable in the Cognition Hypothesis and Limited Capacity Model. In particular, the task versions designed to be more complex exerted greater conceptual demands, as reflected in the neural data and predicted by the models.
Besides yielding evidence for the construct of task complexity and related task-based models, our findings reinforce the value of distinguishing between mid-clause and end-clause pausing. As discussed in the literature review, researchers have identified various types of behavioral evidence in favor of the assumption that mid-clause and end-clause pauses are associated with L2 formulation and conceptualization processes, respectively (e.g., de Jong, Reference De Jong2016; Field, Reference Field and Taylor2011; Lambert et al., Reference Lambert, Kormos and Minn2017; Suzuki & Kormos, Reference Suzuki and Kormos2023; Tavakoli et al., Reference Tavakoli, Nakatsuhara and Hunter2017, Reference Tavakoli, Nakatsuhara and A-M2020). The neural data obtained in the current study are aligned with this assumption, given that the fMRI scans found increased brain activity in conceptualization-related brain areas at end-clause but not in mid-clause positions during tasks designed to be more complex. Also, self-ratings were related only to mid-clause pause frequency and brain activity but not to end-clause pausing. In other words, expanding on existing behavioral evidence, the present research has generated novel neural evidence for the merit of distinguishing between pauses based on location.
Limitations and future directions
This study has several limitations that are important to acknowledge and consider in future research. First, although our results suggest that it is highly profitable to carry out fMRI studies to gain more insights into L2 speech production processes and their links to task complexity, this neuroimaging technique, at least for now, yields results that are relatively low in ecological validity. Performing oral tasks in an fMRI scanner differs considerably from carrying L2 oral tasks in real-life settings, which limits the generalizability of our findings. Second, we exclusively employed neuroimaging to tap the link between task complexity and the speech production processes in which participants engaged. It would be interesting to complement neuroimaging techniques with objective behavioral tools such as dual-task methodology and eye-tracking, given that these behavioral methods could provide complementary insights into how task complexity may influence speech production processes. A further limitation has to do with the absence of more comprehensive information about participants’ thoughts during pauses and their perceptions about mental effort and task difficulty. Besides using more fine-grained self-rating tools as suggested earlier, future studies would benefit from employing introspective methods (e.g., stimulated recall) to achieve a more in-depth understanding of participants’ conscious thought processes and perceptions during tasks of varied complexity. Another limitation of our research lies in its relatively small sample size. While a sample size of 24 allowed us to detect medium effect sizes (f =.32) given the repeated-measures design and within-subjects independent variable according to G-power, it was not sufficiently large to reveal small-size effects. Thus, replicating the study with a larger number of participants would be a worthwhile endeavor in the future. A further useful research direction would be to make more detailed distinctions among pause locations and examine corresponding cognitive and neural processes as a function of task manipulations. For example, it would be worthwhile to distinguish pause locations in terms of more specific syntactic constituents (e.g., different types of phrases). In future research, it would also be interesting to examine how the intended task complexity manipulation affected other linguistic areas, such as the linguistic complexity, accuracy, and functional adequacy of learners’ production. Finally, the current study only included Japanese users of L2 English who carried out disaster-related decision-making tasks, future studies are warranted to investigate whether the results we obtained would transfer to different first-language groups, second languages, and task types.
Conclusion
Being among the first to use neuroimaging to investigate task-related effects on L2 spontaneous oral production, the primary aim of this study was to provide novel insights into the neural correlates of task complexity. Our results identified differences in brain activation patterns across simple and complex versions of decision-making tasks, providing neural evidence in support of the construct of task complexity and the validity of the task manipulation in the present study. In contrast, however, participants’ L2 subjective self-ratings of task difficulty, contrary to their L1 self-ratings, did not yield an effect for task difficulty. Our second goal was to explore the potential value of using neuroimaging to examine the impact of task-related variables on spontaneous oral production. While our L2 behavioral measures identified no influence of task complexity, the fMRI scans revealed that brain activation patterns varied as a function of task complexity consistent with participants’ L1 self-ratings.
Overall, we interpreted these findings as suggesting that brain imaging was more sensitive to detecting small-size task complexity effects than the more traditional L2 self-ratings and pausing measures, thus confirming the value of triangulating behavioral measures with neuroimaging data. Importantly, this is not to suggest that task complexity researchers and practitioners should move away from using subjective self-ratings to establish task difficulty and from linguistic performance measures to examine task effects on L2 production. A large body of research indicates that these tools are likely to detect task complexity differences, probably larger in size than the ones observed here, and thus likely to generate meaningful results from a practical perspective. Importantly, however, our findings, if replicated, do imply that researchers might benefit from greater use of L1-ratings and expert judgments to establish task complexity. As compared to L2 self-ratings, these tools appear to yield more sensitive results aligned with neural measures, while being equally practical.
Supplementary material
The supplementary material for this article can be found at http://doi.org/10.1017/S0272263124000421.
Data availability statement
The experiment in this article earned the Open Materials badge for transparent practices. The materials are available at https://www.iris-database.org/details/gsCp3-6k9Ej.
Acknowledgements
This research was supported by the UCL-Tohoku University Strategic Partner Funds; the Designated National University Core Research Cluster of Disaster Science, Tohoku University, Japan; JSPS KAKENHI Grant Number 23K21946; and the ESRC-AHRC UK-Japan SSH Connections Grants (ES/S013024/1).