INTRODUCTION
Research findings relevant to bi/multilingualism are based on data collected from particular samples of language users. Among the many ways that the particulars of a sample can differ, language proficiency may have the largest effect on responses to linguistic stimuli in a laboratory or interventions in a classroom. As Hulstijn (Reference Hulstijn2012) pointed out, language proficiency plays a crucial role in cognitive studies of bilingualism as a participant selection criterion or as an independent, moderating, or control variable in analyses. Thus, adequately characterizing the proficiency of participants is critical to understanding the findings of any one study as well as being able to generalize findings more broadly (Gaillard & Tremblay, Reference Gaillard and Tremblay2016; Norris & Ortega, Reference Norris and Ortega2000, Reference Norris, Ortega, Gass and Mackey2012; Thomas, Reference Thomas1994; Tremblay, Reference Tremblay2011).
Unfortunately, it has not always been the case that researchers have assessed participant proficiency using standardized measures. Thomas (Reference Thomas1994, Reference Thomas, Norris and Ortega2006) suggested in both of her highly influential syntheses that there is little understanding about what proficiency is and how it can be measured for research purposes. Upon reviewing second language acquisition (SLA) research from the late 1980s /early 1990s and then once again research from 2000 to 2004, she found that it was common for researchers to use their intuition, institutional status of participants (e.g., language course enrollment), or “in-house” measures (i.e., not used outside of a current study or context) to characterize learners’ proficiency. The obvious problem with such approaches is that it limits the comparability of results across studies and hinders the systematic accumulation of knowledge in the field (Norris & Ortega, Reference Norris, Ortega, Doughty and Long2003, Reference Norris, Ortega, Gass and Mackey2012). In another synthesis focusing on research from 2000 to 2008, Tremblay (Reference Tremblay2011) showed limited improvements in the use of standardized measures. Along the same lines, Hulstijn (Reference Hulstijn2012) conducted a review of research published in Bilingualism: Language and Cognition from 1998–2011 where language proficiency was assessed as an independent or moderating factor. He found that although there was an increasing trend to measure language proficiency, there was limited empirical research reporting the use of objective tests to do so.
Similarly, Li et al. (Reference Li, Sepanski and Zhao2006) found that participant self-assessments were widely used in bilingualism research (e.g., self-ratings on Marian et al.’s Reference Marian, Blumenfeld and Kaushanskaya2007 LEAP-Q), yet such measures are difficult to generalize due to differences in scale use within and across populations (Tomoschuk et al., Reference Tomoschuk, Ferreira and Gollan2019) and may be poor reflections of actual proficiency levels (Ross, Reference Ross1998). As observed through these comprehensive syntheses, although language proficiency has played a major role in bi/multilingualism research, only limited attention has been given to the definition of its constructs, the design of reliable tools for measuring them, and the validation of such tools.
Encouragingly, researchers have undertaken efforts to more consistently use, and when necessary develop, standardized yet low-cost and practical measures of language proficiency. Examples include cloze tests (e.g., Brown, Reference Brown1980; Brown & Grüter, Reference Brown and Grüter2020; Tremblay, Reference Tremblay2011), C-tests (e.g., Norris, Reference Norris and Norris2018), picture naming tests (e.g., Luk & Bialystok, Reference Luk and Bialystok2013), and elicited imitation tests (EIT, e.g., Ortega et al., Reference Ortega, Iwashita, Norris and Rabie2002; Wu et al., Reference Wu, Tio and Ortegain press). Such proficiency measures offer many advantages to researchers. They all tend to be resource-friendly (in terms of time to administer and being low/no cost), and researchers have choices in terms of modality they wish to focus on (e.g., an oral/aural measure like an EIT can be used in research concerned with spoken syntax). Even more, similar, if not parallel, versions of these proficiency measures can be found across many languages (e.g., EITs adapted from Ortega et al., Reference Ortega, Iwashita, Norris and Rabie2002 or C-tests following a similar format described in Norris, Reference Norris and Norris2018).
In many ways, the widespread use of any standardized proficiency measure is a clear improvement over researcher impressions, institutional placements, or participant self-assessments. Nonetheless, standardized proficiency measures still warrant scrutiny lest researchers succumb to a “measurement schmeasurement” attitude (Flake & Fried, Reference Flake and Fried2020) where any instrument is seen as good enough, especially if it (a) was used in at least one other study and in turn (b) has a minimally acceptable reliability estimate that can be cited. Rather, it is ideal for researchers to use standardized measures that have convincing validity evidence to support interpretations of participant proficiency (Chapelle, Reference Chapelle, Winke and Brunfaut2021). Such evidence must go beyond reliability of scores, which is a necessary but not sufficient aspect of validity (McKay & Plonsky, Reference McKay, Plonsky, Winke and Brunfaut2021).
Given the comprehensive nature of validation research, it is not feasible for substantive research studies to feature and report such a process. Instead, methodologically focused studies dedicated to validation are needed to probe the quality of proficiency measures used in research. Accordingly, in this study we investigate the validity of the Korean EIT developed by Kim et al. (Reference Kim, Tracy-Ventura and Jung2016) following the same specifications as EITs for several other languages (which we refer to as the “Ortega et al. design”; Ortega et al., Reference Ortega, Iwashita, Norris and Rabie2002). In what follows, we review the current state of validity evidence in support of these EITs with a focus on the Korean EIT and highlight how additional analyses and broader sampling of participants is needed to increase confidence in the interpretation of proficiency scores across research studies.
VALIDITY OF EIT SCORES
EITs, also referred to as sentence repetition tests, are a type of shortcut measure (i.e., they require less time and fewer resources than other standardized proficiency tests, such as the TOEFL) that provide a reliable estimate of learners’ general oral language proficiency (e.g., Jessop et al., Reference Jessop, Suzuki and Tomita2007; Kostromitina & Plonsky, Reference Kostromitina and Plonskyin press; Vinther, Reference Vinther2002; Wu & Ortega, Reference Wu and Ortega2013; Wu et al., Reference Wu, Tio and Ortegain press; Yan et al., Reference Yan, Maeda, Lv and Ginther2016). In these tests, learners are required to listen to a series of sentences with either varying lengths or a certain constant length, and then repeat each sentence as closely as possible to the original stimulus. EITs require learners to process the stimuli, i.e., decode and understand the overall meaning of the sentences, to be able to reconstruct and repeat them. Therefore, the successful completion of the task draws on what Hulstijn (Reference Hulstijn2011) describes as basic language cognition, i.e., efficiently accessed phonological, morphosyntactic, and lexical knowledge (Erlam, Reference Erlam2006; Wu & Ortega, Reference Wu and Ortega2013; Zhou, Reference Zhou2012). EIT sentences are designed to be of lengths which exceed the general working memory capacity of learners, thereby making the processing of meaning and form more crucial for successful reconstruction, and delays between stimulus presentation and responses are also added to further this design goal.
The EITs following Ortega et al.’s (Reference Ortega, Iwashita, Norris and Rabie2002) design are used by the SLA research community to measure learners’ general oral language proficiency for research that involves proficiency as a screening criterion or a variable. These EITs have been particularly useful as they have allowed for some comparability of results across studies within and across various L2s thanks to the creation of parallel versions in different languages including Spanish (Bowden, Reference Bowden2016; Solon et al., Reference Solon, Park, Henderson and Dehghan-Chaleshtori2019), German, Japanese, English (Ortega et al., Reference Ortega, Iwashita, Norris and Rabie2002), French (Tracy-Ventura et al., Reference Tracy-Ventura, McManus, Norris, Ortega, Leclercq, Hilton and Edmonds2014), Mandarin (McManus & Liu, Reference McManus and Liu2020; Wu & Ortega, Reference Wu and Ortega2013; Zhou & Wu, Reference Zhou and Wu2009), Russian (Drackert, Reference Drackert2015), and Korean (Kim et al., Reference Kim, Tracy-Ventura and Jung2016). Generally, these EITs have been found to have high levels of reliability and strong, positive correlations with more spontaneous, meaning-focused speaking performances.
However, the validity of EIT scores (or any other measure used in bilingualism research) cannot be boiled down to a reliability coefficient and a correlation with a criterion measure (Chapelle, Reference Chapelle, Winke and Brunfaut2021; Drackert, Reference Drackert2015; Kane, Reference Kane2013; Révész & Brunfaut, Reference Révész, Brunfaut, Winke and Brunfaut2021). In his influential framework, Kane (Reference Kane2013) suggests that validity can be established by examining the proposed interpretations and uses of test scores, specified through “a network of inferences and assumptions leading from the test performances to the conclusions to be drawn” (p. 8). Kane’s approach to validity is elaborate and intended to be as comprehensive as possible, but we limit our focus here to three inferences, or aspects, of validity: generalization, explanation, and scale-based interpretation. These aspects are also discussed at length in the Standards for Educational and Psychological Testing (American Educational Research Association et al., 2014), another well-regarded authority on validity.
GENERALIZATION
Generalization is related to the consistency of scores across test occasions and conditions. This aspect of validity is most often investigated through reliability studies (e.g., coefficient alpha), and the various versions of the Ortega et al. EIT appear to have high internal consistency reliability (e.g., α = .96 for the Korean version in Kim et al. Reference Kim, Tracy-Ventura and Jung2016). Although the EIT is an independent and standardized measure of oral proficiency, it is also a rater-mediated form of assessment (Knoch & Chapelle, Reference Knoch and Chapelle2018) whereby scores and subsequent interpretations are subject to variability among the human raters who evaluate the EIT responses.
Surprisingly, research on EITs has not seriously reckoned with rater variability; previous studies have either reported simple agreement between two raters (e.g., Kim et al., Reference Kim, Tracy-Ventura and Jung2016; McManus & Liu, Reference McManus and Liu2020; Wu & Ortega, Reference Wu and Ortega2013) or neglected to report inter-rater reliability estimates (e.g., Bowden, Reference Bowden2016) and subsequently resolve scoring discrepancies by consensus or simply taking the scores of one rater. However, as each study that uses an EIT is likely to draw on different human raters, investigating effects that individual raters might have is of considerable importance to the generalization of EIT scores (see also Standards 2.1, 2.2, 2.6, 2.7, American Educational Research Association et al., 2014), especially given that one purpose of the EIT is to facilitate comparisons of participants across studies.
EXPLANATION
The link between test scores and theoretical constructs is central to what Kane (Reference Kane2013) refers to as explanation. Test scores, and differences in scores, should be explainable by differences in standing on the construct being measured (see also Standard 1.16 in American Educational Research Association et al., 2014). Conversely, test scores should not be attributable to construct-irrelevant factors. One of the most popular ways in which researchers have examined the explanation of EIT scores is through correlations with criterion measures, which has led researchers to conclude that the EIT measures a construct that draws on largely automatized language knowledge that overlaps considerably with the construct measured in oral proficiency interviews (e.g., Bowden, Reference Bowden2016; Drackert, Reference Drackert2015; Ortega, Reference Ortega2000). In addition, substantial shared variance has been observed between EIT scores and complexity/accuracy/fluency measures of learners’ spontaneous speech, such as number of clauses, number of error free clauses, and speech rate, among others, suggesting that stronger performances on EITs are closely related to learners’ general ability to adroitly use language for communication (e.g., Burger & Chrétien, Reference Burger and Chrétien2001; Kim et al., Reference Kim, Tracy-Ventura and Jung2016; Park et al., Reference Park, Solon, Henderson and Dehghan-Chaleshtori2020; Tracy-Ventura et al., Reference Tracy-Ventura, McManus, Norris, Ortega, Leclercq, Hilton and Edmonds2014; Wu & Ortega, Reference Wu and Ortega2013).
Some validation efforts have focused on construct-irrelevant variance in EIT scores, that is, probing the extent to which nontarget factors influence scores. For example, Kim et al. (Reference Kim, Tracy-Ventura and Jung2016) examined the relationship between working memory and Korean EIT scores, finding a small (r = .30), nonsignificant correlation and concluding that EIT scores are little influenced by working memory capacity. Similarly, Park et al. (Reference Park, Solon, Henderson and Dehghan-Chaleshtori2020) found that phonological short-term memory (PSTM) capacity is not a main predictor of the Spanish EIT performance. They noted, however, that learners’ language experience level might be a moderating factor on EIT performance, as they found that PSTM played a significant role in EIT performance for low language experience learners.
While existing validation research provides considerable support for the interpretation of EIT scores as measures of general oral proficiency, some important gaps remain. First, it has yet to be confirmed that general oral proficiency explains EIT scores in a similar manner across subgroups relevant to research, e.g., heritage and foreign language learners (Gómez-Benito et al., Reference Gómez-Benito, Sireci, Padilla, Hidalgo and Benítez2018; Zumbo, Reference Zumbo2007). Samples in previous EIT validation studies have tended to be homogenous, making it difficult to ascertain how appropriately EIT scores might be used and compared across subgroups of learners relevant to common research settings. Early work on the Chinese EIT was promising, with Wu and Ortega’s (Reference Wu and Ortega2013) report featuring a sample (n = 80) larger than many later studies and inclusive of two distinct learner groups: foreign and heritage language learners (40 each). This diversity of learner groups was not reflected in McManus and Liu’s (Reference McManus and Liu2020) recent replication of Wu and Ortega (Reference Wu and Ortega2013), which only recruited 65 foreign language learners.
Turning to the Spanish EIT, Bowden (Reference Bowden2016) included only 37 foreign language learners and Solon et al. (Reference Solon, Park, Henderson and Dehghan-Chaleshtori2019) involved 88 foreign language learners. The French EIT was investigated by Tracy-Ventura et al. (Reference Tracy-Ventura, McManus, Norris, Ortega, Leclercq, Hilton and Edmonds2014), who drew on a sample of 29 learners, of which just two were heritage learners and the remainder were foreign language learners. Across these studies, most participants were recruited in the United States and had English as their L1. Most relevant to the present study, Kim et al.’s (Reference Kim, Tracy-Ventura and Jung2016) investigation of the Korean EIT featured 66 second language learners (L1 Chinese = 33, L1 Vietnamese = 33) in South Korea, with no foreign language or heritage learners included.
Second, while strong connections have been established between Ortega et al. EIT scores and linguistic characteristics of non-EIT spoken performances, connections between the linguistic features of EIT items and EIT performances are less well established. In other words, there is little evidence that the content of the test itself is meaningfully related to the ability being measured. In Kim et al. (Reference Kim, Tracy-Ventura and Jung2016) the only significant predictor of item difficulty (i.e., lower item scores) was the number of syllables in the item (Wu & Ortega, Reference Wu and Ortega2013, yielded similar findings for the Chinese EIT). While the syllable is indeed a relevant linguistic measure, it is somewhat of an unsatisfying one, as syllables provide little indication of the internal complexity of a sentence. Consider the following 16 syllable examples in Korean:
-
1. 저희 어머니가 슈퍼마켓에 가셨습니다.
[our(GEN) mother-NOM supermarket-TO go-HON-PST-DEC(deferential)]
“Our mother went to the supermarket.”
-
2. 주택의 가격이 저렴해지기를 원한다.
[Ø(NOM) [residence-GEN price-NOM affordable-PASS-NOMINALIZER]-ACC desire-PRS-DEC(plain)]
“I hope the cost of housing becomes affordable.”
Despite having the same number of syllables, these sentences have some noticeable internal differences in terms of other linguistic features that are likely to present difficulties to learners (Lee et al., Reference Lee, Moon and Long2009). Example 1, which is not part of the Korean EIT, has several high-frequency words (mother, supermarket) that are three and four syllables long, respectively. There are no embedded clauses. The sentence contains five inflectional morphemes and one of those (-습니다, formal indicative) is also three syllables long. In contrast, Example 2, an actual Korean EIT item, includes comparatively less frequent lexical items (residence, affordable, desire), an embedded clause (an object complement), a null subject, and seven inflectional morphemes. In other words, although it is easy to identify linguistic aspects of Example 2 that might create greater challenges for language learners, the current empirical findings related to what makes Korean EIT items difficult (i.e., Kim et al., Reference Kim, Tracy-Ventura and Jung2016) suggests that these two sentences would present similar levels of difficulty.
SCALE-BASED INTERPRETATION
A scale-based interpretation relates observed test scores to another scale and thereby augments the meaning of scores. On their own, scores from the EIT can be directly compared as quantities and support reasonable conclusions such as “Learner A (EIT score = 73) is more proficient in general oral language than Learner B (EIT score = 54)”. Such comparisons can be quite useful within a study for grouping participants or controlling for relative proficiency levels, and also for comparisons of samples across studies. However, scores can be made more meaningful within and across studies by relating them to a larger norming sample drawn from relevant populations. A norm-referenced scale allows for broader inferences about EIT scores, such as “Learner C’s general oral proficiency is equal to or greater than 71% of learners” and “Learners from Study X could be considered low proficiency, with EIT scores placing them all in the bottom 25% of learners.” Examining another widely used proficiency measure, Brown and Grüter (Reference Brown and Grüter2020) analyzed a large, combined dataset (n = 1,724 learners, including substantial numbers of various L1 groups) of the Brown (Reference Brown1980) English cloze test and were able to provide a reference for score interpretations in terms of percentile ranks. This work allows researchers to locate their participants’ proficiency in reference to a larger, diverse population, increasing the meaningfulness and utility of the instrument.
Previous studies of the Ortega et al. EITs have featured samples generally on the smaller side and thus provide only minimally useful points of reference for other researchers to interpret EIT scores. Essentially, support for valid, broader interpretations of EIT scores across studies is largely absent. An analysis of EIT score data from a larger number of diverse learners would be needed to establish a preliminary scale-based interpretation for scores.
CURRENT STUDY
In sum, research suggests that EITs, particularly those following the Ortega et al. design, provide a reliable measure of general oral proficiency as they (a) show high internal consistency, (b) correlate highly with other proficiency measures (e.g., SOPI, DELE), and (c) are not substantially contaminated by construct-irrelevant factors, such as working memory. However, several gaps in empirical evidence to support the interpretations of EIT scores within and across studies remain. The current study aimed to further probe support for the validity of EIT scores by (a) considering effects of different raters, (b) examining potential differences in the measurement of relevant subgroups of language learners/users, (c) better understanding how linguistic features of items contribute to difficulty, and (d) providing a table of score percentiles that will facilitate comparisons across studies. The following research questions guided this study, with aspects of validity (inferences in Kane’s framework) indicated in parentheses:
RQ1. How reliably can different raters score the EIT? (generalization)
RQ2. To what extent do EIT items differ in the measurement of different kinds of learners (i.e., second language, foreign language, and heritage language learners)? (explanation)
RQ3. How do linguistic features influence the difficulty of EIT items? (explanation)
RQ4. How might EIT scores be appropriately interpreted across studies? (scale-based)
METHODS
The present study is an integrative data analysis of the measurement properties of the Korean EIT (Kim et al., Reference Kim, Tracy-Ventura and Jung2016). We combined data from three independent samples: Isbell’s Pilot (N = 27), Isbell’s dissertation (N = 198; Isbell, Reference Isbell2019), and Son’s dissertation (N = 93; Son, Reference Son2018). This enabled the application of more robust analytic techniques to usefully probe the measurement properties of EIT scores across a larger, heterogenous sample of L2 learners (Hussong et al., Reference Hussong, Curran and Bauer2013). Data and analysis scripts for this study are openly available at https://osf.io/h57e8/.
INSTRUMENT
All data in this study were elicited using the Korean EIT developed by Kim and colleagues (Reference Kim, Tracy-Ventura and Jung2016). This EIT was one of the iterations of EITs originally developed by Ortega et al. (Reference Ortega, Iwashita, Norris and Rabie2002). Thus, the test was composed of 30 Korean sentences with increasing lengths (between 8 and 17 syllables) and difficulty levels (test materials available at https://www.iris-database.org/iris/app/home/detail?id=york%3a934325&ref=search). The scoring was rater-mediated and followed a 5-point scale, where 4 = perfect repetition without any discrepancy, 3 = accurate repetition of the content/meaning with some discrepancies in the form, 2 = repetition with discrepancies in form that affected content/meaning, 1 = repetition of only half of the stimulus or less, and 0 = no repetition or only one word repeated (see Kim et al., Reference Kim, Tracy-Ventura and Jung2016, p. 661).
For one of the datasets, collected in South Korea from learners of diverse first language (L1) backgrounds, Korean pretest directions and practice items were created. Additionally, a bilingual (Korean and English) version of the scoring rubric was presented to the rater for this dataset.
DATA
As mentioned, the data used in this study came from three independent samples. They feature Korean heritage language (HL) learners (primarily Son’s dissertation) in the United States, learners of Korean as a foreign language (FL) in the United States (Son’s dissertation, Isbell’s pilot), and learners of Korean as a second language (SL) located in Seoul, South Korea (Isbell’s dissertation). Native Speaker (NS) data collected by Isbell as a baseline are also referenced.
Isbell’s Pilot
Isbell’s pilot was a precursor to his dissertation. Twenty-seven learners at a large Midwestern US university completed the Korean EIT, which was scored by a NS Korean instructor (coded as R1 in the Results section) at the same university. Seven NS Korean graduate students also completed the EIT. Isbell (coded as R2), a nonnative SL speaker, scored a subset of these data as part of a routine inter-rater reliability analysis.
Isbell’s Dissertation
Isbell’s dissertation data were collected in Seoul, South Korea. Most of the 198 learners who completed the EIT were university students, enrolled in intensive Korean language programs, English-medium degree programs, or Korean-medium degree programs; some learners were in Korea for other purposes (residence, work, research). Six NSs also completed the EIT. EIT data were scored by a NS Korean graduate student in applied linguistics (R3). Isbell scored a subset of 20 randomly selected EIT responses as part of routine reliability analysis.
Son’s Dissertation
Son collected EIT data from 93 learners at 10 universities throughout the United States. Among these learners, 41 were self-identified as ethnically heritage language learners and 52 were nonheritage KFL learners. Son (R4), a HL speaker of Korean, and a second rater, a NS Korean graduate student (R5), scored all data.
Integrated Sample Characteristics
When combined, data from these three studies included 318 Korean language learners. Three subgroups were identified as follows: HL learners were those who reported having a Korean-speaking parent and childhood exposure to the language, SL learners as those who were studying/living in Korea, and FL learners as those studying outside of Korea. Across the combined sample, the median order of acquisition/learning Korean was as a third language, with the notable exception of HLs where it was most commonly learned first. For speaker L1, defined here as self-reported most dominant language, the most common was reported as English (115, with three additional individuals indicating co-L1 status with Korean, Spanish, or Turkish) followed by any variety of Chinese (111, including Mandarin and Cantonese), Russian (19), Japanese (14), and Spanish (11). The remaining 48 participants represented 23 other L1s. Table 1 provides additional information on sample characteristics, with breakdowns included for the relevant learner subgroups. FLs represented a somewhat narrower age range and had substantially less experience compared with the other groups.
TABLE 1. Sample characteristics

Note. FL, foreign language learners; SL, second language learners; HL, heritage language learners. Discrepancies in the number of participants is due to missing background data.
Establishing Links in the Data
In an integrative data analysis, data from independent samples must be sufficiently linked. All links need not be direct (e.g., if X is linked to Y and Y is linked to Z, X and Z are linked indirectly). To achieve linkage in the present study, overlap among raters was necessary. To this end, in what Hussong et al. (Reference Hussong, Curran and Bauer2013) refer to as a bridging study, Son scored 20 learners from Isbell’s dissertation. Figure 1 illustrates how all three datasets and five raters are linked. A rater from Son’s dissertation, R5, is not directly linked (i.e., did not score the same learners) to R3, R2, or R1. However, R5 is directly linked to R4, who is in turn directly linked to R3 and R2; through R2 the links extend further to R1.
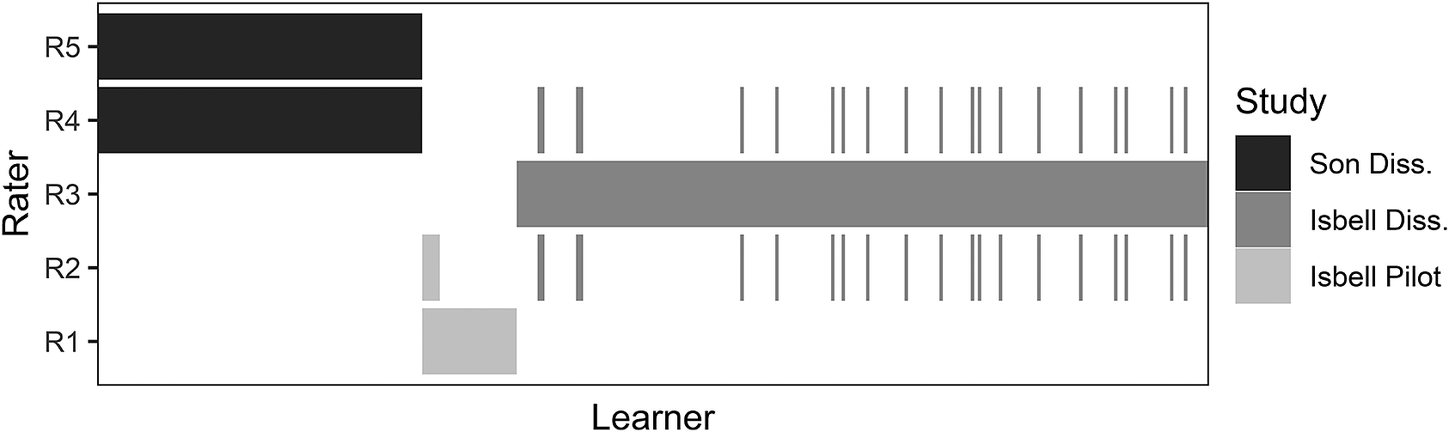
FIGURE 1. Data linkage across three studies.
ANALYSES
INTER-RATER RELIABILITY
Our first analyses investigated rater reliability and assessed the degree to which individual raters might influence EIT scores. From a classical test theory perspective, inter-rater reliability was considered by analyzing the subsets of overlapping score data. Inter-rater agreement for observed scores at the test level was examined by correlating observed total scores. Inter-rater agreement at the item level was analyzed through absolute agreement (%) and the intraclass correlation coefficient (ICC) between/among raters with the irr package (v. 0.84.1; Gamer et al., Reference Gamer, Lemon, Fellows and Singh2019) in R. Two-way ICCs based on absolute agreement were selected (Hallgren, Reference Hallgren2012; Shrout & Fleiss, Reference Shrout and Fleiss1979).
Differences among raters were also investigated through multi-facet Rasch measurement (Linacre, Reference Linacre1989) using the software Facets (v. 3.80.4; Linacre, Reference Linacre2018). We used a three-facet model, which included learners, EIT items, and raters. In this approach, scores from all raters are analyzed simultaneously, resulting in (a) estimates of person ability and item difficulty that control for rater differences, (b) estimates of each rater’s severity and consistency, and (c) statistical indices (separation and reliability) that characterize the degree of differences among several raters. Person ability, item difficulty, and rater severity are all expressed in log-odds units (logits). For elements in each facet, fit statistics are calculated based on model residuals, including outfit (sensitive to outliers) and infit (less sensitive to outliers). For raters, these fit statistics indicate the consistency of assigned scores with model expectations. For items, fit statistics indicate how well responses to the item fit the model. Values between 0.7 and 1.3 are generally desirable (Wright & Linacre, Reference Wright and Linacre1994). Finally, Rasch models (Rasch, Reference Rasch1960/1980) assume unidimensional measurement, i.e., that the test measures a single latent trait (Embretson & Reise, Reference Embretson and Reise2000). To investigate this assumption, we examined the distribution of standardized residuals across all item scores and conducted a principal components analysis (PCA) of model residuals (Fan & Bond, Reference Fan, Bond, Aryadoust and Raquel2019; Linacre, Reference Linacre2020b).
MEASUREMENT CHARACTERISTICS AND DIF
Our next set of analyses were conducted to identify possible differences in measurement among three subgroups commonly featured in bilingual research: second language (immersed) learners, foreign language (nonimmersed) learners, and heritage language learners. Because our analyses of the impact of different raters on measurement found minimal effects (see the Results section), we could proceed with a simpler two facet (persons and items) Rating Scale Model (RSM; Andrich, Reference Andrich1978) in Winsteps (v. 4.5.2; Linacre, Reference Linacre2020a) where one set of item scores for each participant was included, ignoring the trivial differences among raters. We report the measurement characteristics of this two-facet model in detail, and then report on a differential item function (DIF) analysis. In a typical DIF analysis, results of one subgroup are compared with another subgroup that serves as the reference or baseline group. With two subgroups, this is a straightforward matter. When there are more than two subgroups of interest, analysts are faced with many more potential pairwise comparisons (increasing the risk of Type I errors) and must choose a practical baseline. In our DIF analysis, rather than arbitrarily select a reference group (e.g., SL learners due to being the largest group), we chose to compare estimates of item difficulty for the entire sample with those of each subgroup (Linacre, Reference Linacre2020b). As DIF analyses are sensitive to sample size, this approach has the benefit of a more stable baseline item difficulty estimate. At the same time, this approach will tend to be less sensitive, as each subgroup influences the mean of the baselineFootnote 1 . Items showing bias toward one or more groups were flagged based on the criteria of magnitude (0.5 logits or greater, following Draba, Reference Draba1977; Wright & Douglas, Reference Wright and Douglas1975) and statistical significance (i.e., t > |2.0|). Total impacts on measurement were estimated by summing DIF values across all flagged items.
EXPLANATORY ITEM RESPONSE MODELING
Our final set of analyses sought to explain the difficulty of EIT items in terms of their linguistic features. We first replicated Kim et al.’s (Reference Kim, Tracy-Ventura and Jung2016) linear regression of observed EIT item averages onto number of syllables, a vocabulary score based on the NIKL list of Korean vocabulary for basic learners, and number of embedded clauses. Then, to take full advantage of item-level response data and explore accounts of item difficulty potentially more satisfying than number of syllables (Kim et al.’s only significant predictor), we took an explanatory item response modeling (EIRM) approach (De Boeck & Wilson, Reference De Boeck and Wilson2004) and constructed several linear rating scale models (LRSMs) using the eirm package (Bulut, Reference Bulut2021) in R. LRSMs involve the decomposition of item difficulty based on relevant characteristics of items. Such analyses can provide evidence that supports the explanation of test scores when theoretically relevant features of test items substantially account for variation in item scores. Our initial LRSM was based on Kim et al.’s (Reference Kim, Tracy-Ventura and Jung2016) regression of observed item averages on the number of syllables, vocabulary score, and number of embedded clauses in each sentence. We then added two finer-grained linguistic predictors: number of content morphemes and number of function morphemes. In an iterative model building process, we then removed nonsignificant predictors to arrive at a more parsimonious final model. All models were evaluated by using parameter estimates to predict item difficulties for all 30 items; these predicted difficulties were then correlated with the empirical estimates of difficulty from a descriptive (no item predictor) RSM estimated in eirm.
LINGUISTIC ANALYSIS OF EIT ITEMS
Some elaboration on linguistic coding of EIT items for the EIRM analyses is warranted. All 30 items were analyzed in terms of syntactic and lexical structure. For syntactic structure, the sentences were manually coded for number of clauses and number of embedded clauses. In terms of lexical structure, words in each sentence were coded for number of syllables and morphemes. Morphemes were first categorized as content and function morphemes. Following Lee et al. (Reference Lee, Madigan and Park2016), nouns, bound roots, verb or adjective stems, and derivational affixes were coded as content morphemes, and pronouns, numerals, conjunctions, postpositions, and inflectional affixes were coded as function morphemes (p. 78). Moreover, as in Kim et al. (Reference Kim, Tracy-Ventura and Jung2016), all content words were coded based on their vocabulary level (based on corpus-referenced frequency and presentation in instructional materials) as classified by the National Institute of the Korean Language (NIKL, 2003) vocabulary list for Korean learners to gauge the vocabulary difficulty level of each sentence.
RESULTS
RQ1: RATER RELIABILITY
Inter-rater reliability analyses based on the subsets of overlapping rater data suggested high levels of rater agreement, which provides evidence for the generalization of scores (i.e., consistency of scores across different raters). For Son’s dissertation, the correlation between the two raters’ total scores (i.e., in a scale of 120) for 93 learners was r = .99 with exact agreement of 10%, adjacent (within 1) agreement of 31%, and 80% of all total scores within five points. For Isbell’s dissertation, r values of .97–1.00 were obtained (3 raters; 20 learners) for the various rater pairings with exact agreement rates of 5% to 20%, adjacent agreement rates of 15% to 40%, and 65% to 90% of total scores within 5 points. The correlation for the two raters in Isbell’s pilot was r = .99 (n = 5), with 0% exact agreement, 40% adjacent agreement, and 80% were within 5 points. At the item level, agreement was generally high (Online Supplementary Table S1), with average ICCs ≥ .75 (Hallgren, Reference Hallgren2012) and exact agreement rates ranging from 73% to 87% for Son’s dissertation, 40% to 75% for Isbell’s dissertation, and 40% to 100% for Isbell’s pilot.
Many-Facet Rasch Measurement analysis allowed for the combining of data from all three studies, facilitating further analysis of rater-related variability in scores. We constructed a three-facet rating scale model (RSM) which included person, item, and rater facets (Table S2 and Figure S1 in the Online Supplementary Materials). In a RSM estimated in Facets, item difficulty measures correspond to the logit-scaled location in which the highest and lowest response categories are equally likely, with thresholds for higher and lower scores relative to that overall difficulty. Based on 13,678 scores given to EIT item responses across all raters, the three-facet RSM parameters accounted for 72.4% of observed variance in item responses. Looking closer at the measurement characteristics of each rater (Table 2), it was apparent that there were minimal differences in rater severity and that raters demonstrated adequate consistency in scoring, according to fit indices. The rating scale appeared to function well, with all score categories in the expected order and distinct peaks visible for each (Figure S2 in the Online Supplementary Materials). Category statistics (Table S3 in the Online Supplementary Materials) largely confirmed this observation, with monotonically increasing average measures and difficulty thresholds (Linacre, Reference Linacre2020b); somewhat poor outfit (1.7) was found for the score point of 2. A supplementary three-facet hybrid model, in which rating scale category thresholds were held constant across all items (like a typical RSM) but estimated separately for each rater, was also run (Figure S3 in the Online Supplementary Materials). This model showed that each rater generally used the scale in a similar manner, with similar threshold locations among raters.
TABLE 2. Rater measurement characteristics in the three-facet RSM

Note. Rater facet separation strata = 2.32, separation reliability = 0.84. Fixed χ2 (4) = 32.2, p < .01.
a Fair Average represents the expected score a rater would assign to a response from an examinee of average ability to an item of average difficulty.
b Severity reported in logits.
While a χ2 test suggested that the null hypothesis of raters having no difference in severity should be rejected, this is largely expected due to the large number of scores assigned and related precision of severity estimates. Linacre (Reference Linacre2020b) emphasizes the importance of magnitude in interpreting measures in Rasch analyses, and here we can see that in terms of raw scores assigned to average examinees responding to an item of average difficulty (the “Fair Average”), the most lenient rater and most severe rater differ by only 0.22 points on the five-point (0-4) EIT rating scale. Both of their Fair Average scores would round down to 2. Moreover, a difference of .21 logits corresponds to a fraction of the distance between pairs of Rasch-Andrich thresholds on the EIT’s five-point scale (see Figure S1 in the Online Supplementary Materials). In sum, severity differences among the five raters would infrequently be expected to result in item scores that differed by even one point.
RQ2: DIFFERENTIAL TEST/ITEM FUNCTIONING
With the high levels of inter-rater reliability and minimal differences in rater severity observed in the three-facet model, we decided to treat raters as interchangeable and proceed with further analyses using only one score per learner per item, allowing for more straightforward analyses and interpretations related to our research questions. Specifically, we included the scores from R1 for Isbell’s pilot, R3 for Isbell’s dissertation, and R4 for Son’s dissertation. Across all 318 learners, the mean EIT score was 61.26 (SD = 30.54; range = 0–117). FLs had a mean of 34.01 (n = 78; SD = 27.01; range = 0–105), SLs had a mean of 74.96 (n = 195; SD = 24.44; range = 9–116), and HLs had a mean of 68.99 (n = 45; SD = 28.54; range = 12–117).
We then constructed a two-facet RSM (Table S4 in the Online Supplementary Materials). Two items demonstrated misfit at a level potentially deleterious to measurement (Wright & Linacre, Reference Wright and Linacre1994): Item 1 (infit = 2.03; outfit = 4.23) and Item 2 (infit = 1.81; outfit = 2.09). The Rasch reliability of person measures, was 0.96 and Cronbach’s alpha was .98. Based on 9,539 scores (one person had one missing item response) given to test-takers across all EIT items, the two-facet RSM parameters (person ability and item difficulty) accounted for 71.2% of observed variance in item responses. The abilities of test takers and difficulties of EIT items are compared graphically in a Wright map (Figure 2). As shown on the Wright map, FL learners generally had lower abilities than the other two groups, a finding in line with expectations related to the more extensive Korean experience of HL and SL learners.

FIGURE 2. Wright person-item map illustrating the distribution of person ability and item difficulty. Higher ability persons and more difficult items are located higher on the vertical MEASURE (logit) scale. H = heritage language learner, F = nonheritage foreign language learner, and S = nonheritage second language learner. Means (μ) and standard deviation units (σ) are marked on the dividing line.
We also examined the degree to which our data fit the Rasch model and the assumption of unidimensionality. Out of 9,539 responses, 441 (4.6%, less than an expected 5.0%) had standardized residuals (Z) greater than or equal to |2.0| and 116 (1.2%, above yet near an expected 1.0%) were equal to or exceeded |3.0|, indicating acceptable model fit (Linacre, Reference Linacre2020b). A PCA of RSM residuals revealed one contrast with an eigenvalue greater than 2.0 (2.39) that accounted for 2.3% of the observed score variance, a small amount compared with variance explained by Rasch measures. Nonetheless, we probed the extent to which a secondary dimension might impact measurement further. Inspection of a scree plot of the first five contrasts did not reveal a defined elbow, nor did there appear to be any concerning patterning within the first contrast in terms of loadings. Furthermore, the disattenuated correlation between person measures calibrated based on positively and negatively loading items in this cluster was 0.96, which is close to an ideal value of 1.0 (Linacre, Reference Linacre2020b). Thus, it appeared that the assumption of unidimensionality was satisfied.
We then examined the consistency of measurement across three groups in a DIF analysis. Figure 3 shows the relationships of item difficulty estimates between three subgroups of Korean learners: HL learners, SL learners, and FL learners. The correlations of item difficulty estimates between groups were all at least .9, indicating high levels of measurement consistency across groups. In other words, differences among learner groups did not result in substantially different hierarchies of item difficulties: Items that were easier for one group tended to be easier for the others, and vice-versa. These results also provided evidence to support the explanation of EIT scores, as general oral proficiency of different subgroups of language learners is measured in a similar manner.

FIGURE 3. Scatterplots comparing item difficulty estimates among learner subgroups. The gray dashed line represents an ideal identity relationship.
Looking closer at differences in measurement at the level of individual items (Figure 4), we found that five items showed substantial (i.e., > |.5| logits, Bond & Fox, Reference Bond and Fox2015; Linacre, Reference Linacre2020a) and statistically significant DIF compared with whole group item difficulty estimates (Table 3). Positive DIF values indicate greater item difficulty for a subgroup, while negative values indicate the item was easier. The t statistic associated with each item DIF estimate indicates how likely the DIF value is if one hypothesizes that the subgroup item difficulty estimate is no different than the whole-group difficulty estimate, with values greater than |2.0| interpreted as statistically significant.

FIGURE 4. Item averages (top) and Rasch difficulty (bottom) for all learners and each learner group separately.
TABLE 3. EIT items flagged for substantial DIF for at least one subgroup

Note. DIF, difference in logit measure between subgroup from whole-group item difficulty estimate. DIF estimates larger than .5 logits bolded.
* indicates t-values greater than |2.0|.
Four out of the five items demonstrated DIF for HLs with an even split of unexpectedly easier and harder items; one item was easier than expected for FLs. Item 1 (see materials on IRIS database; Kim et al., Reference Kim, Tracy-Ventura and Jung2016), which was difficult for HLs, also had poor fit for the whole group. It may have been the case that generally high-ability HLs would occasionally stumble on the first EIT item, resulting in substantial DIF. We also found that one word in the audio stimulus for this item, 갂아야 (kakkaya, /kɑk*ɑjɑ/, “have to trim”) could be easily confused for 닦아야 (takkaya, [tɑk*ɑjɑ], “have to wash”); repeating 닦아야 would result in a score of two on the item (due to a change in meaning of the sentence) and this may have made the item unexpectedly difficult for heritage language learners who otherwise would have been expected to perform well. It is more difficult to speculate on reasons for DIF seen in other items. Item 10 was one of the easier items overall for all groups, but HLs may have done exceptionally well due to the presence of a subject marker, a difficult-to-master morphosyntactic feature for late-learners, which allowed for earning more scores of 4 on the item.
In contrast, Item 19 was one of the more difficult items overall for all groups, and it may have been scoring criteria which allowed FLs to (unexpectedly, according to the model) salvage a point or two by being able to execute a partial repetition of the sentence. Items 25 and 27 each featured a relatively rare Sino-Korean lexical item (체포하다 “to arrest” and 증가하다 “to increase”, respectively) which are more commonly used in formal contexts (e.g., news broadcasts, written language) and have synonyms more common in casual conversation (e.g., 잡다 “to grab” or “to catch” and 늘다 “to increase”). These words may have been less familiar to HLs exposed to more social/interpersonal Korean input and as such may have underperformed expectations on these items relative to their overall abilities. Conversely, these words are commonly taught (or at least encountered) in the intermediate and upper levels of Korean language programs.
Ideally, tests have no items which differ in how they measure examinee abilities, and as such any evidence of DIF is worthy of consideration. That said, sometimes DIF may reflect real differences in the abilities of subgroups, or it may not cause substantial disturbances of measurement for subgroups at the test level. By summing the DIF size estimates across items for each group, the total impact of DIF on total ability estimates at the test level can be considered. Shown at the bottom of Table 3, these cumulative DIF totals were calculated for (a) the five flagged items as well as (b) for all 30 items of the EIT regardless of DIF size and statistical significance. In both cases, these cumulative DIF size estimates are small and would be expected to have negligible impact on total EIT scores. For example, the cumulative DIF size across the five flagged items for HL learners is 0.16 logits, meaning that the set of flagged items was more difficult than expected. Following Linacre (Reference Linacre2020b), as all EIT items are weighted equally, cumulative DIF for each group can be divided by the length of the test (30 items) to arrive at an estimated impact on measurement of person ability at the test level. For heritage learners, this calculation based on cumulative DIF of flagged items (0.16/30) results in a 0.005 logit underestimation of ability. On the metric of raw EIT scores, this amounts to a small fraction of a single point.
In sum, while several items seemed to function differentially, being easier or harder for one of the three learner subgroups, these differences were largely balanced out at the test level by DIF in the opposite direction on a different item. The impact of DIF on the EIT’s overall measurement of person ability appeared to be largely negligible.
RQ3: EXPLANATORY ITEM RESPONSE MODELING
With support for measurement invariance/lack of bias across learner subgroups established, we turned to investigating what caused items to be more or less difficult across all learners in the integrated data set. As an initial step, we replicated Kim et al.’s (Reference Kim, Tracy-Ventura and Jung2016) linear regression of average item scores on three linguistic predictors using the linguistic coding provided by the authors of that study (Table S5 in the Online Supplementary Materials). While the model provided a statistically superior fit to the data compared with an intercept-only (null) model, we did not find any of the individual predictors to be statistically significant, in contrast with Kim et al. (Reference Kim, Tracy-Ventura and Jung2016), which found the number of syllables to be a statistically significant predictor. However, the total variance in item difficulties explained by the model (adjusted R2 = 0.47) was similar to Kim et al., who reported an R2 of 0.46.
One shortcoming of running a regression on average item scores is the massive loss of information from the individual item responses—thousands of individual responses are reduced to an effective n-size of 30, with accordingly low power and precision. We turned to an EIRM approach, namely the linear rating scale model (LRSM), to more rigorously investigate the degree to which linguistic characteristics of EIT items account for differences in item difficulty (Table 4). We ran five different LRSM models, starting with variables included in Kim et al. (Reference Kim, Tracy-Ventura and Jung2016) and then adding in number of content and function morphemes. Then, we looked to prune variables that were not reliable predictors of item difficulty. To estimate the usefulness of each model, we ran correlations between predicted and empirical item difficulty estimates. The models with more variables tended to correlate slightly better. However, once the variable for syllable number was excluded, the model was able to better explain item difficulty. We chose the model LRSM 5 as our “best” model for its parsimony. Therefore, as seen in Table 4, vocabulary score and number of function morphemes were the variables that best explained item difficulty. We consider these results as more linguistically satisfying explanations for item difficulty than only the number of syllables. At the same time, as presented in Figure 5, we can see that predictions of item difficulty based on these linguistic features correlate substantially with observed measures of item difficulty, r = .77.
TABLE 4. Explanatory item response models for EIT item difficulties


FIGURE 5. Scatterplot showing descriptive item difficulty and predicted item difficulties based on LRSM. All item difficulty locations have been adjusted to the 0/1 threshold, in line with eirm package conventions. Solid line represents an ideal identity relationship.
RQ4: SCALE-BASED INTERPRETATION
To augment Korean EIT score interpretations across studies, we describe the distribution of EIT scores based on our sample of 318 learners in percentiles to serve as a point of reference (Table 5). We also consider the scores of 13 native speakers (NS) raised in South Korea. These NSs, 7 of whom were scored by R1 and 6 by R2, had a mean raw total score of 116.15 (SD = 3.63; min = 107; max =120; only one NS received a maximum score of 120). Thus, one could reasonably interpret EIT scores greater than 107 (96th percentile and greater) as “highly-advanced,” and potentially “near-native” (but see Solon et al., Reference Solon, Park, Henderson and Dehghan-Chaleshtori2019, for the potential need to add more difficult items to further discriminate among high performers). A broad-strokes characterization of the whole proficiency range may also be useful, with scores of 36 or below as “low” (the lowest quartile), 37 to 85 (middle two quartiles) as “intermediate”, and scores of 86 or above (highest quartile) as “high.”
Table 5. Percentile ranks for Korean EIT scores (abbreviated)
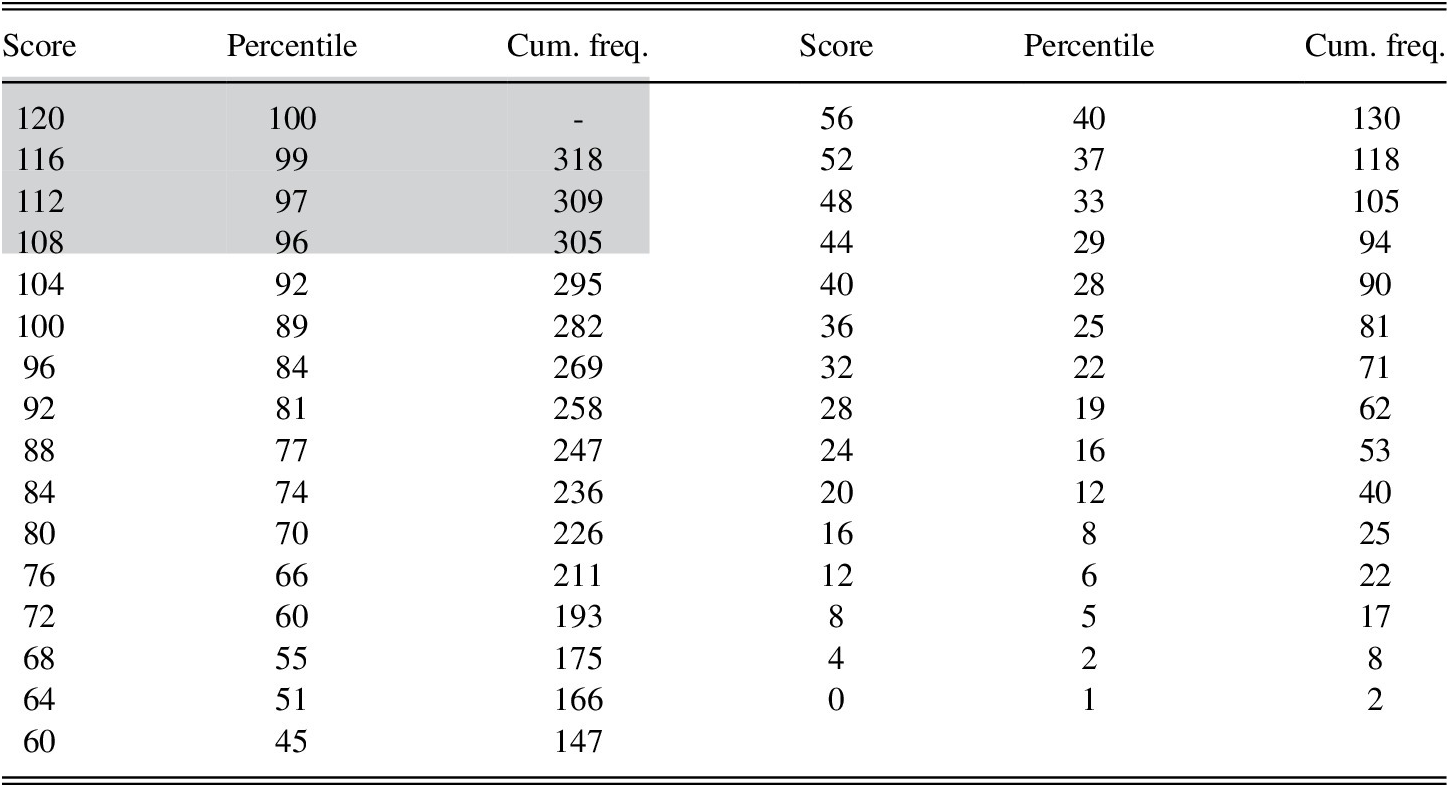
Note. Shaded region indicates range where NS scores were observed (NS scores not included in percentile ranks).
DISCUSSION
The purpose of the present study was to contribute to the extant validation research of EITs by examining evidence that support score interpretations when this instrument is used as a general oral proficiency measure in SLA/bilingualism research. In particular, we investigated the extent to which differences among raters and learners affected the measurement properties of the Korean EIT. We believe that, for an instrument to be most useful within the SLA/bilingualism research domains, it should be applicable in multiple contexts, that is, when being scored by different raters as well as when measuring diverse types of learners (i.e., second, foreign, and heritage language learners). Furthermore, this study explored which item characteristics could better explain item difficulty. Finally, by combining different datasets we were able to provide a point of reference for Korean EIT scores to be more easily interpretable across studies.
One valuable finding of this study was that the five raters showed very little difference in how they scored EIT responses, providing some initial support for the generalization of score interpretations across raters. While five is a relatively small number of raters and more work on scoring behavior would be welcome, we note that the five raters were relatively heterogenous: One was a nonnative speaker (adult learner), one was a bi/multilingual heritage speaker, and three were native speakers with differing amounts of experience with nonnative Korean speech. The present findings allowed us to treat raters as interchangeable in subsequent analyses, which suggests that (a) the EIT rubric supports consistent scoring and (b) Korean EIT results can be viewed as reasonably comparable across different studies and raters. Future validation research on Ortega et al. EITs would benefit greatly from the inclusion of more raters to further test the limits of generalization.
Although we found some instances of DIF, on a whole the EIT appeared to measure oral proficiency in much the same way across demographic groups relevant to SLA/bilingualism research, providing support for the substantive explanation of scores. In total, we found substantial and statistically significant DIF for 5 (out of 30) items, mostly related to HL learners. As discussed previously, we have some hunches about why these items may have functioned differently for the different groups, but we are unable to offer any concrete explanations. Ultimately, however, the magnitude and direction of DIF was not so large and consistent, respectively, that overall measurement of oral proficiency was compromised, providing support for score interpretations across relevant subpopulations. This finding is especially encouraging, as it would support research that, for example, seeks to understand finer-grained differences in the grammars of heritage and nonheritage language learners who are of similar overall oral proficiency.
While there are many reasons to assume that the linguistic systems of heritage and nonheritage bilinguals differ in important ways (Kim et al., Reference Kim, Montrul and Yoon2009; Montrul, Reference Montrul2013; Montrul & Perpiñán, Reference Montrul and Perpiñán2011; Silva-Corvalán, Reference Silva-Corvalán2018), it would seem prudent to contrast groups that are broadly comparable in their ability to process oral language for meaning and form. Often it is the case, as it shown in the combined sample of the present study, that heritage learners appreciably exceed the ability of foreign language learners in their ability to process spoken language, making it potentially difficult to probe more specific differences in grammars and psycholinguistic processing on equal grounds, i.e., whether differences are due to lesser overall proficiency or qualitative differences in their grammars. Use of EIT scores allows for quantification of general proficiency in oral language, which can inform participant selection or matching and/or allow for statistical controls on analyses related to substantive interests as well as explore variation within learner categories (Hulstijn, Reference Hulstijn2012).
Explanatory response models provided some additional support for the construct validity, or explanation inference, of EIT scores. Two linguistic aspects of the items, vocabulary sophistication and number of inflectional morphemes, accounted for 59% (R2) of variance in observed item difficulties (i.e., predictions of item difficulties based on linguistic features correlated with actual observed item difficulties at r = .77). These two predictors were particularly effective in explaining item difficulty because (a) vocabulary sophistication accounts for frequency of use and thereby likelihood of exposure to and acquisition by learners and (b) functional morphemes, as opposed to content morphemes, are less salient in spoken Korean and are often able to be left out in conversation. Furthermore, inflectional morphemes (a type of functional morpheme) are challenging for learners even at advanced proficiency levels (Lee et al., Reference Lee, Moon and Long2009). We consider this an improvement on insights from prior research, which found little statistical support for linguistic factors outside of sentence length in syllables (Kim et al., Reference Kim, Tracy-Ventura and Jung2016; see also Chinese EIT findings in McManus & Liu, Reference McManus and Liu2020; Wu & Ortega, Reference Wu and Ortega2013), despite factors such as vocabulary being identified by test-takers as a source of difficulty in EITs (Wu et al., Reference Wu, Tio and Ortegain press).
The present results, yielded by more robust analyses of item responses, provide a more detailed understanding of how linguistic features contribute to the difficulty of EIT items and, we argue, constitute stronger evidence that the test measures the intended construct. Connections can also be drawn between these findings and the EIT rating scale. For example, a response with accurate reconstruction of meaning that features a missing or malformed functional morpheme would be downgraded from a score of 4 to 3, and similarly, any mistakes or omissions of content words would likely change the meaning of the sentence, warranting a score of 2. And like a simple count of syllables, compounded errors or omissions of content words and functional morphemes could yield a score of 1 or 0.
These findings related to factors influencing item difficulty have implications for future EIT development efforts. If new items or forms for an existing EIT are generated, or an EIT is created for a different language, lexical sophistication and morphosyntactic features should be considered (Wu et al., Reference Wu, Tio and Ortegain press). Often, it has been the case that items for EITs in different languages are primarily generated based on translations from the original Spanish version of the EIT (Ortega et al., Reference Ortega, Iwashita, Norris and Rabie2002), but the linguistic properties of translated items may need adjustments to create similar levels of difficulty. Relatedly, although syntactic complexity (in terms of the number of embedded clauses) was not identified as a systematic source of item difficulty, it nonetheless appeared that some of the most difficult items featured more embedded clauses (cf. Wu & Ortega, Reference Wu and Ortega2013, who arrived at similar conclusions). We suspect the lack of variation in the number of embedded clauses precluded statistically significant effects and speculate that syntactic complexity would be a more reliable predictor of difficulty in a larger universe of possible EIT items. For researchers who wish to create new versions of the EIT for other languages, keeping these points in mind will hopefully lead to high-quality measures that are beneficial to the internal and external validity of research.
We believe that the percentile references reported in the present study offer a useful point of reference for bilingualism researchers doing work with adult Korean speakers. While we recognize that these percentile rankings were derived from a sample that might fall short of standards for norming high-stakes psychological instruments or educational tests (e.g., to identify learning disabilities or afford admission to university, see American Educational Research Association et al., 2014), Kane (Reference Kane2013) reminds us that the rigor of validity evidence may be relaxed when the stakes of score use are lower. For the purposes of interpreting research across studies, we believe that the current percentile ranks are adequately supported: Across the 318 speakers from three separate studies, we were able to include a wide range of nonnative Korean oral proficiency levels of learners of diverse backgrounds and experiences. Some participants were adult learners without even a full semester of formal instruction under their belts while others had been living in Korea for several years to pursue Korean-medium graduate degrees. Participants also represent a diverse selection of L1s and include those from FL, SL, and heritage contexts.
Other researchers, through use of the Korean EIT, could make direct comparisons to our sample, using the data presented here as a norm reference. For example, a hypothetical instructional intervention study involving a sample of first-year university students of Korean as a foreign language whose EIT scores fell into the range of 4–36 (2nd–25th percentiles) could reasonably be characterized as low proficiency. Future research on the Ortega et al. design EITs for other languages that include norm-referenced percentile rankings is encouraged as a first step toward meaningful comparisons of EIT scores across languages.
Despite the generally positive evidence found for the validity of the EIT as a measure of oral proficiency suitable for several key populations, we discovered some aspects of the test which might be improved on. First, the first two items of the Korean EIT demonstrated poor fit to the Rasch model, and, furthermore, heritage language learners tended to perform more poorly than expected on the first item. It may be beneficial for the first item to be revised, though we have some reservations now that the current version of the Korean EIT has been used in several studies. Second, if researchers focus on solely on advanced-level learners, we agree with Solon et al.’s (Reference Solon, Park, Henderson and Dehghan-Chaleshtori2019) conclusion of their study on the Spanish EIT that it may be beneficial to develop more challenging items to better differentiate high ability speakers. While no learners in the present combined sample earned a maximal score of 120, several did score near it, similar to the NSs, which could signal a similar need for additional Korean items to further discriminate among very high ability speakers. Alternatively, the fact that all native speakers do not max out the scale and that some learners can reach similarly high levels as NSs may speak to the utility of the instrument in capturing a broad range of bilingual proficiency.
Last, on a methodological note, we found that integrative data analysis can be a useful approach for measurement analyses in bilingualism and second language research. In our case, we capitalized on laboriously collected data to shed new insights on the validity of a commonly used type of proficiency measure. With the larger and more diverse sample, we were able to conduct measurement analyses involving item response modeling that largely would not have been possible based on any one of our separate studies. We believe integrative data analysis may be a useful approach for other researchers to more rigorously validate instruments used in SLA research. While such work may be retrospective, the new evidence and insights can be drawn on to support future work in the field.
CONCLUSION
In this study, we examined the measurement qualities of the Korean EIT based on a modestly large integrated dataset from three studies. Our findings suggest that the Korean EIT can be scored reliably by different raters and that the general oral proficiency of relevant subgroups of adult Korean learners/speakers (i.e., foreign language, second language, and heritage language) is measured in a similar manner. Furthermore, we demonstrated that the difficulty of EIT items is based on factors related to linguistic sophistication and complexity, beyond sentence length, which aligns well with the EIT’s scoring rubric and supports the meaningfulness of test scores. Given that the Korean EIT is constructed according to common specifications shared by EITs for other languages, we hope that these findings increase the confidence in which researchers and research consumers interpret EIT scores within and across studies.
In closing, when oral proficiency is relevant to research, we exhort researchers to no longer rely on their own intuitions of participants’ ability, class level, written tests, or duration/current levels of exposure: Just ask for 9 more minutes of a participant’s time to administer an EIT. Those 9 minutes will not only facilitate learner group comparisons within a study but also make learner group comparisons across studies possible, contributing to a synthetic understanding of SLA/bilingualism research (Norris & Ortega, Reference Norris, Ortega, Gass and Mackey2012).
Supplementary Materials
To view supplementary material for this article, please visit http://doi.org/10.1017/S0272263121000383.
COMPETING INTERESTS
The authors declare none.