The influence of gender on health is a hot topic nowadays. From then on, research needs to design sex, gender, and intersectional analysis if the aim is to promote global health and biomedicine (Schiebinger, Reference Schiebinger2022). The World Health Organization (WHO) has clearly stated that understanding interactions between gender, biological sex, and health is a priority when it comes to achieving health equity, gender equality and women’s empowerment (Manandhar et al., Reference Manandhar, Hawkes, Buse, Nosrati and Magar2018). Sex (biology) and gender (sociocultural behaviors and attitudes) are variables of individual differentiation that interact with each other to influence health and disease throughout the life cycle (Krieger, Reference Krieger2003). For instance, epidemiology of mental disorders shows differences between men and women generally attributable to sex, when in fact it is gender-related variables that may explain a certain proportion of the prevalence, incidence, and response to treatment of such disorders (Howard et al., Reference Howard, Ehrlich, Gamlen and Oram2017). The gender similarity hypothesis has shown that the context moderates the differences according to sex (Hyde, Reference Hyde2005). The role of gender as a contextual variable exerts an important influence on differences in health, and the study of gender-related contextual variables should be considered together with the traditional sex/gender binary analysis. Therefore, the study of contextual variables should be considered even in the most traditionally binary aspects, such as biological or neuroendocrine dimorphism (Hyde et al., Reference Hyde, Bigler, Joel, Tate and van Anders2019). On the other hand, certain aspects of gender, considered as a social construction and in a multidimensional way, will be different from one culture to another, so it is important to analyze if gender measures created in one cultural group are valid for another different culture. In this research article, the objective was to analyze whether the GVHR, created at Stanford for the North American population, was valid for the Spanish population.
Sex and Gender-Based Analyses
Much work has been done to promote sex and gender-based analyses in health research and to think critically about the influence of sex and gender on health behaviors and outcomes. Public institutions such as the European Commission, the Canadian Institutes of Health Research (CIHR), or the WHO, have made special emphasis on the inclusion of both aspects, sex and gender, in health research (CIHR, 2010; European Commission, 2013; Manandhar et al., Reference Manandhar, Hawkes, Buse, Nosrati and Magar2018). An example of these trends is the publication of practical guidelines to orient researchers in the inclusion of a gender perspective in research, such as Sex and Gender Equity in Research (SAGER) guide (Heidari et al., Reference Heidari, Babor, De Castro, Tort and Curno2016).
Nevertheless, there is little research on health where gender measures are included, since generally only the sex variable is collected, segmenting the data into men and women, and thus, erroneously equating sex with gender (Howard et al., Reference Howard, Ehrlich, Gamlen and Oram2017). Only very rarely, gender is measured through specific instruments such as questionnaires or scales designed to evaluate adherence to gender roles and norms, or gender-related traits. However, none of these instruments is able to capture the specific influence of gender (and its interactions with sex) on health (Nielsen et al., Reference Nielsen, Stefanick, Peragine, Neilands, Ioannidis, Pilote, Prochaska, Cullen, Einstein, Klinge, LeBlanc, Paik and Schiebinger2021). Although the Bem Sex Roles Inventory has been one of the most widely used instruments because of its connection of the androgyny model with health (Bem, Reference Bem1974), its structure does not include other gender-related behaviors and attitudes that could be relevant to health. In addition, the absence of non-binary sex/gender measures hinders the work of researchers, as the available instruments are either designed for men or women, or assess femininity or masculinity from bipolar or unipolar perspectives (Bem, Reference Bem1974; Levant et al., Reference Levant, Richmond, Cook, House and Aupont2007; Mahalik et al., Reference Mahalik, Locke, Ludlow, Diemer, Scott, Gottfried and Freitas2003, Reference Mahalik, Morray, Coonerty-Femiano, Ludlow, Slattery and Smiler2005; Smiler & Epstein, Reference Smiler, Epstein, Chrisler and McCreary2010).
In this context, the implementation of health models that include a sex/gender perspective is of particular importance. Some authors have suggested the development of coefficients for gender in women’s health research that include aspects such as human rights, income, income distribution, and access to education and health caregiver (Phillips, Reference Phillips2005, Reference Phillips2008). The WHO have also recommended to take into account both personal and community-level impacts of gender (World Health Organization, 2003, 2014). Therefore, the use of multivariate models that integrate the complexity of sex/gender variables is a priority, including sex, gender, as well as gender identity, gender relations and institutionalized gender (distribution of power between the genders in the political, educational, religious, media, medical, cultural and social institutions) (Johnson et al., Reference Johnson, Greaves and Repta2009). After all, the “quality-of-life” health model clearly states that research on health should include overall quality of life measures, psychosocial factors, as well as patient’s preferences and perceptions (Wilson & Cleary, Reference Wilson and Cleary1995), which could be particularly significant when applying the sex/gender perspective on health research.
The Gender-Related Variables for Health Research
More recently, Nielsen et al. (Reference Nielsen, Stefanick, Peragine, Neilands, Ioannidis, Pilote, Prochaska, Cullen, Einstein, Klinge, LeBlanc, Paik and Schiebinger2021) have proposed a quantitative measure to incorporate Gender as a Sociocultural Variable (GASV), the Gender-Related Variables for Health Research (GVHR), and thus complement the necessary inclusion of Sex as a Biological Variable (SABV) in health research. According to these authors, three different components should be distinguished when analyzing gender in health research: Gender norms, gender-related traits, and gender relations (Nielsen et al., Reference Nielsen, Stefanick, Peragine, Neilands, Ioannidis, Pilote, Prochaska, Cullen, Einstein, Klinge, LeBlanc, Paik and Schiebinger2021). Gender norms consist in implicit and explicit cultural rules produced and reinforced by social institutions (families, schools, workplaces, etc.), constricting people’s behaviors in terms of persuading men and women to behave and act as it is expected for them to do, according to gender stereotypes (Tannenbaum et al., Reference Tannenbaum, Ellis, Eyssel, Zou and Schiebinger2019). In the instrument developed by Nielsen et al. (Reference Nielsen, Stefanick, Peragine, Neilands, Ioannidis, Pilote, Prochaska, Cullen, Einstein, Klinge, LeBlanc, Paik and Schiebinger2021), adherence to gender norms was measured by three factors: Caregiver strain, time use, and work strain. Gender-related traits concern about how individuals or groups perceive and present themselves in relation to gender norms, analyzing those traits that have been traditionally linked to masculine or feminine gender identities (Wood & Eagly, Reference Wood and Eagly2015). In the cited instrument, gender-related traits were measured as five factors: competitive, risk-taking, independence, communal, and emotional intelligence (Nielsen et al., Reference Nielsen, Stefanick, Peragine, Neilands, Ioannidis, Pilote, Prochaska, Cullen, Einstein, Klinge, LeBlanc, Paik and Schiebinger2021). Lastly, gender relations refer to how people and institutions interact based on their gender or sex (Heise et al., Reference Heise, Greene, Opper, Stavropoulou, Harper, Nascimento and Zewdie2019). It includes power and economic relations, affective and symbolic relations between people with different gender identities (Connell, Reference Connell2012). In the cited instrument, gender relations were measured by three factors: social support, discrimination, and quality of family relationships (Nielsen et al., Reference Nielsen, Stefanick, Peragine, Neilands, Ioannidis, Pilote, Prochaska, Cullen, Einstein, Klinge, LeBlanc, Paik and Schiebinger2021). After analyzing the factorial structure of this model in different samples of the university and adult populations, Nielsen et al. (Reference Nielsen, Stefanick, Peragine, Neilands, Ioannidis, Pilote, Prochaska, Cullen, Einstein, Klinge, LeBlanc, Paik and Schiebinger2021) proposed a shortened version of the GVHR of 25 questions that are grouped into seven dimensions: Caregiver strain, work strain (gender norms), independence, risk taking, emotional intelligence (gender-related traits), social support, and discrimination (gender relations).
In view of all the above, the Gender-Related Variables for Health Research questionnaire (GVHR) is presented as a valid and reliable tool capable of synthesizing and meeting the needs of a gender-sensitive approach to health, addressing how specific gender-related behaviors and attitudes influence health and disease (Nielsen et al., Reference Nielsen, Stefanick, Peragine, Neilands, Ioannidis, Pilote, Prochaska, Cullen, Einstein, Klinge, LeBlanc, Paik and Schiebinger2021). Therefore, the objective of the present study was to conduct the preliminary adaptation of the GVHR to Spanish population, testing its factorial structure and construct validity.
Methods
Participants
The sample included 438 adults (286 women and 152 men) between 19–73 years old (M = 31.90, SD = 12.12). Regarding family status, the 46.0% were single, 49.5% were in a relation (married or partner), 3.7% were divorced, and 0.5% were widowed. The 36.0% of couples lived together and the 28.1% had children. Regarding number of children, the 81.7% had no children, 5.5% had 1 child, 8.4% had 2 children and 4.3% had 3 or more children. Regarding the educational level the 16.7% was secondary, 45.2% was undergraduate, and the 38.1% was post-graduate (master or doctoral studies). The 43.9% reported an income level less than 10,000 euros, the 27.8% indicated between 10,000 and 20,000 euros, the 13.8% indicated between 20,000 and 30,000 euros, the 9,3% indicated between 30,000 and 40,000 euros, and the 5.3% reported an income level greater than 50,000 euros. The occupation was distributed as follows: 56.8% were employed, 18.9% were students and 18.0% studied and worked, 2.7% were unemployed, 2.2% were retired, and 1.8% were housewives.
Regarding ethics approval and consent to participate, we obtained informed consent from all participants. Consent for publication is not applicable.
Variables and Measures
Sociodemographic Data
Participants provided their socio-demographic characteristics, such as sex, age, educational level (secondary, undergraduate and post-graduate), marital status (single, married/partner, divorced and widowed), and income level (< 10,000 euros; 10,000–20,000 euros; 20,000–30,000 euros; 30,000–40,000 euros and > 50,000 euros). Give the low number of divorced and widowed people (n = 14), marital status was dichotomized in data analysis (0 = single (48%); 1 = partner/married (52%)). Also, given the low number of participants in categories greater than 20,000 euros, income level was categorized as 0 = < 10,000 euros (43.9%); 1= 10,000–20,000 euros (27.8%); and 2 = > 20,000 euros (28.3%).
Gender-Related Variables for Health Research (Nielsen et al., Reference Nielsen, Stefanick, Peragine, Neilands, Ioannidis, Pilote, Prochaska, Cullen, Einstein, Klinge, LeBlanc, Paik and Schiebinger2021)
The Gender-Related Variables for Health Research (GVHR) proposed by Nielsen et al. (Reference Nielsen, Stefanick, Peragine, Neilands, Ioannidis, Pilote, Prochaska, Cullen, Einstein, Klinge, LeBlanc, Paik and Schiebinger2021) for North American population, which is composed by 25 items that are grouped into seven factors with a response scale ranges from 1 to 5. The higher the score is, the higher the level in the factor. Caregiver strain refers to the level of emotional stress and physical and emotional exhaustion due to concern for the needs and welfare of others, and is composed by 3 items (e.g., In the past year, how often did you feel physically exhausted because of your caretaking responsibilities?). Work strain refers to the degree to which work is perceived as repetitive, urgent, as well as the degree of emotional stress and perceived physical exhaustion and is composed by 4 items (e.g., How often does your job require working fast?). Independence refers to the degree to which independence and the importance of solving problems for oneself is valuated and is composed by 4 items (e.g., How important is it for you to solve your problems on your own?). Risk taking evaluates the degree to which the person takes risks in general, in the financial and leisure spheres and is composed by 3 items (e.g., In general, how prepared are you to take risks?). Emotional intelligence refers to the degree to which the person recognizes their own feelings and those of others, understanding the role of emotions in interpersonal relationships and is composed by 3 items (e.g., How often do friends talk to you about their problems?). Social support assesses the degree to which the person has people who advise them, show love and affection, and help with day-to-day tasks and is composed by 3 items (e.g., In the past year, how often did you have someone to show you love and affection?). and Discrimination assesses the degree to which the person perceives that they have been discriminated against in education, health care, and public services and is composed by 4 items (e.g., Because of your gender, how often have you felt discriminated against?). The range of reliability values (Raykov’s ρ) of each factor reported for three samples in the English version was ρ = .91–.92, ρ = .86–.91, ρ = .67–.75, ρ = .67–.77, ρ = .65, ρ = .71–.74, ρ = .74–.86, respectively.
Health-Related Quality of Life Core Module, CDC HRQoL–4 (Centers for Disease Control and Prevention, 2000)
This module consists of four items about perceived General Health (i.e., Would you say that in general your health is Excellent, Very good, Good, Fair, or Poor?), recent Physical Health (i.e., Now thinking about your physical health, which includes physical illness and injury, for how many days during the past 30 days was your physical health not good?), Mental Health (i.e., Now thinking about your mental health, which includes stress, depression, and problems with emotions, for how many days during the past 30 days was your mental health not good?), and Activity Limitations (i.e., During the past 30 days, for about how many days did poor physical or mental health keep you from doing your usual activities, such as self-care, work, or recreation?). The variables were dichotomized (0 = good health; 1 = poor health) considering the visual examination of the frequency distribution and the value of the median. Different studies have found acceptable content, construct and criterion validity, and test-retest reliability in this module (Chen et al., Reference Chen, Baumgardner and Rice2011).
Patient-Reported Outcomes Measurement Information System (PROMIS; Ader, Reference Ader2007)
Selected items by the PROMIS were used to measure psychological health. Anxiety (i.e., During the last 30 days, I felt anxiety); Depression (i.e., During the last 30 days, I felt depressed); Resilience (i.e., Over the past 30 days, despite the odds, I have found a way to do what is necessary to continue); and Life Satisfaction (i.e., In general, I am satisfied with my life). Items were answered according to a 5-point Likert scale containing a range of replies from 1 (never) to 5 (always). The variables were dichotomized (0 = poor health; 1 = good health) considering the visual examination of the frequency distribution and the value of the median. Single item measures were chosen to achieve a not very long questionnaire and those PROMIS items that obtained a higher item scale correlation coefficient in previous studies were selected (López-Núñez et al., Reference López-Núñez, Díaz-Morales and Aparicio-García2021; Vilagut et al., Reference Vilagut, Forero, Adroher, Olariu, Cella and Alonso2015).
Work and Family Conflict
“Conflict between Work and Family Relationships” from the abbreviated version of the Gender Role Conflict Scale (O’Neil, Reference O’Neil1981; Wester et al., Reference Wester, Vogel, O’Neil and Danforth2012) was used as measure of work and family conflict. For the present study, the Spanish version by García-Sánchez et al. (Reference García-Sánchez, Almendros, Gámez-Guadix, Martín, Aramayona and Martínez2018) was used. It comprises 4 items (i.e., Finding time to relax is difficult for me; My needs to work or study keep me from my family or leisure more than I would like; My work or school often disrupts other parts of my life (home, health, leisure, etc.); Overwork and stress, caused by a need to achieve on the job or in school, affects/hurts my life), evaluated on a Likert-type scale from 1 (strongly disagree) to 6 (strongly agree). The variable were dichotomized (0 = low; 1 = high) considering the visual examination of the frequency distribution and the value of the median. The reliability of the scale (internal consistency) obtained in the Spanish version was .75 (Cronbach’s alpha).
Health-Risk Behaviors
This measure was composed by the same health indicators used by Nielsen et al. (Reference Nielsen, Stefanick, Peragine, Neilands, Ioannidis, Pilote, Prochaska, Cullen, Einstein, Klinge, LeBlanc, Paik and Schiebinger2021), that is, tobacco consumption, vaping consumption, alcohol consumption, and Body Mass Index (BMI). Smoking and vaping were measured by the number of cigarettes smoked per day and number of times vaping per day, respectively. These variables were dichotomized in the analysis (not smoking = 0, smoking = 1; not vaping = 0, vaping = 1). Alcohol consumption was measured by the frequency of consuming five or more drinks on one occasion for males and four or more drinks on one occasion for females (within the last 3 months). We followed the same procedure used by Nielsen et al. (Reference Nielsen, Stefanick, Peragine, Neilands, Ioannidis, Pilote, Prochaska, Cullen, Einstein, Klinge, LeBlanc, Paik and Schiebinger2021) and recoded these items into a dichotomous variable (binge drinking less than monthly = 0, binge drinking monthly, weekly, or daily/ almost daily = 1). Finally, BMI was calculated based on self-reported height and weight and dichotomized for analysis to reflect normal weight (BMI < 25 = 0) and obese (BMI ≥ 25 = 1).
Procedure and Data Analysis
First, the original test was translated into Spanish with the help of a native expert with extensive knowledge of the study area. Secondly, another English native specialist performed the reverse translation of the items so that finally both specialists could assess the degree of adequacy of the final Spanish version of the items. The sample was recruited via online in October 2022. Participants were encouraged to voluntarily participate in the research through an e-mail list, virtual university campus, and social networks. Then, each participant distributed the link of the study among their contacts in what is known as snowball procedure. They provided their informed consent, and the reward was to receive their individual scores at the end of data collection. In order to test the GVHR’s factorial structure, Confirmatory Factorial Analysis with unweighted least squares (ULS) estimator was used, since it is a better analytical approximation for ordinal variables (Yang-Wallentin et al., Reference Yang-Wallentin, Jöreskog and Luo2010). Model fit was based on several recommended criteria (Bentler, Reference Bentler1992), the chi-square test (χ2), the normed fit index (NFI > .90), the comparative fit index (CFI > .90), the goodness of fit index (GFI > .90), the root mean square error of approximation (RMSEA < .06 acceptable, between .07 – .09 mediocre, and > .10 poor fit). Also χ2/df ratio was used. A small value (< 3) is considered a reasonable adjustment, while values close to 2 are considered as very good. In order to test the factorial invariance across sex, a multiple group confirmatory factor analysis (MGCFA) with LISREL methodology (Jöreskog & Sörbom, Reference Jöreskog and Sörbom1993) was used. The chi-square differences between two-nested models were used as indication of significant improvements. Cheung and Rensvold (Reference Cheung and Rensvold2002) have suggested that change of magnitude in CFI (< .01) incremental index can also be used for testing measurement invariance. Cronbach’s alpha coefficients were calculated for those measures composed by several items. Pearson correlation coefficients were computed to analyze relationships between GVHR sub-scales. In order to analyze the association of gender (GVHR) with Health-Related Quality of Life, Psychological Health, and Health-Risk Behaviors, logistic regression analysis was used to test the association of sex with the health variables. In a second step, the association of gender with the health variables, adjusted by sex, age, income level, marital status, and educational level variables was analyzed. Again, we used logistic regressions to compare our results with those obtained by Nielsen et al. (Reference Nielsen, Stefanick, Peragine, Neilands, Ioannidis, Pilote, Prochaska, Cullen, Einstein, Klinge, LeBlanc, Paik and Schiebinger2021). The Statistical Program for the Social Sciences (version 19; IBM Corp., Armonk, NY) and Lisrel 8.50 (Jöreskog et al., Reference Jöreskog, Sörbom, du Toit and du Toit2001) were used. All statistical tests were two tailed and type–I error rate was set to 5% (α = .05).
Results
Factorial Structure, Sex Factorial Invariance, Reliability and Relations between Sub-Scales of GVHR
Confirmatory Factorial Analysis (CFA) of GVHR indicated acceptable fit, χ² = 921.76, df = 254, RMSEA = .061 (.050–.068), NFI = .77, CFI = .86 and GFI = .86, although fit indices did not comply with the recommended criteria and a χ2/df ratio > 3 (Bentler, Reference Bentler1992). Also, all factors showed good internal consistency except the independence (α = .31) and emotional intelligence (α = .52) factors. Given these results, a second CFA of five factors was performed with better fit indicators (χ² = 227.89, df = 109, RMSEA = .050 (.040–.059), NFI = .91, CFI = .94 and GFI = .90) and a χ2/df ratio (2.09) acceptable. Next, in order to test factorial invariance by sex, the first step was performed CFA for men, χ² = 161.27 (p < .001), df = 109, RMSEA = .056 (.036–.073), NFI = .83, CFI = .93 and GFI = .97; and women, χ² = 179.94 (p < .001), df = 109, RMSEA = .048 (.034–.059), NFI = .89, CFI = .95 and GFI = .97, with acceptable fit indices. The factorial loads and the descriptive statistics of each item of the GVHR appear in Table 1.
Table 1. Factor Loadings of Confirmatory Factor Analysis and Descriptive Statistics of the Items of the Gender-Related Variables for Health Research

Note: Confirmatory Factorial Analysis using Unweighted Least Squares method estimator. M = Mean; SD = Standard Deviation; SE = Standard Error. Response scale ranges from 1 to 5.
The second step was to calculate MGCFA in order to validate the five-factor model across sex. Configural invariance model tests whether the proposed structure would be equal across sex, with acceptable fit indices (χ² = 281.49 (p < .01), df = 228, RMSEA = .033 (.017–.044), NFI = .90, CFI = .98 and GFI = .94. Third, metric invariance refers to the analysis of equal factor loadings across sex and the results indicated good indices, χ² = 336.16 (p < .01), df = 245, RMSEA = .041 (.029–.051), NFI = .89, CFI = .96 and GFI = .95. However, the chi-square difference test indicated that factor loadings were different across sex Δχ2(17) = 54.67***. In order to test if any of five factors was invariant, we tested metric invariance in each one. Results indicated metric invariance in: Caregiver Strain factor, χ² = 287.62 (p < .01), df = 231, RMSEA = .033 (.018–.045), NFI = .90, CFI = .98 and GFI = .95; Δχ2(3) = 6.13. Risk Taking factor, χ² = 283.77 (p < .05), df = 231, RMSEA = .032 (.016–.044), NFI = .90, CFI = .98 and GFI = .94; Δχ2(3) = 2.28. Social Support factor, χ² = 284.77 (p < .01), df = 231, RMSEA = .032 (.016–.044), NFI = .90, CFI = .98 and GFI = .94. Δχ2(3) = 3.28. Although the chi-square difference test was significant in Work Strain factor, Δχ2(4) = 15.4**), and Discrimination factor, Δχ2(4) = 30.15***, the change of magnitude in CFI (< .01) incremental index proposed by Cheung and Rensvold (Reference Cheung and Rensvold2002) suggested that can also be considered sex invariant regarding factorial loadings: Work Strain factor, χ² = 296.89 (p < .01), df = 232, RMSEA = .036 (.022–.047), NFI = .90, CFI = .97 and GFI = .93. Discrimination factor, χ² = 296.89 (p < .01), df = 232, RMSEA = .036 (.022–.047), NFI = .90, CFI = .97 and GFI = .93. Finally, scalar invariance was also supported in all factors, χ² = 303.56 (p < .05), df = 256, RMSEA = .029 (.012–.041), NFI = .90, CFI = .98 and GFI = .93; Δχ2(28) = 22.07. Scalar invariance indicates that the factors latent means can be meaningfully compared across sex.
The pattern of correlations was coherent according to the theoretical framework, indicating that the relationship between the scales was low (Table 2). Caregiver strain was positively related to work strain and discrimination, and negatively with social support. Work strain was positively related to discrimination. Risk taking was negatively related with sex, indicating a higher tendency to take risks in men. Social support was negatively related to age and positively relate with sex (women). Finally, discrimination was negatively related with age and positively related with sex (women).
Table 2. Means (M), Standard Deviations (SD) and Pearson Correlation Coefficients between Sub-Scales of the Gender-Related Variables for Health Research (GVHR)

Note: n = 438; Sex: 0 = men; 1 = women.
* p < .05.
** p < .01.
*** p < .001.
Sex Differences on Health-Related Quality of Life, Psychological Health and Health-Risk Behaviors
As displayed in Table 3, women reported worse mental and physical health, health limitations, and a lower BMI. Regarding the psychological health variables, there were no differences according to sex. Considering the health risk behaviors, low BMI was associated with being a woman. Identical results were obtained adjusting for educational level, marital status, and income level.
Table 3. Univariate Logistic Regressions with of Sex as Predictor Variable and Health-Related Quality of Life, Psychological Health, and Health Risk Behaviors as Criteria Variables
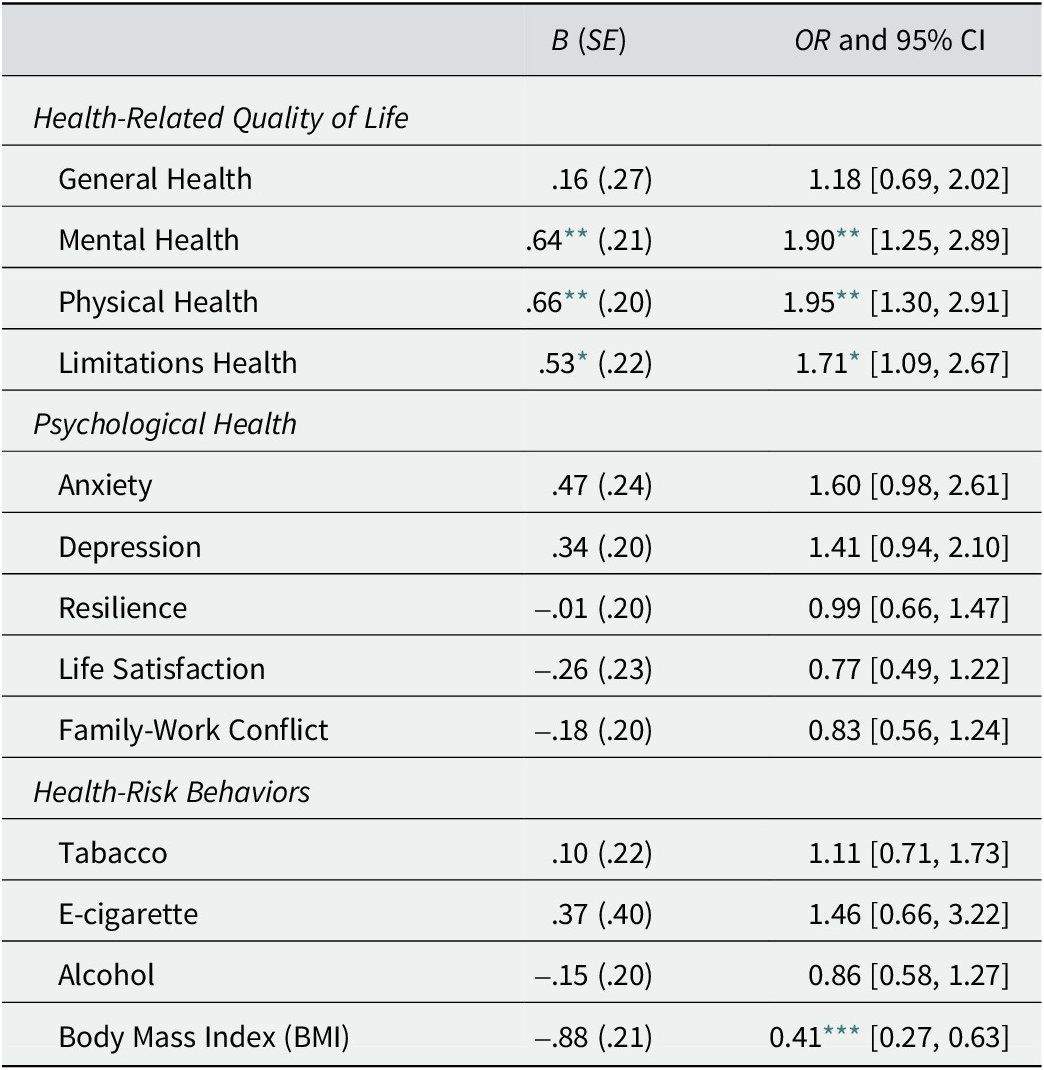
Note: Sex: men = 0; women = 1; B = Beta Coefficient; SE = Standard Error; OR = Odd Ratio; CI = confidence interval; Health-Related Quality of Life variables dichotomized as 0 = good health and 1 = poor health; Psychological Health variables dichotomized as 0 = poor health and 1 = good health; Health-Risk Behaviors variables was dichotomized as 0 = low; 1 = high.
* p < .05.
** p < .01.
*** p < .001.
Sex and Gender Differences on Health-Related Quality of Life, Psychological Health and Health-Risk Behaviors
Regarding Health-Related Quality of Life variables (see Table 4) worse general health was associated with higher caregiver strain and discrimination, and with lower risk taking. Worse mental health was associated with younger age, higher caregiver strain, work strain and discrimination, and lower social support. Finally, worse physical health and greater health limitations were both associated with higher caregiver strain.
Table 4. Multivariate Logistic Regressions with Demographic Variables (Sex, Education, Income and Age) and GVHR (Caregiver Strain, Work Strain, Risk Taking, Social Support and Discrimination) as Predictor Variables and Health-Related Quality of Life (General, Mental, Physical and Limitations) as Criteria Variables

Note: Sex (reference group = men); Personal Income (reference group = < 10,000 euros); Educational level (reference group = secondary studies); Marital Status (reference group = single). A higher score in General, Mental and Physical Health, and Health Limitations indicates worse health (0 = good health; 1 = poor health). GVHR = Gender-Related Variables for Health Research; B = Beta Coefficient; SE = Standard Error; OR = Odd Ratio; CI = confidence interval.
* p < .05.
** p < .01.
*** p < .001.
Regarding the relationships of sex and gender with Psychological Health variables (see Table 5), the results indicated that high anxiety was associated with higher caregiver strain, and discrimination. Depression was associated with higher caregiver strain and discrimination. Resilience was associated with higher risk taking and social support. Life satisfaction was associated with a post-graduate education level and higher social support, and with lower work strain. Finally, family and work conflict was associated with higher caregiver strain and work strain.
Table 5. Multivariate Logistic Regressions with Demographic Variables (Sex, Education, Income and Age) and GVHR (Caregiver Strain, Work Strain, Risk Taking, Social Support and Discrimination) as Predictor Variables and Psychological Health (Anxiety, Depression, Resilience, Life Satisfaction and Work-Family Conflict) as Criteria Variables

Note: Sex (reference group = men); Personal Income (reference group = < 10,000 euros); Educational level (reference group = secondary studies); Marital Status (reference group = single). A higher score in criteria variables indicated higher level (0 = poor health; 1 = good health). GVHR = Gender-Related Variables for Health Research; B = Beta Coefficient; SE = Standard Error; OR = Odd Ratio; CI = confidence interval.
* p < .05.
** p < .01.
*** p < .001.
Lastly, regarding the relationships of sex and gender with Health Risk Behaviors (see Table 6), the results indicated that greater alcohol consumption was associated with being a man, undergraduate level, discrimination, and younger age. Tobacco and E-cigarette consumption use were not associated with any variable. Finally, a higher BMI was associated with being a man, a high income level, being single, and being older.
Table 6. Multivariate Logistic Regressions with Demographic Variables (Sex, Education, Income and Age) and GVHR (Caregiver Strain, Work Strain, Risk Taking, Social Support and Discrimination) as Predictor Variables and Health Risk Behaviors (Alcohol, Tobacco, E-cigarette and BMI) as Criteria Variables

Note: Sex (reference group = men); Personal Income (reference group = < 10,000 euros); Educational level (reference group = secondary studies); Marital Status (reference group = single). A higher score in criteria variables indicated higher level (0 = low; 1 = high). GVHR = Gender-Related Variables for Health Research; B = Beta Coefficient; SE= Standard Error; OR = Odd Ratio; CI = confidence interval.
* p < .05.
** p < .01.
*** p < .001.
Discussion
Existing research showed notable sex differences in health-related quality of life, psychological health, and risky health behaviors. Here, we examined the relevance of both sex and gender-related variables in predicting these health behaviors by using the Spanish version of the GVHR. Two main results could be highlighted. First, the seven-factor structure of the GVHR was not replicated in the Spanish population, with the independence and emotional intelligence factors not reaching an adequate level of reliability. This result is not surprising, given that as a social construct, different aspects of gender can differ across cultures (Else-Quest & Hyde, Reference Else-Quest and Hyde2017). Secondly, the gender aspects evaluated allow a better understanding of the differences according to sex in health (Bauer & Scheim, Reference Bauer and Scheim2019), especially for the Health-Related Quality of Life and Psychological Health variables, and not for the Health-Risk Behaviors.
As already explained by Nielsen et al. (Reference Nielsen, Stefanick, Peragine, Neilands, Ioannidis, Pilote, Prochaska, Cullen, Einstein, Klinge, LeBlanc, Paik and Schiebinger2021), each of these constructs assess an individual self-report behavior or attitude and is separately scored as a different conduct or trait, without being labelled into unipolar or bipolar leads (e.g., “masculine” or feminine”). Concerning independence trait, it refers to the person as an individual, versus collectivism, including aspects such as self-confidence, decision-making ability, or agency (Oyserman et al., Reference Oyserman, Coon and Kemmelmeier2002). The concept of independence may entail a different connotation in a Spanish context, where dependence on family and social connections in various spheres of life is probably more pronounce (Moreno Mínguez, Reference Moreno Mínguez2010). Also, this construct might share some behaviors and attributes with emotional intelligence and social support. In the current study, we found a negative correlation between these variables and independence. Same result has been described before, showing a relationship between collectivistic orientation and greater emotional intelligence (Bhullar et al., Reference Bhullar, Schutte and Malouff2012). The same argument could be used for the emotional intelligence factor, since the aspects related to the communication of problems and concerns, as well as the expression of feelings may be aspects that have not been well captured by the items. It is also necessary to point out the inherent difficulty of evaluating this construct from the perspective of individual differences (Martins et al., Reference Martins, Ramalho and Morin2010). Future studies should address the evaluation of these two factors, analyzing whether a larger number of items could give a better account of both constructs.
Regarding whether the scale can be considered equivalent for each sex, the results of the factorial invariance analysis indicated that the scale is invariant in terms of its structure (configural invariance), the factorial loads are equivalent for each sex (metric invariance) and the latent factors are equivalent to compare the means of both groups (scalar invariance).
Second, regarding GVHR relationship with health variables, caregiver strain was the variable associated with worse results in all Health-Related Quality of Life measures (general, mental, physical, and health limitations). Caregiver strain refers to the level of emotional stress and physical and emotional exhaustion due to concern for the needs and welfare of others (Caprara et al., Reference Caprara, Steca, Zelli and Capanna2005). The negative relations between worse general health and higher risk taking was an unexpected outcome. This construct focuses on risk-taking behaviors when making financial decisions and with respect to leisure activities (Dohmen et al., Reference Dohmen, Falk, Huffman, Sunde, Schupp and Wagner2011). However, a better perception of health status has been previously associated with increased risk attitudes in lottery games (Martín-Fernández et al., Reference Martín-Fernández, Ariza-Cardiel, Polentinos-Castro, Gil-Lacruz, Gómez-Gascón, Domínguez-Bidagor and del-Cura-González2016). People in good health might assurance its productivity and prevent from the health-maintaining expense, which releases economic reserves for other goods and services (Smith & Keeney, Reference Smith and Keeney2005). Also, the way that risk taking was measured in the study may be connected with assertiveness in decision making, which has been previously positively associated to mental health in different studies (Pourjali & Zarnaghash, Reference Pourjali and Zarnaghash2010).
Worse mental health was associated with being younger, greater caregiver strain, work strain, discrimination, and low social support. These results are consistent with existing previous literature and several publications related to recent COVID-19 pandemic (Varma et al., Reference Varma, Junge, Meaklim and Jackson2021; Zheng & Echave, Reference Zheng and Echave2021). For instance, Varma and colleagues (Reference Varma, Junge, Meaklim and Jackson2021), in a survey with more than 1,600 participants from over 60 countries found that younger respondents were at a higher risk of low mental health (anxiety, stress, and depression), linked to subjective strain, loneliness, and financial uncertainty (Varma et al., Reference Varma, Junge, Meaklim and Jackson2021). Finally, worse physical health and limitations were related with caregiver strain, result in line with different research studies that state that the evidence on the health effects of caregiving is a fact (Pinquart & Sörensen, Reference Pinquart and Sörensen2003; Vitaliano et al., Reference Vitaliano, Zhang and Scanlan2003).
With reference to psychological health variables (anxiety, depression, resilience, and life satisfaction), again caregiver strain and discrimination were the variables most associated with anxiety and depression. While caregiving strain is a well-known predictor of mental morbidity, gender discrimination should be also highlighted. Gender discrimination, both in public and private spheres of life, has a great cost for mental health. Gender discrimination is linked to multiple mental health risk factors such as unequal access to wealth, discrimination at work, isolation, abuse, and loss of rights, and a host of other variables that threaten the mental health and wellbeing of those who suffer discrimination (World Health Organization, 2000). Lastly, regarding the association of sex and gender with health risk behaviors, being a man was associated with greater alcohol consumption and higher BMI. On the other hand, discrimination was related to higher alcohol consumption.
Health effects of caregiving are widely well known (Litzelman et al., Reference Litzelman, Skinner, Gangnon, Nieto, Malecki and Witt2015; Pinquart & Sörensen, Reference Pinquart and Sörensen2007). The novel result of the present study is that caregiver strain gender variable has stronger correlations with physical and psychological health (i.e., anxiety and depression), than biological sex. This study evidences that gender-related contextual variables can be great predictors of health, especially for health-related quality of life and for psychological health. This is not the case for the health risk behaviors, where only social support was associated with tobacco use. Although future research will be needed to confirm this outcome, interventions to improve caregiver health might be focus on high stress caregivers and other contextual variables such as work strain and gender discrimination, rather than just biological sex. This idea has important consequences for practice, policy, and research, and it could be explored in larger sample of participants or in specific population groups such as health professionals, formal and informal caregivers, and other groups.
When comparing the results of Nielsen et al. (Reference Nielsen, Stefanick, Peragine, Neilands, Ioannidis, Pilote, Prochaska, Cullen, Einstein, Klinge, LeBlanc, Paik and Schiebinger2021) with those obtained in the Spanish population, many similarities can be observed. Among all gender-related variables, caregiving strain and discrimination were those consistently associated with poorer health indicators (physical health, mental health, and general health, varying from one study to another). Also, social support showed to be associated to better mental health in both studies. In addition, risk taking was unexpectedly associated to some positive health outcomes (general, self-rated health) in both studies, indicating that future studies should pay attention to this variable and its connections with health under a gender perspective. However, work strain was not associated to a poorer health in the study run by Nielsen et al. (Reference Nielsen, Stefanick, Peragine, Neilands, Ioannidis, Pilote, Prochaska, Cullen, Einstein, Klinge, LeBlanc, Paik and Schiebinger2021), as it was in the Spanish study.
Spanish clinical professionals and researchers might use the GVHR to obtain a better understanding of gender differences in health variables. As well as their authors, we recommend measuring GVHR variables beside sex assigned at birth, self-reported gender identity, sexual orientation, education, income, and age, to define which attributes, traits and conducts may be predicted to analyze a particular disease (Nielsen et al., Reference Nielsen, Stefanick, Peragine, Neilands, Ioannidis, Pilote, Prochaska, Cullen, Einstein, Klinge, LeBlanc, Paik and Schiebinger2021). Nevertheless, future studies should refine the concepts of independence and emotional intelligence in order to capture its influence on health, in the Spanish context. The current instrument was developed to show how gender norms, related traits, and relations outline the health-illness relationship.
Following Nielsen and collaborators’ guidelines of test the measure within different cultures to increase its validity, with the present study we aimed to contribute to the inclusion of gender on health research by the Spanish adaption of the GVHR. Nevertheless, the results of this study should be interpreted considering some limitations. In the first place, given the cross-sectional and correlational design, no causality can be inferred from the found associations. Additionally, the used measures were validated in a non-probability sample, online recruited, which might restrain generalizability. Finally, we were forced to remove the independence and emotional intelligence factors from the 7-construct original model because of its low reliability reached. Future studies should be carried out across more cultures to confirm or disprove these results, as well as their potential implications for health.
As strength, to our knowledge, this is the first study that has adapted the GVHR questionnaire to the Spanish language. This instrument can be readily implemented in population surveys to track whether gender-related behaviors and attitudes take part on health and disease pathways, apart from biological sex and gender identity. The results presented in this study open the way for further research to elucidate if gender domains are relevant measures not only at the assessment sphere, but also at the health intervention one.
Funding statement
Research reported in this publication was supported by a grant from the Plan Estatal de Investigación Científica, Técnica y de Innovación (2021–2023). Ministerio de Ciencia e Innovación, Gobierno de España (Principal Investigator: JFDM; Ref. PID2020–116600RB–I00).
Competing interest
None.
Data sharing
Data are available on request from the first author of the study.
Authorship credit
J.F.D.M. was the project administrator, participated in its formal analysis and data curation, supervised and validated the manuscript; S.E. participated in the writing and supervision of the study; N.M.M. helped to write and validate the study; Y.P.N. helped with the formal analysis and data curation. All authors read and approved the final manuscript and participated in the interpretation of the data.
Author’s note
Juan F. Díaz-Morales, Sara Esteban-Gonzalo, and Yaiza Puig-Navarro work for the Departamento de Psicología Social, del Trabajo y Diferencial; Facultad de Psicología, Universidad Complutense de Madrid. Besides, Juan F. Díaz-Morales works for the Instituto de Investigaciones Feministas, Universidad Complutense de Madrid, and Yaiza Puig-Navarro works for Hogrefe TEA Ediciones.
Natalia Martín-María works for the Departamento de Psicología Biológica y de la Salud, Facultad de Psicología, Universidad Autónoma de Madrid. Natalia Martín-María also works for Centro de Investigación Biomédica en Red de Salud Mental, CIBERSAM.
Appendix
Items of Spanish Version of Stanford Gender-Related Variables for Health Research

Note: Caregiver Strain (Items 1-3); Work Strain (Items 4-7); Risk Taking (Items 8-10); Social Support (Items 11-13); Discrimination (Items 14-17).