Introduction
Antimicrobial resistance (AMR) is a global One Health crisis (McEwen & Collingnon, Reference McEwen and Collignon2017; Søgaard Jørgensen et al., Reference Søgaard Jørgensen, Wernli and Carroll2016; van Helden et al., Reference van Helden, van Helden and Hoal2013; Shomaker, Reference Shomaker2014; Robinson et al., Reference Robinson, Bu, Carrique-Mas, Fèvre, Gilbert, Grace, Hay, Jiwakanon, Kakkar, Kariuki, Laxminarayan, Lubroth, Magnusson, Thi Ngoc, Van Boeckel and Woolhouse2016; The European Commission, 2018) causing economic and health burdens in humans, animals, and the environment (The European Commission, 2018; WHO Regional office for Europe, 2017). In 2019, 4.95 million deaths worldwide (23,100 deaths in western and central Europe) were associated with AMR (Murray et al., Reference Murray, Ikuta, Sharara, Swetschinski, Robles Aguilar, Gray, Han, Bisignano, Rao, Wool, Johnson, Browne, Chipeta, Fell, Hackett, Haines-Woodhouse, Kashef Hamadani, Kumaran, McManigal, Achalapong, Agarwal, Akech, Albertson, Amuasi, Andrews, Aravkin, Ashley, Babin, Bailey, Baker, Basnyat, Bekker, Bender, Berkley, Bethou, Bielicki, Boonkasidecha, Bukosia, Carvalheiro, Castañeda-Orjuela, Chansamouth, Chaurasia, Chiurchiù, Chowdhury, Clotaire Donatien, Cook, Cooper, Cressey, Criollo-Mora, Cunningham, Darboe, Day, De Luca, Dokova, Dramowski, Dunachie, Duong Bich, Eckmanns, Eibach, Emami, Feasey, Fisher-Pearson, Forrest, Garcia, Garrett, Gastmeier, Giref, Greer, Gupta, Haller, Haselbeck, Hay, Holm, Hopkins, Hsia, Iregbu, Jacobs, Jarovsky, Javanmardi, Jenney, Khorana, Khusuwan, Kissoon, Kobeissi, Kostyanev, Krapp, Krumkamp, Kumar, Kyu, Lim, Lim, Limmathurotsakul, Loftus, Lunn, Ma, Manoharan, Marks, May, Mayxay, Mturi, Munera-Huertas, Musicha, Musila, Mussi-Pinhata, Naidu, Nakamura, Nanavati, Nangia, Newton, Ngoun, Novotney, Nwakanma, Obiero, Ochoa, Olivas-Martinez, Olliaro, Ooko, Ortiz-Brizuela, Ounchanum, Pak, Paredes, Peleg, Perrone, Phe, Phommasone, Plakkal, Ponce-de-Leon, Raad, Ramdin, Rattanavong, Riddell, Roberts, Robotham, Roca, Rosenthal, Rudd, Russell, Sader, Saengchan, Schnall, Scott, Seekaew, Sharland, Shivamallappa, Sifuentes-Osornio, Simpson, Steenkeste, Stewardson, Stoeva, Tasak, Thaiprakong, Thwaites, Tigoi, Turner, Turner, van Doorn, Velaphi, Vongpradith, Vongsouvath, Vu, Walsh, Walson, Waner, Wangrangsimakul, Wannapinij, Wozniak, Young Sharma, Yu, Zheng, Sartorius, Lopez, Stergachis, Moore, Dolecek and Naghavi2022). AMR has also impacted the agricultural sector by causing loss of production due to animal illness with resistant infections and has decreased trade due to a fear of resistance (The European Commission, 2018). AMR emerges from interactions in the complex One Health system that spans the human-animal-environment interface (O’Neill, Reference O’Neill2016; McEwen and Collignon, Reference McEwen and Collignon2017; The European Commission, 2018; WHO, 2018) and is exacerbated by globalization (Frost et al., Reference Frost, Van Boeckel, Pires, Craig and Laxminarayan2019; Holmes et al., Reference Holmes, Moore, Sundsfjord, Steinbakk, Regmi, Karkey, Guerin and Piddock2016; Lambraki et al., Reference Lambraki, Cousins, Graells, Léger, Henriksson, Harbarth, Troell, Wernli, Søgaard Jørgensen, Desbois, Carson, Parmley, Majowicz and Islam2022a; Wegner et al., Reference Wegner, Murray, Springmann, Muller, Sokolow, Saylors and Morens2022). Antimicrobial use (AMU) in human medicine and food production has been at the forefront of research and the focus of targeted action to reduce AMR (The European Commission, 2018; WHO, 2018), however there are a multitude of factors that affect why and how we use antimicrobials, including: socioeconomic factors (e.g., poverty, access to nutritious food and clean water); society and social pressures (e.g., quick fix to get back to work); and economic factors (e.g., decreased losses in food production, lowering production costs) (Holmes et al., Reference Holmes, Moore, Sundsfjord, Steinbakk, Regmi, Karkey, Guerin and Piddock2016; McEwen and Collignon, Reference McEwen and Collignon2017; Alividza et al., Reference Alividza, Mariano, Ahmad, Charani, Rawson, Holmes and Castro-Sánchez2018; Lambraki et al., Reference Lambraki, Cousins, Graells, Léger, Henriksson, Harbarth, Troell, Wernli, Søgaard Jørgensen, Desbois, Carson, Parmley, Majowicz and Islam2022a; Wernli et al., Reference Wernli, Jørgensen, Harbarth, Carroll, Laxminarayan, Levrat and Pittet2017; Søgaard Jørgensen et al., Reference Søgaard Jørgensen, Folke, Henriksson, Malmros, Troell and Zorzet2020). Many past attempts to address AMR have failed to account for interactions in the One Health and socio-ecological system and lacked integration and communication between the multiple actors involved in the complex system (Government of Canada, 2017; The European Commission, 2018; Lambraki et al., Reference Lambraki, Cousins, Graells, Léger, Henriksson, Harbarth, Troell, Wernli, Søgaard Jørgensen, Desbois, Carson, Parmley, Majowicz and Islam2022a). For example, if policies or interventions were taken in one sector (e.g., reducing antibiotic use in food animals and agriculture), this could negatively impact another sector (e.g., more costs for human consumers). Therefore, a One Health perspective is needed to adequately address AMR.
Due to the intimate relationship between the environment, humans, and animals, climate change is predicted to worsen the problem of AMR, however the impacts across the One Health system are associated with high levels of uncertainty (Fouladkhah et al., Reference Fouladkhah, Thompson and Camp2020; Burnham, Reference Burnham2021; Pepi and Focardi, Reference Pepi and Focardi2021; Rodríguez-Verdugo et al., Reference Rodríguez-Verdugo, Lozano-Huntelman, Cruz-Loya, Savage and Yeh2020). Furthermore, the livestock production system is both a driver of AMR and a large contributing factor of climate change, thus perpetuating both issues in tandem (Søgaard Jørgensen et al., Reference Søgaard Jørgensen, Folke, Henriksson, Malmros, Troell and Zorzet2020). Within Sweden, temperatures are predicted to increase (especially in the northern part of the country) with increased precipitation events and unpredictable weather patterns (Meehl et al., Reference Meehl, Stocker, Collins, Friedlingstein, Gaye, Gregory, Noda, M., Solomon, D.Qin, Manning, Chen, Marquis and Averyt2007). The changes in weather in Sweden and globally may lead to an increase of disease in humans, animals, and crops (World Health Organization, 2017; Rodríguez-Verdugo et al., Reference Rodríguez-Verdugo, Lozano-Huntelman, Cruz-Loya, Savage and Yeh2020; Carlson et al., Reference Carlson, Albery, Merow, Trisos, Zipfel, Eskew, Olival, Ross and Bansal2022), impact food production (Morse, Reference Morse1995; Hoffmann, Reference Hoffmann2010; Van Dijk et al., Reference Van Dijk, Sargison, Kenyon and Skuce2010; Lacetera, Reference Lacetera2019; Abirham, Reference Abirham2020; Reverter et al., Reference Reverter, Sarter, Caruso, Avarre, Combe, Pepey, Pouyaud, Vega-Heredía, de Verdal and Gozlan2020), and cause mass migrations into less vulnerable countries such as Sweden, potentially leading to overcrowding (Parry et al., Reference Parry, Rosenzweig and Livermore2005; Semenza and Ebi, Reference Semenza and Ebi2019; Abirham, Reference Abirham2020). Overall, there is expected to be great need for effective antimicrobials in the future. Therefore, understanding how climate change will shape the One Health system and how changed in the system drive AMR, especially how it may impact AMU and AMR, and identifying sustainable interventions that can help mitigate these impacts using a One Health perspective in the future is vital.
Simulation modelling (gray and Rumpe, Reference Gray and Rumpe2016) explores how a system may be affected by different scenarios (e.g., climate change) and assess interventions. AMR has been modelled within specific areas (e.g., agricultural system, health system), but the entire One Health system of drivers has yet to be modelled (Cousins et al., Reference Cousins, Parmley, Greer, Neiterman, Lambraki, Vanderheyden, Wernli, Jorgensen, Carson and Majowicz2024). Fuzzy cognitive mapping is a semi-quantitative simulation modelling technique (Kosko, Reference Kosko1986) that enables a One Health lens to account for the complex socio-ecological drivers of AMR (Cousins, Reference Cousins2022a). First introduced by Kosko in 1986 (Kosko, Reference Kosko1986), fuzzy cognitive maps (FCMs) have shown promise in modelling complex dynamic systems in many disciplines (Alipour et al., Reference Alipour, Hafezi, Papageorgiou, Hafezi and Alipour2019; Ntarlas and Groumpos, Reference Ntarlas and Groumpos2015; Dorokhov et al., Reference Dorokhov, Dorokhova, Delibasic and Streimikis2017; Poomagal et al., Reference Poomagal, Sujatha, Kumar and Vo2021). These models use expert knowledge to construct representations of the causal relationships between components that describe a system (Kokkinos et al., Reference Kokkinos, Lakioti, Papageorgiou, Moustakas and Karayannis2018). FCMs consist of concepts (or components or nodes), connected by weighted causal relationships, defined in linguistic terms (e.g., strong vs weak, high vs low (Kosko, Reference Kosko1986; Nápoles et al., Reference Nápoles, Espinosa, Grau and Vanhoof2018). FCMs are useful for decision-making in systems with incomplete or non-specific data or undefined interactions (Sypher, Reference Sypher2017). As a case study, Cousins, Reference Cousins2022a created a FCM of AMR in the Swedish One Health system, informed by literature and expert opinion (Cousins, Reference Cousins2022a). Using the previously defined FCM, the objectives of this study were to: 1) identify areas in the system to target interventions; and 2) test the potential ability and viability of interventions to reduce AMR under a changing climate.
Methods
Using a previously created FCM of AMR development and transmission in the Swedish One Health system, this study further explored the system that drives AMR and assessed interventions under climate change conditions (Cousins, Reference Cousins2022a). The structure of the FCM was based upon a qualitative model that was created during two participatory modelling workshops held in Sweden with experts from within the European food system (Lambraki et al., Reference Lambraki, Cousins, Graells, Léger, Henriksson, Harbarth, Troell, Wernli, Søgaard Jørgensen, Desbois, Carson, Parmley, Majowicz and Islam2022a,Reference Lambraki, Cousins, Graells, Léger, Abdelrahman, Desbois, Gallagher, Staaf Larsson, Mattson, Henriksson, Troell, Søgaard Jørgensen, Wernli, Carson, Parmley and Majowicz2022b). A second set of workshops were used as to help inform the interventions and compare outcomes from the scenario analyses. Together these will further be referred to as the workshops. The methods for the creation of the FCM are fully described in the initial case study (Cousins, Reference Cousins2022a), but a brief outline is described to provide a basis of understanding.
The participatory modelling workshops
A set of workshops took place on September 19th and 20th, 2019 at the Stockholm Resilience Centre in Stockholm, Sweden and online on September 11th and 12th, 2020 with seventeen experts from across the One Health spectrum in fields such as veterinary and aquatic sciences, consumer and public health advocacy, agricultural crops, and pharmaceutical marketing (Lambraki et al., Reference Lambraki, Cousins, Graells, Léger, Henriksson, Harbarth, Troell, Wernli, Søgaard Jørgensen, Desbois, Carson, Parmley, Majowicz and Islam2022a). The purpose of these workshops was for experts from within the broad One Health system in Europe to: 1) map out the drivers of AMR including the major factors and interrelationships (Lambraki et al., Reference Lambraki, Cousins, Graells, Léger, Henriksson, Harbarth, Troell, Wernli, Søgaard Jørgensen, Desbois, Carson, Parmley, Majowicz and Islam2022a), and 2) discuss the success of two interventions to combat AMR (taxation of antimicrobials (AMs) at point of sale, and increased infection prevention and control measures) under potential climate change conditions (Lambrakiet al., Reference Lambraki, Cousins, Graells, Léger, Abdelrahman, Desbois, Gallagher, Staaf Larsson, Mattson, Henriksson, Troell, Søgaard Jørgensen, Wernli, Carson, Parmley and Majowicz2022b). During these workshops, experts discussed the major drivers of AMR and the interrelationships between these drivers, which were visually represented as a causal loop diagram (CLD) consisting of 92 nodes (drivers) and 334 relationships (). This CLD served as the base structure of the FCM.
Brief description of building the FCM
FCMs (Kosko, Reference Kosko1986) are dynamic models that combine fuzzy logic, neural networks, and cognitive mapping (Kosko, Reference Kosko1986; Kokkinos et al., Reference Kokkinos, Lakioti, Papageorgiou, Moustakas and Karayannis2018; Nápoles et al., Reference Nápoles, Espinosa, Grau and Vanhoof2018). The components and causal relationships between the components together form a neural network (Kokkinos et al., Reference Kokkinos, Lakioti, Papageorgiou, Moustakas and Karayannis2018; Nápoles et al., Reference Nápoles, Espinosa, Grau and Vanhoof2018). Each component has an activation value (AV) assigned a value from [0,1] and each relationship has a weight (reflects the degree of causality between the components) assigned a value between [−1,1], with negative values indicating an inverse relationship (Nápoles et al., Reference Nápoles, Espinosa, Grau and Vanhoof2018). Fuzzy logic (Zadeh, Reference Zadeh1990) is used to convert quantitative data (e.g., surveillance data) and qualitative data (e.g., linguistic terms) into a common format to inform the AVs and weights.
The CLD (Lambraki et al., Reference Lambraki, Cousins, Graells, Léger, Henriksson, Harbarth, Troell, Wernli, Søgaard Jørgensen, Desbois, Carson, Parmley, Majowicz and Islam2022a) served as the base structure, refined by the available data from the literature and accounts made by the participants during the workshops (Cousins, Reference Cousins2022a), resulting in 90 components (Cousins, Reference Cousins2022a, Table S1). The relationships between the remaining components were added, including those from: the CLD, identified in the literature, and the transcripts. Fuzzy logic was used to combine the data to inform the AV and weights of the relationships were converted into levels using fuzzy logic (Figure 1); AVs were divided into eight categories that represented the level of the component, and weights were divided into 15 categories. Intercoder reliability (Lavrakas, Reference Lavrakas2008) was assessed for the AV and weights on a subset (10%) of the components (n = 11) and relationships (n = 43). All decisions and deviations from the CLD were documented in a decision matrix (Cousins, Reference Cousins2022b).

Figure 1. An example of how fuzzy logic was used to create the categories for the activation values for the components (and the weights of the relationships) in the fuzzy cognitive map of the development and transmission of antimicrobial resistance in a Swedish One Health system context. Fuzzy logic uses “degree of truth” as opposed to “true or false,” or Boolean logic (0 or 1). Therefore, the degree of membership refers to the relative amount the factor belongs within each category. If the factor belongs fully to a category, it will have a degree of membership of 1.
Components and relationships and their initial AVs and weights were inputted into the software FCM Expert (Nápoles et al., Reference Nápoles, Espinosa, Grau and Vanhoof2018). An inference process was performed and the model reached equilibrium (Lavin and Giabbanelli, Reference Lavin and Giabbanelli2017). Structural measurements of the model were calculated using Mental Modeler and Excel (Cousins, Reference Cousins2022a).
Scenarios
FCMs uses an inference process to simulate a system’s changes over time (Nápoles et al., Reference Nápoles, Espinosa, Grau and Vanhoof2018). The process evaluates the AV of each component based on its relationships at each discrete time step (iteration), leading to three different behaviours, equilibrium, a cyclical state, or total chaotic behaviour (Harmati et al., Reference Harmati, Hatwágner and Kóczy2021). FCM software enables exploration of the system dynamics, pattern recognition, and “what-if” scenarios for decision processes and policy assessment (Liu et al., Reference Liu, Triantis, Zhao and Wang2018; Alipour et al., Reference Alipour, Hafezi, Papageorgiou, Hafezi and Alipour2019; Harmati et al., Reference Harmati, Hatwágner and Kóczy2021). Scenario analyses involved altering the AV of components, reflecting a certain scenario (an intervention), performing an inference process in FCM Expert (Mcculloch and Pitts, Reference Mcculloch and Pitts1990), and calculating percentage change between the AVs for the indicator components (Table 1) at steady state (equilibrium) and the AV from the baseline scenario (inference process conducted with the initial AV of all components). The indicator components were chosen by the research team because they covered many areas important to assessing impacts of interventions to combat AMR and on the broader system from a One Health lens. Therefore, the indicators cover the range of sectors in the One Health spectrum (human, animal, and environment), include important human and animal health indicators (illness in humans, illness in food-producing animals), are important indicators for assessing AMR (AMU and antimicrobial-resistant organisms (AROs) within the various sectors), and were of special interest to the research team (impacts on healthcare costs, cost of food, food security, and trade). Eighteen scenarios were assessed to determine the impact on the AMR system when certain interventions were implemented (outlined in Supplementary Materials). Each scenario was assessed at three intensities: low; medium; and high. These represent the strength of the intervention, and relates to the magnitude of the change in activation value implemented in the model. The different intensities of the scenarios are described in the following naming conventions: low (X.1); medium (X.2); and high (X.3). Therefore, Scenario 10 at the low intensity would be 10.1.
Table 1. List of components (referred to as indicator components) in the fuzzy cognitive map of the emergence and transmission of antimicrobial resistance in a Swedish One Health system used to assess the impacts of various scenarios on the system
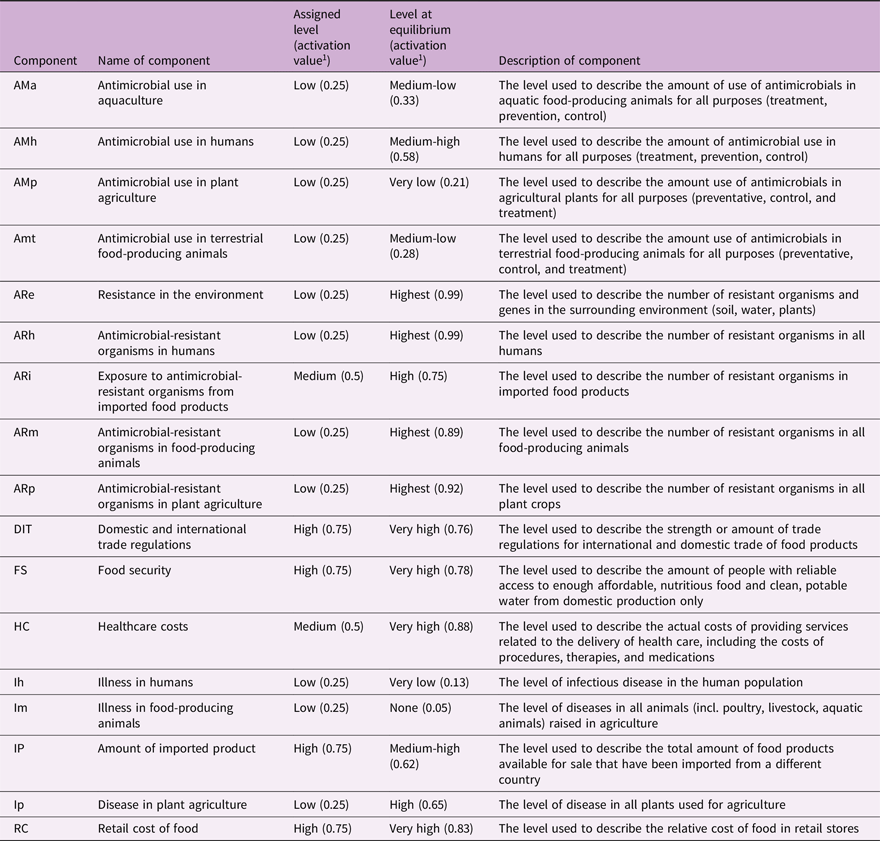
1 Activation values represents the level at which the different drivers (components) of AMR in the Swedish One Health system context exist and were informed by expert opinion and a literature review, and a description of the component. The activation value can take on a value between [0,1] and was divided into eight categories to represent the different levels with the following cut-off values: none (0), very low (0.13), low (0.25), medium-low (0.38), medium (0.5), medium-high (0.63), high (0.75), very high (0.88).
A priori scenarios
Nine scenarios were initially explored representing three interventions under current conditions and a climate change scenario (Table 2). A description of the a priori interventions and the reason for assessing them are outlined in Table 2. Climate change was also implemented into the model to determine how it may impact AMR and the other indicator components as well as to assess the sustainability of the interventions. The a priori scenarios were implemented into the model by altering the AVs of select components and running an inference process. For example, Scenario 1 represented the intervention of increased infection prevention and control. Therefore the AVs for Non-antimicrobial disease prevention and control in health and social care and Non-antimicrobial disease prevention and control in food-producing animal agriculture were increased, and an inference process was performed. The impact of the scenarios were assessed by comparing the AVs of the indicator components at equilibrium to the baseline scenario. A more detailed description of the rationale for the four a priori interventions and climate change are outlined in Supplementary Materials, and details on how they were implemented into the model can be found in Supplementary Materials, Table S1.
Table 2. Description of interventions assessed in a fuzzy cognitive map of the emergence and transmission of antimicrobial resistance in a Swedish One Health system and the reason for including them in the analysis

A posteriori scenarios
It was found that altering the AVs of components and performing an inference process alone were unable to significantly change the system. A significant impact was determined by a difference of greater than 1.0% in the AV of a component at equilibrium from the baseline compared to the scenario being tested. This was done for the a priori interventions and two additional scenarios that altered the AVs of highly impactful nodes; 10 components with the highest centrality, and the components with the highest outdegree, not including indicator components (see Supplementary Materials 2, Table S2). However, the sensitivity analysis (see section 4.3.3), which altered the weights of the relationships, was able to cause significant impacts and therefore new interventions were created aimed at altering the relationships. The experts (Lambrakiet al., Reference Lambraki, Cousins, Graells, Léger, Henriksson, Harbarth, Troell, Wernli, Søgaard Jørgensen, Desbois, Carson, Parmley, Majowicz and Islam2022a,Reference Lambraki, Cousins, Graells, Léger, Abdelrahman, Desbois, Gallagher, Staaf Larsson, Mattson, Henriksson, Troell, Søgaard Jørgensen, Wernli, Carson, Parmley and Majowicz2022b) stressed the importance of attending to the underlying causes (e.g., poverty, inequality) and achieving the sustainable development goals (SDG; Søgaard Jørgensen et al., Reference Søgaard Jørgensen, Wernli and Carroll2016, Reference Søgaard Jørgensen, Folke, Henriksson, Malmros, Troell and Zorzet2020; United Nations Department of Economic and Social Affairs, 2022) as fundamentally critical. Thus, by using the experts’ suggestions (Lambrakiet al., Reference Lambraki, Cousins, Graells, Léger, Henriksson, Harbarth, Troell, Wernli, Søgaard Jørgensen, Desbois, Carson, Parmley, Majowicz and Islam2022a,Reference Lambraki, Cousins, Graells, Léger, Abdelrahman, Desbois, Gallagher, Staaf Larsson, Mattson, Henriksson, Troell, Søgaard Jørgensen, Wernli, Carson, Parmley and Majowicz2022b), and further evidence from other research in AMR (Søgaard Jørgensen et al., Reference Søgaard Jørgensen, Wernli and Carroll2016, Reference Søgaard Jørgensen, Folke, Henriksson, Malmros, Troell and Zorzet2020), four interventions addressing various aspects of the SDGs, were tested under current and climate change conditions (Table 2). These interventions were found to be ineffective at reducing AMR, thus, a final intervention (the “Hail Mary” scenario), which combined all a posteriori interventions was assessed. The details for the rationale for the four a posteriori interventions are outlined in Supplementary Materials and how they were implemented into the model can be found in Supplementary Materials, Table S3.
Sensitivity analysis
Formal sensitivity analyses are not common-place in fuzzy cognitive mapping as the models are typically expert-driven and created through discussion (Lavin and Giabbanelli, Reference Lavin and Giabbanelli2017). However, because there were many assumptions made for the weight values (Cousins, Reference Cousins2022a), an adjusted sensitivity analysis was performed to determine the influence of a subset of the relationships on the system. The outward relationships of the five components with the highest centrality (components with the most incoming and outgoing relationships (Kosko, Reference Kosko1986); that were assigned “medium” as an assumption were chosen for the sensitivity analysis because these components have the most influence within the system. To determine the sensitivity of a set of components of interest (further referred to as indicator components, Table 1) to the selected relationships, the weights of the relationships were adjusted to the 0 and 1 or −1 (see Supplementary Materials, Table S4), and the percentage change for each indicator component was calculated (Figure 2).
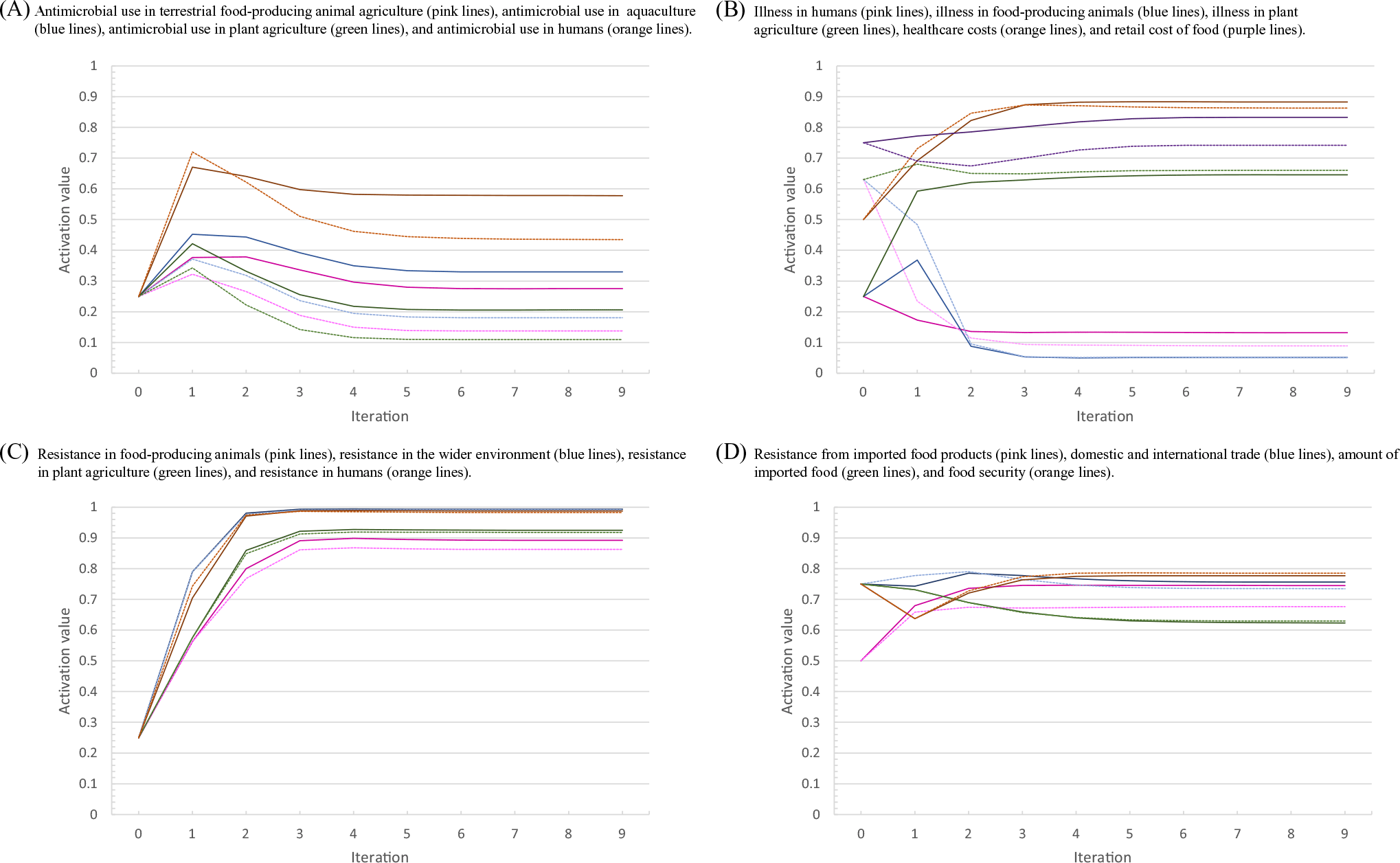
Figure 2. Results of the sensitivity analysis performed on a fuzzy cognitive map of the drivers of antimicrobial resistance in the Swedish One Health system context. The activation values for the indicator variables over the nine iterations of the inference process for the sensitivity analysis with the relationships tested at the lowest possible value (dotted lines) and highest possible value (light solid lines) compared to the baseline (dark solid lines). (a) The activation values for: antimicrobial use in terrestrial food-producing animal agriculture (pink lines), antimicrobial use in aquaculture (blue lines), antimicrobial use in plant agriculture (green lines), and antimicrobial use in humans (orange lines). (b) The activation values for illness in humans (pink lines), illness in food-producing animals (blue lines), illness in plant agriculture (green lines), healthcare costs (orange lines), and retail cost of food (purple lines). (c) The activation values for: resistance in food-producing animals (pink lines), resistance in the wider environment (blue lines), resistance in plant agriculture (green lines), and resistance in humans (orange lines). (d) The activation values for: resistance from imported food products (pink lines), domestic and international trade (blue lines), amount of imported food (green lines), and food security (orange lines).
Results
The final FCM consisted of 90 components with 491 relationships. The components with the highest indegree (number of incoming relationships, ID), outdegree (number of outgoing relationships, OD), and centrality are outlined in Table 3. All network metrics for the FCM are reported in Cousins, Reference Cousins2022a. The model reached equilibrium (as opposed to cyclical or chaotic behaviour) and therefore could be used for scenario analysis (Cousins, Reference Cousins2022a).
Table 3. The nodes with the five highest indegree, 1 outdegree 2 andcentrality 3 from a fuzzy cognitive map of antimicrobial resistance in a Swedish One Health system context, originally created by Cousins, Reference Cousins2022a
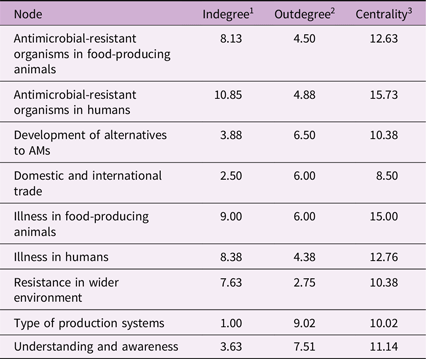
1 Indegree: the number of incoming relationships (+).
2 Outdegree: the number of outgoing relationships (−).
3 Centrality: the absolute value of either: (a) overall influence in the model (all positive (+) and negative (–) relationships indicated, for entire model); or (b) influence of individual concepts as indicated by positive (+) or negative (−) values placed on connections between components.
Scenarios
The AVs for each component during the inference processes during each iteration of the 18 scenarios were published on Borealis (Cousins, Reference Cousins2022b). Visual representations of the AVs for the 17 indicator components during the scenario analyses can be found in Supplementary Materials; includes the eight interventions (Figures S1–17), the high centrality and high outdegree test scenario (Figure S18), the “Hail Mary” scenario (Figure S19), and the sensitivity analysis (Figure 2). The results for the scenarios are described by percentage change in the AV of the given component after an inference process for a scenario compared to the baseline. This change does not represent a numerical value but a way to compare the scenario to the baseline (e.g., a larger percentage change implies a larger impact on the component). To qualitatively describe the magnitude of the relative changes, the following terms were used: significant (any difference >1.0%); slight/minor (>3.0% difference); moderate (3.1%–7.0% difference); large/a lot/greatly (>7.1% difference). The changes in AV are also described in terms of the change in level (e.g., went from “high” to “medium”) based on the cut-off points that were assigned during model building (Figure 1) and these levels must be taken with caution.
Base scenario
The AVs for the 17 indicator components are listed in Table 1 and shown in Figure 2. The AVs for AMU (except for AMU in plant agriculture), AMR, disease in plant agriculture, healthcare costs, retail cost of food, domestic and international trade, food security all reached equilibrium at higher levels than the initial AVs assigned (Table 1). Illness in humans, illness in food-producing agriculture, and amount of imported product had lower levels at equilibrium. In general, if the system was to continue in its current state, then although disease (and thus AMU) will remain very low, there may still be a large increase in AMR to a very high level, which may have trade implications (increase in trade regulations) and economic impacts (increased healthcare costs and cost of food).
A priori interventions, climate change conditions, high centrality, and high outdegree scenarios
The interventions that were created a priori (Supplementary Materials, Figures S1–4,6–9), the climate change scenario (Supplementary Materials, Figures S5), and the high centrality and high outdegree scenarios (Supplementary Materials, Figures S18) had very little impact on the system, with a difference of less than 1.0% in the 17 indicator components at all levels of the intervention.
A posteriori interventions
The relative changes in AV at equilibrium for the indicator components between the baseline scenario and each a posteriori scenario at the highest intensity are depicted in Figure 3. For further detail, the AVs for the indicator components at each time step of the inference processes for the three intensities of each a posteriori scenario are depicted in Supplementary Materials, Figure S10–19.

Figure 3. The relative reduction in the activation value of the indicator components at equilibrium from Scenarios 10 to 13 (A), Scenarios 14 to 17 (B), and Scenario 18 (C). (A) Scenarios 1013 at the highest intensity: Scenario 10 represents a reduction in barrier as a cost for nutritious food and sustainable production practices under current conditions (blue), Scenario 11 represents increased international trade regulations and implantation under current conditions ( pink), Scenario 12 represents technological advancement and innovation under current conditions ( orange), and Scenario 13 represents addressing poverty and social inequalities under current conditions (r green). (B) Scenarios 1417 at the highest intensity: Scenario 14 represents a reduction in barrier as a cost for nutritious food and sustainable production practices under climate change conditions (blue), Scenario 15 represents increased international trade regulations and implantation under climate change conditions ( pink), Scenario 16 represents technological advancement and innovation under climate change conditions ( orange), Scenario 17 represents addressing poverty and social inequalities under climate change conditions ( green). (C) Scenario 18 represents scenarios 10–13 in combination at the highest intensity.
Reducing the cost barrier by a small amount (Scenario 10.1) significantly reduced illness in humans, illness in food-producing animals, and retail cost of food. When the cost barrier was reduced further (Scenario 10.3), there was a significant change in six of the indicator components, causing a reduction in retail cost of food, illness in food-producing animals, illness in humans, AMU in terrestrial animals, AMU in aquaculture, and increase in food security (Figure 3(A)). The largest impact was in retail cost of food, with a reduction from the very high to the high level (16.5% reduction). A moderate reduction in illness in food-producing animals (5.7% reduction) and illness in humans (3.9% reduction) were noticed but did not cause a change to the level at equilibrium.
Increasing trade regulations slightly (Scenario 11.1) significantly reduced AMU in terrestrial food-producing animals, AMU in aquaculture, AMU in plant agriculture, and exposure to AROs from imported food, and a slight increase in illness in food-producing animals. When trade regulations was strengthened further (Scenario 11.3), there were significant changes in ten of the indicator components, causing a reduction in AMU in terrestrial food-producing animals, AMU in plant agriculture, AMU in aquaculture, exposure to AROs from imported food, illness in humans, domestic and international trade regulations, and AROs in food-producing animals, and increases in illness in food-producing animals, retail cost of food, and disease in plant agriculture (Figure 3(A)). The largest impacts were in AMU in agriculture, specifically in AMU in terrestrial food-producing animals (25.0% reduction), AMU in plants (23.3% reduction), and AMU in aquaculture (21.8% reduction). This caused AMU in terrestrial food-producing animals to fall from the medium-low level to the low level. AROs from imported food significantly improved (9.7% reduction) but remained in the high level. A moderate increase to illness in food-producing animals were noticed (5.7% increase), remaining in the very low level.
A small increase in technological advancements (Scenario 12.1) caused a significant reduction in AMU in all sectors (AMU in humans, AMU in terrestrial food-producing animals, AMU in aquaculture, and AMU in plant agriculture), but caused slight increases in illness in humans and illness in food-producing animals. With even more effective technological advancements (Scenario 12.3), significant changes occurred in ten of the indicator components, including reductions in AMU (AMU in humans, AMU in terrestrial food-producing animals, AMU in aquaculture, and AMU in plant agriculture), AROs in food-producing animals, and domestic and international trade regulations, and increases in illness in humans, illness in food-producing animals, disease in plants, and retail cost of food (Figure 3(A)). The largest impacts were seen in AMU in all sectors, with large reductions in AMU in plant agriculture (29.5% reduction), AMU in aquaculture (27.8% reduction), AMU in terrestrial food-producing animals (21.6% reduction), and AMU in humans (20.8% reduction). These reductions caused AMU in aquaculture and AMU in terrestrial food-producing animals to move from a level of medium-low to low, and AMU in humans to move from medium-high to medium. AMU in plant agriculture remained in the low level. There were moderate increases to illness in humans (6.1% increase), moving from the very low to the low level, and illness in food-producing animals (4.7% increase), remaining in the very low level.
Slightly improving social inequalities and poverty (Scenario 13.1) only slightly improved the system through the reduction of AMU in humans, illness in humans, and illness in food-producing animals. However, through further improvements to addressing social inequalities and poverty (Scenario 11.3), greater reductions occurred in not only AMU in humans, illness in humans, and illness in food-producing animals, but reductions were also found in AMU in terrestrial food-producing animals, and healthcare costs (Figure 3(A)). Improving vulnerable populations access to healthcare, social supports, and nutritious food, caused a significant reduction to illness in humans (33.5% reduction). Additional moderate reductions were found in AMU in humans (3.4% reduction) and illness in food-producing animals (3.7% reduction).
The impact of the four interventions at the highest intensity under climate change conditions (Scenario 14.3, 15.3, 16.3, and 17.3) on the 17 indicator components are depicted in Figure 3(B). Overall, climate change conditions did not significantly change how the interventions impacted the system, except for technological advancements and innovation (Scenario 16). At the highest intensity of the intervention (Scenario 16.3), technological advancements and innovation decreased AMU in terrestrial animals by 10% more under climate change than under current conditions (Scenario 12.3) and led to a larger increase in illness in food-producing animals compared to a under current conditions (5.9% increase compared to a 4.7% increase).
The “Hail Mary” Scenario assessed all a posteriori interventions together, under current conditions (Scenarios 10–13). These interventions in combination were able to significantly reduce AMU in all sectors, with the largest reduction seen in AMU in food-producing animals (50.1% reduction, Figure 3(C)), moving from the medium-low to the low level. They were also able to greatly reduce illness in humans (32.6% reduction, Figure 3(C)). However, these interventions were unable to significantly impact most resistant outcomes, aside from AROs from imported foods (9.2% reduction, Figure 3(C)) and AROs in food-producing animals (3.3% reduction, Figure 3(C)). The reduction in AROs in food-producing animals was able to shift the level from highest to the very high, but the reduction did not have an impact on the level of AROs from imported foods, remaining in the high level.
Sensitivity analysis
The sensitivity analysis showed that altering the 10 relationships (Supplementary Materials, Table S4) had varying results on the system, with some components being relatively unaffected (amount of imported food, AROs in imported food, resistance in the environment, food security, and healthcare costs) and some being significantly affected (AMU in terrestrial food-producing animals, AMU in aquaculture, AMU in plant agricultural, and retail cost of food). The results of the inference process for the sensitivity analysis are depicted in Figure 2.
Discussion
This study presents an innovative way to analyse the system of drivers for AMR and uses a systems thinking approach to analyse the effects of interventions to address AMR within the One Health system, including under a climate change scenario. The FCM highlighted the ability of components to influence and be influenced by the system, thus identifying high-leverage factors that when altered, could have great impact on changing the system (Meadows, Reference Meadows1999). High centrality indicates components that are the most interconnected and thus these important factors within the system may be of particular interest when choosing where in the system to act. Many of the components with the highest centrality were expected (such as illness in food-producing animals and illness in humans) as they are typically the target of current intervention strategies (for example increased biosecurity (Niewiadomska et al., Reference Niewiadomska, Jayabalasingham, Seidman, Willem, Grenfell, Spiro and Viboud2019; Pinto et al., Reference Pinto, Keestra, Tandon and CHandler2020; Smith et al., Reference Smith, Coast, Millar, Wilton and Karcher2003), hand washing (Smith et al., Reference Smith, Coast, Millar, Wilton and Karcher2003; Niewiadomska et al., Reference Niewiadomska, Jayabalasingham, Seidman, Willem, Grenfell, Spiro and Viboud2019), or vaccination (Smith et al., Reference Smith, Coast, Millar, Wilton and Karcher2003; Niewiadomska et al., Reference Niewiadomska, Jayabalasingham, Seidman, Willem, Grenfell, Spiro and Viboud2019; Pinto et al., Reference Pinto, Keestra, Tandon and CHandler2020). Similarly, interventions that aim to increase understanding and awareness (another highly connected node) in consumers to reduce the demand for AMs (Wutzke et al., Reference Wutzke, Artist, Kehoe, Fletcher, Mackson and Weekes2007; Azevedo et al., Reference Azevedo, Pinheiro, Yaphe and Baltazar2013; Price et al., Reference Price, Gozdzielewska, Young, Smith, MacDonald, McParland, Williams, Langdridge, Davis and Flowers2018). However, animal welfare, the component with the fourth highest centrality, is not typically the target of intervention The experts from the participatory modelling workshop (Lambrakiet al., Reference Lambraki, Cousins, Graells, Léger, Henriksson, Harbarth, Troell, Wernli, Søgaard Jørgensen, Desbois, Carson, Parmley, Majowicz and Islam2022a) and the ReAct Group, an international network to provide education on AMR (ReAct, 2020), have identified farming systems that enable high levels of animal welfare as a key factor in reducing the need for AMs and may be an important factor missing in current interventions. Components with high ODs are also of interest as these factors have a lot of outward influence on the system. The proportion of alternative production systems (e.g., organic, and antimicrobial free farming), and good farm practices were two components with a lot of outward influence. Alternative production systems may have practices that promote high animal welfare (Carlsson-Kanyama and Lindén, Reference Carlsson-Kanyama and Lindén2001; Mie et al., Reference Mie, Andersen, Gunnarsson, Kahl, Kesse-Guyot, Rembiałkowska, Quaglio and Grandjean2017; The European Food Information Council, 2013), and therefore may also have a large influence on the system.
Scenarios
Baseline model
When the FCM was simulated with the initial AVs, all AMR and many AMU indicators at final equilibrium were much higher than the initial values, especially in humans and the environment. However, it is well documented that AMR in all sectors is quite low in Sweden, especially compared to other countries (Axén et al., 2019; European Food Safety Authority, & European Centre for Disease Prevention and Control, 2020; Nulty et al., Reference Nulty, Soon, Wallace and Nastasijevic2016; The Centre for Disease Dynamics & Economics & Policy, n.d.), and has not been increasing rapidly or in some cases has been decreasing in recent years (Nulty et al., Reference Nulty, Soon, Wallace and Nastasijevic2016; Axén et al., 2019; The Centre for Disease Dynamics & Economics & Policy, n.d.). Therefore, it was concerning that the model predicted rapid and large increases in AMR. This could indicate that some balancing factors may be missing from the system, or that relationships that increase AMR may be too strong, or those that decrease AMR are too weak. One hypothesis is that the workshop aimed to identify and analyse the drivers of AMR (Lambraki et al., Reference Lambraki, Cousins, Graells, Léger, Henriksson, Harbarth, Troell, Wernli, Søgaard Jørgensen, Desbois, Carson, Parmley, Majowicz and Islam2022a). Therefore, many of the factors identified aimed to drive (increase) AMR, but fewer may have been identified to reduce AMR. Overall, the over-estimated AMR levels and potential missing relationships and feedback loops would greatly impact the system behaviour and limit the ability to accurately interpret intervention impacts.
A priori interventions, climate change, high centrality, and high outdegree scenarios
The a priori interventions (including the high centrality and high outdegree scenarios) demonstrated that a change in the AV of a few components were unable to cause system-wide changes. The low system density (Cousins, Reference Cousins2022a) may limit an intervention’s reach within the system due to poor connectivity (Eden et al., Reference Eden, Ackermann and Cropper1993; gray et al., Reference Gray, Gray, Cox and Henly-Shepard2013). Smaller FCMs (8–42 components) prove that AV changes can impact low-density systems (Giles et al., Reference Giles, Findlay, Haas, LaFrance, Laughing and Pembleton2007; gray et al., Reference Gray, Gray, Cox and Henly-Shepard2013). However, large numbers of components may limit an intervention’s reach the outer edges of the system. Experts in the scenario planning workshops (Lambrakiet al., Reference Lambraki, Cousins, Graells, Léger, Abdelrahman, Desbois, Gallagher, Staaf Larsson, Mattson, Henriksson, Troell, Søgaard Jørgensen, Wernli, Carson, Parmley and Majowicz2022b) agreed that simple interventions like taxing AMs alone would not be enough to alter the system. They recommend multi-pronged interventions to address underlying causes of AMR (e.g., poverty, social inequalities, basic hygiene, and access to resources), and a shift in worldviews of the population (e.g., reducing capitalism, prioritizing public health) were essential in reducing AMR (Lambraki et al., Reference Lambraki, Cousins, Graells, Léger, Abdelrahman, Desbois, Gallagher, Staaf Larsson, Mattson, Henriksson, Troell, Søgaard Jørgensen, Wernli, Carson, Parmley and Majowicz2022b).
The way in which climate change was modelled in the FCM, by only altering AVs, caused no significant changes in the system. However, it is hypothesized that climate change will impact AMR and the One Health system that drives AMR in multiple ways, including but not limited to: increasing and emerging diseases in humans, animals, and the environment causing greater transmission, a loss of food production, and a greater need for AMs (World Health Organization, 2017; Rodríguez-Verdugo et al., Reference Rodríguez-Verdugo, Lozano-Huntelman, Cruz-Loya, Savage and Yeh2020; Carlson et al., Reference Carlson, Albery, Merow, Trisos, Zipfel, Eskew, Olival, Ross and Bansal2022); increased migration leading to overcrowding causing poor living conditions (Parry et al., Reference Parry, Rosenzweig and Livermore2005; Semenza and Ebi, Reference Semenza and Ebi2019; Abirham, Reference Abirham2020); increased chronic illnesses and heat stress leading to reduced immunity and increased susceptibility to infectious diseases in humans and animals (World Health Organization, 2017; Rodríguez-Verdugo et al., Reference Rodríguez-Verdugo, Lozano-Huntelman, Cruz-Loya, Savage and Yeh2020; Carlson et al., Reference Carlson, Albery, Merow, Trisos, Zipfel, Eskew, Olival, Ross and Bansal2022). Due to these changes, AMR is predicted to increase under climate change conditions (World Health Organization, 2017; Fouladkhah et al., Reference Fouladkhah, Thompson and Camp2020; Burnham, Reference Burnham2021; Pepi and Focardi, Reference Pepi and Focardi2021). Therefore, this may indicate issues in the FCM (described above) or that it was modelled too simplistically (e.g., climate change may impact relationships as well as AVs). Further research is required to assess the model structure and dynamics as well as to further develop the climate change scenario both with research and with experts to more accurately capture the changes to the system and how they should be inputted into the model.
A posteriori interventions
Reducing cost as a barrier to sustainable food production systems had most impact on reducing illness in food-producing animals. This was most likely due to the impacts of increased animal welfare both directly (through animal-welfare friendly practices) and indirectly (through organic production systems which are more likely to have more animal-welfare friendly practices). There was a correlation between poor animal welfare conditions and stressed animals and a reduction in immunity in these animals (Ashenafi et al., Reference Ashenafi, Yidersal, Hussen, Solomon and Desiye2018; Gunnarsson and Cerenius, Reference Gunnarsson and Cerenius2004; Lambrakiet al., Reference Lambraki, Cousins, Graells, Léger, Henriksson, Harbarth, Troell, Wernli, Søgaard Jørgensen, Desbois, Carson, Parmley, Majowicz and Islam2022a). A potential explanation for how reducing the cost barrier could improve animal welfare and reduce disease in animals based on the relationships that exist in the FCM could be as follows: reducing the cost barrier early in the chain (e.g., reducing the cost of production through subsidies to farmers) could enable farmers to prioritize health interests of their animals, rather than on their practical economic constraints affecting health decisions where these two considerations come into conflict (e.g., the farmer cannot afford to use the best medication and has to choose a more affordable one which might have less efficacy or where antibiotic treatment is cheaper than vaccination). Two positive unintended consequences found through the FCM were a reduction in human illness (due to increased access to nutritious foods) and an increase food security (due to increased yield).
Enhanced diagnostic technology and development of better alternatives to AMs was most effective at reducing AMU in humans, animals, and plants. These interventions specifically targeted AMU, either through better prescribing from enhanced diagnostics or better alternatives. Increasing access to diagnostics has improved prescribing behaviour (Engström et al., Reference Engström, Mölstad, Lindström, Nilsson and Borgquist2004; Llor et al., Reference Llor, Bjerrum, Munck, Cots, Hernández and Moragas2014; O’Neill, Reference O’Neill2015). Therefore, if diagnostics were more widely available and more specific (better at determining organisms), this could greatly improve prescribing and reduce AMU. The development and accessibility of alternatives to AMs (e.g., vaccines, phage therapy) compounded this by also reducing traditional AMU. Vaccines are the most researched alternative to AMs and have been associated with reductions in animals and human AMU (Buckley et al., Reference Buckley, Henschke, Bergman, Skidmore, Klemm, Villanueva, Garritty and Paul2019; Doherty et al., Reference Doherty, Hausdorff and Kristinsson2020).
Increased trade regulations and enforcement of trade regulations was effective at reducing AMU in agriculture and the only intervention to significantly reduce the importation of AROs through food, as restrictions included food with trace AROs or AM residues. This was under the assumption that Sweden would conform to the trade restrictions and reduce their on-farm AMU to remain trading partners with other countries in the European Union. A scenario analysis in the United States of America (USA) from 2011 assessed the trade and economic implications of the USA conforming, or not conforming, to the restrictions on the use of certain AMs in feed for growth promotion and other AM practices (e.g., antimicrobial rinses) some of their largest trading partners (e.g., South Korea and Russia) put in place (Johnson, Reference Johnson2011). This analysis estimated great economic losses if the USA were to conform because the current competitive advantages they hold (low cost of their products) would be reduced (due to increased costs of production), and thus decrease exports (Johnson, Reference Johnson2011). The assumption that Sweden would conform to these regulations is valid as Sweden is less likely to be impacted by these trade regulations compared to the USA due to their existing strict AMU policies (Wierup, Reference Wierup2001; Wierup et al., Reference Wierup, Wahlström and Bengtsson2021), the production systems they have in place (Lambraki et al., Reference Lambraki, Cousins, Graells, Léger, Henriksson, Harbarth, Troell, Wernli, Søgaard Jørgensen, Desbois, Carson, Parmley, Majowicz and Islam2022a, Reference Lambraki, Cousins, Graells, Léger, Abdelrahman, Desbois, Gallagher, Staaf Larsson, Mattson, Henriksson, Troell, Søgaard Jørgensen, Wernli, Carson, Parmley and Majowicz2022b; Wierup et al., Reference Wierup, Wahlström and Bengtsson2021), and their weak reliance on income from exports (World Integrated Trade Solutions, 2022; Lambraki et al., Reference Lambraki, Cousins, Graells, Léger, Henriksson, Harbarth, Troell, Wernli, Søgaard Jørgensen, Desbois, Carson, Parmley, Majowicz and Islam2022a).
Technological advancements and increased trade regulations, however, both caused an increase to the cost of food. The reduction in AMU on-farm could increase production costs through the need for better farm practices and animal welfare (Lambraki et al., Reference Lambraki, Cousins, Graells, Léger, Henriksson, Harbarth, Troell, Wernli, Søgaard Jørgensen, Desbois, Carson, Parmley, Majowicz and Islam2022a). However, when Sweden and Denmark banned AMU for growth promotion, there were limited economic consequences to farmers (Wierup, Reference Wierup2001), and thus may not be as impacted within this context. If cost of food were to increase, however, could cause other negative impacts throughout the system such as inaccessibility to nutritious foods, especially to vulnerable populations, thus impacting health outcomes in these populations (Lambraki et al., Reference Lambraki, Cousins, Graells, Léger, Henriksson, Harbarth, Troell, Wernli, Søgaard Jørgensen, Desbois, Carson, Parmley, Majowicz and Islam2022a). Other negative unintended consequences due to AMU reductions were an increase in illness in animals (from increasing trade regulations and technological advancements) and in humans (from enhanced technological advancements). AMs are necessary for life-saving treatment, and therefore accessibility is required (Government of Canada, 2017).
Reducing negative impacts to vulnerable populations was most effective at reducing human illness and AMU. Vulnerable populations are at higher risk of negative health outcomes and AMR (Elisabeth et al., Reference Elisabeth, Maneesh, Katarina, Slobodan and Michael2021; Planta, Reference Planta2007), and addressing poverty and social inequalities was identified as integral to combatting AMR by experts from within the system (Lambraki et al., Reference Lambraki, Cousins, Graells, Léger, Henriksson, Harbarth, Troell, Wernli, Søgaard Jørgensen, Desbois, Carson, Parmley, Majowicz and Islam2022a, Reference Lambraki, Cousins, Graells, Léger, Abdelrahman, Desbois, Gallagher, Staaf Larsson, Mattson, Henriksson, Troell, Søgaard Jørgensen, Wernli, Carson, Parmley and Majowicz2022b). This intervention was successful at reducing illness and AMU in humans but was the least impactful on broader factors within the system. Some possible explanations for this phenomena could be: 1) the factors and relationships associated with population vulnerabilities and social inequalities were not fully developed; 2) the level of population vulnerabilities, human illness, and human AMU are already so low in Sweden (Cousins et al., Reference Cousins, Parmley, Greer, Neiterman, Lambraki, Vanderheyden, Wernli, Jorgensen, Carson and Majowicz2024) and may cause major issues within the system that reducing these further would not provide large changes to the system; or 3) human-centred interventions may not be enough to shift the system and multi-faceted approaches are required. One positive unintended consequence was a moderate reduction in illness in food-producing animals, most likely due to the relationship between farmers and their ability to care for their animals; healthy farmers (both physically and mentally) provide better care to their animals, thus improving animal welfare and reducing animal illness (Lambraki et al., Reference Lambraki, Cousins, Graells, Léger, Henriksson, Harbarth, Troell, Wernli, Søgaard Jørgensen, Desbois, Carson, Parmley, Majowicz and Islam2022a).
Overall, no intervention had significant impacts on resistance in any sector (humans, animals, or environment). This is an important and not overly surprising result due to the complexity of the system. A study on interventions of climate change, another extremely complex system, found that current interventions to tackle climate change are not quick or deep enough to slow climate change and that “radical” interventions are needed to combat this complex challenge (Morrison et al., Reference Morrison, Adger, Agrawal, Brown, Hornsey, Hughes, Jain, Lemos, McHugh, O’Neill and Van Berkel2022). Further research is needed to brainstorm and assess such “radical” interventions to combat AMR.
The only intervention to reduce AMR greatly in the FCM was enhanced trade regulations, with a reduction in the exposure to AROs from imported food. However, this did not cause a reduction in AROs in humans, meaning this may not be a significant source of resistance in humans in this FCM. Literature is scarce on the relative contribution of imported food to overall resistance in humans (Jung et al., Reference Jung, Morrison and Rubin2022) and further research is required to validate this result.
Increasing trade regulations and technological advancements had the largest impacts on AMR in the FCM. Both caused small reductions to resistance in food-producing animals and technological advancements also had minor impacts on resistance in humans and plant agriculture. However, the modelled outcomes showed that large reductions in AMU lead to minimal changes in AMR, and thus AMU is not a major driver of resistance in this FCM of the Sweden context. One potential explanation for this could be there is already very low AMU and AMR in Sweden (Cousins et al., Reference Cousins, Parmley, Greer, Neiterman, Lambraki, Vanderheyden, Wernli, Jorgensen, Carson and Majowicz2024) and therefore reducing AMU further may not have much impact in this context. This is similar to the minimal impacts that reducing human illness and the level of population vulnerability had on AMR, as these are also very low in this context. However, reducing AMU in different contexts, with differing AMU and AMR levels, may result in larger impacts to AMR. However, in Sweden, interventions aimed to reduce AMU may not be the best place to target action, and focusing on upstream drivers (e.g., improving animal welfare and good farming practices) may provide larger impacts within the system.
Sensitivity analysis
The sensitivity analysis revealed two interesting behaviours of the system. Firstly, by removing or setting to the highest possible value the 10 selected relationships that were highly influential to the system, there was not significant changes in the AMU and AMR components as expected. Therefore, these relationships may have influence in the system but not on AMR or there are other relationships that are more important or “take over” when others are removed.
Secondly, the final activation values were different than the baseline when the relationship weights were altered and thus, to change the final activation values of the components at equilibrium and provide sustainable change over time, the weights of the relationships must be altered, not just the AVs of the components. Therefore, the relationships are important drivers of the system and future work is needed to better define the weights of the relationships through further engagement of stakeholders and a formal scoping review of these associations.
Strengths & limitations
This study highlighted the benefits of FCM in a Swedish One Health system, including socio-ecological drivers from multiple sectors, to assess interventions. Current quantitative models of AMR are limited by data availability, have difficulties capturing real-world behaviour, and may overlook important factors like political forces or human decision-making (Birkegård et al., Reference Birkegård, Halasa, Toft, Folkesson and Græsbøll2018; Ramsay et al., Reference Ramsay, Invik, Checkley, Gow, Osgood and Waldner2018; Cousins, Reference Cousins2022a; Trochim et al., Reference Trochim, Cabrera, Milstein, Gallagher and Leischow2006). Thus, models currently used to assess interventions for AMR are limited in scope and do not include drivers from sectors across the One Health system (e.g., not accounting for potential unintended consequences in the broader system), and contain much uncertainty, thus limiting the ability to adequately assess interventions in the real world. This FCM brings together factors in the human, animal, and environment, and therefore, provided a tool assess multiple scenarios and analyse unintended consequences and unforeseen interactions across sectors under potential climate change conditions (Gray et al., Reference Gray, Gray, Cox and Henly-Shepard2013; Sypher, Reference Sypher2017; Nápoles et al., Reference Nápoles, Espinosa, Grau and Vanhoof2018).
This FCM has limitations inherent to fuzzy cognitive mapping, the way in which the FCM was created (outlined by Cousins, Reference Cousins2022a), and in use for scenario analysis. One limitation was related to the uncertainty in the data used to inform relationships, requiring further engagement with stakeholders (Cousins, Reference Cousins2022a). Exclusive to this study, there are challenges of interpreting intervention outcomes due to the arbitrary time steps and the relative nature of AVs; although AVs are numerical, they do not have an absolute meaning but rather relative ordinal interpretations (Bueno and Salmeron, Reference Bueno and Salmeron2009; Mpelogianni and Groumpos, Reference Mpelogianni and Groumpos2016; Vassiliki Mpelogianni and Groumpos, Reference Mpelogianni and Groumpos2018). Therefore, intervention impacts cannot be quantified (e.g., an intervention reduces the average cost of food from $10 per day to $8 per day) but can be used to compare interventions (e.g., one intervention reduces cost of food more than another). Despite these limitations, fuzzy cognitive mapping provided invaluable insight into system dynamics, identified impactful factors, and allowed comparison of interventions under climate change from a systems perspective.
Conclusion
The use of fuzzy cognitive mapping allowed us to evaluate eight interventions under climate change conditions, of which none of the initial interventions had any impact on the system and no intervention was able to reduce AMR in the system, thus highlighting the complexity of the system that drives AMR. Network analysis of the FCM allowed us to identify influential components for potential future interventions. This study highlighted the need for multi-faceted interventions that target the underlying system, but more work is needed to adequately assess how interventions will impact AMR within the complex system. Finally, this work advocates for further participatory and mixed methods in the exploration of AMR and the complex One Health system that drives it.
Supplementary materials
The supplementary material for this article can be found at https://doi.org/10.1017/one.2024.2.
Data availability statement
The data that support the findings of this study are openly available at the following:
Cousins, M. (2022). Mapping out a One Health model in the context of the Swedish food system using a modified scoping review methodology: Scoping Review Database. Borealis. https://doi.org/10.5683/SP3/UQZJMB
Cousins, M. (2022). Using expert knowledge and experience to parameterize a simulation model of AMR emergence and transmission in a Swedish food system context: Framework Matrices. Borealis, V1. https://doi.org/10.5683/SP3/WUXL5F
Cousins, M. (2022). A One Health and fuzzy cognitive map-based approach to assess interventions to reduce antimicrobial resistance in a Swedish food system context under potential climate change conditions: Decision matrix and Scenario results. Borealis, V2. https://doi.org/10.5683/SP3/Z2IJTE
Acknowledgements
We would like to thank Stephan Harbarth and Max Troell for their involvement on the AMResilience team and inputs into the broader research project for which this is a part of.
Author contributions
Conceptualization: MC, SEM, IAL, EJP, CAC. Data analysis: MC. Visualization: MC. Validation: MC, SEM, EJP, CAC. Funding acquisition: DW, PSJ, SEM. Project administration: IAL, JEP, PSJ, DW, SEM. Supervision: IAL, SEM. Writing of main manuscript: MC. Editorial comments: EJP, ALG, EN, IAL, TG, AL, PJGH, DW, PSJ, CAC, SEM.
Financial support
This study is funded through an operating grant of the 5th Joint Programming Initiative on Antimicrobial Resistance (JPIAMR 2017). Funding was provided by an operating grant from the Canadian Institutes for Health Research (Institute of Infection and Immunity, Institute of Population and Public Health, grant number 155210, PI SEM), a Swedish Research Council grant (grant number 2017-05981, PI and Project Coordinator PSJ); and an operating grant from the Swiss National Science Foundation (grant number 40AR40_180189, PI DW). The funders had no role in the design analysis or writing of this article.
Competing interests
Melanie Cousins and Irene A. Lambraki are employed at the Public Health Agency of Canada. E. Jane Parmley is engaged in research funded by the Canadian Institutes for Health Research, Natural Sciences and Engineering Research Council, Ontario Ministry of Agriculture, Food and Rural Affairs, the Public Health Agency of Canada, and the Canadian Safety and Security Program. She is currently President of the Board of Directors of the Centre for Coastal Health, president of the Canadian Association of Veterinary Epidemiology and Preventive Medicine, member of the Board of Directors of the McEachran Institute, member of the Advisory Council for Research Directions: One Health, and a member of the Royal Society of Canada One Health Working Group. Prior to February 2019, she was employed by the Public Health Agency of Canada. Peter Søgaard Jørgensen was funded via an ERC starting grant: INFLUX, grant number 101039376. He holds/has held grants as principal or co-investigator, from the following agencies and foundations all related to the topics of social-ecological systems and/or AMR: Swedish Research Council FORMAS, Wallenberg Foundations, IKEA Foundation, Erling Persson Family Foundation. Carolee A. Carson is employed by the Public Health Agency of Canada. In that role she has been a subject matter expert for the World Organisation for Animal Health and the World Health Organization (WHO). She is a member of the advisory committee for Animal Health Canada. She has previously been engaged in research funded by the Canadian Institutes for Health Research, Ontario Ministry of Agriculture, Food and Rural Affairs, and the Canadian Safety and Security Program. Patrik JG Henriksson is partially funded by FORMAS Inequality and the Biosphere Project (2020-00454) and partially by CGIAR Trust Fund. Amy L. Greer, Elena Neiterman, Tiscar Graells, Anaïs Léger, Didier Wernli, and Shannon E. Majowicz declare no competing interests.
Ethics statement
This study received ethics clearance from the University of Waterloo’s Research Ethics Committee (ORE# 40,519).
Comments
No accompanying comment.