Introduction
Addiction has now become a concerning public health issue. For instance, about 35 million people were reported to develop substance use disorder (SUD), while approximately 20% of the global population use tobacco products (Fouad et al., Reference Fouad, Commar, Hamadeh, El-Awa, Shen and Fraser2021). Meanwhile, behavioral addictions (BA) have gained prominence in recent years, with an estimated weighted average prevalence of 2.47% for internet gaming disorder (IGD) (Pan, Chiu, & Lin, Reference Pan, Chiu and Lin2020) and 4.5% for pathological gambling disorder (PGD) (Çakıcı, Çakıcı, Babayiğit, & Karaaziz, Reference Çakıcı, Çakıcı, Babayiğit and Karaaziz2021). In addition, increasing addiction patients have been found to suffer from comorbid psychiatric disorders. For example, dysfunctions of emotion regulation are frequently experienced by individuals with addiction (Estévez, Jáuregui, Sánchez-Marcos, López-González, & Griffiths, Reference Estévez, Jáuregui, Sánchez-Marcos, López-González and Griffiths2017). Further, although numerous treatment techniques have been developed for the intervention of addiction in recent years, the clinical treatment outcomes still need further improvements, since addiction is a complex and multifaceted disease that interacts with neurobiological, environmental, cognitive, and genetic factors (Sutherland, McHugh, Pariyadath, & Stein, Reference Sutherland, McHugh, Pariyadath and Stein2012). Therefore, it is essential to inspect the neuro-cognitive mechanism of BA and SUD, which might pave a new avenue for improving individual-specific treatment strategies and enhancing the efficacy of intervention.
Interestingly, BA and SUD share abundant identical or similar characteristics in diagnostic criteria including clinical symptoms, cognitive data, neurobiology measurements, and neuroimaging features. In particular, these similar clinical symptoms exhibit significant relationships with the shared cognitive and physiological alternations (Verdejo-Garcia, Garcia-Fernandez, & Dom, Reference Verdejo-Garcia, Garcia-Fernandez and Dom2019). For example, both the two types of addictions demonstrated the alterations in sensation seeking, inhibition control, attentional bias, etc. (Jentsch & Pennington, Reference Jentsch and Pennington2014; Tatari et al., Reference Tatari, Farnia, Momeni, Davarinejad, Salemi, Soltani and Alikhani2020; Weinstein, Abu, Timor, & Mama, Reference Weinstein, Abu, Timor and Mama2016; Zhao et al., Reference Zhao, Li, Hu, Li, Gillebert, Mantini and Liu2017), whereas those shared cognitive changes in BA and SUD showed correlations with the abnormal functions of neural circuits in reward, response inhibition, and salience attribution (Bari & Robbins, Reference Bari and Robbins2013; Gardner, Reference Gardner2011).
Besides, SUD and BA also exhibit distinct patterns of cognitive function changes, due to the specific neurotoxic profiles and neurotransmitter systems of consumed substance in SUD. For example, the addiction in BA is unpredictable, requiring the participation of learning (Clark, Boileau, & Zack, Reference Clark, Boileau and Zack2019). By contrast, SUD involves the substance in an automatic way, irrespective of the consequences (Perales et al., Reference Perales, King, Navas, Schimmenti, Sescousse, Starcevic and Billieux2020). Meanwhile, sensation seeking in SUD has consistently demonstrated a robust correlation with addiction degree (Acheson, Reference Acheson2020), whereas in BA, this association seems to be less consistent, with studies reporting weak positive (Tsilosani, Chan, Steffens, Bolton, & Kowalczyk, Reference Tsilosani, Chan, Steffens, Bolton and Kowalczyk2023; Velezmoro, Lacefield, & Roberti, Reference Velezmoro, Lacefield and Roberti2010) and negative association (Mesgarani, Shafiee, Ahmadi, & Zare, Reference Mesgarani, Shafiee, Ahmadi and Zare2013). This variability may be attributed to the fact that sensation seeking in BA likely arises from a combination of genetic and environmental influences (Chen et al., Reference Chen, Sun, Ai, Li, Lu, Shi, Zhang, Shi and Tao2017). Likewise, regarding psychosocial factors, BA and SUD demonstrated significant differences in social support, tolerance of physical discomfort, reappraisal, and cognitive confidence (Akbari et al., Reference Akbari, Bahadori, Mohammadkhani, Kolubinski, Nikcevic and Spada2021). More importantly, a body of prior neuroimaging research has illuminated that the varying patterns of changes observed in clinical symptoms, cognitive functions, and behavioral attributes across diverse psychiatric disorders, including addiction, consistently correlate with discernible anomalies in functional network communications (Anticevic et al., Reference Anticevic, Gancsos, Murray, Repovs, Driesen, Ennis and Corlett2012; Koziol, Barker, Joyce, & Hrin, Reference Koziol, Barker, Joyce and Hrin2014; Menon, Reference Menon2011). This substantial body of evidence underpins the notion that distinctive alterations in behaviors, cognition, and neurotransmitter systems within BA and SUD are intrinsically linked to divergent modifications in brain system dynamics.
Resting-state functional connectivity (rs-FC) can be applied to inspect the abnormalities of large-scale intrinsic functional networks associated with different brain disorders (Lv et al., Reference Lv, Wang, Tong, Williams, Zaharchuk, Zeineh and Wintermark2018; van den Heuvel & Hulshoff Pol, Reference van den Heuvel and Hulshoff Pol2010). In particular, seed-based analysis method is widely used to construct the brain networks, which is able to measure the correlations of time series between a seed region-of-interest (ROI) and the target regions (Smitha et al., Reference Smitha, Akhil Raja, Arun, Rajesh, Thomas, Kapilamoorthy and Kesavadas2017; van den Heuvel & Hulshoff Pol, Reference van den Heuvel and Hulshoff Pol2010). Extensive rs-FC studies illustrated that both SA and BA showed the disrupted functional connectivity in default mode network (DMN), frontoparietal network (FPN), and salience network (SN) as well as the abnormal interaction between these networks (Cooper, Robison, & Mazei-Robison, Reference Cooper, Robison and Mazei-Robison2017; Gardner, Reference Gardner2011; Jiang et al., Reference Jiang, Sun, Zhou, Lu, Li, Huang and Gong2021; Solinas, Belujon, Fernagut, Jaber, & Thiriet, Reference Solinas, Belujon, Fernagut, Jaber and Thiriet2019). However, there are remaining controversies regarding the findings from various neuroimaging studies at rest for both BA and SUD. For example, stronger functional connectivity within DMN was detected for BA (Chen et al., Reference Chen, Wang, Zhou, Sun, Ding, Zhuang and Du2014; Ding et al., Reference Ding, Sun, Sun, Zhou, Li, Xu and Du2013) while Zhang et al. (Reference Zhang, Ma, Yip, Wang, Chen, Yan and Fang2015) demonstrated the weaker one was associated with BA (Zhang et al., Reference Zhang, Ma, Yip, Wang, Chen, Yan and Fang2015). Besides, Bae, Han, Jung, Nam, and Renshaw (Reference Bae, Han, Jung, Nam and Renshaw2017) illustrated strong FPN rs-FC, whereas Ge et al. (Reference Ge, Sun, Han, Wang, Ding, Cao and Zhou2017) discovered weak rs-FC between FPN and SN in IGD (Bae et al., Reference Bae, Han, Jung, Nam and Renshaw2017; Ge et al., Reference Ge, Sun, Han, Wang, Ding, Cao and Zhou2017). Further, Halcomb, Chumin, Goñi, Dzemidzic, and Yoder (Reference Halcomb, Chumin, Goñi, Dzemidzic and Yoder2019) reported increased rs-FC between SN and the inferior frontal gyrus (IFG) while decreased rs-FC between SN and the cerebellum for the SUD group as compared to those from the healthy control (HC) group (Halcomb et al., Reference Halcomb, Chumin, Goñi, Dzemidzic and Yoder2019). By contrast, Verdejo-Garcia et al. (Reference Verdejo-Garcia, Contreras-Rodríguez, Fonseca, Cuenca, Soriano-Mas, Rodriguez and de la Torre2014) demonstrated that no difference was detected between the SUD and HC groups when adopting the insula as seeds (Verdejo-Garcia et al., Reference Verdejo-Garcia, Contreras-Rodríguez, Fonseca, Cuenca, Soriano-Mas, Rodriguez and de la Torre2014). Those conflicting findings enable us to carry out qualitative analysis and distinguish well between the functional connectivity of BA and SUD, compared to HC. It was found that the inconsistent findings in the large-scale network changes between BA and SUD might be due to: (1) the locations and sizes of seed ROI are different for various studies, making it extremely hard to integrate those findings, and (2) the sample size is not large enough and heterogeneities also exist across each individual studies, caused by the differences in age, gender proportion, addiction degree, etc. Therefore, it is essential to integrate and analyze those work to reveal the differences and similarities in neural findings between BA and SUD.
Meta-analysis has the capability to inspect the inconsistencies among previously published studies and find out the consistent results. To date, Tolomeo and Yu (Reference Tolomeo and Yu2022) have integrated the seed-based rs-FC studies of BA and SUD, and detected the hyper-connectivity in putamen for both BA and SUD groups relative to that of the HCs (Tolomeo & Yu, Reference Tolomeo and Yu2022). However, since the brain regions work in synchronization with other ones either in a task or resting state (Brandl et al., Reference Brandl, Avram, Weise, Shang, Simões, Bertram and Sorg2019; Power, Goodyear, & Crockford, Reference Power, Goodyear and Crockford2012), their results in a scale of brain regions may not be sufficient to reveal the brain alternations of BA and SUD, and might bring confounds to some extent. For instance, different studies have reported either increased or decreased FC between different brain regions belonging to different networks and precuneus in the SUD relative to HCs (Blanco-Hinojo et al., Reference Blanco-Hinojo, Pujol, Harrison, Macià, Batalla, Nogué and Martín-Santos2017; Zou et al., Reference Zou, Wu, Zhai, Lei, Shao, Jin and Yang2015), which might mislead the FC results of the precuneus in meta-analysis since only brain-region-based FC was reported. In addition, due to the limited spatial resolution of fMRI and a group of neurons may execute multiple functions, same brain regions may fall in different networks simultaneously. Therefore, it might be problematic to only report abnormal FC at the level of brain regions, which cannot anchor to the corresponding pathway dysfunctions of the patients. Moreover, the relationships between the clinical properties of the patients and brain alternations have not yet been explored in the existing meta-analysis (Tolomeo & Yu, Reference Tolomeo and Yu2022), which are important to reveal the underlying neuro-mechanism of the aberrant behaviors in the patients. Meanwhile, it is necessary to conduct control analyses such as heterogeneity tests, sensitivity analyses, etc., as so to gauge the reliability and robustness of results.
To address these limitations, we conducted a novel meta-analysis for seed-based rs-FC studies of large-scale networks to inspect the common and distinct patterns of brain network abnormalities between SUD and BA. In particular, the functional connectivity was categorized into seven networks, namely, the DMN, FPN, SN, dorsal attention network (DAN), affective network (AN), somatosensory network (SSN), and visual networks (VN) (Buckner, Krienen, Castellanos, Diaz, & Yeo, Reference Buckner, Krienen, Castellanos, Diaz and Yeo2011; Choi, Yeo, & Buckner, Reference Choi, Yeo and Buckner2012; Shirer, Ryali, Rykhlevskaia, Menon, & Greicius, Reference Shirer, Ryali, Rykhlevskaia, Menon and Greicius2012; Smith et al., Reference Smith, Fox, Miller, Glahn, Fox, Mackay and Beckmann2009; Yeo et al., Reference Yeo, Krienen, Sepulcre, Sabuncu, Lashkari, Hollinshead and Buckner2011). It is noted that the SN corresponds to the ‘ventral attention network’ and AN refers to ‘limbic network’, due to its alignment with the pronounced salience attraction and emotion regulation roles. Our meta-analysis aims to: (1) examine the rs-FC abnormalities in BA and SUD as compared to those from HCs, (2) explore the common and distinctive patterns of networks in functional connectivity between BA and SUD, and (3) establish the relationship between brain network dysfunctions and clinical characteristics in BA and SUD. It is expected that our findings will pave a new avenue for improved understanding of the cognitive neural mechanism and for the detection and treatment of BA and SUD.
Methods
This review was conducted in accordance with the Preferred Reporting Items for Systematic Reviews and Meta-Analyses (PRISMA) guidelines (Moher, Liberati, Tetzlaff, & Altman, Reference Moher, Liberati, Tetzlaff and Altman2009), registered in the Prospero database (CRD42020210835).
Literature search strategy
Four electronic databases, including PubMed, OVID Embase, OVID Medline, and Web of Science, were searched for papers published in English as of June 2021 that were associated with rs-FC networks of addictions. The search terms for SUD contained ‘alcohol’ OR ‘cigarette’ OR ‘cocaine’ OR ‘cannabis’ OR ‘amphetamine’ OR ‘methamphetamine’ OR ‘heroin’ OR ‘substance use disorders’ OR ‘dependence’, while those for BA consisted of ‘internet addiction’ OR ‘internet gaming’ OR ‘gambling’ OR ‘addictive behavior’. Meanwhile, the neuroimaging terms searched were ‘functional magnetic resonance imaging’ OR ‘functional MRI’ OR ‘fMRI’. The whole search strategies and keywords were respectively presented in the appendix. Additional meta-analysis databases, such as BrainMap and Neurosynth, were also searched as supplements.
Inclusion criteria and study selection
Publications selected for this meta-analysis were original case-control fMRI studies using whole-brain seed-based rs-FC to compare the findings between various categories of addiction (diagnosed according to the DSM-IV, DSM-5, or ICD-10 criteria) with HCs and reporting coordinates in Montreal Neurological Institute (MNI) or Talairach (TAL) space. However, the following studies should be excluded: (1) no HC group, (2) no peak effect coordinates or the center of seed ROIs except that the study had reported the network to which the seed belonged, and (3) overlapping samples using the same seed ROIs for different studies. In particular, publications that utilized the same sample with different seed ROIs were coded as separate datasets. In addition, if the studies compared the results between multiple types of addictions (e.g. IGD and gambling) with the same HC samples, these comparisons were considered as separate contrasts (Brandl et al., Reference Brandl, Avram, Weise, Shang, Simões, Bertram and Sorg2019). Two members (ZX and HX) reviewed the studies independently based on the abovementioned criteria and reached a consensus for the analysis in the end.
Data extraction
The data were then extracted and analyzed as follows: (1) coordinates of the center (mass of each seed ROI) or peak effect coordinates with demonstrated group difference were extracted, and (2) the seed ROIs were categorized into seven predefined resting-state functional networks based on the coordinates, including DMN, FPN, VAN, DAN, AN, SSN, and the VN (Buckner et al., Reference Buckner, Krienen, Castellanos, Diaz and Yeo2011; Choi et al., Reference Choi, Yeo and Buckner2012; Shirer et al., Reference Shirer, Ryali, Rykhlevskaia, Menon and Greicius2012; Smith et al., Reference Smith, Fox, Miller, Glahn, Fox, Mackay and Beckmann2009; Yeo et al., Reference Yeo, Krienen, Sepulcre, Sabuncu, Lashkari, Hollinshead and Buckner2011). Thalamus is an important hub for the study of the cognitive neural mechanism of addiction, yet the ROIs of thalamus are out of the seven pre-defined resting-state functional networks. As such, thalamus was considered as an independent seed network in the current analysis. Furthermore, hyperconnectivity was defined as the increased positive and the decreased negative rs-FC in addiction, as compared to that of HCs (addiction > HCs), whereas hypoconnectivity was denoted as the decreased positive and the increased negative rs-FC (addiction < HCs). Additionally, the results showing no group difference in whole-brain seed-based rs-FC between the addiction and HC groups were also included in the meta-analysis as an independent category.
Meta-analysis
The seed-based d mapping (SDM) software package (5.15 version, https://www.sdmproject.com/software/) was used for the meta-analysis, which is a statistical analysis tool to integrate fMRI studies to generate the results regarding different brain activities and structural information changes (Radua et al., Reference Radua, Mataix-Cols, Phillips, El-Hage, Kronhaus, Cardoner and Surguladze2012). For this method, seed center coordinates and peak coordinates with between-group differences were firstly extracted from each study, which need be converted to MNI coordinates if reported in Talairach space. Then the effect-size map between the addiction and HC groups was regenerated for each study via an anisotropic unnormalized Gaussian kernel, in which the voxel closer to the peak coordinates was assigned a higher value (Radua et al., Reference Radua, Rubia, Canales-Rodríguez, Pomarol-Clotet, Fusar-Poli and Mataix-Cols2014). Further, voxel-wise meta-analytic computation was carried out for the random effect of study maps, which were weighted by the sample size, intra-study variance, and inter-study heterogeneity (Salimi-Khorshidi, Smith, Keltner, Wager, & Nichols, Reference Salimi-Khorshidi, Smith, Keltner, Wager and Nichols2009). To reduce the false positive rate, a conservative threshold was chosen, in which only abnormalities with a voxel-level (height) threshold of p < 0.001 with a peak Z > 1 and a cluster-level (extent) threshold of 100 contiguous voxels were reported (Radua et al., Reference Radua, Mataix-Cols, Phillips, El-Hage, Kronhaus, Cardoner and Surguladze2012; Tang et al., Reference Tang, Lu, Zhang, Hu, Bu, Li and Huang2018). The spatial correlation analysis between the effect cluster and predefined networks was used to categorize the effect clusters into different networks. Bedsides, the networks defined by Shirer et al. (Reference Shirer, Ryali, Rykhlevskaia, Menon and Greicius2012) and Smith et al. (Reference Smith, Fox, Miller, Glahn, Fox, Mackay and Beckmann2009) were also used as template references to improve the classification accuracy of brain networks (Shirer et al., Reference Shirer, Ryali, Rykhlevskaia, Menon and Greicius2012; Smith et al., Reference Smith, Fox, Miller, Glahn, Fox, Mackay and Beckmann2009).
In particular, we also examined the whole-brain differences in rs- FC alterations between SUD and BA by calculating the differences of each voxel between the two groups and determining its statistical significance with a randomization test. Likewise, conjunction analysis was then performed to inspect the shared abnormalities in regions and networks across both addiction groups relative to HCs. The p values of common network alterations in both groups were calculated by using the two probability maps of obtained separate meta-analyses within each voxel: pcommon = pBA + pSUD-pBA*pSUD. Accounting for noise in the estimation of meta-analytic p values, a conservative threshold with a voxel level of p < 0.001 and cluster size > 100 contiguous voxels were used for contrast and conjunction analysis (Radua, Romeo, Mataix-Cols, & Fusar-Poli, Reference Radua, Romeo, Mataix-Cols and Fusar-Poli2013).
Complementary analysis
To access the reliability and robustness of the results and reduce the potential confounding moderators, five complementary analyses were conducted for each seed-based network:
(a) Subgroup analysis: Considering different neurotoxic effects on the brain and neurobiological mechanisms of SUD, sub-group analysis was adopted to examine the contrast rs-FC alterations caused by stimulants and depressants. In our study, the stimulants include caffeine, nicotine, amphetamines, methamphetamine, and cocaine, while the depressants include alcohol, heroin, opioid, and codeine.
(b) Heterogeneity test: Inter-study heterogeneity was calculated by using the Q value. Clusters were considered as heterogeneous among studies if they showed significant heterogeneity (I 2 > 50%) when the results were extracted for a comprehensive meta-analysis.
(c) Meta-regression (Radua & Mataix-Cols, Reference Radua and Mataix-Cols2009): Several demographic and clinical data were used as regressors to inspect their effects on rs-FC, including the mean age and gender proportion for all addictions, depression, anxiety, impulsivity, severity for BA, and substance use onset year and duration for SUD. The scale scores for depression, anxiety, impulsivity, and addictive severity were converted to standard centesimal grades according to the score range in each scale (Qiu & Wang, Reference Qiu and Wang2021). Likewise, to reduce the false positive rate, a voxel-level threshold of p < 0.0001 and a cluster-level threshold of 100 contiguous voxels were chosen.
(d) Sensitivity analyses: Jackknife sensitivity analyses were used to examine the reliability and stability of all main findings by repeating the analyses while discarding one dataset each time (Radua & Mataix-Cols, Reference Radua and Mataix-Cols2009). The results can be concluded as highly replicable if a certain effect remains significant in all or most of the repeats.
(e) Publication bias (Lin & Chu, Reference Lin and Chu2018): Since positive outcomes were more likely to be published than negative ones, publication bias was inspected by using Egger's test with the threshold of p < 0.05 when more than 10 studies were included in the meta-analysis.
Results
Literature search and study characteristics
A total of 1990 studies were retrieved during the initial electronic search from the four databases after removing 1115 duplicated studies automatically and 795 studies through title, abstract, and full-text review. A total of 84 studies with 92 datasets (eight studies containing three study groups) were included for the following analysis, involving 2444 patients with addictions (1143 with BA and 1510 with SUD) and 2712 HCs. For the included studies, the average age of patients with addiction was 29.41 years old (BA: 22.14; SUD: 35.13) and that of HCs was 29.07. It was discovered that no significant difference was detected in age between the addiction and HC groups (online Supplementary Table S3 and Table S4). And then 167 seed ROIs from the abovementioned seven networks were extracted from the 92 datasets consisting of 39 BA and 54 SUD datasets (one dataset had both BA and SUD subgroups). In addition, 57 seeds belonging to DMN, 23 seeds to FPN, 41 seeds to AN, and 29 seeds to SN were included in the quantitative meta-analysis. By contrast, seeds in SSN, DAN, and VN were insufficient (less than five) to make the meta-analysis (online Supplementary Table S2). Information about the methodologies of fMRI equipment and rs-FC analysis were listed in the Supplementary Materials (Table S5), illustrating acceptable consistency although some divergences exist.
Functional network alterations in both groups of BA and SUD
Interestingly, the patients with addictions demonstrated both within- and between-network rs-FC alterations as compared to the HCs (Fig. 1a, Table 1). For within-network FC alterations, addiction groups were characterized by hypoconnectivity between the DMN seeds and a cluster of anterior thalamic projections (ATP) extending to the thalamus region. However, no significant within-network hyperconnectivity was detected for the patient groups.
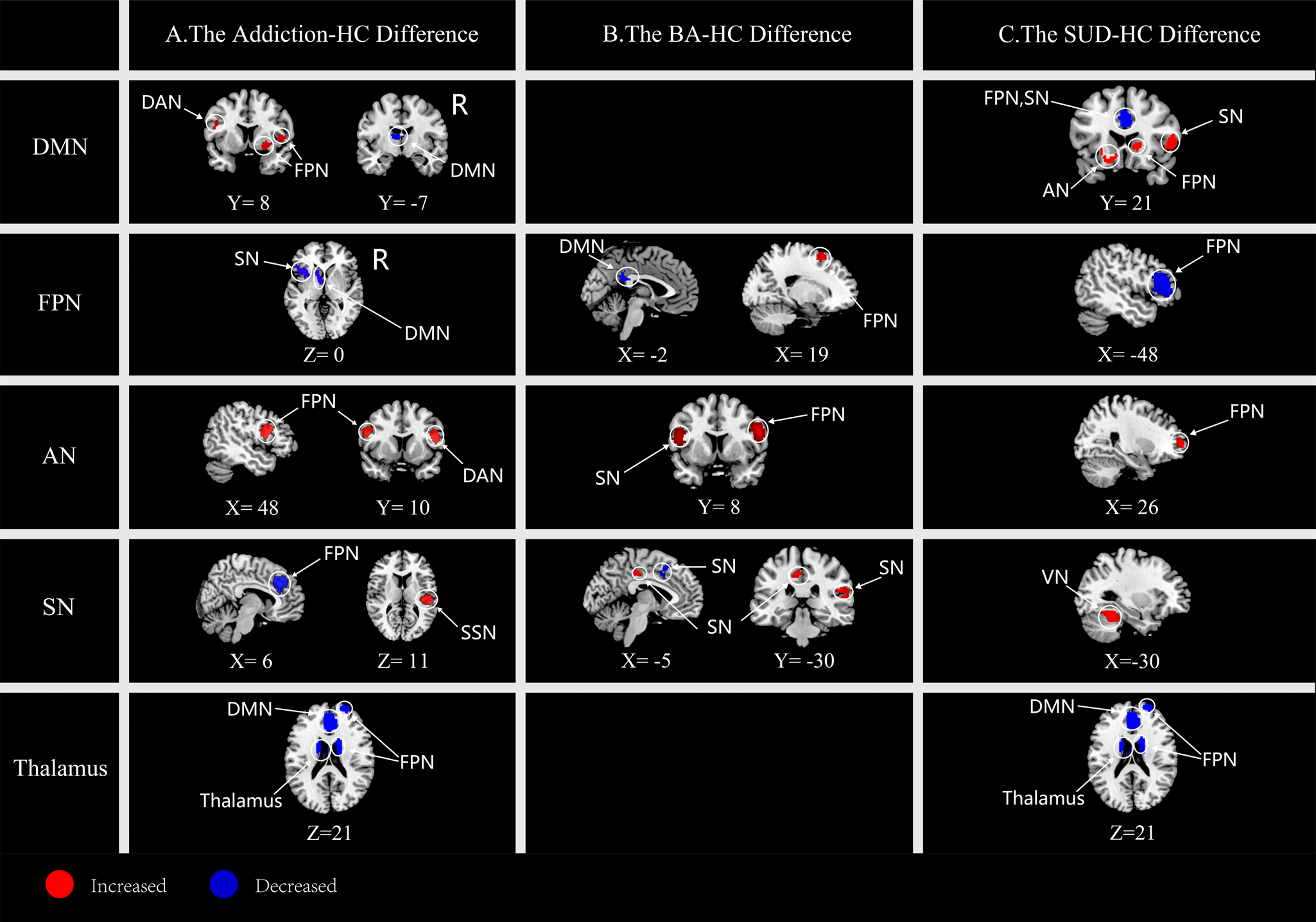
Figure 1. Comparisons in rs-FC findings between both types of addiction (a), behavioral addictions (b), substance use disorder (c), and HC groups. The first column denotes the seed-belonging networks. AN, affective network; DAN, dorsal attention network; DMN, default mode network; FPN, frontal parietal network; SSN, somatosensory network; SN, salience network; BA, behavioral addiction; SUD, substance use disorder.
Table 1. Aberrant intrinsic functional connectivity in behavioral and substance use disorder as compared to that of healthy controls
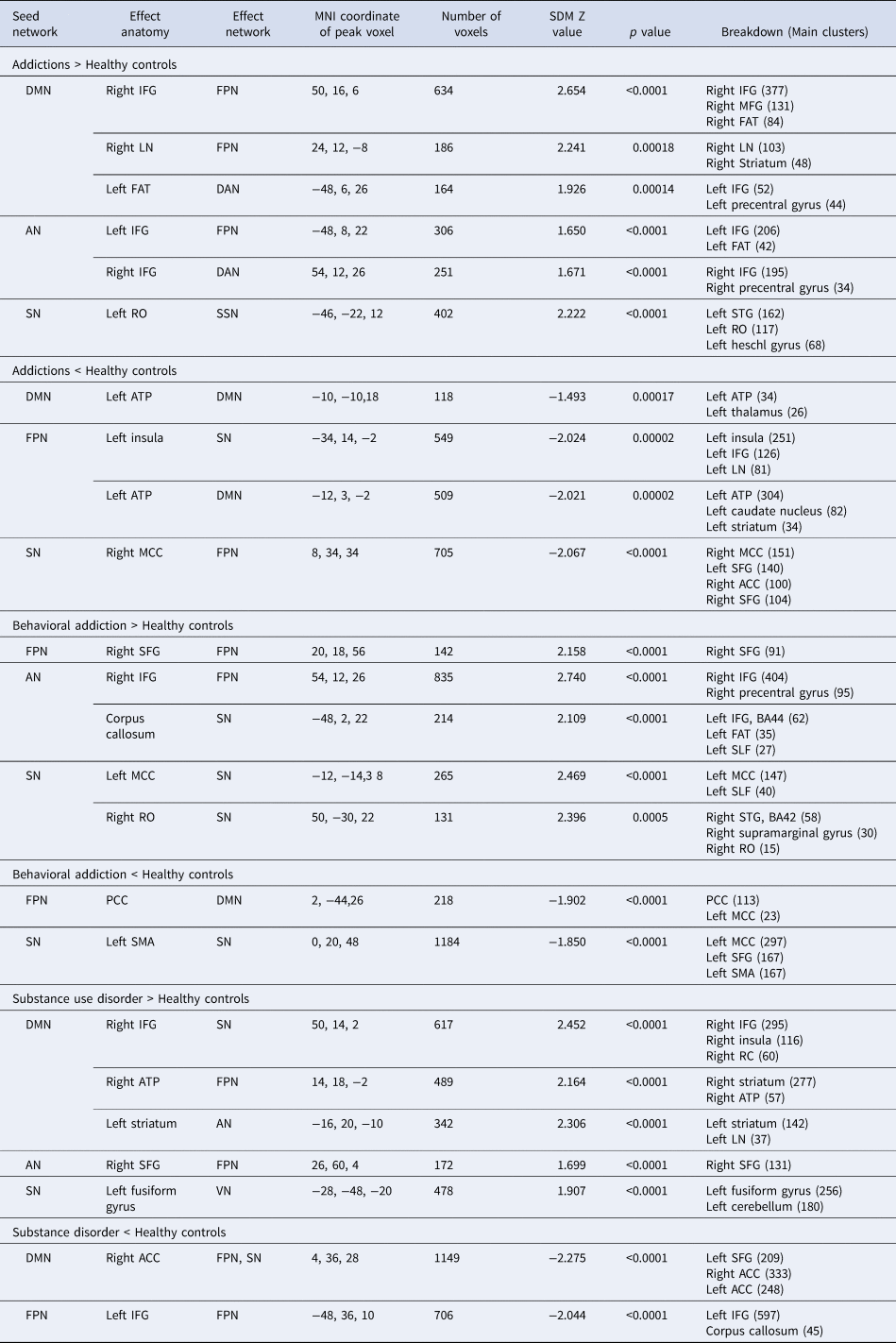
ATP, anterior thalamic projections; FAT, frontal aslant tract; IFG, inferior frontal gyrus; LN, lenticular nucleus; MCC, median cingulate cortex; MFG, middle frontal gyrus; RO, Rolandic operculum; SFG, superior frontal gyrus; SLF, superior longitudinal fasciculus; STG, superior temporal gyrus; AN, affective network; DAN, dorsal attention network; DMN, default mode network; FPN, frontal parietal network; SSN, somatosensory network; SN, salience network.
Regarding the between-network alterations, addiction groups demonstrated several rs-FC alterations, such as hyperconnectivity: (1) between DMN seeds with the clusters within the right IFG and the right lenticular nucleus in FPN and a cluster in the left IFG in DAN, (2) between AN seeds with the left IFG in FPN and the right IFG in DAN, and (3) between SN seeds and the cluster centered in the left Rolandic operculum (RO) in SSN.
Besides, between-network hypoconnectivity was revealed in addition groups as compared to that of the HC groups, including the hypoconnectivity: (1) between FPN seeds and the left insula in SN and a cluster of the left ATP extending to caudate in DMN, and (2) between SN seeds and the cluster of the right median cingulate cortex (MCC) extending to anterior cingulate cortex (ACC) belonging to DMN and superior frontal cortex (SFG) belonging to FPN.
Functional network alterations in BA
Compared with the HC group, patients with BA were characterized by within-network hyperconnectivity in FPN between FPN seeds and the right SFG as well as in SN between SN seeds and regions of the right RO and the left MCC. Meanwhile, regarding BA, within-network hypoconnectivity was also detected in SN between SN seeds and the left supplementary motor area (SMA). In addition, the BA patient group showed between-network hyperconnectivity between AN seeds with the right IFG in FPN and corpus callosum in SN as well as hypoconnectivity between FPN seeds and areas of the corpus callosum in DMN (Fig. 1b, Table 1).
Functional network alterations in SUD
SUD demonstrated within-network hypoconnectivity in the thalamus and in FPN between FPN seeds and left IFG regions as compared to HC group. In addition, SUD showed between-network hyperconnectivity between DMN seeds, the right IFG extending to insula in SN, the right ATP extending to striatum in FPN, and the left striatum in AN. SUD also demonstrated between-network hyperconnectivity between AN seeds and the right SFG of FPN (Fig. 1c, Table 1). By contrast, SUD showed hypoconnectivity between SN seeds and the left fusiform gyrus in VN, between the thalamus and clusters in the right ACC extending to the MCC in DMN, and between regions of the right SFG and the right middle frontal gyrus (MFG) in FPN.
To date, no whole-brain seed-based FC studies of BA that use the thalamus as seeds have been carried out. To the best of our knowledge, this was the best study that illustrated the results of voxel wise thalamic FC alternations in SUD.
Common alterations in functional connectivity networks for BA and SUD
The conjunction analyses showed that BA and USD shared a bunch of common significant rs-FC alterations (Fig. 2 Right, Table 2). For example, hyperconnectivity was detected: (1) between DMN seeds and FPN (the right IFG extending to lenticular nucleus) and SN (insula), (2) between AN seeds and FPN (left IFG), and (3) between SN seeds and SSN (the cluster of left RO extending to superior temporal gyrus (STG)).
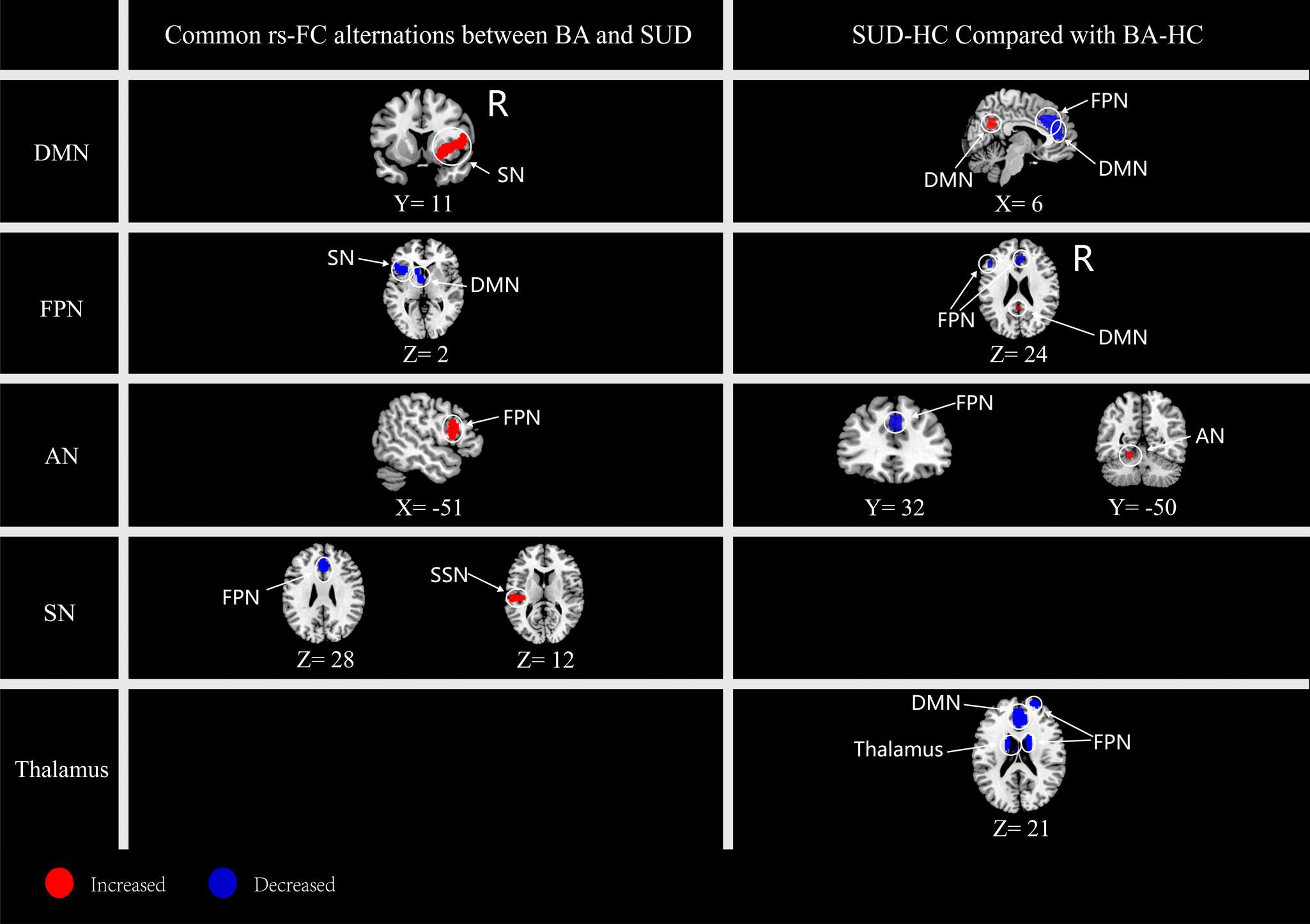
Figure 2. Common (left panel) and distinct (right panel) alteration patterns in rs-FC network between BA and SUD groups. The first column denotes the seed-belonging networks. AN, affective network; DAN, dorsal attention network; DMN, default mode network; FPN, frontal parietal network; SSN, somatosensory network; SN, salience network; BA, behavioral addiction; SUD, substance use disorder.
Table 2. Common alterations of intrinsic functional connectivity between behavioral addiction and substance use disorder
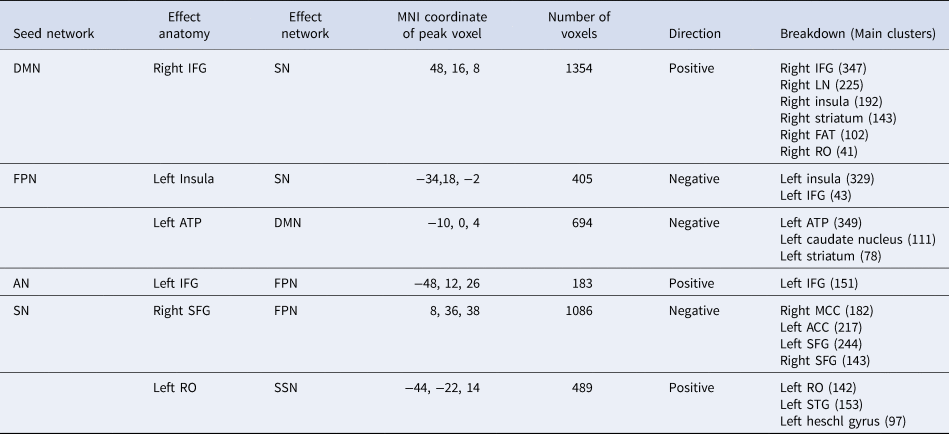
ATP, anterior thalamic projections; IFG, inferior frontal gyrus; RO, Rolandic operculum; SFG, superior frontal gyrus; STG, superior temporal gyrus; AN, affective network; DMN, default mode network; FPN, frontal parietal network; SSN, somatosensory network; SN, salience network.
In addition, hypoconnectivity was identified: (1) between FPN seeds with the ATP clusters in DMN and the left insula in SN, and (2) between SN seeds and the cluster centered in the right SFG extending to ACC, MCC, belonging to FPN.
Differences in functional connectivity networks between BA and SUD
To inspect the distinct alteration patterns of functional connectivity networks between BA and SUD, the contrast analysis with age as the covariate was carried out. It was discovered that SUD showed several within-network FC alterations as compared to BA, including hyperconnectivity: (1) within DMN between DMN seeds and the right posterior cingulate cortex (PCC), and (2) within AN between AN seeds and the left cerebellum. Additional hypoconnectivity were also detected: (1) within FPN between FPN seeds and regions of the left IFG and right ACC, and (2) within DMN between DMN seeds and MCC extending to ACC and SFG.
Besides, between-network FC alterations were discovered in SUD as compared to those of BA, including hyperconnectivity between FPN seeds and DMN (the right PCC), and hypoconnectivity between DMN seeds and FPN (the SMA extending to SFG) and between AN seeds and the FPN (the MCC) (Fig. 2 Left, Table 3).
Table 3. Distinct alteration patterns of intrinsic functional connectivity between behavioral addiction and substance use disorder
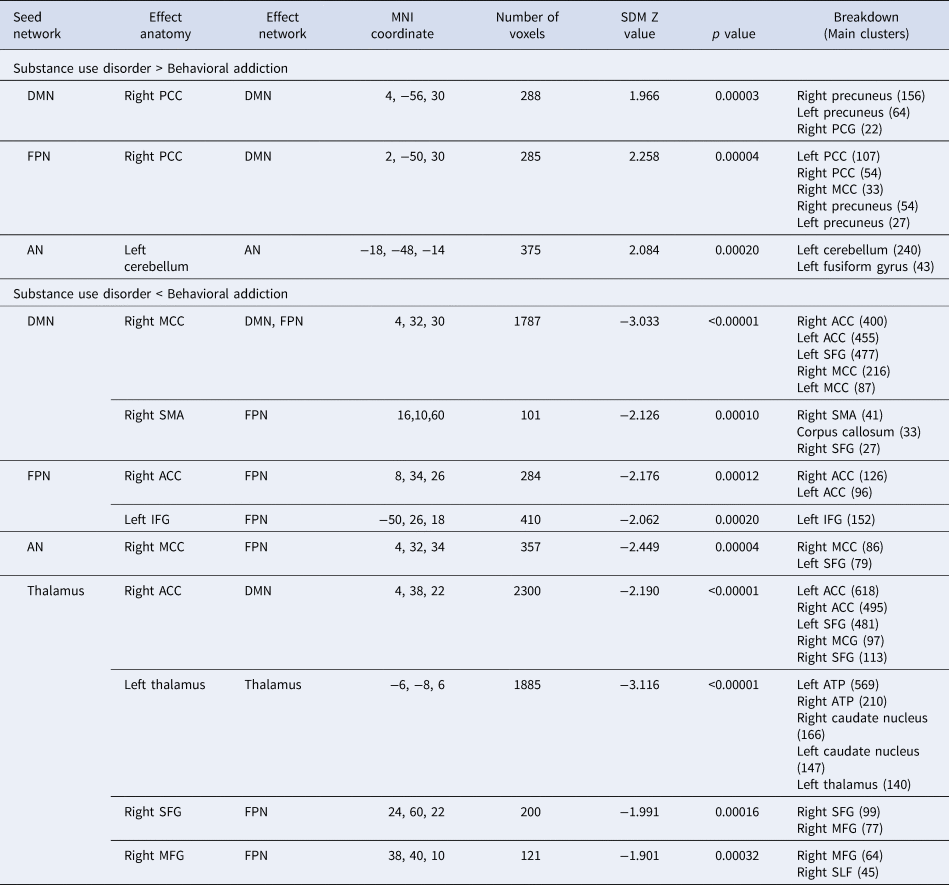
ACC, anterior cingulate cortex; FG, fusiform gyrus; IFG, inferior frontal gyrus; MCC, median cingulate cortex; MFG, middle frontal gyrus; PCC, posterior cingulate cortex; SFG, superior frontal gyrus; AN, affective network; DAN, dorsal attention network; DMN, default mode network; FPN, frontal parietal network; SSN, somatosensory network; SN, salience network.
Consistent findings between group comparison and conjunction analyses
The consistent results between group comparison and conjunction analyses were demonstrated in Fig. 3. Both BA and SUD exhibited hyperconnectivity between DMN and FPN and between SN and SSN, whereas hypoconnectivity between FPN, AN and SN (Fig. 3a). The consistent distinct rs-FC alternations between BA and SUD showed hypoconnectivity in FPN. In particular, FPN exhibited hyperconnectivity with the posterior DMN (pDMN) but hypoconnectivity with the anterior DMN (aDMN).
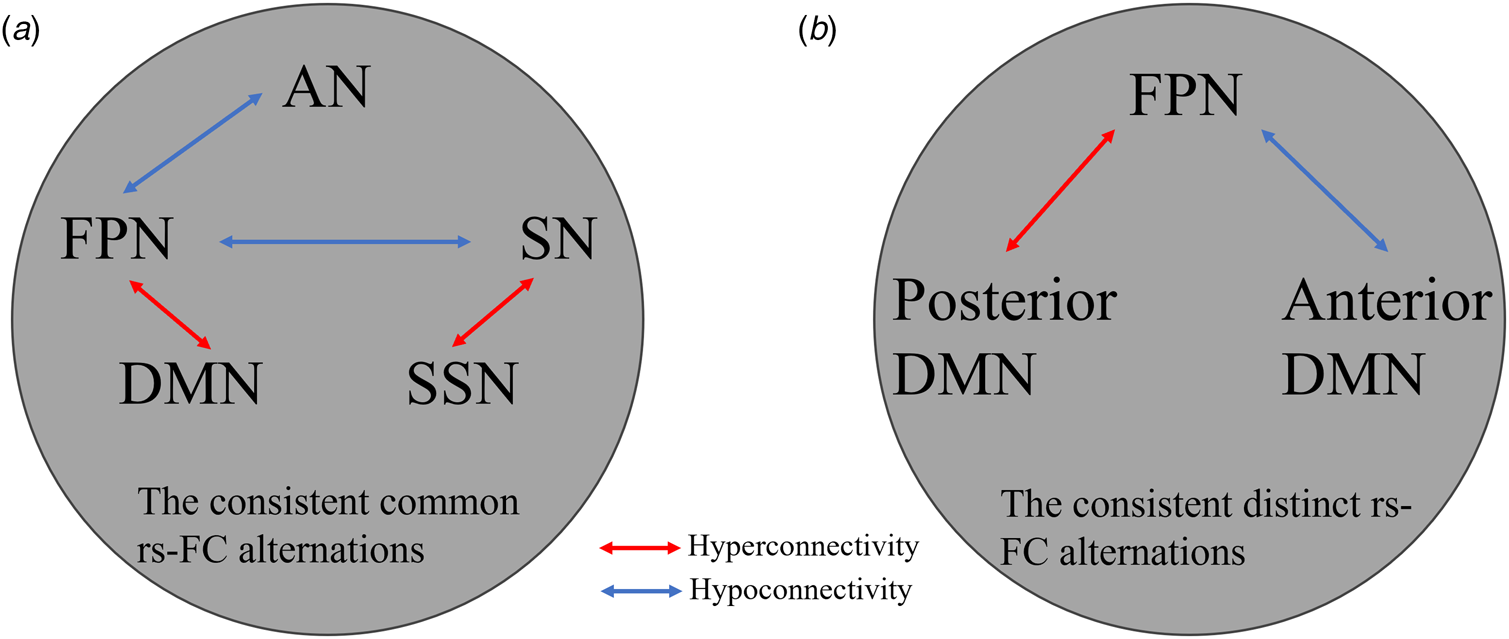
Figure 3. (a) Consistent rs-FC findings through the group comparisons and conjunction analyses in BA and SUD as compared to those of HCs. (b) The consistent rs-FC alterations showed good agreement with those from the contrast analyses between SUD and BA and those form the separate group comparisons in contrast to HCs as well. AN, affective network; DAN, dorsal attention network; DMN, default mode network; FPN, frontal parietal network; SSN, somatosensory network; SN, salience network; BA, behavioral addiction; SUD, substance use disorder.
Control analysis
The subgroup analysis showed distinct patterns of connectivity in between patients using depressant and stimulant. Specifically, patients using depressant showed hyperconnectivity between DMN seeds and the FPN left ACC (left ACC), and between SN seeds and the FPN (ATP). Meanwhile, patients using depressant compared with those using stimulant showed hyperconnectivity within the AN, and hypoconnectivity between the AN seeds and DMN (located in SFG), DMN seeds and FPN (brain region of IFG). These findings highlight differential rs-FC patterns associated with different types of SUD (online Supplementary Fig. S2, Table S1).
The jackknife sensitivity analysis demonstrated that all findings in our study showed high replicability and reliability because all results remained significant in more than 85% of the repeated procedures except those of FC alterations between thalamus seeds, DMN (right MFG) and FPN (right SFG) (for details, see supplementary results and online Supplementary Tables S6–S7).
Regarding the heterogeneity tests, most results showed no or low heterogeneity with I 2 < 10% (I 2 < 10% was regarded as low heterogeneity). The exceptional cases that showed moderate heterogeneity were: (1) rs-FC between FPN seeds and the left ATP in DMN when we compared the addictions with HCs group (I 2 = 20.7), (2) rs-FC between SN seeds and the left SMA in BA as compared to that from HCs (I 2 = 31.1), (3) rs-FC between FPN seeds and the right PCC in BA as compared to that of the HCs (I 2 = 17.5), (4) rs-FC between SN seeds and the left fusiform gyrus in SUD group as compared to that of HCs (I 2 = 21.6), and (5) rs-FC between FPN seeds and the left IFG for the SUD group as compared to that of the HC group (I 2 = 14.6).
In addition, Egger's tests demonstrated that most of the clusters reported in our main results showed no significant publication bias (p > 0.05). The exceptional case involved the clusters of the right IFG (hyperconnectivity with DMN seeds, p = 0.005), the left FAT (hyperconnectivity with DMN seeds, p = 0.001), and the left ATP (hypoconnectivity with DMN seeds, p = 0.023) in comparing the addiction with the HC group. The exceptional case also touched upon the cluster of the right IFG when we compared the BA with HC groups (hyperconnectivity with AN seeds, p = 0.019) (details see the supplementary results and online Supplementary Table S9).
The meta-regression analysis showed that the severity of BA was positively associated with the within-network functional connectivity between SN seeds and the left insula in SN (peak coordinate: −30, −6, 12; SDM-Z = 3.875; p < 0.0001; 263 voxels) for the comparison between BA and HC groups. Interestingly, the duration of substance use showed positive relationship with the between-network hyperconnectivity between AN seeds and the left superior frontal gyrus in FPN (peak coordinate: −2, 32, 42; SDM-Z = 4.555; p < 0.0001; 347 voxels) for the SUD group as compared to those from the HC group Figure 4.
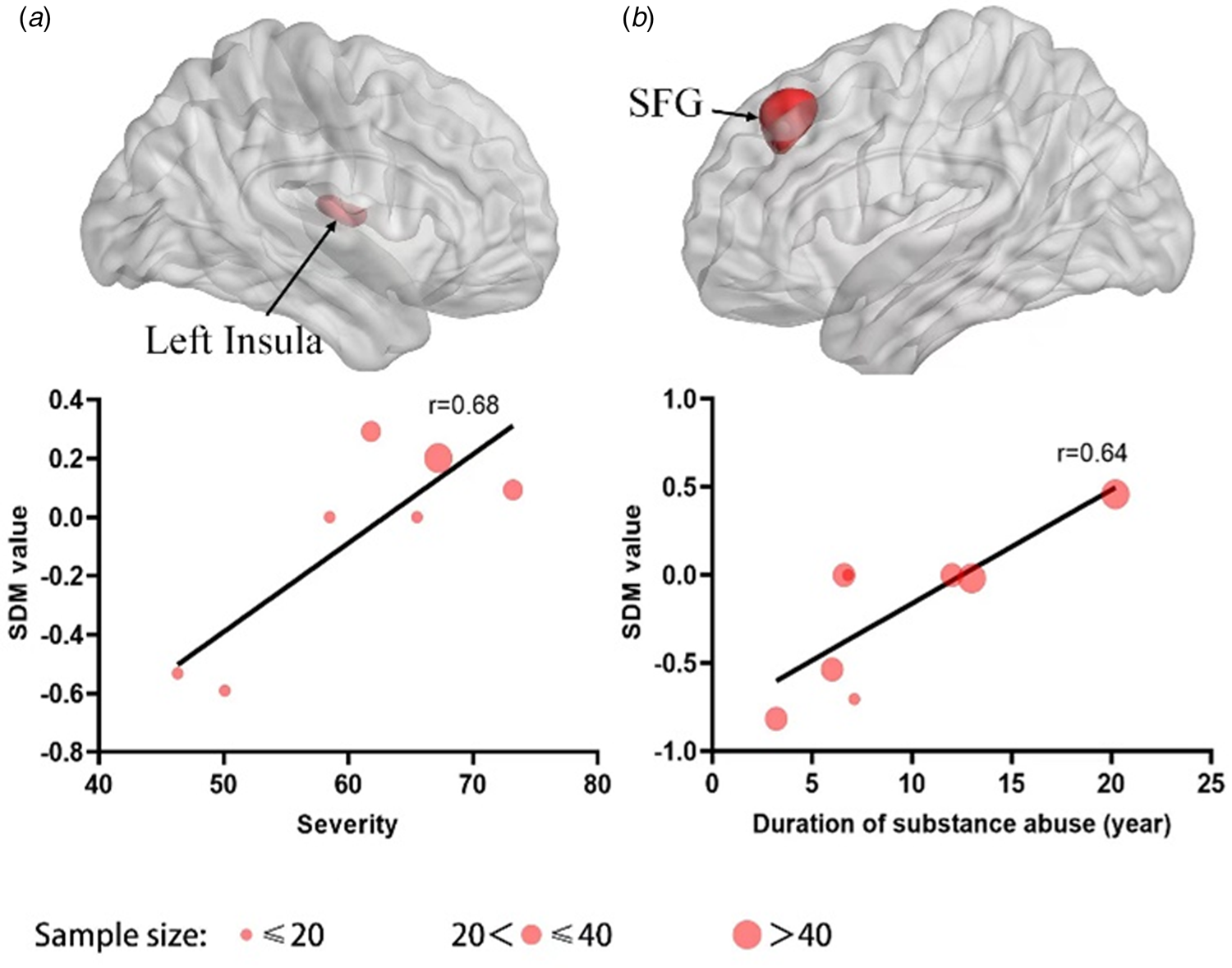
Figure 4. Meta-regression analysis results. (a) Positive correlation was detected in SN between the severity of BA and within-network FC among SN seeds and the insula, and (b) positive correlation was identified between the duration of substance use and between-network FC among SN seeds and FPN in SUD group as compared to that from the HC group. The regression line (meta-regression signed differential mapping slope) was presented as a straight line. The SDM values (effect sizes) were extracted from the peak of maximum slope significance.
Discussion
According to the common and distinct alternations of rs-FC between BA and SUD in contrast to HCs, three main findings were revealed for the present study. First, both BA and SUD exhibited common alterations in rs-FC between FPN and other high-level neurocognitive networks (i.e. DMN, AN and SN), demonstrating the hyperconnectivity between SN seeds and the RO in SSN. In addition, SUD exhibited several distinct within- and between-network rs-FC alterations mainly involved in DMN and FPN as compared to BA, which included: (1) hypoconnectivity within FPN in the left IFG due to a large extent of hypoconnectivity, and (2) hyperconnectivity between FPN and the PCC in DMN due to a large extent of hyperconnectivity, and (3) hypoconnectivity between FPN and the medial prefrontal cortex (PFC) in DMN due to a large extent of hypoconnectivity. Further, it was found that altered within- and between-network FC was significantly associated with the clinical characteristics, i.e., the severity of addiction in BA and the duration of substance usage in SUD. These findings not only provide evidence for better understanding into the shared and specific neural mechanism for BA and SUD, respectively, but also offer potential targets for treating addictive disorders and preventing their recurrence.
The common rs-FC alterations for both BA and SUD
Our findings showed both BA and SUD exhibited hyperconnectivity between FPN and AN, and hypoconnectivity between FPN, SN and DMN. More importantly, these aberrant FCs showed strong correlation with the core features of impaired response inhibition and salience attribution (I-RISA) syndrome in addiction. For example, individuals with addiction are characterized by the behaviors at the core of aberrant attribution of primary salience to drug cue stimuli at the expense of other available rewarding stimuli and persistent decision-making deficits. AN, also known as the limbic network, including the nucleus accumbens, striatum, amygdala, parts of the thalamus, orbitofrontal cortex (OFC), and subgenual/rostral ACC, are implicated in reward processing and computation of subjective value. Likewise, neuroimaging studies also demonstrated that individuals with addiction showed a high level of activation of AN to drug cue-related signals, particularly in the OFC, frontolimbic areas, and ACC. Besides, FPN, also referred to as the central executive network, is anchored in the dorsolateral PFC (dlPFC) and lateral posterior parietal cortex, involving fundamental roles in working memory and decision-making in goal-directed behaviors (Gong et al., Reference Gong, He, Ma, Liu, Huang, Dong and Yao2016; Marek & Dosenbach, Reference Marek and Dosenbach2018). In this study, detected hyperconnectivity between FPN and AN is consistent with previous neuroimaging findings that individuals with addiction showed generally increased engagement of FPN and AN during exposure to drug cues but not nondrug-related tasks (Zilverstand, Huang, Alia-Klein, & Goldstein, Reference Zilverstand, Huang, Alia-Klein and Goldstein2018) while methamphetamine users exhibited strong FC between dlPFC and brain regions in AN during rest (Kohno, Morales, Ghahremani, Hellemann, & London, Reference Kohno, Morales, Ghahremani, Hellemann and London2014).
In addition, the SN, anchored in the anterior insula and dorsal ACC, showed extensive connectivity with subcortical and limbic structures involved in detecting and processing salient events from sensory and limbic inputs and subsequently initiating appropriate control signals to regulate behavior and homeostatic states (Menon, Reference Menon2011; Seeley et al., Reference Seeley, Menon, Schatzberg, Keller, Glover, Kenna and Greicius2007; Sridharan, Levitin, & Menon, Reference Sridharan, Levitin and Menon2008). While both AN and SN attribute salience to stimuli, AN shows a stronger response to the positive effects in the reward domain while SN is implicated in assessments of risk or salience and arousal independent of valence (Bartra, McGuire, & Kable, Reference Bartra, McGuire and Kable2013). Hence, the hypoconnectivity between the SN and the FPN could be a key neural mechanism contributing to their abnormal behaviors. This dysfunction in the SN is associated with altered salience detection, which, in turn, leads to reduced attentional allocation for inhibitory control over risky behaviors processed by the FPN (Menon, Reference Menon2011).
Further, the dysfunctional process of compulsive behaviors despite adverse outcomes in addiction might also involve DMN, which mainly consists of the medial PFC (mPFC), posterior cingulate cortex (PCC), and lateral temporal cortex. DMN usually get activated at rest while deactivated at external attention-oriented tasks, playing an essential role in self-reference and autobiographical information (Menon, Reference Menon2011). Neuroimaging studies also demonstrate that individuals with addiction persist in risk-taking behavior, probably due to impaired awareness into the potential loss and insensitivity to negative affective information reflected by less engagement of DMN (Moeller & Goldstein, Reference Moeller and Goldstein2014; Wesley, Hanlon, & Porrino, Reference Wesley, Hanlon and Porrino2011). Therefore, the measured hypoconnectivity between FPN and DMN in addictions may also contribute to their risky decision-making and other behaviors. Taken together, the observed hyperconnectivity between FPN and AN and hypoconnectivity between FPN, SN and DMN for the addiction groups supports the hypothesis of the I-RISA model that multifaceted impairments in value assessment, salience attribution, redirection attentional resources, and response selection and inhibition are the core mechanisms involved in the maintenance of addiction (Goldstein & Volkow, Reference Goldstein and Volkow2002; Zilverstand et al., Reference Zilverstand, Huang, Alia-Klein and Goldstein2018).
Finally, the hyperconnectivity was also detected between SN and SSN in the cluster with the left RO as the center for both BA and SUD. Interestingly, the cluster of the left RO extending to the STG plays a key role in processing integrated exteroceptive (e.g. visual, sensory) and interoceptive (visceral) signals that are essential for self-awareness and consciousness (Blefari et al., Reference Blefari, Martuzzi, Salomon, Bello-Ruiz, Herbelin, Serino and Blanke2017). It has been documented that cardiovascular control is altered in individuals with addiction, which may also involve addictive behavior maintenance through its influence on visceral reaction to addictive behavior-related cues processed by SSN, thus affecting attention capture processed by SN and thereby serving to perpetuate or exacerbate preoccupation, salience, anticipation, and craving (Eddie, Bates, & Buckman, Reference Eddie, Bates and Buckman2022). Therefore, the detected hyperconnectivity between SSN and SN for individuals with addiction might represent the dysfunctions in the cluster centered in the left RO underlying abnormal sensory information perception and awareness, resulting in interrupting salience detection as the core symptom in addiction.
The distinct rs-FC alternations between BA and SUD
In addition to the common alterations, we also inspected the rs-FC alteration differences between SUD and BA and found: (1) hypoconnectivity within FPN in the left IFG due to a larger extent of hypoconnectivity in SUD as compared to that of BA, (2) hyperconnectivity between FPN and PCC in pDMN due to a larger extent of hyperconnectivity in SUD in contrast to BA, and (3) hypoconnectivity between FPN and mPFC in aDMN due to a larger extent of hypoconnectivity in SUD than that of BA.
Firstly, we found a larger extent of hypoconnectivity within FPN in SUD than that of BA. FPN is involved in response selection and inhibition to maintain goal-directed behavior, whereas the disruption of FPN is linked to the dysregulation of executive function, which is a key component driving compulsive drug seeking (Koob & Volkow, Reference Koob and Volkow2016). In SUD, persisting for months after protracted detoxification, substantial reduction in D2 receptors are detected, which are linked to decreased baseline glucose metabolism in dlPFC, the core hub of FPN (Koob & Volkow, Reference Koob and Volkow2016). However, no significant difference in D2 receptors has been detected in BA as compared to those of HCs (Boileau et al., Reference Boileau, Payer, Chugani, Lobo, Behzadi, Rusjan and Zack2013; Stormezand et al., Reference Stormezand, Doorduin, Chaves, García, Nienhuis, Schoevers and Dierckx2021). Therefore, a larger extent of hypoconnectivity within FPN in SUD as compared to that of BA might be linked to the significant reduction of D2 receptors in SUD.
In addition, different FC alteration directions between FPN, anterior and pDMN (i.e. hyperconnectivity between FPN and pDMN and hypoconnectivity between FPN and aDMN) were detected in SUD as compared to those of BA. In particular, both aDMN (including mPFC, ACC, and etc.) and pDMN (including PCC, precuneus, and etc.) are a subnetwork of DMN, in which the former mainly plays a role in the attribution of personal value and emotional regulation while the latter draws attention to the internal world (Tanabe et al., Reference Tanabe, Nyberg, Martin, Martin, Cordes, Kronberg and Tregellas2011). In this study, our findings demonstrated different change directions in the anterior and pDMN for SUD, i.e., decreased aDMN and increased pDMN activity during the withdrawal period (Zhang & Volkow, Reference Zhang and Volkow2019). Meanwhile, PCC was found anchored in pDMN, showing higher connectivity to FPN involved in cognitive control during attentional demanding tasks (Leech, Braga, & Sharp, Reference Leech, Braga and Sharp2012). Therefore, reduced emotional control (mediated by the aDMN) and increased self-referential processing (mediated by the pDMN) can enhance ruminatory behaviors and negative affect during withdrawal, contributing to the negative reinforcement of drug use. Besides, increased network communications between pDMN and FPN and decreased network interactions between aDMN and FPN for SUD as compared to those of BA might implicate impaired self-awareness, negative emotions, and deeper ruminations states in SUD. According to Wise (Reference Wise2004), substance perturbs the brain reward system much more powerfully and persistently than primary rewards, such as winning in gambling (Wise, Reference Wise2004). Redish (Reference Redish2004) also showed that the dopamine (DA) response to substance delivery persists despite learning by pharmacologically activating DA, which is fully predicted and certain (Redish, Reference Redish2004). However, in BA, the DA release relies on reward delivery, which is influenced by uncertain and never fully predicted reward (e.g. win a game or money). Consequently, the sensitization mechanism of BA and SUD is different because the former arises from unpredictable reinforcement learning while the latter is due to repeated exposure to substances by direct pharmacological stimulation.
In this study, we only used the thalamus as seeds to explore rs-FC alterations in SUD yet not in BA (Denier et al., Reference Denier, Schmidt, Gerber, Vogel, Huber, Lang and Borgwardt2015; Gu et al., Reference Gu, Salmeron, Ross, Geng, Zhan, Stein and Yang2010; Kohno et al., Reference Kohno, Morales, Ghahremani, Hellemann and London2014; Liao et al., Reference Liao, Tang, Liu, Xie, Yang, Johnson and Hao2016; Liu et al., Reference Liu, Cai, Zhao, Cai, Sui, Hou and Yuan2019; Wang et al., Reference Wang, Bai, Wang, von Deneen, Yuan and Cheng2017). Therefore, we detected decreased hypoconnectivity within the thalamus and between the thalamus, DMN, and FPN in SUD. The thalamus is a critical hub of the cortico-striatal-thalamo-cortical reward circuit, playing roles in DA release (Huang et al., Reference Huang, Jiang, Xia, Tang, Zhang, Cui and Wang2018a). Initially, the midbrain dopamine areas, such as the VTA and substantia nigra, receive dopamine inputs from substances, transfer the major dopaminergic projections to the striatum. And then the striatum transmits dopamine to the thalamus, which is partially controlled by the cortex. In particular, the information is eventually sent back to the cortex from the thalamus, and overloaded substances increase DA transmission efficiency in striatum and thalamus (Samaha, Khoo, Ferrario, & Robinson, Reference Samaha, Khoo, Ferrario and Robinson2021). In addition, the thalamus is also involved in the I-RISA model, enhancing thalamic activation towards substance cues during the cue reactivity task and reducing thalamic activation during response inhibition by the Stroop task (Huang, Mohan, De Ridder, Sunaert, & Vanneste, Reference Huang, Mohan, De Ridder, Sunaert and Vanneste2018b; Wang et al., Reference Wang, Bai, Wang, von Deneen, Yuan and Cheng2017). These hypoconnectivities of thalamus might be associated with the abovementioned impairments, abnormal DA release, salience attribution, and response selection and inhibition. Meanwhile, our findings were also consistent with previous findings of structural changes that the thalamus showed a structural degeneration decrease in gray matter volumes and reduced fractional anisotropy in white matter projections from the thalamus to the cortex (Franklin et al., Reference Franklin, Wetherill, Jagannathan, Johnson, Mumma, Hager and Childress2014; Oh et al., Reference Oh, Gosnell, Nguyen, Tran, Kosten and Salas2021).
Comparison with previous meta-analysis on addiction
In comparison to the previous meta-analysis conducted by Tolomeo and Yu (Reference Tolomeo and Yu2022), our study yielded more profound findings. They observed commonly altered functional connectivity in the Putamen for both BA and SUD, which is insufficient to explain the comprehensive behavior alterations observed, such as abnormal salience attribution and reduced inhibition, etc. Our study, on the other hand, established consistency with their findings regarding SUD with striatum alterations. Meanwhile, the alterations of functional connectivity under resting state or working state were correlated with structural alterations (Pang et al., Reference Pang, Aquino, Oldehinkel, Robinson, Fulcher, Breakspear and Fornito2023). Our study aligned with the findings of Qiu and Wang (Reference Qiu and Wang2021), who reported hypoactivation within the FPN and the VAN during inhibition control tasks in individuals with addiction. Furthermore, compared with HCs, individuals with BAs showed brain atrophy in the left ACC, right putamen and right SMA (Qin et al., Reference Qin, Zhang, Chen, Li, Li, Suo and Gong2020), and these regions have also been reported altered functional connectivity in our study. Similarly, in the case of SUD, our study aligns with the findings of Pando-Naude et al. (Reference Pando-Naude, Toxto, Fernandez-Lozano, Parsons, Alcauter and Garza-Villarreal2021), as atrophy of brain regions in insula, anterior cingulate cortex, putamen, and thalamus has been found altered functional connectivity in our analysis. These consistent findings across different modality provide compelling evidence for the involvement of these brain regions in addiction pathology and highlight their significance in addiction-related behaviors.
Relationships between functional connectivity and clinical characteristics
Interestingly, in our meta-regression analysis, the SN alternations in both addiction groups exhibited positive correlation with the clinical characteristics. For example, the severity degrees of BA exhibited positive correlation with SN alternations, whereas the duration of substance use in SUD also showed positive correlation with the alternation between SN seeds and FPN. In addition, deeper degrees (BA) or longer indulges (SUD) are more likely to be automatically oriented to addiction-related salient stimuli (e.g. drugs and internet games), although they do not voluntarily intend to do that (Nestor, McCabe, Jones, Clancy, & Garavan, Reference Nestor, McCabe, Jones, Clancy and Garavan2011; Qiu & Wang, Reference Qiu and Wang2021). Further, the hypoconnectivity between SN and FPN was linked to weak salience detection and risk decision making (Menon, Reference Menon2011). Likewise, long indulgence in substance showed positive correlation with hypoconnectivity between FPN and SN, indicating that the longer indulged SUD is more likely to make risky decisions and harder to inhibit. In support of our findings, consistent evidence from previous studies in rest and task performance confirms that prolonged addictive behavior results in continuous brain alterations in SN (Hendrick, Luo, Zhang, & Li, Reference Hendrick, Luo, Zhang and Li2012; Nestor et al., Reference Nestor, McCabe, Jones, Clancy and Garavan2011). The results of the meta-regression provide evidence for SN alterations in the neural mechanisms underlying addiction progression and suggest that SN may serve as a potential biomarker for assessing treatment response or predicting treatment outcome. However, we did not find significant correlations between rs-FC and symptoms of depression, anxiety, and impulsivity. This may due to the conservative p value threshold criteria we used in this study and limited number of researches recruited.
Limitations
There are several limitations warranting discussion for the present study. First, only seed-based rs-FC studies were adopted in this meta-analysis, while studies using other analytic methods were not included, such as independent component analysis (ICA). Future work can use different analysis methods to conduct conjunction analysis, which can test the reliability of these methods. Secondly, SUD is rather complicated, containing different types of substances with different neurotoxic effects on the brain system. In addition, multiple substance intake and comorbidity are rather common in individuals with addiction, which makes it hard to eliminate the confounding effects of these factors on brain functional networks. Importantly, different demographic and clinical conditions of the participants might bring potential bias for this meta-analysis. For instance, different developmental timepoints have different neurobiological implications in the context of addiction. Future studies based on individuals are needed to explicitly explore the effects of these factors on rs-FC. Further, inconsistent methodologies, particularly the preprocessing methods, exist in the field of rs-FC fMRI, which might affect the analysis results of this study. Standard processing pipelines are urgently needed in this field, while meta-analyses for methodology comparisons are also needed to solve this problem. Lastly, while our meta-analysis focused on rs-FC, it is worth noting that brain function in patients with addiction could also be explored by cognitive tasks. Future task-based meta-analyses could provide valuable insights into how the brain function of addiction is altered during specific tasks and how these alterations relate to the characteristics of addiction.
Conclusion
By exploring the common and distinct patterns of BA-related and SUD-related rs-FC changes, this study detected substantial common dysconnectivity between BA and SUD, which is also consistent with the impaired triple-network dysfunction model. Meanwhile, compared with BA, SUD-related large-scale functional network alterations might be related to damage from physiological consequences. These findings may offer evidence for further effective treatment strategies targeting the brain regions with common rs-FC changes or conducting precise intervention based on distinct mechanisms of the addictions via neuromodulation technique, neurofeedback training, longitudinal treatment evaluation.
Supplementary material
The supplementary material for this article can be found at https://doi.org/10.1017/S0033291723003434.
Acknowledgements
We thank Dr Fei Gao (Fudan University) for revising the manuscript and response to reviewers.
Authors' contribution
X.Z designed the study; X.Z and X.H searched and screened the literature; X.Z, and X.H collected the required data for meta-analysis; X.Z conducted meta-analysis; X.Z write the manuscript; D.Z, P.J and Z.Y revised the manuscript.
Funding statement
This work was supported by Sichuan Natural Science Foundation (2022NSFSC1454), the University of Macau (Nos. MYRG 2020-00067-FHS, MYRG2019-00082-FHS, and MYRG2018-00081-FHS), the Macao Science and Technology Development Fund (No. FDCT 0020/2019/AMJ and FDCT 0011/2018/A1), Guangdong Natural Science Foundation (EF017/FHS-YZ/2021/GDSTCRSKTO), Shenzhen Basic Research Project (No. JCYJ20180305125425815), and Guangdong-Hong Kong-Macao Greater Bay Area Center for Brain Science and Brain-Inspired Intelligence Fund (No. 2019011).
Competing interests
The authors report no conflicts of interest. The authors are responsible for the content and writing of the paper.