Introduction
Attention-deficit/hyperactivity disorder (ADHD) is a childhood onset neurodevelopmental condition, characterised by clinical symptoms of impulsivity, hyperactivity and inattention as well as emotional dysregulation (Kieling et al., Reference Kieling, Kieling, Rohde, Frick, Moffitt, Nigg and Castellanos2010) that often persists into adulthood (Faraone, Reference Faraone2007) with far-reaching negative consequences on quality of life (Barkley & Fischer, Reference Barkley and Fischer2010). In addition to the clinical symptoms and deficits in core cognitive functions (McLean et al., Reference McLean, Dowson, Toone, Young, Bazanis, Robbins and Sahakian2004), emerging research now indicates anatomical and neurophysiological abnormalities associated with this disorder. Reductions in grey matter volume and cortical thickness (Carmona et al., Reference Carmona, Vilarroya, Bielsa, Tremols, Soliva, Rovira and Bulbena2005; Shaw et al., Reference Shaw, Lerch, Greenstein, Sharp, Clasen, Evans and Rapoport2006, Reference Shaw, Eckstrand, Sharp, Blumenthal, Lerch, Greenstein and Rapoport2007), disruptions to white matter tracts (Valera, Faraone, Murray, & Seidman, Reference Valera, Faraone, Murray and Seidman2007) and aberrant brain functional network organisation (Konrad & Eickhoff, Reference Konrad and Eickhoff2010) have all been suggested to form the neuropathophysiology of ADHD. Collectively, this evidence indicates wide-spread dysfunctions in core neuronal circuits, highlighting the biological underpinnings of ADHD symptomatology.
In this regard, a recent review on the genetic basis of ADHD indicates the mean heritability across 37 twin studies as 74% (Faraone & Larsson, Reference Faraone and Larsson2019). Furthermore, in a population-based family sample, greater genetic relatedness was linked to greater familial aggregation of ADHD with a heritability estimate of around 80% that was heightened with the persistence of ADHD into adulthood (Chen et al., Reference Chen, Brikell, Lichtenstein, Serlachius, Kuja-Halkola, Sandin and Larsson2017). However, despite this reported heritability (Franke et al., Reference Franke, Faraone, Asherson, Buitelaar, Bau and Ramos-Quiroga2012; Mick & Faraone, Reference Mick and Faraone2008; Thapar, O'Donovan, & Owen, Reference Thapar, O'Donovan and Owen2005), genetic studies for the identification of ADHD susceptibility genes have so far produced disparate results (Hinney et al., Reference Hinney, Scherag, Jarick, Albayrak, Putter, Pechlivanis and Psychiatric2011). This might possibly be due to the heterogeneity of the disorder, intrinsic limitations of the commonly employed nosographic systems, experimental focus that is largely confined to young children, and limited reports on endophenotype-based research, which requires further investigation.
Defined as covert, quantitative traits, endophenotypes are assumed to be highly heritable and closely related to the expression of genes linked to a disorder (Cannon & Keller, Reference Cannon and Keller2006). Furthermore, the number of genes associated with such basic neurocognitive components are expected to be fewer as compared with those linked to the overt clinical phenotype, which enhances the statistical power in studies aimed at discovering susceptibility genes (Gottesman & Gould, Reference Gottesman and Gould2003). Thus, similar to the strategies employed in assessing ‘at-risk’ individuals in other mental health disorders such as schizophrenia (Whitfield-Gabrieli et al., Reference Whitfield-Gabrieli, Thermenos, Milanovic, Tsuang, Faraone, McCarley and Seidman2009), further research into familial endophenotypes that incorporate parents, full siblings or children who share 50% of their genetic makeup with the patients, may provide a more fruitful route to identifying genes that will ultimately improve translational research and drug discovery (Castellanos & Tannock, Reference Castellanos and Tannock2002). This better understanding of the neuropathophysiology of ADHD should in turn improve success rates in the search for novel pharmaceutical agents (Doyle et al., Reference Doyle, Willcutt, Seidman, Biederman, Chouinard, Silva and Faraone2005).
To this end, emerging familial investigations have recently illustrated shared abnormalities in sustained attention performance (Gau & Huang, Reference Gau and Huang2014) and brain morphology (Pironti et al., Reference Pironti, Lai, Muller, Dodds, Suckling, Bullmore and Sahakian2014) between ADHD patients and their first-degree relatives that may potentially make up vital endophenotypes of ADHD. In line with these findings, another latent neurocognitive trait may be rooted in the intrinsic brain connectivity dynamics as measured via resting-state functional magnetic resonance imaging (rs-fMRI). Termed functional connectivity (Friston, Reference Friston1994), investigations into the temporal correlations of spontaneous blood-oxygen-level-dependent (BOLD) signal fluctuations from distinct brain regions (Biswal, Yetkin, Haughton, & Hyde, Reference Biswal, Yetkin, Haughton and Hyde1995) have been largely popularised for their utility in identifying modifications to the intrinsic brain functional network organisation across mental health disorders (Fox & Greicius, Reference Fox and Greicius2010). Specifically, one particular large-scale system, namely the default mode network (DMN), has been recognised as a new locus of dysfunction in ADHD, with studies reporting on its altered levels of integrity and connectivity during wakeful states of rest (Castellanos, Kelly, & Milham, Reference Castellanos, Kelly and Milham2009; Konrad & Eickhoff, Reference Konrad and Eickhoff2010; Posner, Park, & Wang, Reference Posner, Park and Wang2014).
Comprising the anterior/posterior midline as well as the posterior parietal cortices, the DMN has been associated with internal, self-referential thoughts during unconstrained resting-state conditions (Andrews-Hanna, Smallwood, & Spreng, Reference Andrews-Hanna, Smallwood and Spreng2014) with further implications in executive functions commonly impaired in ADHD such as sustained attention, working memory and cognitive flexibility (Bozhilova, Michelini, Kuntsi, & Asherson, Reference Bozhilova, Michelini, Kuntsi and Asherson2018; McLean et al., Reference McLean, Dowson, Toone, Young, Bazanis, Robbins and Sahakian2004). Across both adults (Castellanos et al., Reference Castellanos, Margulies, Kelly, Uddin, Ghaffari, Kirsch and Milham2008) and adolescents (Sun et al., Reference Sun, Cao, Long, Sui, Cao, Zhu and Wang2012) with ADHD, previous research has shown significant reductions in anti-correlations (or negative connectivity) of a core DMN hub, namely the posterior cingulate cortex and precuneus (PCC/PCUN) (Fransson & Marrelec, Reference Fransson and Marrelec2008), and regions commonly implicated in cognitive control. In combination with these reports, the significant heritability estimate of DMN in the general population (Glahn et al., Reference Glahn, Winkler, Kochunov, Almasy, Duggirala, Carless and Blangero2010) suggests the potentially utility of this large-scale network as putative brain-based endophenotype under neurodevelopmental conditions (Korgaonkar, Ram, Williams, Gatt, & Grieve, Reference Korgaonkar, Ram, Williams, Gatt and Grieve2014). Nevertheless, endophenotype-based research studies into the neuropathophysiology of this network across ADHD probands and their first-degree relatives in comparison with typically developing controls, as well as direct investigations into its cognitive and clinical correlates, have not been previously conducted.
To this end, here we investigated alterations in brain functional network connectivity within the DMN during rs-fMRI and tested the suitability of this measure as a potential neurocognitive endophenotype in a cohort of 20 adult ADHD probands, their 20 unaffected first-degree relatives and 20 typically developing control participants (n = 60). Given prior research, we hypothesised that alterations in the connectivity of DMN to the frontal brain regions would be shared between adults with ADHD and their first-degree relatives, which would significantly correlate with deficits in sustained attention performance and severity of ADHD symptoms. Finally, based on recent reports that highlight ADHD symptomatology as dimensional traits across a continuum of normal to abnormal functioning (Chabernaud et al., Reference Chabernaud, Mennes, Kelly, Nooner, Di Martino, Castellanos and Milham2012; Elton, Alcauter, & Gao, Reference Elton, Alcauter and Gao2014), in a separate dataset from the Human Connectome Project (HCP) (n = 100) we aimed to assess the generalisability of our findings by examining the potential utility of this neurocognitive trait in explaining dimensional variation in ADHD symptomatology in a cohort of unrelated healthy young adults.
Methods
Participants and clinical assessment
Ethical approval for this experiment was obtained from the Cambridgeshire 3 Research Ethics Committee (REC: 09/H0306/38). All individuals gave informed consent prior to their participation. ADHD probands and their first-degree relatives were recruited from the Adult ADHD Research Clinic, Addenbrooke's Hospital, Department of Psychiatry, University of Cambridge, whereas typically developing controls were recruited from the local community.
For the patient cohort, the diagnosis of ADHD (DSM-IV Text Revision) was based on a full clinical interview with the patient and an informant who had known the patient since childhood (e.g. parents). The clinical assessment included Barkley Adult ADHD Rating Scale Version IV (BAARS-IV) for childhood and adult symptoms, provided by both the patient and the informant (Barkley, Reference Barkley2011). In addition, the Mini International Neuropsychiatric Interview (MINI) (Sheehan et al., Reference Sheehan, Lecrubier, Sheehan, Amorim, Janavs, Weiller and Dunbar1998) was administered to screen for DSM-IV Axis I disorders, and the National Adult Reading Test (NART) (Bright, Jaldow, & Kopelman, Reference Bright, Jaldow and Kopelman2002) was used to estimate full scale IQ. Eligible ADHD patients were then asked to contact a first-degree relative (e.g. parents, full siblings). Both the first-degree relatives of the ADHD probands and the typically developing control participants were screened for undiagnosed adult ADHD or a comorbid disorder using the same clinical assessments, except for only the self-report BAARS-IV (Barkley & Murphy, Reference Barkley and Murphy2006) for adult symptoms was administered in these groups, without the informant.
The exclusion criteria were: NART full scale IQ score equal to or less than 90, current or past history of developmental, neurological or psychiatric disorders based on a formal DSM-IV Text Revisions diagnosis and contraindication to an MRI scan. The final group of ADHD participants recruited for this study was 20 (mean age = 32.20 years, s.d. = 10.31, 3/17 female to male ratio), comprising 16 patients with combined type and four with inattentive type; 16 of which were medicated with methylphenidate, while four were not receiving any medication for their ADHD diagnosis. In addition to the patient group, a total of 20 age and gender-matched first-degree relatives (mean age = 38.85 years, s.d. = 15.31, 10/10 female to male ratio) of the ADHD probands and 20 typically developing control participants (mean age = 32.5 years, s.d. = 5.8, 7/13 female to male ratio) were recruited for this study. Neither the first-degree relatives, nor the typically developing control participants showed ADHD symptoms meeting the DSM-IV Text Revision diagnostic threshold for ADHD, or a diagnosis of any other comorbid disorder. With the aim of minimising the potential impact of psychotropic medication on cognitive performance and brain dynamics, ADHD participants were asked to withhold taking their medication 24 h before (Gualtieri et al., Reference Gualtieri, Wargin, Kanoy, Patrick, Shen, Youngblood and Breese1982; Turner, Blackwell, Dowson, McLean, & Sahakian, Reference Turner, Blackwell, Dowson, McLean and Sahakian2005) and to refrain from consuming alcohol or caffeine-containing drinks on the day of the testing. The same request was made for the first-degree relatives and control participants.
In order to ensure matching of demographic information between groups, age, gender and NART full scale IQ scores were entered into multivariate and univariate analyses of variance (ANOVA) and post-hoc t tests (online Supplementary Table S1) using the SPSS Statistics software package (Version 23.0) (https://www.ibm.com/products/spss-statistics). The self-report BAARS-IV current total symptom scores as well as the inattentive and hyper-impulsive sub-scales were also assessed for group differences with the same statistical procedures, correcting for age, gender and NART full scale IQ scores (online Supplementary Table S2).
Cognitive assessment
All participants completed the Rapid Visual Information Processing (RVP) test of sustained attention from the Cambridge Neuropsychological Test Automated Battery (CANTAB) (Robbins, James, Owen, & Sahakian, Reference Robbins, James, Owen and Sahakian1994; Sahakian & Owen, Reference Sahakian and Owen1992). In this task, participants were asked to pay attention to a computer screen in which pseudo-random digits [2–9] were presented one at a time, at a frequency of 100 per min, next to a list of three-digit sequences. Participants had to press a button any time they detected one of the three-digit target sequences. Requiring both sustained attention and working memory, performance in this task has been previously shown to be lower in ADHD patients and their siblings as compared to typically developing controls (Gau & Huang, Reference Gau and Huang2014). Univariate analysis of covariance (ANCOVA) was used to assess group differences in performance, with group membership (i.e. ADHD probands, their first-degree relatives, healthy controls) entered as fixed factor and total hits in the RVP task as the dependent variable. Age, gender and NART full scale IQ scores were included as covariates and post-hoc t tests were corrected for multiple comparisons using the Bonferroni method.
MRI data acquisition and preprocessing
Participants were scanned using a Siemens MAGNETOM Tim Trio 3T scanner (32-channel head coil) at the Wolfson Brain Imaging Centre, Cambridge. The brain imaging session started with a high resolution T1-weighted, magnetisation-prepared rapid gradient-echo (MPRAGE) structural scan (TR = 2300 ms, TE = 2.98 ms, slice thickness = 1.00 mm). The echo planar imaging sequence parameters for the 8.73-min eyes-closed resting-state functional data acquisition were as follows: 32 slices in each volume, 3.0 mm slice thickness, 3.0 × 3.0 × 3.0 voxel size, TR = 2000 ms, TE = 30 ms, flip angle = 78 degrees, 262 volumes. The MRI data preprocessing pipeline included slice-time and motion (six degrees of freedom) corrections, co-registration to the high resolution T1-weighted structural image, normalisation to the Montreal Neurological Institute (MNI) space through the unified segmentation–normalisation algorithm (Ashburner & Friston, Reference Ashburner and Friston2005) and smoothing with an 8 mm FWHM Gaussian kernel using the SPM software package (Version 12.0) (http://www.fil.ion.ucl.ac.uk/spm/), based on the MATLAB platform (Version 15a) (http://www.mathworks.co.uk/products/matlab/).
Functional connectivity analysis
Seed-based functional connectivity analyses were shown to be influenced by the definition of seed regions of interest (ROI) (Smith et al., Reference Smith, Miller, Salimi-Khorshidi, Webster, Beckmann, Nichols and Woolrich2011), which can be partially alleviated by the use of large-scale meta-analyses. For this reason, we employed an objective approach to define our seed ROI using Neurosynth (http://neurosynth.org/) (Yarkoni, Poldrack, Nichols, Van Essen, & Wager, Reference Yarkoni, Poldrack, Nichols, Van Essen and Wager2011). Following the meta-analysis performed on fMRI brain activity data for three search terms stored in the Neurosynth database, namely ‘default (n = 519), default mode (n = 419), default network (n = 80)’, three false-positive discovery rate corrected, statistical co-activation maps (P term|activation) were created. The three maps corresponding to the three search terms were binarised, overlapped and masked with an anatomical automated labelling atlas-based PCC/PCUN template obtained from the Wake Forest University PickAtlas toolbox (http://fmri.wfubmc.edu/software/pickatlas) (Maldjian, Laurienti, Kraft, & Burdette, Reference Maldjian, Laurienti, Kraft and Burdette2003). This procedure provided us with a PCC/PCUN seed that was previously utilised in a resting-state study (Vatansever, Manktelow, Sahakian, Menon, & Stamatakis, Reference Vatansever, Manktelow, Sahakian, Menon and Stamatakis2016). PCC, together with PCUN (Fransson & Marrelec, Reference Fransson and Marrelec2008) is considered to be one of the most robust DMN hubs (Leech & Sharp, Reference Leech and Sharp2014), hence providing a reliable seed region to assess DMN connectivity.
The functional connectivity analysis was carried out using the Conn functional connectivity toolbox (Version 17.f) (https://www.nitrc.org/projects/conn) (Whitfield-Gabrieli & Nieto-Castanon, Reference Whitfield-Gabrieli and Nieto-Castanon2012). At first, a strict pipeline of denoising procedure was employed that included CompCor components attributable to the signal from white matter and cerebrospinal fluid (Behzadi, Restom, Liau, & Liu, Reference Behzadi, Restom, Liau and Liu2007) as well as a linear detrending term, eliminating the need for global signal normalisation that reportedly enhances spurious anti-correlations (Chai, Castanon, Ongur, & Whitfield-Gabrieli, Reference Chai, Castanon, Ongur and Whitfield-Gabrieli2012; Murphy, Birn, Handwerker, Jones, & Bandettini, Reference Murphy, Birn, Handwerker, Jones and Bandettini2009). The subject-specific six realignment parameters, the main effect of rest-condition and their second-order derivatives were also included in the analysis as potential confounds (Fair et al., Reference Fair, Schlaggar, Cohen, Miezin, Dosenbach, Wenger and Petersen2007). In an additional step to remove potential motion artefacts, the data were motion scrubbed using the composite motion score. The settings of a global signal greater than z = 9 and head motion greater than 2 mm were used to identify volumes with high composite motion score which were then added as potential confounds to the linear regression. Furthermore, mean framewise displacement, denoting scan-to-scan motion, was also calculated to be used as a covariate in our subsequent correlation analyses. Moreover, a temporal filter of 0.009 and 0.08 Hz was applied to focus on low frequency fluctuations (Fox et al., Reference Fox, Snyder, Vincent, Corbetta, Van Essen and Raichle2005).
Following this procedure, seed-based functional connectivity analyses were performed for each subject using the average BOLD signal from the binarised ROI map described above. One-sample t-statistics were used to obtain PCC/PCUN connectivity maps representing the DMN and its anti-correlations for each group. All reported clusters were multiple-comparison corrected using the family-wise error detection technique at the 0.05 level of significance (voxel-level 0.001 uncorrected). Moreover, group-level ANOVA was carried out using F-statistics to examine changes in DMN connectivity (PCC/PCUN seed) across ADHD probands, their first-degree relatives and the typically developing control group. The individual connectivity values (z-scores) extracted from the cluster that showed significant alterations in connectivity to the chosen seed were then entered into a univariate ANCOVA (correcting for age, gender and NART full scale IQ score) to further examine the fixed-effects of group membership on DMN connectivity. Post-hoc t tests were carried out to assess pair-wise group differences that were corrected using the Bonferroni method.
Brain, cognition and symptom correlation analysis
Across the whole study cohort, the association between PCC/PCUN connectivity to the brain regions which showed a significant group interaction (z-scores), BAARS-IV current total symptom scores and the number of total hits from the RVP sustained attention task was examined using bivariate non-parametric partial Spearman's correlations (correcting for age, gender, NART full scale IQ score and mean framewise displacement). All measures were assessed for normality using the Shapiro–Wilk test and Q–Q plots, and the correlations were Bonferroni corrected for multiple-comparisons. The results were visualised using the Seaborn package (Waskom et al., Reference Waskom, Botvinnik, O'Kane, Hobson, Ostblom, Lukauskas and Qalieh2018) from Python library. In addition, a mediation analysis was performed with the RVP task (total hits) sustained attention scores as the mediator using PROCESS macro Version 3 (Hayes, Reference Hayes2018) with a percentile bootstrap estimation approach (10 000 samples) (Shrout & Bolger, Reference Shrout and Bolger2002).
External validation dataset and analysis
An independent dataset from the HCP was used to provide external validation to our results from the main experiment, to assess generalisability of our findings and to test the potential utility of the identified neurocognitive traits in explaining dimensional variation in ADHD symptomatology across a group of unrelated healthy young adult participants. A total of 100 participants' high-quality, openly available data were downloaded from the HCP Q5 Release (mean age = 29.11 years, s.d. = 3.676, 54/46 female to male ratio). Full details on the HCP dataset, including the acquisition parameters are provided in the literature (Van Essen et al., Reference Van Essen, Smith, Barch, Behrens, Yacoub, Ugurbil and Consortium2013). The output from the HCP minimal preprocessing pipeline was used for the analyses. Specifically, T1-weighted images from the output of the HCP PreFreeSurfer pipeline that was biased corrected and normalised to the MNI space were utilised as high-resolution structural images. Similarly, the functional data output of the fMRIVolume pipeline from two-runs of 15-min rs-fMRI sessions that was artefact/motion corrected and normalised to the MNI space was used for the seed-based functional connectivity analyses. This preprocessing pipeline largely matched the one used for the main experimental data besides few exceptions mentioned below.
The high-quality HCP data were acquired with a very short, 0.72 s TR meaning each slice in a given volume was obtained highly close together, thus eliminating the need for slice-time correction (Glasser et al., Reference Glasser, Sotiropoulos, Wilson, Coalson, Fischl, Andersson and Consortium2013). In addition, previous connectivity studies using HCP data have shown that spatial smoothing did not alter the results and thus no smoothing was used (Finn et al., Reference Finn, Shen, Scheinost, Rosenberg, Huang, Chun and Constable2015). Finally, instead of the typical anterior–posterior or posterior–anterior phase encoding directions, the HCP rs-fMRI images were acquired with left–right (LR) and right–left (RL) phase encoding directions using an asymmetric acquisition matrix with the purpose of reducing signal loss and distortions that result from high-resolution data acquisition. Hence, functional data from both the LR and RL phase-encoding runs were used to calculate average functional connectivity maps (Glasser et al., Reference Glasser, Sotiropoulos, Wilson, Coalson, Fischl, Andersson and Consortium2013; Van Essen et al., Reference Van Essen, Smith, Barch, Behrens, Yacoub, Ugurbil and Consortium2013).
Subsequently the same data denoising procedures (except for scrubbing) and functional connectivity analyses from the main experimental study was employed for the HCP functional data. A one-sample t test was performed in order to assess DMN connectivity, and a binarised mask for the region that showed significant alterations in connectivity between groups from the main experiment was used to extract connectivity values (z-scores). We then employed bivariate non-parametric partial Spearman's correlation to assess the link between DMN connectivity and Achenbach Adult Self-Report (ASR) DSM-oriented Attention Deficit/Hyperactivity (AD/H) scale (Achenbach & Rescorla, Reference Achenbach and Rescorla2003) within the upper 50th percentile of the full cohort, who scored relatively high on ADHD symptoms (corrected for age, gender and mean framewise displacement). This cut-off point was chosen to avoid floor effects in this neurotypical cohort (mean age = 28.98 years, s.d. = 3.583, female to male ratio = 22/28).
Finally, the cluster of brain regions showing significant alteration in its connectivity to the chosen seed from the main experiment was decoded for cognitive terms using Neurosynth meta-analysis toolbox (Rubin et al., Reference Rubin, Koyejo, Gorgolewski, Jones, Poldrack and Yarkoni2017). The top 100 terms were chosen, from which we removed anatomical terms such as ‘prefrontal cortex’, leaving behind only the cognitive terminology. These terms were then entered into a word cloud with the associated weights. A total of 23 cognitive terms (after removal of anatomical terms) most associated with activity in this cluster of brain regions was used to create a word cloud to aid in the cognitive interpretation of our results.
Results
Default mode connectivity alterations
In line with previous studies, our PCC/PCUN seed-based functional connectivity analysis (Fig. 1a) revealed an intact DMN across all three groups, covering regions of the posterior cingulate, medial prefrontal cortices, bilateral angular gyri, anterior/medial temporal lobes and superior frontal gyri (Fig. 1b). However, an ANCOVA across the three groups with DMN connectivity as the dependent variable highlighted a cluster of brain regions centred on the right middle frontal gyrus (MFG) [extending into the inferior frontal gyrus (IFG)] [MNI peak: +48 + 48 + 16, cluster size = 414 voxels] as showing significant alterations in its connectivity to the chosen seed ROI (F (2,54) = 14.402, p < 0.001, partial η 2 = 0.348) (Fig. 1b). Post-hoc t tests on the connectivity values extracted from this cluster showed a significant difference (i.e. reduced anti-correlation) between ADHD probands and controls [t (54) = 5.288, 95% confidence interval (CI) (0.163–0.363), p < 0.001, partial η 2 = 0.341] as well as between first-degree relatives and controls [t (54) = 3.440, 95% CI (0.071–0.271), p = 0.001, partial η 2 = 0.180]; but there were no significant differences between the ADHD probands and their first-degree relatives [t (54) = 1.844, 95% CI (−0.008 to 0.192), p = 0.071, partial η 2 = 0.059] (Table 1, Fig. 2a).

Fig. 1. Group differences in resting-state default mode connectivity in ADHD probands, their unaffected first-degree relatives and typically developing control participants. (a) An overlap of the meta-analytic activation maps related to the terms ‘default, default mode and default network’ from the Neurosynth database was used to create an objective seed of the PCC/PCUN for seed-based functional connectivity analysis of the DMN. (b) Group-level one sample t tests and an F-contrast revealed consistent yet altered DMN connectivity across the three groups (displayed on an inflated brain surface from the Montreal Neurological Institute – MNI). Specifically, the group difference was centred on the right middle frontal gyrus (MFG) [MNI peak: +48 + 48 + 16, cluster size = 414 voxels], extending into the inferior frontal gyrus (IFG).

Fig. 2. Shared neurocognitive impairments in adults with ADHD and their unaffected first-degree relatives in relation to cognitive performance and ADHD symptomatology. (a) Default mode functional connectivity as characterised by PCC/PCUN to right MFG interactions and (b) cognitive assessment on the RVP task of sustained attention revealed neurocognitive endophenotypes i.e. traits that are potentially closer to the genetic expression as opposed to the clinical outcome. (c) All groups were also assessed for ADHD symptoms using Barkley Adult ADHD Rating Scale Version IV (BAARS-IV) self-reports. In line with the clinical diagnosis, the ADHD probands scored higher than both their first-degree relatives and typically developing control participants, whereas no significant difference was observed between the latter two groups. (d–f) Non-parametric bivariate partial Spearman's correlations (r s) across the three experimental groups illustrated significant links between brain connectivity, cognitive performance and ADHD symptom scores (corrected for age, gender, NART full scale IQ scores and mean framewise displacement). In other words, participants with higher BAARS-IV current total symptom scores showed reduced DMN anti-correlation and a smaller number of total hits on the RVP sustained attention task, which in turn was linked to reduced DMN anti-correlation. The violin plots illustrate the kernel density estimation of the underlying distribution, whereas the boxplots show the interquartile range. *** denotes p < 0.001 and ** denotes p < 0.01. The shaded areas represent 95% CIs.
Table 1. Statistical comparison of resting-state brain connectivity, cognitive performance and symptom scores across ADHD probands, their unaffected first-degree relatives and typically developing control participants, corrected for age, gender and NART full scale IQ score.

PCC/PCUN, posterior cingulate cortex/precuneus; rMFG, right middle frontal gyrus; RVP, rapid visual information processing; BAARS-IV, Barkley Adult ADHD Rating Scale Version IV.
Cognitive and clinical assessments
In parallel to the DMN connectivity results, ANCOVA for the sustained attention scores showed a significant difference in the RVP total hits across the three experimental groups (F (2,54) = 6.300, p = 0.003, partial η 2 = 0.189), in which both the ADHD probands [t (54) = −3.037, 95% CI (−7.477 to −1.531), p = 0.004, partial η 2 = 0.146] and their unaffected first-degree relatives [t (54) = −3.110, 95% CI (−7.595 to −1.641), p = 0.003, partial η 2 = 0.152] performed significantly worse than the typically developing control participants, whereas the former two groups showed no significant difference to each other [t (54) = −0.077, 95% CI (−3.088 to 2.861), p = 0.939, partial η 2 < 0.001] (Table 1, Fig. 2b).
In contrast, an ANCOVA between groups on the BAARS-IV current symptom scores showed a significant group difference (F (2,54) = 62.328, p < 0.001, partial η 2 = 0.698) that diverged away from brain connectivity and sustained attention task performance results (Table 1, Fig. 2c). Post-hoc t tests revealed that the ADHD probands scored significantly higher than both their first-degree relatives [t (54) = 8.982, 95% CI (21.061–33.165), p < 0.001, partial η 2 = 0.599] and typically developing controls [t (54) = 10.229, 95% CI (24.816–36.916), p < 0.001, partial η 2 = 0.660]; however, the latter two groups did not show a significant difference to each other [t (54) = 1.241, 95% CI (−2.304 to 9.811), p = 0.220, partial η 2 = 0.028]. Similar results were obtained for the inattentive and hyperactive-impulsive sub-scales (online Supplementary Table S2).
Association of brain connectivity, cognition and ADHD symptomatology
Across all groups, the BAARS-IV current total symptom scores showed a significant correlation with PCC/PCUN to right MFG connectivity [partial r s = 0.405, 95% CI (0.168–0.598), p = 0.002] (Fig. 2d). Moreover, there was a significant negative correlation between the PCC/PCUN to right MFG connectivity and total hits in the RVP sustained attention task [partial r s = −0.360, 95% CI (−0.563 to −0.117), p = 0.006] (Fig. 2e), which was in turn negatively correlated with BAARS-IV current total symptom scores [partial r s = −0.456, 95% CI (−0.636 to −0.229), p < 0.001] (Fig. 2f, online Supplementary Table S3), corrected for age, gender and mean framewise displacement. Findings from the inattentive and hyperactive-impulsive sub-scales were in line with the main results (online Supplementary Tables S4 and S5). In addition, a mediation analysis indicated that there was a significant indirect effect of PCC/PCUN to right MFG connectivity on BAARS-IV total current symptom scores through the participants' performance (total hits) on the RVP sustained attention task [b = 8.054, 95% CI (0.920–19.3727)]. In other words, DMN connectivity was linked to ADHD symptom scores that were approximately 8.1 points higher as mediated by attentional performance.
Generalisability to an independent dataset
Similar to the results obtained from our main experimental study, the PCC/PCUN seed-based functional connectivity analysis revealed a DMN covering brain regions commonly associated with this network in the literature (Andrews-Hanna et al., Reference Andrews-Hanna, Smallwood and Spreng2014) (Fig. 3a). In the upper 50th percentile of this group of unrelated participants, who scored relatively high on ADHD symptoms, the same brain connection identified in our main experiment showed a significant correlation with DSM-oriented AD/H scores [partial r s = 0.341, 95% CI (0.07–0.565), p = 0.019], corrected for age, gender and mean framewise displacement (Fig. 3b). In other words, reduced anti-correlation between the PCC/PCUN and right MFG was associated with greater ADHD symptoms. Moreover, further analysis using the ASR DSM-oriented AD/H sub-scales indicated that the observed relationship was mainly originating from the inattentiveness scores [partial r s = 0.397, 95% CI (0.134–0.608), p = 0.006], whereas the hyperactivity scores showed no significant correlation with DMN connectivity [partial r s = 0.109, 95% CI (−0.174 to 0.375), p = 0.465]. Finally, a meta-analytic inquiry into the right MFG on the Neurosynth database revealed terms strongly associated with working memory, attentional load, task demands and other higher executive functions (Fig. 3c).
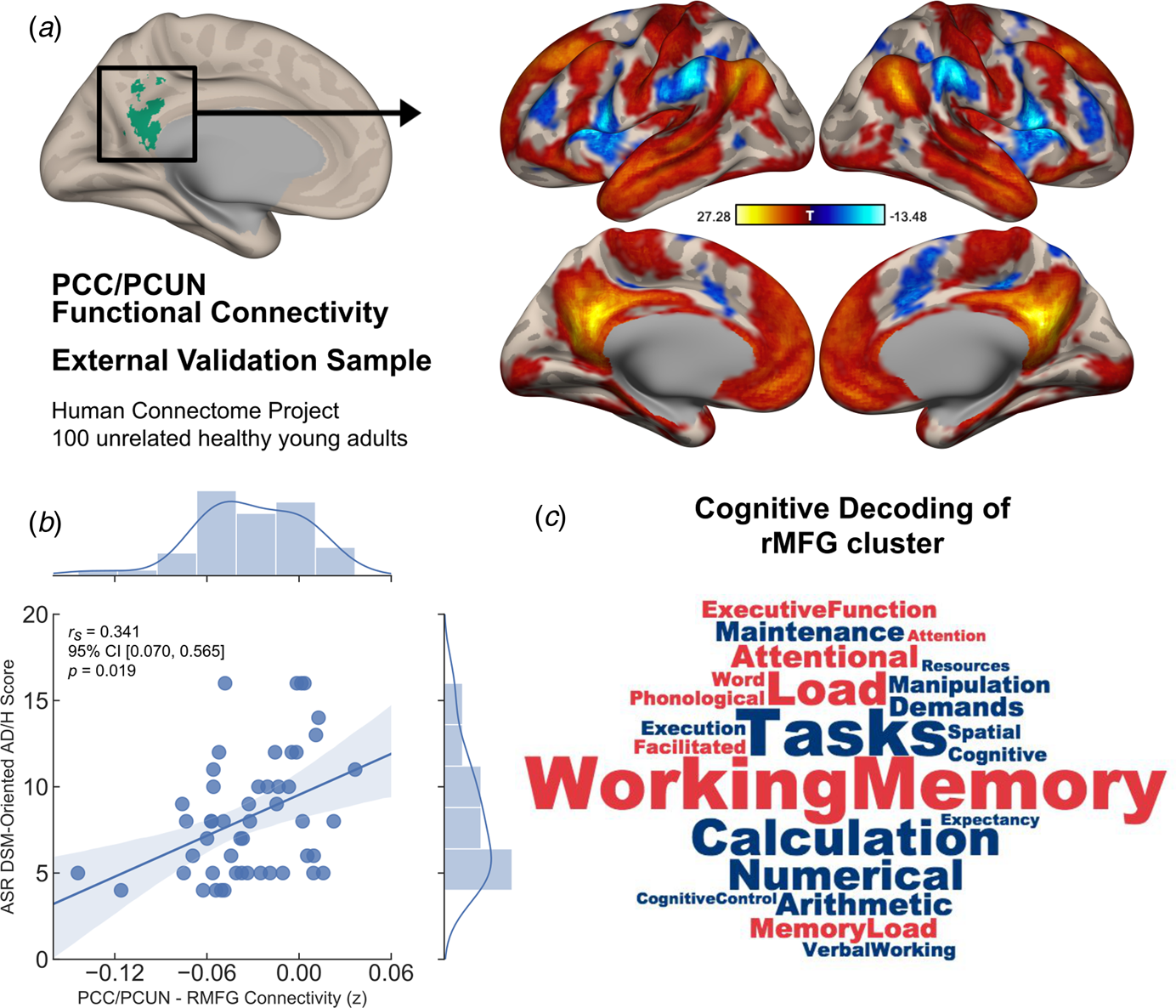
Fig. 3. Generalisability of the identified neurocognitive link as a dimensional trait in an independent sample of unrelated healthy young adults. (a) Default mode connectivity as revealed by PCC/PCUN seed-based functional interactions across two runs of 15-min resting-state sessions in a sample of 100 unrelated participants from the HCP showed a similar pattern of connectivity as identified in the main experimental study. (b) In the upper 50th percentile of high symptom scorers, Achenbach Adult Rating Scale (ASR) DSM-oriented AD/H scores and the PCC/PCUN anti-correlation with the right MFG cluster showed a significant link, in which reduced anti-correlation was associated with greater AD/H symptoms (r s = partial Spearman's correlation corrected for age, gender and mean framewise displacement). (c) Cognitive terms that were most commonly associated with activation in the right MFG was extracted from the Neurosynth database. This right hemispheric cluster depicted strong association with working memory, attentional load, task demands and other higher executive functions. While the font size of the terms indicates strength, the colour represents directionality of the loadings (red = positive, blue = negative). The shaded areas represent 95% CIs.
Discussion
The primary goal of this investigation was to assess whether the default mode functional interactions at rest would show shared alterations in adults with ADHD and their unaffected first-degree relatives as compared to typically developing controls, thus constituting a brain-based endophenotype of this disorder. Our main results revealed significantly reduced anti-correlation (or negative connectivity) of the chosen PCC/PCUN seed, a main hub of the DMN, with a cluster of brain regions centred on the right MFG extending into the IFG that is commonly associated with cognitive control. Moreover, across the whole cohort of participants, the identified functional connection was linked to ADHD symptom severity with performance in the sustained attention task significantly mediating this relationship. Finally, in an external validation step, we illustrated that the same brain connection identified in our main study also explained dimensional variability in ADHD symptoms in an independent cohort of unrelated healthy young adults, providing evidence for the generalisability of our findings. Taken together, such results collectively highlight the central importance of the identified default mode functional interaction as a familial neurocognitive trait in adults with ADHD, hence meeting the criteria for an endophenotype of this disorder.
Previous studies investigating resting-state brain functional connectivity alterations have highlighted DMN connectivity, specifically that of the PCC/PCUN, as a potential new locus of dysfunction in ADHD (Castellanos et al., Reference Castellanos, Kelly and Milham2009; Sonuga-Barke & Castellanos, Reference Sonuga-Barke and Castellanos2007). For example, in comparison with age and gender-matched healthy volunteers, Castellanos and colleagues reported on significantly reduced anti-correlations between the PCC/PCUN and dorsal anterior cingulate cortex in adults with ADHD (Castellanos et al., Reference Castellanos, Margulies, Kelly, Uddin, Ghaffari, Kirsch and Milham2008), which was further replicated in a group of male adolescents (Sun et al., Reference Sun, Cao, Long, Sui, Cao, Zhu and Wang2012). Though generally in line, our results instead revealed an impairment in the PCC/PCUN interaction with a cluster centred on the right MFG that is typically associated with a large-scale cognitive control system (Dosenbach, Fair, Cohen, Schlaggar, & Petersen, Reference Dosenbach, Fair, Cohen, Schlaggar and Petersen2008). This is further evidenced by this cluster's strong association with terms linked to higher cognitive processes in our meta-analytic decoding. Importantly, such connectivity alterations mirrored the observed group differences in performance during a sustained attention task, but also showed a stark contrast to the overt clinical phenotype. This ‘exophenotype’ remained in line with the existing diagnosis, revealing heightened symptoms in ADHD probands, which did not reach a diagnostic threshold in either the unaffected first-degree relatives or the typically developing controls, yet it still showed significant links with the identified neurocognitive endophenotypes.
Notably, the PCC/PCUN to MFG connectivity at rest showed a positive correlation with the current total symptoms and a negative correlation with sustained attention task performance, suggesting a close link between the DMN's functional interactions at rest and both inattention and overall symptomatology, which we replicated in an independent cohort of healthy young adults. Collectively, these functional and behavioural links emphasise the significance of the identified disconnection between the PCC/PCUN and right MFG as a neurocognitive trait, and thus a potential endophenotype of ADHD. Moreover, further mediation analysis revealed a significant indirect effect of DMN connectivity on ADHD symptom severity through the participants' performance in the sustained attention task. In a watershed model of biological pathways that link upstream genes to downstream observable phenotypes, Cannon and Keller have argued for the potential utility of identifying intermediary endophenotypes such as neural circuits and more broader cognitive processes in order to improve our understanding of the mechanisms behind complex mental health disorders (Cannon & Keller, Reference Cannon and Keller2006). In light of this analogy, our results indicate that alterations in the connectivity of the main DMN hub, namely the PCC/PCUN, may represent an upstream biological dysregulation. This aberrant connectivity in turn may lead to and be further influenced by downstream cognitive dysfunctions such as attentional problems that result in heightened ADHD symptoms. However, taken together with prior reports (Posner et al., Reference Posner, Park and Wang2014), it is clear that further research will be required to decipher the exact neural mechanisms behind the DMN functional interactions that are impaired in ADHD, specifically the relevance of anti-correlations between default mode and frontal brain regions to the neuropathophysiology of this disorder.
Since the initial report by Fox et al., (Reference Fox, Snyder, Vincent, Corbetta, Van Essen and Raichle2005), anti-correlation (or negative connectivity) in the spontaneous BOLD signal fluctuations in rs-fMRI data has been an important topic of debate in the neuroimaging literature. Specifically, the biological basis of this relationship remains largely unknown, with some reports advocating for its foundation in the employed computational techniques (Chai et al., Reference Chai, Castanon, Ongur and Whitfield-Gabrieli2012; Murphy et al., Reference Murphy, Birn, Handwerker, Jones and Bandettini2009). Nevertheless, emerging evidence now highlights the potential contribution of this functional interaction to healthy and adaptive brain processing (Fox, Zhang, Snyder, & Raichle, Reference Fox, Zhang, Snyder and Raichle2009; Uddin, Kelly, Biswal, Castellanos, & Milham, Reference Uddin, Kelly, Biswal, Castellanos and Milham2009), with important modifications observed across ageing (Spreng, Stevens, Viviano, & Schacter, Reference Spreng, Stevens, Viviano and Schacter2016) and in mental health disorders (Whitfield-Gabrieli et al., Reference Whitfield-Gabrieli, Thermenos, Milanovic, Tsuang, Faraone, McCarley and Seidman2009). In parallel, the observed modifications to the segregation between default mode and cognitive control networks have been suggested as a potential mechanism behind the attentional lapses in ADHD (Mills et al., Reference Mills, Miranda-Dominguez, Mills, Earl, Cordova, Painter and Fair2018; Posner et al., Reference Posner, Park and Wang2014). However, direct behavioural and functional evidence will be required to not only decipher the biological basis and cognitive relevance of default mode anti-correlations, but also their contribution to the attentional deficits in this disorder.
Originally described as a network of brain regions that showed task-induced deactivations during goal-oriented, attention-demanding paradigms (Mazoyer et al., Reference Mazoyer, Zago, Mellet, Bricogne, Etard, Houdé and Tzourio-Mazoyer2001; Raichle et al., Reference Raichle, MacLeod, Snyder, Powers, Gusnard and Shulman2001; Shulman et al., Reference Shulman, Fiez, Corbetta, Buckner, Miezin, Raichle and Petersen1997) and increased activity in autobiographical memory-based tasks (Addis, Wong, & Schacter, Reference Addis, Wong and Schacter2007), the role of the DMN in human cognition has long been confined to self-referential internal mentation processes such as mind-wandering or daydreaming (Andrews-Hanna et al., Reference Andrews-Hanna, Smallwood and Spreng2014). Combined with evidence relating lapses in attention to DMN activity (Weissman, Roberts, Visscher, & Woldorff, Reference Weissman, Roberts, Visscher and Woldorff2006), and alterations in the core DMN regions in ADHD (Castellanos et al., Reference Castellanos, Margulies, Kelly, Uddin, Ghaffari, Kirsch and Milham2008), the overarching hypothesis for the contribution of this large-scale network to ADHD symptomatology has been the excessive generation of mental content that potentially leads to the observed impairments in sustained attention (Posner et al., Reference Posner, Park and Wang2014).
On the other hand, our group has recently shown DMN engagement during the stable application of learned rules in an externally-oriented, attention-demanding cognitive flexibility task (Vatansever, Menon, & Stamatakis, Reference Vatansever, Menon and Stamatakis2017), which highlights the vital role of this network in core cognitive processes that include, yet transcend beyond internal mentation (Vatansever, Manktelow, Sahakian, Menon, & Stamatakis, Reference Vatansever, Manktelow, Sahakian, Menon and Stamatakis2018). Called the ‘autopilot hypothesis’, the potential role that the DMN might play in the maintenance of contextual-goals for fast and efficient processing while avoiding distractors, even that of our thoughts (Bozhilova et al., Reference Bozhilova, Michelini, Kuntsi and Asherson2018), might provide a fresh perspective on the DMN's dysfunction in ADHD. In fact, along these lines, we recently provided evidence for the link between impaired context-regulation of thoughts, brain connectivity and ADHD symptom severity (Vatansever, Bozhilova, Asherson, & Smallwood, Reference Vatansever, Bozhilova, Asherson and Smallwood2018), highlighting the heterogeneity of this association. In sum, resting-state aberrations found in this study might represent altered DMN contribution to core cognitive and attentional processes.
In relation to the findings of this study, a few limitations should be highlighted. First of all, the results of our study are based on a single brain region within a larger default mode system. Although we used an objective approach to define this seed and focused on a DMN hub that was previously implicated in ADHD, in light of recent evidence indicating functional sub-specialisation within this network (Kernbach et al., Reference Kernbach, Yeo, Smallwood, Margulies, Thiebaut de Schotten, Walter and Bzdok2018), there might be broader ADHD related alterations in the functional interaction of the DMN that should be further considered. For that purpose, other analysis techniques such as independent component analysis (Beckmann, Mackay, Filippini, & Smith, Reference Beckmann, Mackay, Filippini and Smith2009) or the assessment of multivariate interactions of the DMN within the wider complex brain network architecture via graph theoretical analyses might prove important avenues for future research (Fornito & Bullmore, Reference Fornito and Bullmore2015). In addition, our study focused on resting-state alterations in brain connectivity that was related to cognitive performance outside the scanner with a computerised task. Given recent evidence that suggests task-based modifications to the interaction of large-scale systems (Vatansever, Menon, Manktelow, Sahakian, & Stamatakis, Reference Vatansever, Menon, Manktelow, Sahakian and Stamatakis2015), it will be pertinent to directly assess the contribution of default mode interactions to attentional task performance in ADHD. Finally, it is important to note that although all of our analyses were corrected for factors such as age and gender, prior studies do indicate certain characteristic differences related to the subtypes and comorbidities in children with ADHD, that are suggested to normalise in adults (Rucklidge, Reference Rucklidge2010; Williamson & Johnston, Reference Williamson and Johnston2015). Hence, future research should explicitly test the potential age and gender effects on the brain-based endophenotypes of ADHD. Notwithstanding, the results of our study highlight shared alterations in resting-state DMN connectivity between ADHD probands and their unaffected first-degree relatives that might have a role into modulating both overall symptoms and inattention.
Overall, this study suggests that the abnormal DMN connectivity is a significant aspect of the adult ADHD neuropathophysiology that could be employed as a latent neurocognitive trait to enhance statistical power of genetic studies in adult ADHD and as a surrogate efficacy endpoint in the research and development of novel pharmaceuticals for treatment. In addition to previous research showing volumetric differences in cortical grey matter and aberrant white matter connections in ADHD (Pironti et al., Reference Pironti, Lai, Muller, Dodds, Suckling, Bullmore and Sahakian2014) the identification of candidate genes linked to neuronal growth, myelination, maintenance and protection (Franke et al., Reference Franke, Faraone, Asherson, Buitelaar, Bau and Ramos-Quiroga2012) has strengthened the hypothesis that the observed deficits in ADHD may arise from developmental abnormalities in cortical maturation and pruning that persist into adulthood (Shaw et al., Reference Shaw, Eckstrand, Sharp, Blumenthal, Lerch, Greenstein and Rapoport2007). Such developmental differences may underlie the sustained attention deficits observed in adults with ADHD (Martinussen, Hayden, Hogg-Johnson, & Tannock, Reference Martinussen, Hayden, Hogg-Johnson and Tannock2005), which seem to benefit from methylphenidate administration (Turner et al., Reference Turner, Blackwell, Dowson, McLean and Sahakian2005) that also normalises DMN alterations (Silk, Malpas, Vance, & Bellgrove, Reference Silk, Malpas, Vance and Bellgrove2016). However, more extensive investigations linking physiological and behavioural endophenotypes with susceptibility genes will be required to find causal interactions. To this end, further research aiming to integrate large-scale datasets (Milham, Fair, Mennes, & Mostofsky, Reference Milham, Fair, Mennes and Mostofsky2012) will be necessary to further characterise the potential endophenotypes of ADHD.
Supplementary material
The supplementary material for this article can be found at https://doi.org/10.1017/S0033291719003374
Acknowledgements
We thank Mrs Victoria Lupson and the rest of the staff in the Wolfson Brain Imaging Centre (WBIC) at Addenbrooke's Hospital for their assistance in MRI scanning of the original participant cohort. In addition, we thank all the participants for their contribution to this study. All raw data for the main experiment is archived at the Wolfson Brain Imaging Centre, Cambridge and Division of Anaesthesia, School of Clinical Medicine, University of Cambridge, Cambridge, UK. Furthermore, data were provided (in part) by the Human Connectome Project, WU-Minn Consortium (Principal Investigators: David Van Essen and Kamil Ugurbil; 1U54MH091657) funded by the 16 NIH Institutes and Centers that support the NIH Blueprint for Neuroscience Research; and by the McDonnell Center for Systems Neuroscience at Washington University.
Financial support
This work was funded by a Core Award from the Medical Research Council and the Wellcome Trust to the Behavioural and Clinical Neuroscience Institute (MRC Ref G1000183; WT Ref 093875/Z/10/Z). VAP was supported by a Medical Research Council Doctoral Training Grant. DV was supported by the Shanghai Municipal Science and Technology Major Project (No. 2018SHZDZX01). BJS was funded by the National Institute of Health Research Biomedical Research Centre (Mental Health Theme).
Conflict of interest
BJS is a consultant for Cambridge Cognition, PEAK and Mundipharma.
Ethical standards
The authors assert that all procedures contributing to this work comply with the ethical standards of the relevant national and institutional committees on human experimentation and with the Helsinki Declaration of 1975, as revised in 2008.