Background
The incidence of psychotic disorders is not evenly distributed across different geographical and social conditions, but patterned across socioenvironmental gradients such as urbanicity (van Os, Linscott, Myin-Germeys, Delespaul, & Krabbendam, Reference van Os, Linscott, Myin-Germeys, Delespaul and Krabbendam2009; Vassos, Pedersen, Murray, Collier, & Lewis, Reference Vassos, Pedersen, Murray, Collier and Lewis2012), deprivation (Kirkbride, Jones, Ullrich, & Coid, Reference Kirkbride, Jones, Ullrich and Coid2014; O'Donoghue, Roche, & Lane, Reference O'Donoghue, Roche and Lane2016), crime (Bhavsar, Boydell, Murray, & Power, Reference Bhavsar, Boydell, Murray and Power2014), and air pollution (Gao, Xu, Guo, Fan, & Zhu, Reference Gao, Xu, Guo, Fan and Zhu2017; Horsdal et al., Reference Horsdal, Agerbo, McGrath, Vilhjálmsson, Antonsen, Closter and Webb2019). Consistent with this literature, this team has previously shown that subclinical psychotic experiences, such as hearing voices and paranoia, are also more common among children and teenagers raised in urban, deprived, dangerous, and polluted environments v. those raised in more advantaged settings (Newbury et al., Reference Newbury, Arseneault, Caspi, Moffitt, Odgers and Fisher2016, Reference Newbury, Arseneault, Caspi, Moffitt, Odgers, Baldwin and Fisher2017, Reference Newbury, Arseneault, Caspi, Moffitt, Odgers and Fisher2018, Reference Newbury, Arseneault, Beevers, Kitwiroon, Roberts, Pariante and Fisher2019; Polanczyk et al., Reference Polanczyk, Moffitt, Arseneault, Cannon, Ambler, Keefe and Caspi2010). Early psychotic experiences overlap symptomatically and etiologically with adult psychotic disorders (Polanczyk et al., Reference Polanczyk, Moffitt, Arseneault, Cannon, Ambler, Keefe and Caspi2010; Poulton et al., Reference Poulton, Caspi, Moffitt, Cannon, Murray and Harrington2000; van Os et al., Reference van Os, Linscott, Myin-Germeys, Delespaul and Krabbendam2009), which makes them a valuable and important phenotype for researchers and clinicians.
Socioenvironmental exposures could plausibly increase the risk for psychosis via various non-mutually exclusive mechanisms, such as by promoting psychosocial stress and disrupting neurocognitive development (Alvarez, Kubzansky, Campen, & Slavich, Reference Alvarez, Kubzansky, Campen and Slavich2018; Howes & Murray, Reference Howes and Murray2014; Lederbogen et al., Reference Lederbogen, Kirsch, Haddad, Streit, Tost, Schuch and Deuschle2011; van Winkel, Stefanis, & Myin-Germeys, Reference van Winkel, Stefanis and Myin-Germeys2008). An enduring question, however, is whether associations of socioenvironmental risk factors with psychosis are truly causal. The process by which people ‘choose’ where to live is not random: it is influenced by our individual characteristics and resources. These selection processes likely result in compositional differences between individuals living in advantaged v. disadvantaged environments. For example, functional impairment due to high genetic risk could lead individuals to drift downward in social mobility into more crowded and impoverished settings. This selection process could occur over an individual's lifetime (intra-generational), as well as across generations (inter-generational) (Solmi, Lewis, Zammit, & Kirkbride, Reference Solmi, Lewis, Zammit and Kirkbride2019), and thereby confound the observed associations between the environment and psychosis.
Findings from Scandinavian registry data (Mortensen et al., Reference Mortensen, Pedersen, Westergaard, Wohlfahrt, Ewald, Mors and Melbye1999; Pedersen, Reference Pedersen2001) have long shown that associations between urbanicity and psychosis are not confounded by family psychiatric history, the workhorse measure of genetic risk. However, family history captures only the diagnosed fraction of genetic risk for psychopathology (Yang, Visscher, & Wray, Reference Yang, Visscher and Wray2010). Polygenic risk scores (PRS) – which aggregate the effects of thousands of genetic variants to index an individual's genetic risk for a phenotype (Dudbridge, Reference Dudbridge2013; Plomin, Haworth, & Davis, Reference Plomin, Haworth and Davis2011) – offer new opportunities to examine the role of genetics in the link between socioenvironmental risk and psychosis. A handful of recent studies have explored this question. Higher schizophrenia PRS has been associated with urbanicity and deprivation in adulthood (Colodro-Conde et al., Reference Colodro-Conde, Couvy-Duchesne, Whitfield, Streit, Gordon, Kemper and de Zeeuw2018; Sariaslan et al., Reference Sariaslan, Fazel, D'Onofrio, Långström, Larsson, Bergen and Lichtenstein2016), and with deprivation and air pollution at birth (Horsdal et al., Reference Horsdal, Agerbo, McGrath, Vilhjálmsson, Antonsen, Closter and Webb2019; Solmi et al., Reference Solmi, Lewis, Zammit and Kirkbride2019). In contrast, other studies have reported null associations between PRS and urbanicity at birth (Paksarian et al., Reference Paksarian, Trabjerg, Merikangas, Mors, Børglum, Hougaard and Agerbo2018; Solmi et al., Reference Solmi, Lewis, Zammit and Kirkbride2019). Furthermore, we recently identified consistent associations between PRS for education and ecological risk in childhood; but associations were inconsistent for PRS for schizophrenia (Belsky et al., Reference Belsky, Caspi, Arseneault, Corcoran, Domingue, Harris and Prinz2019). Findings remain equivocal. Therefore, the present study addressed this topic by examining the association between genetic risk and socioenvironmental risk for schizophrenia during upbringing.
Like family history, PRS are imperfect measures of genetic risk. Complex traits are polygenic, and PRS currently capture only a small portion of this genetic variance (Ripke et al., Reference Ripke, Neale, Corvin, Walters, Farh, Holmans and Huang2014; Wray et al., Reference Wray, Ripke, Mattheisen, Trzaskowski, Byrne and Abdellaoui2018); while at the same time confirming the presence of substantial pleiotropy between different disorders like psychosis and depression (Legge et al., Reference Legge, Jones, Kendall, Pardiñas, Menzies, Bracher-Smith and Hotopf2019; Pain et al., Reference Pain, Dudbridge, Cardno, Freeman, Lu, Lundstrom and Ronald2018). Therefore, this study incorporated five genetic risk indices, including schizophrenia PRS, depression PRS, maternal psychotic symptoms, family psychiatric history, and latent genetic risk scores derived using the twin design. This allowed us to contrast findings between PRS, which are partial through direct measures of genetic risk that are uninfluenced by the environment, with family- and twin-based measures, which capture risk more comprehensively but reflect both genetic and environmental influences. Furthermore, as well as area-level features like urbanicity, our study included detailed, high-resolution measures of neighborhood- and family-level risks that are typically enriched in cities. Based on our previous findings, measures included urbanicity, air pollution, neighborhood deprivation, neighborhood crime, neighborhood disorder, neighborhood social cohesion, residential mobility, and family poverty. Finally, rather than focusing on one time-point (e.g. birth or adulthood), our study spanned birth to age 18. Our rationale was that socioenvironmental exposures early in life, rather than in adulthood, often associate with psychosis most strongly (Pedersen & Mortensen, Reference Pedersen and Mortensen2001), meaning that interventions during childhood and adolescence hold the best promise for improving mental health outcomes (Davidson, Grigorenko, Boivin, Rapa, & Stein, Reference Davidson, Grigorenko, Boivin, Rapa and Stein2015). Additionally, children v. young adults have relatively little choice about where they live, meaning that intra-generational drift may increase across development. A focus from birth to adolescence will therefore highlight whether correlations between genetic and environmental risk indicate (a) reverse causation in which genetic risk leads adults into riskier environments, meaning that addressing risks would have little impact on psychosis outcome; or (b) that genetic risk influences exposure to causal risk factors, in which case addressing risks would reduce young people's risk for psychosis.
Methods
Study cohort
Participants were members of the Environmental Risk (E-Risk) Longitudinal Twin Study, which tracks the development of a nationally representative birth cohort of 2232 twin children born in 1994–1995 across England and Wales and initially assessed at age 5. Follow-up home-visits were conducted when participants were aged 7, 10, 12 and 18 (participation rates were 98%, 96%, 96% and 93%, respectively). At age 18, the E-Risk sample included 2066 participants, comprising 56% monozygotic (MZ) twin pairs and 49% males. There were no differences between those who did and did not take part at age 18 in terms of age-5 socioeconomic status (SES) (χ2 = 0.86; p = 0.65), age-5 IQ scores (t = 0.98; p = 0.33), or age-5 internalizing or externalizing behavioral problems (t = 0.40; p = 0.69 and t = 0.41; p = 0.68, respectively). E-Risk families are representative of UK households across the spectrum of neighborhood socioeconomic conditions according to consumer-classification systems (CACI Information Services, 2006): at follow-up, 27% of E-Risk participants lived in ‘wealthy achiever’ neighborhoods compared to 25% of households nationwide; 7% v. 12% lived in ‘urban prosperity’ neighborhoods; 28% v. 27% lived in ‘comfortably off’ neighborhoods; 13% v. 14% lived in ‘moderate means’ neighborhoods; and 26% v. 21% lived in ‘hard-pressed’ neighborhoods (Odgers et al., Reference Odgers, Caspi, Russell, Sampson, Arseneault and Moffitt2012b). Likewise, E-Risk families are representative of UK households according to the Index of Multiple Deprivation (online Supplementary Figure 1). The Joint South London and Maudsley and the Institute of Psychiatry Research Ethics Committee approved each phase of the study. Parents gave written informed consent and participants gave written assent at ages 5–12 and written informed consent at age 18. Further details about the sample are reported elsewhere (Moffitt, Reference Moffitt2002) and in the Supplementary Materials. Table 1 displays characteristics for the analysis sample, together with information on missing data.
Table 1. Sample characteristics and missing data
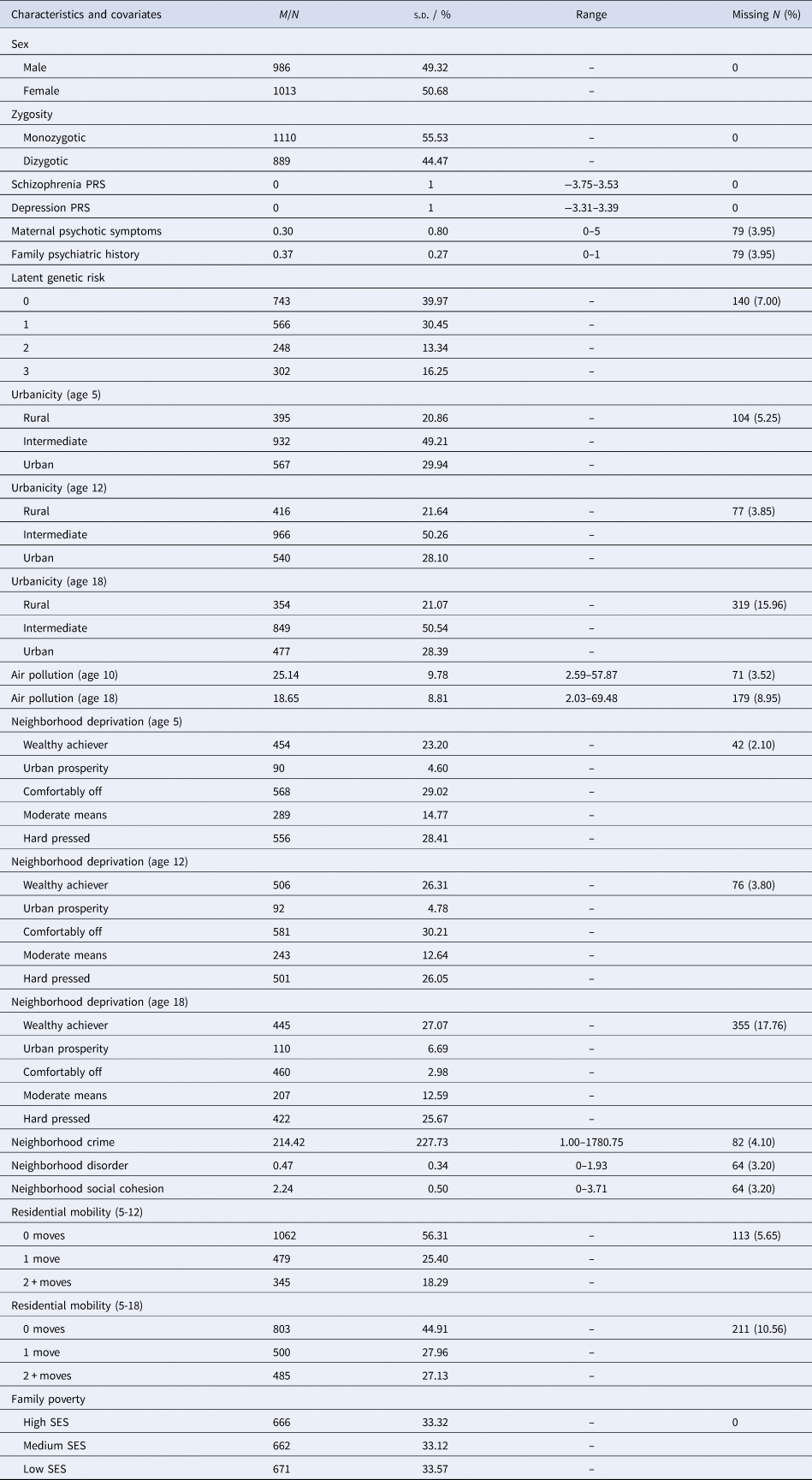
Note: M, mean; N, number; PRS, polygenic risk score; s.d., standard deviation; SES, socioeconomic status.
Measures
Genotyping participants
We used Illumina HumanOmni Express 24 BeadChip arrays (Version 1.1; Illumina, Hayward, CA) to assay common single-nucleotide polymorphism (SNP) variation in the genomes of cohort members. The resulting database was restricted to SNPs called successfully in >98% of the cohort and in Hardy-Weinberg equilibrium (p > 0.001). We imputed additional SNPs using the IMPUTE2 software (Version 2.3.1; https://mathgen.stats.ox.ac.uk/impute/impute_v2.html; Howie, Donnelly, & Marchini, Reference Howie, Donnelly and Marchini2009) and the 1000 Genomes Phase 3 reference panel (Genomes Project Consortium, 2015). Imputation was conducted on autosomal SNPs appearing in dbSNP (Version 140; http://www.ncbi.nlm.nih.gov/SNP/; Sherry et al., Reference Sherry, Ward, Kholodov, Baker, Phan, Smigielski and Sirotkin2001) that were ‘called’ in more than 98% of the samples. Invariant SNPs were excluded. The E-Risk cohort contains MZ twins, who are genetically identical; we therefore empirically measured genotypes of one randomly selected twin per pair and assigned these data to their MZ co-twin. We directly measured genotypes of both members of dizygotic (DZ) twin pairs. Prephasing and imputation were conducted using a 50-million-base-pair sliding window. The resulting genotype databases included genotyped SNPs and SNPs imputed with 90% probability of a specific genotype among the European descent members of the E-Risk cohort (N = 1999 participants in 1011 families). Note that the analysis sample for the present study included only ethnically White participants because PRS currently has uncertain validity in non-European descent populations (Martin et al., Reference Martin, Gignoux, Walters, Wojcik, Neale, Gravel and Kenny2017; Vassos et al., Reference Vassos, Di Forti, Coleman, Iyegbe, Prata, Euesden and Patel2017).
Polygenic risk scores
PRS were created for European descent E-Risk participants following the method described by Dudbridge (Reference Dudbridge2013) using PRSice (Version 1.22; http://prsice.info/; Euesden, Lewis, and O'Reilly, Reference Euesden, Lewis and O'Reilly2014). For schizophrenia PRS, SNPs reported in the results of the latest GWAS for schizophrenia (Pardiñas et al., Reference Pardiñas, Holmans, Pocklington, Escott-Price, Ripke, Carrera and Hamshere2018) were matched with SNPs in the E-Risk cohort, irrespective of nominal significance with schizophrenia. We then performed clumping by retaining the SNP with the smallest p value from each LD block (excluding SNPs with r 2 > 0.1 in 500-kb windows), then weighted retained SNPs by effect estimate. To control for possible population stratification, we conducted a principal component analysis of our genome-wide SNP database using PLINK (Version 1.9; Chang et al., Reference Chang, Chow, Tellier, Vattikuti, Purcell and Lee2015). One twin was selected at random from each family for principal component analysis. SNP loadings for principal components were applied to co-twin genetic data to compute principal component values for the full sample. The 10 principal components explained 2.8% of the variance in the schizophrenia PRS. We residualized polygenic scores for the first 10 principal components estimated from the genome-wide SNP data. The residualized scores were normally distributed. We standardized residuals for analysis (M = 0, s.d. = 1, range = −3.75–3.53). For depression PRS, SNPs reported in the latest GWAS for major depression (Wray et al., Reference Wray, Ripke, Mattheisen, Trzaskowski, Byrne and Abdellaoui2018) were matched with SNPs in the E-Risk cohort, irrespective of nominal significance with depression. Clumping and principal components analysis were conducted as above. Residualized scores were normally distributed, and these were once again standardized for analysis (M = 0, s.d. = 1, range = −3.31–3.39).
Maternal psychotic symptoms and family psychiatric history
Maternal psychotic symptoms and family history (Table 1) were assessed in private interviews with the mothers when children were aged 12. For maternal psychotic symptoms, mothers were interviewed using the Diagnostic Interview Schedule (DIS; Robins, Cottler, Bucholz, and Compton, Reference Robins, Cottler, Bucholz and Compton1995) for DSM-IV (American Psychiatric Association, 1994) which provides a symptom count for characteristic symptoms of schizophrenia (e.g. hallucinations, delusions, anhedonia). For family psychiatric history, mothers reported on their own mental health history and the mental health history of their biological mothers, fathers, sisters, brothers, and the twins' biological father (Weissman et al., Reference Weissman, Wickramaratne, Adams, Wolk, Verdeli and Olfson2000). This was converted into the proportion of family members with a history of psychiatric disorder (Milne et al., Reference Milne, Moffitt, Crump, Poulton, Rutter, Sears and Caspi2008).
Latent genetic risk scores
Latent genetic risk scores were derived based on each participant's zygosity and whether their co-twin had psychotic experiences. As described by Arseneault et al. (Reference Arseneault, Cannon, Fisher, Polanczyk, Moffitt and Caspi2011), each twin is represented in the data twice, first as the target and then as the co-twin. A continuum of genetic risk is generated, on the basis that MZ twins are ~100% genetically identical, whereas DZ twins share ~50% of the same genes. Thus, we assigned a score of 0 to participants if their MZ co-twin did not have psychotic experiences; a score of 1 to participants if their DZ co-twin did not have psychotic experiences; a score of 2 to participants if their DZ twin did have psychotic experiences; and a score of 3 to participants if their MZ twin did have psychotic experiences.
Adolescent psychotic experiences
At age 18, each E-Risk participant was privately interviewed by a research worker about 13 psychotic experiences occurring since age 12. Seven items referred to delusions and hallucinations (Polanczyk et al., Reference Polanczyk, Moffitt, Arseneault, Cannon, Ambler, Keefe and Caspi2010), such as ‘have you ever thought you were being watched, followed or spied on?’ and ‘do you hear voices that others cannot?’. Six items referred to unusual experiences which drew on item pools since formalized in prodromal psychosis instruments including the PRIME-screen and SIPS (Loewy, Pearson, Vinogradov, Bearden, & Cannon, Reference Loewy, Pearson, Vinogradov, Bearden and Cannon2011), such as ‘I believe I have special abilities or powers beyond my natural talents’. Further information on this measure is provided in Supplementary Materials. Research workers coded each item 0, 1, or 2 indicating respectively: ‘not present’, ‘probably present’ and ‘definitely present’. All 13 items were summed (M = 1.19, s.d. = 2.58, range = 0–18), and scores were placed into an ordinal scale, corresponding to the number of different psychotic experiences rather than frequency of occurrence. Just over 30% of participants had at least one psychotic experience between ages 12 and 18: 69.8% reported no psychotic experiences (coded 0; n = 1440), 15.5% reported one or two psychotic experiences (coded 1; n = 319), 8.1% reported three–five psychotic experiences (coded 2; n = 166), and 6.7% reported six or more psychotic experiences (coded 3; n = 138). This is similar to the prevalence of psychotic experiences self-reported in other general population non-twin samples (Spauwen, Krabbendam, Lieb, Wittchen, & van Os, Reference Spauwen, Krabbendam, Lieb, Wittchen and van Os2004; Yoshizumi, Murase, Honjo, Kaneko, & Murakami, Reference Yoshizumi, Murase, Honjo, Kaneko and Murakami2004; Yung et al., Reference Yung, Nelson, Baker, Buckby, Baksheev and Cosgrave2009).
Socioenvironmental risks
Socioenvironmental risk variables are described below, in Fig. 1, and in the Supplementary Materials. Some variables were on ordinal scales and some were continuous. All continuous variables were placed into ordinal scales to allow consistent analyses and comparison between variables. Categorizations were based on official classifications and/or precedent. Urbanicity, neighborhood deprivation, and air pollution were available at several time-points. Therefore, we included data from multiple time-points across childhood and adolescence to explore temporal changes in associations.
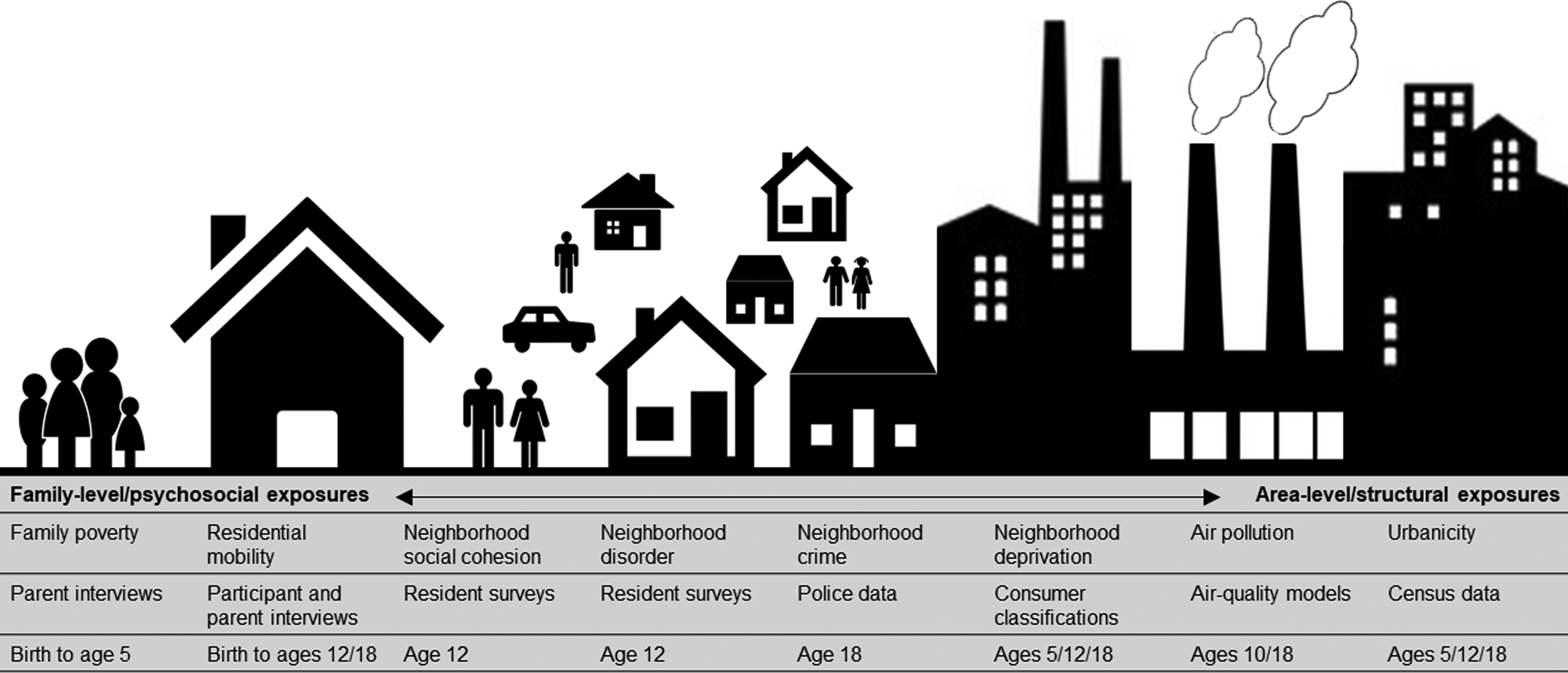
Fig. 1. Illustration of the nature and source of environmental risk variables used in this study.
Urbanicity was derived from classifications from 2011 census data (Office for National Statistics, 2013) and linked to participants' home postcodes at ages 5, 12, and 18. Classifications incorporate data on residential density, settlement size and population sparsity. Resolution is at the Lower Layer Super Output Area (LSOA) level, which contain an average of 1500 residents. A three-level urbanicity variable was used representing rural, intermediate and urban settings (Table 1). E-Risk participants are nationally-representative in terms of ONS urbanicity classifications; for instance, the nationwide distribution of rural, intermediate, and urban residents in the UK is 18.9%, 45.0%, and 36.1%, respectively.
Air pollution exposure was measured using a coupled regional chemical transport model and street-scale dispersion model (Beevers, Kitwiroon, Williams, & Carslaw, Reference Beevers, Kitwiroon, Williams and Carslaw2012; Carslaw, Reference Carslaw2011). Based on our previous findings in this cohort (Newbury et al., Reference Newbury, Arseneault, Beevers, Kitwiroon, Roberts, Pariante and Fisher2019), the present study focusses on nitrogen dioxide (NO2) exposure. Annualized estimates of ambient NO2 were linked to participants' home postcodes at ages 10 and 18. The model achieves 20 × 20 meter resolution. For this study, annualized NO2 exposure was categorized into quartiles (1 = least polluted; 4 = most polluted).
Neighborhood deprivation was constructed using A Classification of Residential Neighborhoods (ACORN), a geodemographic discriminator developed by CACI Information Services (CACI Information Services; http://www.caci.co.uk/). Detailed information about ACORN's classification of neighborhood-level socioeconomic-status (SES) has been provided previously (Caspi, Taylor, Moffitt, & Plomin, Reference Caspi, Taylor, Moffitt and Plomin2000; Odgers et al., Reference Odgers, Moffitt, Tach, Sampson, Taylor, Matthews and Caspi2009, Reference Odgers, Caspi, Russell, Sampson, Arseneault and Moffitt2012b). Briefly, CACI utilized over 400 census variables for Great Britain (e.g. educational qualifications, unemployment, housing tenure) and CACI's consumer lifestyle database. Classifications ranged from ‘Wealthy Achiever’ (coded 1) to ‘Hard Pressed’ (coded 5) neighborhoods (Table 1). Each family in our sample was matched to the ACORN code for its neighborhood via postcodes at ages 5, 12, and 18 (Caspi et al., Reference Caspi, Taylor, Moffitt and Plomin2000).
Neighborhood crime was measured using police data for England and Wales for 2011 and linked to participants' home postcodes at age 18. The total number of crimes occurring each month within a one-mile radius of each participant's home was tallied (Table 1). Scores were placed into quartiles for analysis (1 = lowest crime; 4 = highest crime).
Neighborhood disorder and social cohesion were measured via resident reports sent to >5000 immediate neighbors of participants in 2008 (Odgers et al., Reference Odgers, Moffitt, Tach, Sampson, Taylor, Matthews and Caspi2009; Odgers, Caspi, Bates, Sampson, & Moffitt, Reference Odgers, Caspi, Bates, Sampson and Moffitt2012a). Items enquired about physical and social signs of threat in the neighborhood (neighborhood disorder) and the quality and quantity of social interactions between neighbors (social cohesion). Items were averaged across each neighborhood characteristic and across respondents from the same neighborhood, and linked to home postcodes at age 12 (Table 1). Scores were grouped into tertiles for analysis at the 33rd and 66th centile (1 = lowest neighborhood disorder/highest social cohesion; 3 = highest neighborhood disorder/lowest social cohesion).
Residential mobility was measured from birth to age 18 via parent (ages 5–12) and participant (age 18) interviews. Residential moves were summed across phases 5–12 and phases 5–18, and collapsed into three categories including 1 (no moves), 2 (1 move), and 3 (2 or more moves) (Table 1).
Family poverty (SES) was measured as a composite of parental income, education, and occupation via interviews with mothers when children were aged 5. The latent variable was categorized into tertiles (i.e. low-, medium-, and high-SES; Trzesniewski, Moffitt, Caspi, Taylor, and Maughan, Reference Trzesniewski, Moffitt, Caspi, Taylor and Maughan2006; Table 1). The present study uses a reverse-scored measure from 1 (high-SES) to 3 (low-SES).
Cumulative environmental risk scale
To compare participants with the most v. least disadvantaged upbringings we created a cumulative environmental risk scale by summing the top scores of each of the socioenvironmental variables. For analyses with the genetic risk indices, the variable included data up to age 18. For analyses with psychotic experiences, the variable included data only up to age 12 to ensure temporal ordering of exposures and outcome. For risk factors measured at multiple phases (urbanicity, deprivation, NO2) we averaged scores across phases prior to constructing the cumulative scale. Percentages of participants exposed to 0, 1, 2, 3, 4 or 5 or more risks up to age 12 were: 26.5%, 20.8%, 16.7%, 15.2%, 10.9% and 10.0%; and up to age 18 were; 22.5%, 21.0%, 15.8%, 13.7%, 11.4%, and 15.6%, respectively (scores of 5–8 risks were collapsed due to small numbers within these categories).
Statistical analyses
We conducted the analyses using Stata (v16.0) following three steps. First, we used ordinal logistic regression to examine associations of the genetic risk indices with adolescent psychotic experiences. Second, we used ordinal logistic regression to examine associations of the genetic risk indices with the socioenvironmental risk variables, with the genetic risk indices treated as the independent variables. For step 2, one twin per each MZ twin pair was randomly dropped, because MZ twins correlate ~100% for their genetic sequence and typically share the same neighborhood environments during childhood. Alongside steps 1 and 2, we plotted genetic risk Z-scores across psychotic experience scores and the cumulative environmental risk scale. Third, we used ordinal logistic regression to examine the associations of socioenvironmental risks from birth to age 12 with adolescent psychotic experiences and added the genetic risk indices as covariates to examine attenuation of effects. All genetic risk indices were standardized for analysis (i.e. M = 0, s.d. = 1) to allow comparison between measures. Steps 1–3 accounted for the non-independence of twin observations using the cluster command in Stata. This procedure is derived from the Huber-White variance estimator and provides robust standard errors adjusted for within-cluster correlated data (Rogers, Reference Rogers1994). Analyses were conducted following multiple imputation using chained equations to handle missing covariate data (described further in the Supplementary Materials). Multiple imputations were only conducted for the 1999 White, genotyped participants because schizophrenia PRS currently has uncertain validity in non-European descent populations (Martin et al., Reference Martin, Gignoux, Walters, Wojcik, Neale, Gravel and Kenny2017; Vassos et al., Reference Vassos, Di Forti, Coleman, Iyegbe, Prata, Euesden and Patel2017). Complete case analyses are reported in the Supplementary Materials.
Results
Is genetic risk associated with adolescent psychotic experiences?
Adolescents with higher depression PRS (OR = 1.17, 95% CI = 1.05–1.31, p = 0.005), maternal psychotic symptoms (OR = 1.15, 95% CI = 1.05–1.26, p = 0.002), family psychiatric history (OR = 1.32, 95% CI = 1.18–1.47, p < 0.001) and latent genetic risk (OR = 1.71, 95% CI = 1.49–1.96, p < 0.001) tended to report more psychotic experiences than those with lower genetic risk. However, the association between schizophrenia PRS and adolescent psychotic experiences failed to meet conventional levels of statistical significance (OR = 1.12, 95% CI = 1.00–1.25, p = 0.052). Figure 2a illustrates these associations with genetic risk Z-scores plotted across psychotic experience scores.
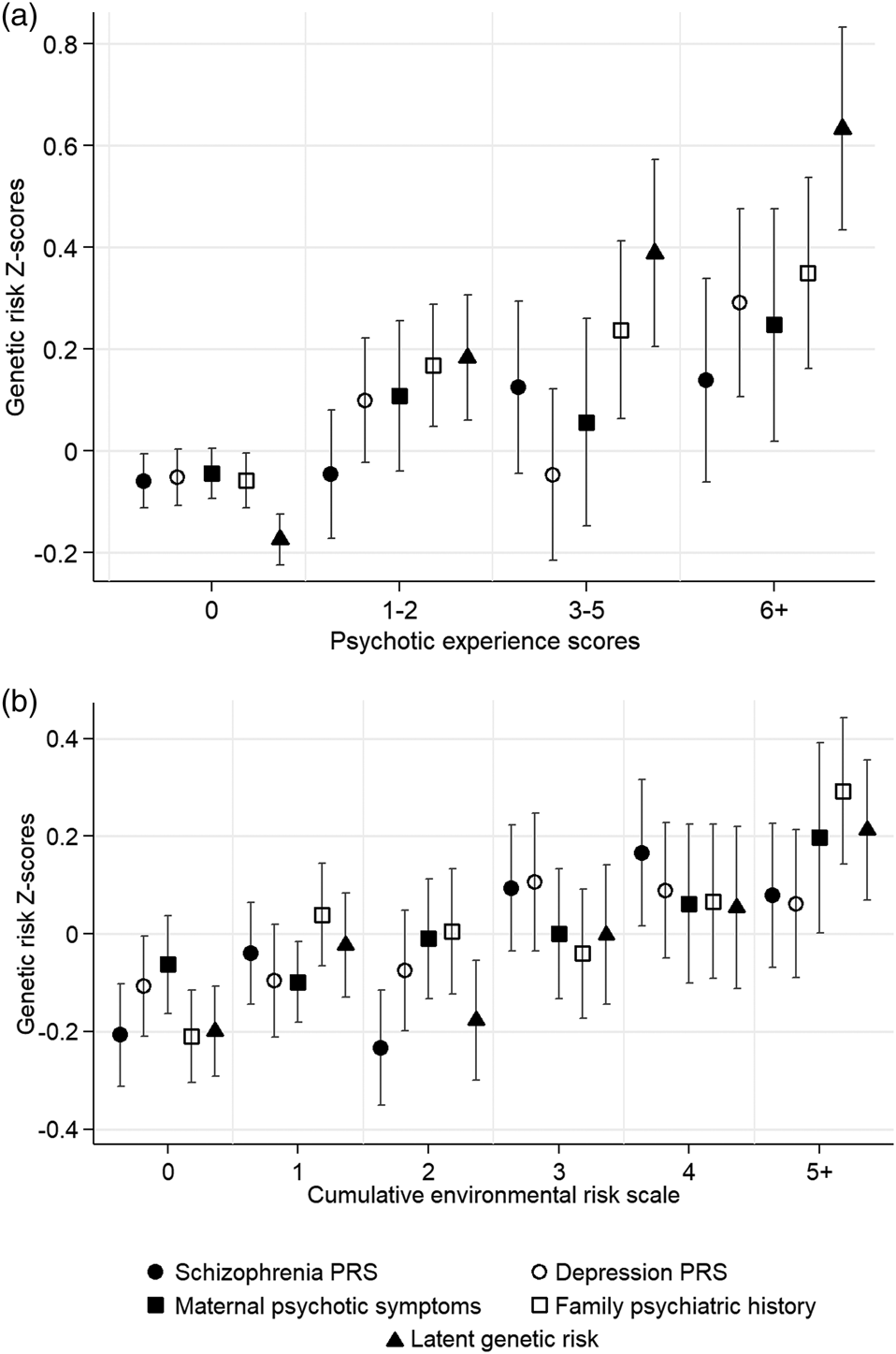
Fig. 2. Genetic risk Z-scores and 95% confidence intervals (indicated by double-headed lines) across (a) adolescent psychotic experiences scores, and (b) the cumulative environmental risk scale. Note: PRS, polygenic risk score.
Is genetic risk associated with socioenvironmental risk for schizophrenia during upbringing?
Figure 2b plots genetic risk Z-scores across the cumulative environmental risk scale and shows that participants with higher genetic risk tended to experience more socioenvironmental risks for schizophrenia during upbringing. Table 2 displays odds ratios for these associations. Schizophrenia PRS was associated at p < 0.05 with the cumulative environmental risk scale, age-18 urbanicity, neighborhood crime, neighborhood disorder, residential mobility, and family poverty (all OR's >1.12). Depression PRS was associated at p < 0.05 with the cumulative environmental risk scale, age-12 and age-18 neighborhood deprivation, neighborhood crime, and neighborhood social cohesion (all ORs >1.11). Maternal psychotic symptoms were associated at p < 0.05 only with residential mobility and family poverty (all OR's >1.18). Family psychiatric history was associated at p < 0.05 with the cumulative environmental risk scale, age-12 and age-18 neighborhood deprivation, neighborhood disorder, residential mobility, and family poverty (all OR's >1.15). Finally, latent genetic risk was associated at p < 0.05 with the cumulative environmental risk scale, age-5 and age-18 neighborhood deprivation, neighborhood crime, residential mobility, and family poverty (all ORs >1.12).
Table 2. Association of genetic risk indices with socioenvironmental risk factors for schizophrenia
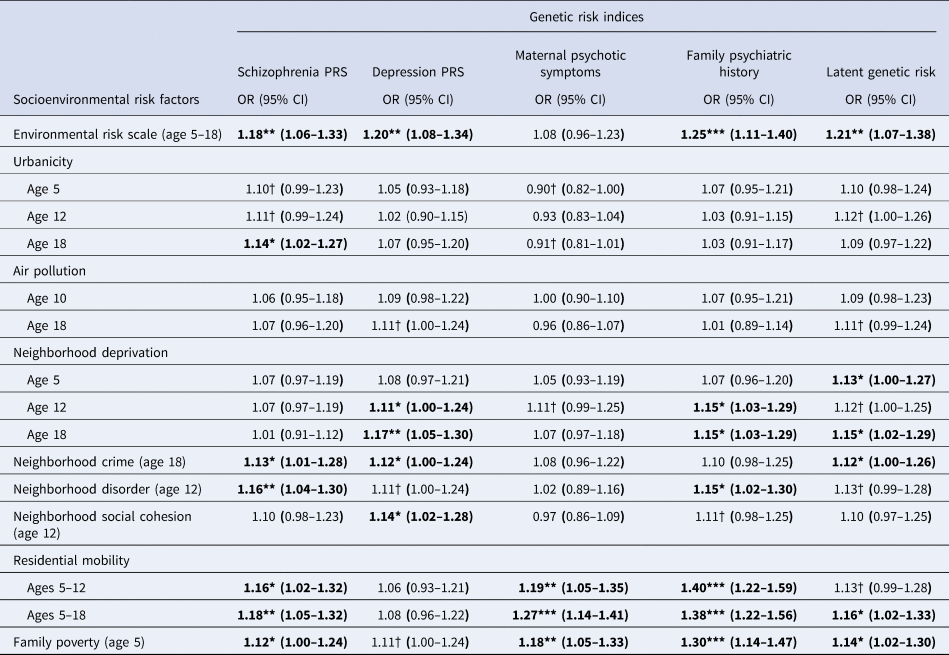
Note: CI, confidence intervals; OR, odds ratio; PRS, polygenic risk score; † p < 0.1; * p < 0.05; ** p < 0.01; *** p < 0.001. Bold font indicates p < 0.05. All analyses control for the non-independence of twin observations and include only one twin per MZ twin pair. Analyses were conducted following multiple imputations via chained equations for genotyped, European ancestry participants (N = 1999).
Are associations between socioenvironmental risks and adolescent psychotic experiences attenuated by covariate adjustment for genetic risk?
Associations between socioenvironmental risks and adolescent psychotic experiences are reported in Table 3 and online Supplementary Table 1. Children exposed to the highest levels of each risk factor had greater odds for psychotic experiences compared to children with the lowest exposure to these risks (all unadjusted ORs = 1.43–2.98; all ps < 0.05). Effect sizes were slightly reduced following covariate adjustment for schizophrenia PRS (2–9% reduction), depression PRS (2–12% reduction) and maternal psychotic symptoms (0–12% reduction), and were often reduced more substantially following covariate adjustment for family history (0–45% reduction) and latent genetic risk (8–29% reduction). Effect sizes were reduced by 15% (urbanicity) to 75% (residential mobility) after simultaneous adjustment for all genetic risk indices together. Nevertheless, associations mostly remained significant.
Table 3. Association of socioenvironmental risk factors with adolescent psychotic experiences
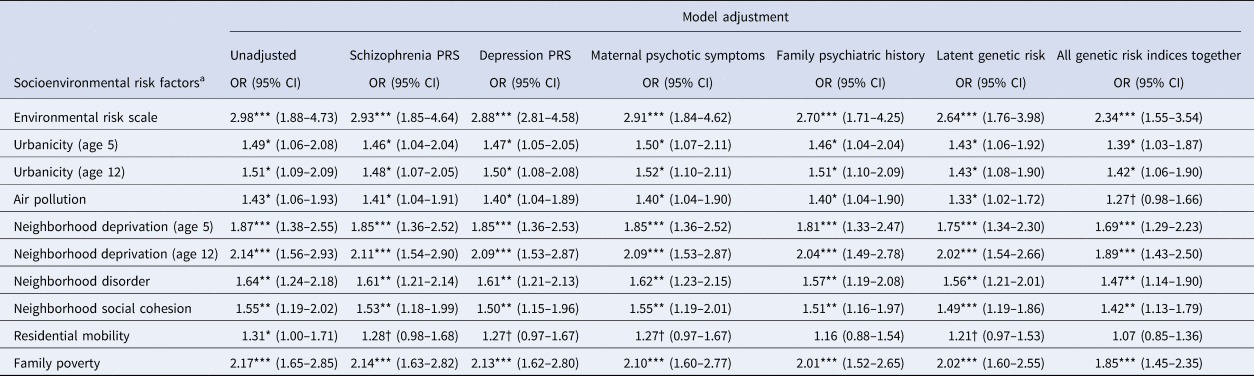
Note: CI, confidence intervals; OR, odds ratio from ordinal logistic regression; †p < 0.1; *p < 0.05; **p < 0.01; ***p < 0.001.
a Odds ratios are for the top v. bottom level of each socioenvironmental risk factor (association across all levels are shown in online Supplementary Table 1). Aside from the unadjusted model, all models control for sex in addition to the specified genetic risk measure. Missing values were multiply imputed using chained equations for genotyped European ancestry participants (N = 1999). All analyses control for the non-independence of twin observations.
Complete case analyses
Complete case analyses revealed similar point estimates (online Supplementary Tables 2 and 3).
Discussion
In a cohort of British youth followed from birth to age 18, participants at higher genetic risk were exposed to more socioenvironmental risk factors for schizophrenia during upbringing. Associations in terms of elevated odds were apparent for almost all risk factors examined. Beyond urbanicity, our study incorporated detailed neighborhood- and family-level risks measured from multiple sources. Our sample also captures the full range of socioeconomic and neighborhood conditions found in the UK, and we were thus able to contrast high- and low-risk groups. In doing so, we found that youth with higher genetic risk tended to grow up in more deprived neighborhoods with higher crime and disorder, and within poorer families who moved house more frequently. In fact, gene-environment correlations were more consistent for neighborhood- and family-level risks compared to the area-level risks. This could suggest that gene-environment correlations at the area-level comprise more pronounced gene-environment correlations at the neighborhood- and family-level. It could also be that larger sample sizes than ours are required to powerfully detect gene-environment correlations at the area-level. Therefore, future research into geosocial patterning of genetic risk requires both precise measures of the environment and representative samples that include high-risk groups.
There was tentative evidence that gene-environment correlations increased across development. For instance, schizophrenia PRS was associated with urbanicity at age 18, but not at ages 5 or 12; and depression PRS and family history were associated with deprivation at ages 12 and 18, but not at age 5. Our findings are consistent with those from a previous report in which schizophrenia PRS was associated with urbanicity at age 15 but not urbanicity at birth (Paksarian et al., Reference Paksarian, Trabjerg, Merikangas, Mors, Børglum, Hougaard and Agerbo2018). These findings provide support for a process of intra-generational drift, in which gene-environment correlations increase over time due to selection into different geographical and social conditions. A process of intra-generational drift is also supported by our novel finding that genetic risk is associated with more residential mobility during upbringing. Continued follow-up of the E-Risk cohort will allow us to test for downward drift further into adulthood. However, effect size differences across time-points were small and should be interpreted with caution. Furthermore, given that several risk factors were measured early in development, our findings cannot entirely be explained by intra-generational drift. Instead, our findings also support an inter-generational process in which families at higher genetic risk drift downward in social mobility across generations into more disadvantaged environments. That is, participants who inherited higher genetic risk also tended to be born and raised in riskier environments.
Following covariate adjustment for the genetic risk indices, effect sizes were often attenuated more substantially by family history and latent genetic risk v. PRS. This likely reflects, in part, that family- and twin-based measures capture genetic risk more comprehensively at present compared to PRS. This could also be because family history captures unmeasured environmental risks shared between family members which contributed to the expression of mental illness in the family. Nevertheless, the association between socioenvironmental risk factors and psychotic experiences existed above and beyond five genetic risk indices in our study. Though residual confounding is inevitable, our findings lend support for an important role of socioenvironmental conditions during upbringing in early expressions of psychosis.
Limitations
We acknowledge several limitations. First, PRS for schizophrenia currently capture ~30% of the SNP-based heritability for schizophrenia (Ripke et al., Reference Ripke, Neale, Corvin, Walters, Farh, Holmans and Huang2014), despite behavioral genetics studies demonstrating that >70% of schizophrenia variance is due to genetic factors (Lichtenstein et al., Reference Lichtenstein, Yip, Björk, Pawitan, Cannon, Sullivan and Hultman2009). Indeed, schizophrenia PRS was only weakly associated with adolescent psychotic experiences in our study. The ability of PRS to capture genetic liability and predict phenotypic variance is expected to increase as the size of GWAS samples increases (Visscher et al., Reference Visscher, Wray, Zhang, Sklar, McCarthy, Brown and Yang2017). Second, family psychiatric history (and by design, maternal psychotic symptoms) was biased towards maternal family history (though mothers also reported on the twins' father's psychiatric history). In large cohort studies with finite resources it is common to focus on maternal reports, partly because mothers typically retain main custody in instances of parental separation (Braver, Ellman, Votruba, & Fabricius, Reference Braver, Ellman, Votruba and Fabricius2011). Third, it was necessary to restrict all analyses (MICE and complete case) to European descent participants because PRS are currently suitable only within ancestries matched to the original GWAS (Martin et al., Reference Martin, Gignoux, Walters, Wojcik, Neale, Gravel and Kenny2017; Vassos et al., Reference Vassos, Di Forti, Coleman, Iyegbe, Prata, Euesden and Patel2017). Fourth, adolescent psychotic experiences were self-reported and may have included false positives. In addition, the measure covered only positive psychotic symptoms. Negative and disorganized symptoms are potentially more clinically-pertinent (Ho, Nopoulos, Flaum, Arndt, & Andreasen, Reference Ho, Nopoulos, Flaum, Arndt and Andreasen1998), but are also, unfortunately, harder to assess (Stahl & Buckley, Reference Stahl and Buckley2007). Finally, the E-Risk sample comprises twins, who could differ from singletons in terms of both measured and unmeasured factors. However, E-Risk families are representative of the UK population in terms of urbanicity (Office for National Statistics, 2013) and socioeconomic conditions (CACI Information Services, 2006; Department for Communities and Local Government, 2015). The prevalence of psychotic experiences in our sample was also comparable to those from general population studies of non-twins (Spauwen et al., Reference Spauwen, Krabbendam, Lieb, Wittchen and van Os2004; Yoshizumi et al., Reference Yoshizumi, Murase, Honjo, Kaneko and Murakami2004; Yung et al., Reference Yung, Nelson, Baker, Buckby, Baksheev and Cosgrave2009).
Conclusion
Urbanicity, deprivation, neighborhood crime and disorder, residential mobility and poverty have been consistently associated with both clinical and subclinical expressions of psychosis. Our findings have implications for interpreting the causality of these associations. It is very unlikely that associations are entirely confounded by genetics. Indeed, associations between socioenvironmental risk factors and adolescent psychotic experiences existed above and beyond five indices of genetic risk in our study. However, our findings add to growing evidence that a degree of genetic confounding exists. Future investigations into the environment and psychosis should continue to consider gene-environment correlation as a non-causal contributor to associations. Understanding the processes leading to intra- and inter-generational drift could also open up valuable new avenues for improving psychiatric interventions and reducing socioenvironmental inequalities.
Supplementary material
The supplementary material for this article can be found at https://doi.org/10.1017/S0033291720003347.
Acknowledgements
We are grateful to the study mothers and fathers, the twins, and the twins' teachers for their participation. Our thanks to members of the E-Risk team for their dedication, hard work, and insights and, the Nuffield Foundation, the Avielle Foundation, and to CACI Inc. We would like to acknowledge the assistance of the Duke Molecular Physiology Institute Molecular Genomics core, plus Dr Sean Beevers, Dr Nutthida Kitwiroon and Dr Andrew Beddows for the generation of data for this manuscript. The E-Risk Study is funded by the UK Medical Research Council [G1002190]. Additional support was provided by the National Institute of Child Health and Human Development [HD077482]; the British Academy [SQ140024]; and the Jacobs Foundation. This work used a high-performance computing facility partially supported by grant 2016-IDG-1013 (‘HARDAC + : Reproducible HPC for Next-generation Genomics’) from the North Carolina Biotechnology Center. The pollution data were funded by a joint grant from the Natural Environment Research Council, the UK Medical Research Council, and the Chief Scientist Office [NE/P010687/1] and also supported by the King's Together Multi and Interdisciplinary Research Scheme (Wellcome Trust Institutional Strategic Support Fund; [204823/Z/16/Z]). JBN received a Postdoctoral Research Fellowship [ES/S011196/1] from the Economic and Social Research Council (ESRC), an Early Career Bridging and Career Development Grant [IS-BRC-1215-20018] from the NIHR-Maudsley Biomedical Research Centre, and a Sir Henry Wellcome Research Fellowship from the Wellcome Trust [218632/Z/19/Z]. This paper represents independent research part funded by the National Institute for Health Research (NIHR) Biomedical Research Centre at South London and Maudsley NHS Foundation Trust and King's College London. LA is the Mental Health Leadership Fellow for the UK ESRC. HLF was supported by a British Academy Mid-Career Fellowship [MD\170005] and by the ESRC Centre for Society and Mental Health at King's College London [ES/S012567/1]. DWB and CLO were supported by fellowships from Jacobs Foundation. CLO is supported by the Canadian Institute for Advanced Research. DWB is supported by a Russell Sage Foundation award. TM is a British Academy Postdoctoral Fellow. The views expressed are those of the authors and not necessarily those of the NHS, the NIHR, the Department of Health and Social Care, the ESRC, the Wellcome Trust or King's College London.
Conflict of interest
None.
Ethical standards
The authors assert that all procedures contributing to this work comply with the ethical standards of the relevant national and institutional committees on human experimentation and with the Helsinki Declaration of 1975, as revised in 2008.