Introduction
The burden of non-communicable diseases (NCDs) such as cardiovascular diseases (CVDs), chronic obstructive lung diseases, cancer and diabetes affects health and the socio-economic development of countries (Mendis, Reference Mendis2014; Bommer et al., Reference Bommer, Sagalova, Heesemann, Manne-Goehler, Atun, Barnighausen, Davies and Vollmer2018; Niessen et al., Reference Niessen, Mohan, Akuoku, Mirelman, Ahmed, Koehlmoos, Trujillo, Khan and Peters2018). High systolic blood pressure, fasting plasma glucose and body mass index are the leading risk factors of disability-adjusted life years globally (GBD 2017 Risk Factor Collaborators, 2018). These metabolic risk factors are known to increase CVD which is the main contributor to NCD-related premature death (World Health Organization, 2018). However, the causes of CVDs are largely amenable to change through behavioural modification and risk factors prevention.
A comprehensive situational analysis of the Malaysian health system undertaken in 2016–2018 revealed that the NCD burden is very high and is rapidly increasing with almost two-thirds of Malaysian adult population having at least one of the three NCDs – Type 2 diabetes mellitus (T2DM), hypertension or hypercholesterolaemia (Institute for Public Health, 2015; Ministry of Health Malaysia and Harvard University TH Chan School of Public Health, 2016). This analysis, which used a ‘care cascade’ framework, revealed major gaps in awareness of risk, timely screening, definitive diagnosis, engagement and treatment for T2DM, hypertension and hypercholesterolaemia (Institute for Public Health, 2015). The shortcomings in NCD care in primary healthcare clinics are mostly due to lack of continuity and coordination of care, poor organisational management of healthcare providers, long waiting times and limited operational hours, low screening and counselling activities, limited awareness of the need for screening services and preventive care and suboptimal therapeutic prescribing for the treatment of T2DM and hypertension (Ministry of Health Malaysia and Harvard University TH Chan School of Public Health, 2016). These findings reflect the need to address the care gaps in the health system to effectively prevent and treat CVDs.
In order to improve the performance of the Malaysian health system in managing NCDs, an integrated care model was designed to enhance existing healthcare services by incorporating public health, primary healthcare and social support as part of a ‘network’ linked to appropriate secondary and tertiary hospital services. This new framework, called ‘Enhanced Primary Healthcare’ (EnPHC), uses primary healthcare as an agent of change to deliver efficient and effective service and in turn contributes to the entire care continuum. EnPHC framework includes multiple interventions: (i) establishment of a population database with population enrolment and risk profiling, (ii) community-based intervention programmes, (iii) branding and social marketing, (iv) development of integrated multidisciplinary care pathways based on international evidence with local adaptation, (v) continuous improvement of care delivery, (vi) improved organisational practices and (vii) integrated care networks (Institute for Public Health, 2019b). All interventions are necessary for the cohesive and successful implementation of EnPHC but adaptations to the intervention are allowed.
EnPHC aims to improve management of T2DM, hypertension and hypercholesterolaemia across the cascade of care by increasing early detection and management of the target NCDs, surveillance and monitoring of diagnosed patients with improved continuity and coordination and by providing enhanced person-centred quality of care. The EnPHC framework is implemented as a demonstration project to inform decisions on further expansion to the rest of the country (Institute for Public Health, 2019b). The implementation is carried out by the Family Health Development Division of Ministry of Health Malaysia, and the evaluation is a collaborative effort between Institute for Clinical Research (ICR), Institute for Health Management (IHM), Institute for Public Health (IPH), Institute for Health Systems Research (IHSR) and Institute for Health Behavioural Research (IHBR), working with researchers at Harvard University.
The conceptual framework to guide the implementation and evaluation is discussed below. An impact evaluation that spans from the community to the patients and providers of primary healthcare is carried out (Institute for Public Health, 2019a). To further strengthen the findings from the impact evaluation studies, a process evaluation study is carried out to evaluate the implementation process in terms of fidelity and quality of implementation (Institute for Health Systems Research, 2019).
To evaluate the interventions, complementary data from multiple sources are used to measure outcomes. Evaluation performed at primary healthcare clinics aims to measure health service and patient outcomes. Therefore, the primary objective is to evaluate the impact of EnPHC interventions on processes of care and intermediate clinical outcomes among T2DM and hypertension patients, and the secondary objective is to evaluate the impact of the EnPHC interventions on patients’ experience and providers’ job satisfaction. The aim of this paper is to describe the sequential steps taken to evaluate the EnPHC interventions at primary healthcare clinics (EnPHC-EVA: Facility) and the methods used.
EnPHC interventions
EnPHC interventions started from July 2017 and took three months for all clinics to fully implement. The EnPHC interventions represent activities in the community, facilities and networks targeting the three NCDs and risk factors (Institute for Public Health, 2019b). The EnPHC intervention is aimed at two levels which are the community and the facility level. The community level targets Malaysian adults aged 18 and above, who live within the operational area of the primary healthcare clinics. The facility level targets Malaysian adults aged 30 and above, utilising the primary healthcare clinics. The seven components of the new EnPHC framework (the intervention) are listed in the introduction and the comparison of the current public sector primary healthcare model in Malaysia, with the EnPHC framework shown in Table 1 (Institute for Public Health, 2019b).
Table 1. Components of the EnPHC intervention and comparison of the current public sector primary healthcare model in Malaysia with EnPHC model

EnPHC, enhanced primary healthcare.
Note: Adapted from the Malaysia Health Systems Research Study: Implementation Plan 2016 and Enhanced Primary Healthcare Lab 2017.
Conceptual framework
We used a health systems approach when conceptualising multiple interventions along the care cascade – as improvements in outcomes will require addressing every step in the care cascade. Care cascades have been used to examine the effectiveness and efficiency of management of infectious diseases in health systems, for example, for human immunodeficiency virus (HIV) (Gardner et al., 2011), Hepatitis C (Yehia et al., Reference Dimick and Ryan2014), tuberculosis (Kim et al., Reference Kim, Keshavjee and Atun2019), as well as for NCDs such as diabetes (Prenissl et al., Reference Prenissl, Jaacks, Mohan, Manne-Goehler, Davies, Awasthi, Bischops, Atun, Barnighausen, Vollmer and Geldsetzer2019a) and hypertension (Prenissl et al., Reference Prenissl, Manne-Goehler, Jaacks, Prabhakaran, Awasthi, Bischops, Atun, Barnighausen, Davies, Vollmer and Geldsetzer2019b) and examine health system performance using these conditions as tracers (Manne-Goehler et al., Reference Manne-Goehler, Atun, Stokes, Goehler, Houinato, Houehanou, Hambou, Mbenza, Sobngwi, Balde, Mwangi, Gathecha, Ngugi, Wesseh, Damasceno, Lunet, Bovet, Labadarios, Zuma, Mayige, Kagaruki, Ramaiya, Agoudavi, Guwatudde, Bahendeka, Mutungi, Geldsetzer, Levitt, Salomon, Yudkin, Vollmer and Barnighausen2016; Manne-Goehler et al., Reference Manne-Goehler, Geldsetzer, Agoudavi, Andall-Brereton, Aryal, Bicaba, Bovet, Brian, Dorobantu, Gathecha, Singh Gurung, Guwatudde, Msaidie, Houehanou, Houinato, Jorgensen, Kagaruki, Karki, Labadarios, Martins, Mayige, McClure, Mwalim, Mwangi, Norov, Quesnel-Crooks, Silver, Sturua, Tsabedze, Wesseh, Stokes, Marcus, Ebert, Davies, Vollmer, Atun, Barnighausen and Jaacks2019). In essence, the Malaysian care cascades for T2DM and hypertension refer to four main steps, which include proportions of patients who are (i) screened, (ii) aware of their disease, (iii) treated or advised and (iv) have their disease under control (Prenissl et al., Reference Prenissl, Jaacks, Mohan, Manne-Goehler, Davies, Awasthi, Bischops, Atun, Barnighausen, Vollmer and Geldsetzer2019a; Reference Prenissl, Manne-Goehler, Jaacks, Prabhakaran, Awasthi, Bischops, Atun, Barnighausen, Davies, Vollmer and Geldsetzer2019b). Figure 1 shows the Malaysian T2DM care cascade which revealed that there is under-detection and under-treatment (Atun et al., Reference Atun, Berman, Yap, Kusuma and Lim2016).

Figure 1. Care cascade for T2DM in Malaysia (Atun et al., Reference Atun, Berman, Yap, Kusuma and Lim2016).
Our theory of change is that improvements in every step of the care cascade are necessary if outcomes are to improve, and the use of evidence-based integrated care pathways (ICPs), supported by the use of clinical audits (Grimshaw et al., Reference Norris, Engelgau and Narayan2001) and ongoing learning (Ivers et al., 2012) that optimised efficiency and effectiveness of services provided along the care cascade, would help improve health outcomes, user experience and user satisfaction (Baxter et al., 2018).
Methods
Study design and study sites
The EnPHC-EVA: Facility study is a quasi-experimental controlled study which involves 20 intervention and 20 control clinics. We drew on a methodology developed by Imai, King and Nall (Imai et al., Reference Imai, King and Nall2009). After considering regional representativeness, budget and capacity to implement EnPHC interventions, 20 matched pairs from two states (Johor and Selangor) were selected to be the study sites. The clinics were matched based on the criteria listed in Table 2 (Family Health Development Division, 2018). In addition, ArcGIS software (ESRI, 2011) was used to ensure that the matched pairs were in different districts to minimise patients’ overlap. The matched pairs were then randomly allocated to the ‘intervention’ or ‘control’ arm of the study by ‘flipping a coin’.
Table 2. Clinic inclusion and matching criteria

There are nine and 11 matched pairs, in Selangor and Johor states, respectively. Figure 2 shows the matching pairs in both Johor and Selangor states, where intervention clinics were identified with crosses.

Figure 2. Selected clinic pairs in Johor and Selangor.
Study population
The criteria for selecting the sample for each questionnaire are listed in Table 3. The different questionnaires used are described under the subheading Data Collection Tools. We include patients of 30 years and above to match with EnPHC interventions. Pregnant women are not included as they would have different clinical management plans.
Table 3. Inclusion and exclusion criteria for retrospective chart review, patient exit survey and provider survey

Outcome measures
Outcome measures used in this study are captured using different data collection tools and are summarised in Table 4. It is anticipated that improvements of blood pressure, lipid levels and glycaemic control will be observable after at least one year as changes have been detected after 12.5 months of implementation in similar evaluation studies (Norris et al., Reference Grimshaw, Shirran, Thomas, Mowatt, Fraser, Bero, Grilli, Harvey, Oxman and O’Brien2001). Hence, for post-intervention, information on processes of care (investigations, counselling and prescribing patterns) will be gathered via RCRs 15 months after implementation.
Table 4. Outcome measures of the EnPHC-EVA: facility study
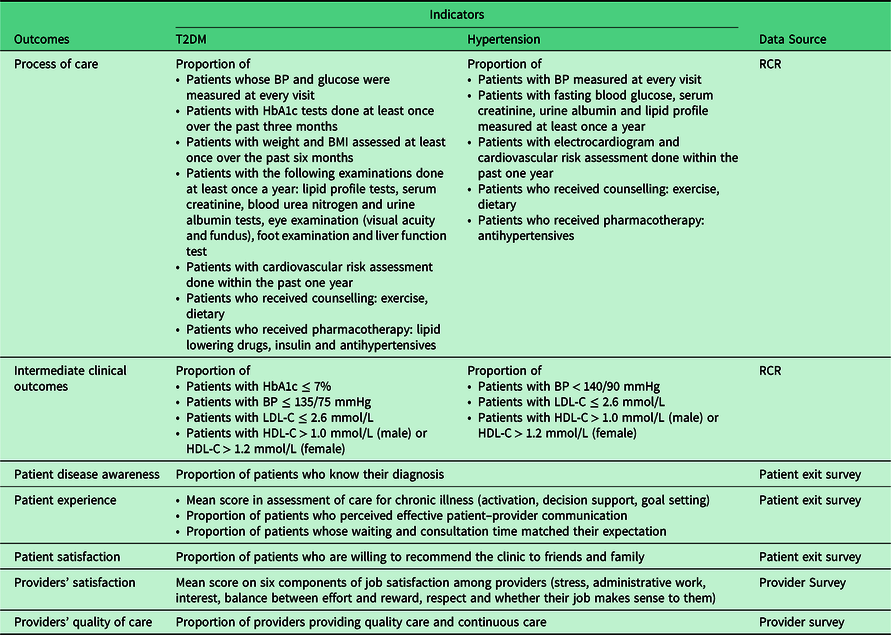
BP: Blood pressure; HbA1c: Haemoglobin A1c; BMI: Body mass index; LDL-C: Low-density Lipoprotein Cholesterol; HDL-C: High-density Lipoprotein Cholesterol; TG: Triglyceride.
Improved self-efficacy, improved health beliefs and a clear care pathway are expected to increase patients’ satisfaction with the healthcare system and improve utilisation (Panagioti et al., Reference Panagioti, Richardson, Small, Murray, Rogers, Kennedy, Newman and Bower2014). These outcomes will be captured via a patient exit survey. In addition, this study also includes a measure of potential unintended outcomes, such as the job satisfaction of the healthcare providers involved in implementing the interventions. Patient exit survey and provider survey will be carried out at 20 months post-intervention. In the long run, there are expected to be reductions in complications of T2DM, hypertension and hypercholesterolaemia and improvement in patients’ quality of life. However, this evaluation study was not designed to measure the changes in hypercholesterolaemia and the long-term outcomes.
Data collection tools and sources
This study gathers data from patient medical records, a self-administered questionnaire for healthcare providers and patient interviews. The data collection tools include a data extraction form for RCR, three questionnaires (patient exit, provider and facility questionnaires) and an intervention checklist. All data collection tools are available in Appendices A–E. All the data collection tools underwent a pre-test for comprehensibility.
Data extraction form for retrospective chart review
An electronic data extraction form was designed to collect information on the process of care and intermediate clinical outcomes, as described in Table 4. The source of data extraction is T2DM and hypertension patients’ medical records that were available as paper-based documents, within the legacy tele-primary care (TPC) computer system or any standalone electronic medical system.
Patient exit questionnaire
The research committee comprising intervention implementers, family medicine specialists (FMS), public health specialists and study researchers had reached a consensus to adapt a subset of items from the Quality and Cost of Primary Care (QUALICO-PC): Patient Experience Survey (Sivasampu et al., Reference Sivasampu, Mohamad Noh, Husin, Wong, Ismail and Harvard2016) and the Patient Assessment of Chronic Illness Care (PACIC) survey instruments (Glasgow et al., Reference Højsgaard, Yan and Halekoh2005) for the patient exit questionnaire. This decision was reached after taking into account the length of the questionnaire and health literacy level of the study population (Azreena et al., 2016) in order to reduce respondent fatigue and increase completion rates. The questionnaire contained 43 items and was completed through face-to-face interviews, which measure socio-demographic characteristics, patient experience and satisfaction, disease awareness and self-management support.
Provider questionnaire
Similarly, the research committee adapted the questions from QUALICO-PC: General Practitioner questionnaire (Sivasampu et al., Reference Sivasampu, Mohamad Noh, Husin, Wong, Ismail and Harvard2016) for the provider questionnaire. The main aim of the provider survey is to gather information on job satisfaction. The decision to measure job satisfaction is based on the conceptual framework that was described in the earlier section. Six components of job satisfaction are measured, which include stress, administrative work, interest, balance between effort and reward, respect and whether their job makes sense to them. It also measures the use of latest clinical practice guidelines, continuity and coordination of care and workload. As such, a self-administered provider questionnaire consisting of 28 questions, which focused on the structural aspects of primary care, workload, demographic and the provider’s satisfaction, was developed.
Facility questionnaire
The facility questionnaire was developed to measure information on clinic resources, including staff, infrastructure and equipment, as well as services provided, which can determine the success of the intervention.
Translation of English version questionnaires into Malay language
The translation of the English version of the patient exit questionnaire, provider questionnaire and facility questionnaire into Malay language was performed using forward and backward procedures. Two study collaborators who were fluent in both English and Malay and familiar with the primary care practice prepared the Malay questionnaire. The Malay questionnaire was then back-translated into the English version independently by another two translators. The researchers compared both versions to ensure that no change in the context occurred during translation and discrepancies were resolved prior to pre-test of the surveys. Subsequently, modifications of the Malay version questionnaire were made in accordance to findings of the pre-test.
Intervention checklist
As the EnPHC framework is a complex intervention with multiple elements, it is important to measure the details of its implementation at each clinic in order to quantify the percentage of the intended interventions that actually took place, the level of contamination within control clinics and also to determine the correlation between the degree of implementation and the observed outcomes (O’Donnell, Reference O’Donnell2008). This is important as contamination is expected as some of the EnPHC intervention components are built upon existing primary care programmes (Safurah et al., 2018) (Refer to Table 1).
The 18-item intervention checklist was checked for content validity through a consensus meeting with ten content experts who were EnPHC intervention implementers. The implementers weighed the items based on the expected impact of each item on the study outcomes. The final score of the intervention checklist will be reported as a percentage and used as a covariate in the analysis.
Study procedures
Preparation
A pre-test was carried out in two primary healthcare clinics that were not in the study sample to confirm the strengths and weaknesses of the developed questionnaires. Patients of differing ages, genders, education levels and languages were interviewed and comments on the interview and questionnaire were recorded. Similar steps were applied to the provider questionnaire. Following that, all necessary changes and improvements to the questionnaire were made. Also, the study team did a trial run of the processes for data collection such as tracing patient medical records and interviewing patients and providers. The duration taken for each of these processes was also recorded. This allowed for further refinement of the data collection process and estimation of missing medical records, which can be accounted for in the sample size calculation.
This study recruits fieldworkers who are either medical or pharmacy graduates as data collection requires fieldworkers to be familiar with extracting data from medical records. Fieldworker training included the conduct of quantitative data collection such as manual data extraction from paper-based records, patient recruitment procedures and interview techniques.
Data collection period
The availability of documents and number of fieldworkers were taken into consideration when planning the data collection processes. Data for RCR will be collected for 31 time points (i.e. in months): (i) from November 2016 to June 2017 (pre-intervention) and (ii) from August 2017 to June 2019 (post-intervention). To keep the workload manageable and prevent exhaustion among the study team members, data collection for the 31 time points was divided into four phases, one pre-intervention phase and three post-intervention phases. Data collection phases were also decided based on the feasibility of recruiting for patient interviews during the fasting month. The data collection period for each phase is indicated in Figure 3. Each team is led by one team leader and four fieldworkers.

Figure 3. Study timeline and data collection period for EnPHC-EVA: Facility.
Quality control
Validation rules were embedded within the mobile applications to limit data entry error. The information captured will be uploaded to a central database with continuous monitoring by a data management team that performs quality checks and addresses discrepancies. The final captured data will undergo a systematic data cleaning process preceding data analysis. There is a full audit trail for data management which covers data entry and any amendments to the data. This measure will ensure that all the changes are monitored and there will be no accidental deletion.
Target sample size and sampling
Retrospective chart review
Data analysis for RCR will use two methods – DiD analysis and interrupted time series (ITS) analysis. For DiD analysis, the sample size was calculated using clustersampsi in STATA version 14.2 (StataCorp, 2015).
First for T2DM, 1800 patient visits (900 in each arm) were needed to have 80% power to detect a relative change of 28% in the proportion of the patients receiving an annual HbA1c test from the baseline proportion of 52.5% (Mastura et al., Reference Mastura, Chew, Lee, Cheong, Sazlina, Jamaiyah, Alwi, Taher and Zaiton2011). This sample size was calculated while taking into account the estimated cluster effect [intracluster correlation (ICC) = 0.091] (Singh et al., 2015; Solorio et al., 2015). The minimum sample size was rounded up to 2000 or a cluster size of 50 patient visits per clinic. Therefore, the final target sample size for T2DM was 84 patient visits per clinic, after adjusting for an estimated 40% untraceable records.
Second, for hypertension, 1760 patient visits (880 in each arm) were needed to have 80% power to detect a 28% relative change in the proportion of hypertension patients with at least one lipid profile from the baseline proportion of 46% (Wong et al., 2015). This calculation took into account the estimated clustering of this outcome at the clinic level (ICC = 0.076) (Singh et al., 2015). After adjusting for 40% potentially untraceable records, the sample size required for hypertension patient visits was also 84 patient visits per clinic.
For ITS analysis, a minimum of 100 patient visits per arm at each time point is needed (Wagner et al., 2002). A minimum of eight time points was needed for both before and after intervention. In this study, eight time points pre-intervention and nine time points post 15-month intervention were chosen to evaluate the trend and level change over time. For each clinic, the estimated sample size required is ten patient visits for each disease. The final sample size after accounting for 40% untraceable records was 17 patient visits per clinic for each disease.
Systematic random sampling is used to sample the medical records by creating a sampling frame from the patients’ register either in a paper or in an electronic format. In facilities where neither of these is available, the lists are created from patient appointment books.
Patient exit survey
For the patient exit survey, we aim to detect a 15% change in the proportion of patients who would recommend the clinic to family or friends, while accounting for an estimated ICC from a previous study of 0.116 (Sivasampu et al., Reference Sivasampu, Mohamad Noh, Husin, Wong, Ismail and Harvard2016). With a statistical power of 80%, we would require at least 920 patient surveys (460 in each arm). Hence, the data collection teams aim to recruit 23 patients per clinic. Patients are sampled consecutively using convenience sampling until the required numbers are achieved.
Provider survey
All clinic staff who meet study inclusion criteria will be interviewed. All visiting staff who visit the clinic on a regular schedule are also included in the survey. As EnPHC interventions would involve a change in clinic flow and staff allocation, it is necessary to measure the change in numbers for all categories of staff.
Data analysis
The data will be analysed using STATA version 14 and R version 3.3.2 (StataCorp, 2015; RStudio Team, 2016; R Core Team, 2017). Descriptive data will be expressed as means or proportions with their corresponding 95% confidence intervals or medians with interquartile ranges. Statistical significance will be determined with a 2-sided P-value with P < 0.05. A complete case analysis will be used.
An interim analysis will be conducted at the end of Phase 2. A research management team is tasked to monitor the study at the end of Phase 2 and Phase 3b. The research management team will review and comment on the interim and final analysis.
Difference-in-differences
DiD analysis will be used to analyse all outcome measures. DiD analysis measures the average effect of the interventions, taking into account two differences: first is the difference between the intervention and control arm and second is the difference between pre- and post-intervention (Gertler et al., Reference Gertler, Sebastian, Rawlings, Premand and Vermeersch2011; Dimick et al., Reference Mendis2014). As this is a large-scale field experiment, the difference at baseline is often not negligible and must be accounted for when measuring the intervention effect.
A multivariable model that controls for patient- and clinic-level covariates will be used. Details on the key covariates for each objective can be found in Appendix F. For RCR, patient visits from November 2016 to June 2017 will be grouped to estimate outcome levels in the pre-intervention phase and those from October 2018 until June 2019 will be grouped to estimate outcomes after 15 months of intervention.
Generalised estimating equations will be used to estimate the parameters for both linear and non-linear outcomes while adjusting for clustering at the clinic level. Thus, cluster robust standard errors will be reported. In addition, the key assumptions for DiD analysis which are ‘common shocks’ and ‘parallel trends’ will be checked where trend data are available.
The ‘geeglm’ function from the ‘geepack’ package in R will be used to perform the analysis (Yan, Reference Yan2002; Yan and Fine, Reference Yan and Fine2004; Højsgaard et al., Reference Højsgaard, Yan and Halekoh2005). For RCR outcome measures that will be reported as proportions, the DiD estimates will be reported as odds ratios with 95% confidence intervals. For provider survey and patient exit survey outcome measures, the DiD estimates will be reported as changes in means with 95% confidence intervals.
Interrupted time series
ITS analysis will examine changes in trends and levels of the outcome measures using monthly data. The observation period started in November 2016 while the interventions were implemented from July 2017. Data from August 2017 to September 2018 will be used to plot a segment to show trend or level change during intervention whereas data from October 2018 to June 2019 will be used to measure the change after 15 months of intervention. ITS analysis can provide an insight into the expected trends of changes, especially for measures that do not show immediate effects. This can show the potential benefits of the interventions, even though the differences in levels are not significant in the early stage of the intervention.
Equivalence of baseline trends in intervention and control groups will be checked. If equivalence cannot be ensured, we will not infer causality between intervention and outcome (Lopez Bernal et al., 2018). We assume an impact model with both level and trend change for all outcomes. The final model will be estimated using generalised least squares. All covariates are included based on clinical relevance, hence this will be retained in the model regardless of statistical significance. The ‘nlme’ package in R will be used for segmented regression (Pinheiro et al., 2013). Results will be presented in ITS charts with the corresponding statistical results for trend and level changes post-intervention.
Discussion
The EnPHC intervention and evaluation studies aim to apply a set of multifaceted, person-centred interventions that are believed to be effective on the overall management and prevention of NCDs. This is the first study of its kind in the Malaysian context where a public health intervention involving a community outreach component, together with changes at the health system, facility, and patient level, is empirically evaluated in a ‘real-world’ setting. The community outreach component is evaluated by a separate study team and will not be discussed here. The EnPHC intervention was designed to provide organizational-level solutions such as integrated multidisciplinary care, continuous improvement of care delivery and improved organisational practices. It is aimed that, with all intervention components coming together, we will observe from the facility and health providers’ perspective increases in screening and detection rates for NCDs including T2DM, hypertension and hypercholesterolaemia and improvements in processes of care and adherence to T2DM, hypertension and hypercholesterolaemia practice guidelines. Also, it is known that workload in public primary healthcare clinics in Malaysia is so high that little time is left for meaningful interactions between patients and providers (Risso-Gill et al., Reference Risso-Gill, Balabanova, Majid, Ng, Yusoff, Mustapha, Kuhlbrandt, Nieuwlaat, Schwalm, McCready, Teo, Yusuf and McKee2015). Therefore, encouraging proactive involvement of other health professionals such as allied health allows more time for providers to strengthen patient–provider relationships and improve knowledge transfer to patients. With these multifaceted approaches, it is hypothesised that the EnPHC intervention will enhance adherence to clinic appointments and treatment and improve self-management practices and patient-reported experience at the patient level. This will subsequently improve disease control and can be measured through intermediate clinical outcomes such as HbA1c, blood pressure and lipid levels.
Hypercholesterolaemia was among the NCDs that were shown to be under-diagnosed and suboptimally managed in Phase 1 of the Malaysian Health Systems Research (MHSR) report (Ministry of Health Malaysia and Harvard University TH Chan School of Public Health, 2016). EnPHC-EVA: Facility study was designed to include a sub-sample of hypercholesterolaemia patients but this plan had to be dropped because there was no means to identify a list of patients diagnosed with hypercholesterolaemia. Patient visits for T2DM and hypertension, on the other hand, had defined appointment registers for each clinic work day. However, T2DM and hypertension patients with hypercholesterolaemia as comorbidity were captured.
Process evaluation of EnPHC interventions in the primary healthcare clinic is conducted by a separate study team to improve execution of the interventions. This, together with an impact evaluation done at both the community and facility level, will complement each other and allow for triangulation of findings. Only then, the intervention can be refined and replicated for further nationwide scale up.
The strengths of this study include the presence of a control group, random intervention allocation and repeated data points which maintained internal validity. Furthermore, the clusters were matched to minimise bias that can exist from differences in clinic composition. An intervention checklist was also incorporated to adjust for the degree of intervention implementation within each clinic and explain potential contamination or incomplete implementation in the control and intervention clinics, respectively. In addition, the transparency in reporting this study by adhering to the Transparent Reporting of Evaluations with Nonrandomized Designs (TREND) statement is a form of knowledge sharing and contributes to rigorous evidence to guide future research and practice (Des Jarlais et al., 2004). Another strength of this study is that its implementation and evaluation teams were from separate institutions, the former is a planning and governing division for public health clinics while the latter is a research organisation. This maintained objectivity of the study and minimises the risk of publication and outcome-reporting bias. Lastly, this study also captures potential unintended effects on the healthcare staff, measured as change in health provider job satisfaction following the implementation of EnPHC through the provider survey.
There are several limitations in this study. First, it is not possible to distinguish the effects from each activity of the intervention as it is a complex intervention. Next, the findings of this study will only be generalisable to clinics with similar characteristics. Long-term outcomes such as micro- and macrovascular complications and behavioural changes could not be measured as the study duration is not sufficient to observe these changes. Missing data arising from untraceable records and incomplete documentation in medical records may introduce bias to the findings. This could be improved by integrated electronic information systems in the long run.
In summary, this study will provide evidence on the effectiveness of a complex intervention designed to reduce the number of undiagnosed T2DM and hypertension patients as well as improve the overall quality of care in this group of patients to delay overall disease progression.
Supplementary material
To view supplementary material for this article, please visit https://doi.org/10.1017/S1463423620000250
Acknowledgements
The authors would like to thank the Director General of Health Malaysia for permission to publish this article. The authors would also like to thank the EnPHC Project Management Team for their support and assistance. The authors’ appreciation goes to the Johor and Selangor state liaison officers who are involved in coordinating the clinics to ensure smooth data collection phase. The authors would also like to thank all the healthcare providers and patients from the 40 clinics for their participation in this study and ensuring a smooth data collection process. The authors would also wish to thank the team leaders and fieldworkers involved in data collection for this study.
Author contributions
S.S.1., Y.M.F.L., S.M.O., D.K., R.A. and DRD were responsible for the concept and design of the study. SS1 acquired the funding and supervised the study. X.R.T., S.H.A., W.J.W., M.H., D.K. and R.A. contributed to the coordination of the study. S.S.1., Y.M.F.L., S.M.O., X.R.T., S.H.A. and M.H. constructed the draft manuscript. Y.M.F.L., S.M.O., X.R.T., S.H.A., N.K., F.S.AJ., W.J.W., S.S.2., S.A. and S.H.L.P. made a substantial contribution to the acquisition of the data. All authors critically revised the manuscript. All authors read and approved the final manuscript.
Financial support
The funding of this study was supported by a grant from the Ministry of Health Malaysia (NMRR-17-267-34768) under the Malaysia Health System Research (MHSR) initiative. The publication of this article was sponsored by the Ministry of Health Malaysia. During the period of this study, Dr. Dennis Ross-Degnan was supported in part by the United States National Institute of Diabetes and Digestive and Kidney Diseases Award Number P30DK092924.
Conflicts of interest
None.
Ethical standards
Ethical approval for the EnPHC-EVA: Facility study was obtained from the Medical Research Ethics Committee (MREC) Malaysia (research registration number: NMRR-17-267-34768). Consent was waived for RCR by MREC as retrospective data were used and it was not possible to obtain consent from all the sampled patients. Informed written consent is taken from all the patients prior to the patient exit survey interview. Consent is implied for provider survey.