1. Introduction
From the United States to Europe and beyond, populist leaders are enjoying a resurgence propelled by widespread resentment toward globalization. The United Kingdom's decision to withdraw from the European Union is widely viewed as a rejection of integration. Marine Le Pen took National Front from the fringes of French politics to the forefront by condemning immigration and criticizing international institutions. Globalization was vehemently attacked by the left and right during the 2016 American presidential election. Populists blame globalization for causing underemployment, wage stagnation, growing inequality, and the disappearance of well-paid factory jobs.
Although globalization has important distributional effects, neither trade, immigration, nor offshoring explain the preponderance of affected workers’ plight. The existing scholarship highlights technology's link to many of the structural economic changes witnessed in advanced industrialized countries (Bekman et al., Reference Bekman, Bound and Machin1998; Acemoglu and Restrepo, Reference Acemoglu and Restrepo2018, Reference Acemoglu and Restrepo2020). Acemoglu and Restrepo (Reference Acemoglu and Restrepo2020), for example, find large and robust negative effects of robots on employment and wages across commuting zones. Technological change is also linked to job polarization and increasing income inequality, hurting especially those in the middle of the education and earnings distribution (Goos and Manning, Reference Goos and Manning2007). Although automation had mostly threatened workers who perform routine and repetitive tasks in the past, rapid developments in robotics and artificial intelligence now threaten even non-routine jobs. Frey and Osborne (Reference Frey and Osborne2017) estimate that 47 percent of American jobs are at high risks of automation.
Globalization receives outsized attention in political discussions, while automation is often ignored. This paper argues that the threat of automation intensifies efforts to restrict globalization. Individuals tend to displace blame for economic dislocations toward outgroups, immigrants and workers abroad. Using the American National Elections Studies (ANES), a nationally representative survey, I show that American workers facing higher risk of automation are significantly more likely to oppose free trade agreements and immigration, even when controlling for standard explanations for these attitudes. These findings suggest that workers are misattributing blame for harmful changes in labor markets toward immigrants and foreign workers.
This argument relates to two traditional approaches to the study of mass attitudes toward globalization. The first posits that individual preferences should reflect economic self-interest. In studying attitudes toward trade, analysts typically derive predictions about individuals’ material interests based on the Ricardo–Viner or Stolper–Samuelson model (Scheve et al., Reference Scheve, Slaughter and Slaughter2001). In doing so, they assume that citizens understand and can accurately identify the sources of their financial stressors or fortunes. The second approach demonstrates that citizens are either disinterested or unable to engage in such reasoning. These researchers instead emphasize non-economic influence on preferences. Opponents of globalization are not necessarily economic losers. Economic ignorance, ethnocentrism, political framing, and other symbolic attitudes, rather than pocketbook concerns, determine attitudes toward globalization (Mansfield and Mutz, Reference Mansfield and Mutz2013; Goldstein and Peters, Reference Goldstein and Peters2014; Rho and Tomz, Reference Rho and Tomz2017).
In this paper, I contend that grievances against globalization have economic origins, but economic losers often misattribute blame for their anxieties, and consequently, demand policies that poorly advance their interests. Although automation threat is associated with lower levels of job security, citizens do not always make the correct inference about the source of such anxiety. This paper uses the United States as an illustrative case where conditions are ripe for blame displacement, but the country is not unique. People in advanced economies tend to overestimate the extent of globalization (e.g., size of immigrant population) (Alba et al., Reference Alba, Rumbaut and Marotz2005; Semyonov et al., Reference Semyonov, Raijman and Gorodzeisky2008) and underestimate personal risks of automation. Many also see foreigners and robots through different lenses. Although many believe that technology will raise the competitiveness of their firms and facilitate human progress, outgroups are often viewed in zero-sum terms. Populist leaders tap into this sentiment by attributing economic anxieties to the competition from immigrants and workers abroad. Outside of the USA, globalization has also become a salient issue and is openly attacked by politicians across much of Europe (Mader et al., Reference Mader, Steiner and Schoen2020). According to theories of motivated reasoning, this vilification of foreigners is likely to be a particularly convincing narrative for those who are economically threatened as it validates their preexisting beliefs about zero-sum competition with outgroups, leading to higher rates of blame misattribution. Psychological research also demonstrates that blaming outgroup helps individuals restore a sense of personal control.
This misattribution of blame may lead to poor policy outcomes. If trade and immigration have key positive benefits for consumers and the overall growth of the economy, responding to technological change with closed borders may harm constituencies that derive key benefits from imported goods and immigrant communities. Protectionism may even hurt those who demand these policies in the first place if they are misidentifying the source of their economic problems. In addition, the overwhelming focus on tariffs and border controls as means to reduce inequality and prop up the middle class takes public attention away from other pertinent issues such as job automation. Technology destroys jobs, but it may also create new ones. It is important for individuals to be aware of the challenges and opportunities related to technology, and to discuss how governments may facilitate the development of skills that complement technological change and formulate appropriate adjustment policies for displaced workers. Compensating losers of globalization has also long been believed to weaken protectionist impulses (Hays et al., Reference Hays, Ehrlich and Peinhardt2005; Walter, Reference Walter2010; Schaffer et al., Reference Schaffer and Spilker2016). Finally, the misplacement of hostility has had important political ramifications on the state of globalization. Populist leaders in the United States, United Kingdom, and elsewhere have found electoral successes by framing globalization as the main cause of structural economic changes and promising a retreat from the global market. The fracturing of international political and economic unions and agreements are often economically detrimental and difficult to reverse.
2. Structural changes in the American economy
2.1 Globalization and secular economic trends
In developed economies, secular economic trends—deindustrialization, wage stagnation, and growing income inequality—coincided with immense increases in trade, offshoring, and immigration. The concurrence of growing global integration and structural economic change has led many to believe that reversing globalization will undo these changes. However, the scholarship shows that trade only accounts for a minor share of layoffs and other labor market shocks (Freeman, Reference Freeman1995; Di Tella and Rodrik, Reference Di Tella and Rodrik2020). Most workers in advanced economies are employed in the service sector and are largely immune to pressures of import competition. The manufacturing sector, which experienced higher incidences of trade-related layoffs, employs less than 10 percent of Americans. Although recent study on the “China shock” shows the negative effects of Chinese imports on jobs and wages, scholars find that employment gains due to export expansion roughly offset these losses (Feenstra et al., Reference Feenstra, Ma and Xu2019). Overall, trade barriers may protect only a modest number of workers while hurting many others: the export sector (Weymouth, Reference Weymouth2017) and consumers who enjoy welfare gains due to lower prices.
Populists also point the finger at immigrants for displacing native workers and depressing their wages, but research in economics provides little support for their claims. Using a natural experiment, Card (Reference Card1990) shows that the sudden influx of Cuban migrants had virtually no effect on wages or unemployment rates for low-skilled workers in Miami.Footnote 1 Peri and Sparber (Reference Peri and Sparber2009) further argue that immigrants boost productivity and wages in the long run. Native workers tend to transition into communication- and cognitive-intensive jobs as immigrants take lower-skill and manual jobs. Immigrants also contribute more in taxes than the benefits they receive. Populists’ economic case against immigrants is largely unsupported. Although protectionists have not gone so far as to advocate capital controls, they shame multinational firms for moving production abroad and call for onshoring. However, offshore workers are not good substitutes for native workers due to different task specializations (Ottaviano et al., Reference Ottaviano, Peri and Wright2013). Offshore workers are often assigned tasks of lower complexity and this leads to task-upgrading among natives. In addition, only a small number of workers lost their jobs due to offshoring. According to the Bureau of Labor Statistics (BLS), movement-of-work accounted for just 3 percent of layoffs.Footnote 2 Capital flows are also a two-way street, but the BLS does not collect data on jobs created by foreign firms in the United States.
2.2 Technological and automation threat
Although globalization has no doubt created winners and losers, neither international trade, immigration, nor offshoring explain the preponderance of the latter's plight. The literature on structural economic change emphasizes technology as a more important source of economic disruption. Since the 1980s, a marked increase in labor productivity due to technological upgrades enabled real-manufacturing output to almost double despite a 30 percent decrease in employment (Figure 1).Footnote 3

Figure 1. Manufacturing output and employment (1987–2018).
Note: Data from the Federal Reserve Bank of St. Louis Economic Research Division.
Although declining manufacturing employment was at the forefront of issues during the 2016 US presidential election, technology has played (and will likely play) a more important role in reshaping labor market outcomes than globalization, whether in the primary, manufacturing, or service sectors. Acemoglu and Restrepo (Reference Acemoglu and Restrepo2020) find large and robust negative effects of robots on employment and wages. The introduction of one new robot decreases employment by 5.6 workers, and an additional industrial robot per thousand workers reduces wages by 0.5 percent. These labor market effects most affected individuals with less than college education and workers in routine occupations. These findings are consistent with extant research on technological change which shows that post-1980 mechanization has been skill-biased and routine-biased, privileging skilled over unskilled workers, and non-routine over routine jobs (Bekman et al., Reference Bekman, Bound and Machin1998; Acemoglu and Restrepo, Reference Acemoglu and Restrepo2020). Importantly and perhaps surprisingly, technology has not produced offsetting employment gains in any occupation or education groups (Acemoglu and Restrepo, Reference Acemoglu and Restrepo2020).
Automation had thus far most threatened workers who perform routine tasks as machines have comparative advantage in performing well-defined tasks. Routine jobs can be cognitive or manual. Routine manual work tends to be blue collar jobs, such as food batchmakers and plant operators; whereas routine cognitive jobs generally require some level of precision, training, or education, examples include bookkeepers and travel agents. These jobs tend to be in the middle of the wage distribution (Goos and Manning, Reference Goos and Manning2007). Automation has yet to pose widespread challenges to those in non-routine cognitive and manual jobs that occupy the opposite ends of the skill- and wage-spectrum, although this is set to change. The former includes well-paid managerial, professional, and technical workers, including doctors and software programmers, whereas the latter are generally low-paying service sector jobs such as cleaners and servers. Taken together, technological change has led to the hollowing out of the middle and is linked to growing inequality. Figure 2 shows the most and least automated jobs in the early 2010s as reported by occupational experts and job incumbents.

Figure 2. The most and least automated jobs.
Note: Drawn from a survey sponsored by the Department of Labor. It asks a representative sample of job incumbents or occupation experts the extent of automation of their jobs.
Advances in artificial intelligence and robotics are putting more jobs at risk. Frey and Osborne (Reference Frey and Osborne2017) estimate that 47 percent of American jobs can be replaced by machines due to these new developments. Different from the past, computerization is no longer confined to routine tasks. Machine learning, including data mining, machine vision, and neural networks allow even cognitive tasks to be automated. Occupations which had not been computerized could face a high risk of automation in the future as technology becomes more sophisticated and cheaper.Footnote 4
Technology may in the long run create new jobs,Footnote 5 but workers displaced by technology may not be qualified for the new jobs that are created. Many former workers in routine occupations shifted into low-wage service jobs or dropped out of the labor force altogether. Oftentimes, they had to exit highly unionized sectors and settle for precarious non-unionized jobs and were, in many cases, deprived of medical benefits and pension rights. Laborers in demand in the future would tend to be those who are technically skilled or possess a comparative advantage over robots (e.g., good interpersonal skills and creativity).
3. Blame misattribution: scapegoating globalization
Although technology has disrupted workers’ livelihoods in the past and is poised to do so at a faster pace and larger scale in the near future, globalization is disproportionately blamed in politics. Populist leaders and their supporters believe retreating from the global economy will address the negative changes in the labor market of the last few decades.
3.1 Politically motivated framing by elites
The narrative that foreign imports and offshoring are killing the manufacturing sector became the dominant frame to understand job insecurity across much of the developed world. Campaign advertisements on trade had also been predominately negative, emphasizing trade as a source of unemployment (Guisinger, Reference Guisinger2017). Citizens’ poor grasp of economic trendsFootnote 6 makes them particularly susceptible to framing effects (Dür, Reference Dür2019). Although globalization is at the forefront of issues during elections, automation receives much less attention in most countries. In 2016, Clinton was the only major US presidential candidate who acknowledged the challenge of automation, but the issue was only mentioned six times on her campaign website of all materials she posted during the entire election cycle. Trump's senior advisors plainly rejected the role of automation in the decline of manufacturing.Footnote 7
There are political motivations for elites to emphasize globalization over automation. First, the nature of globalization renders it convenient for elites to use group cues, a potent political tool. Outgroups, immigrants, and workers abroad, who are seemingly making considerable gains make clear and conceivable targets to blame. Political psychology research shows that group cues can trigger anxiety independently of the actual threat posed by the outgroup (Brader et al., Reference Brader, Valentino and Suhay2008). Anxiety is known to cause behavioral changes that might be desired by politicians: anxious individuals are more likely to seek information, engage in protective responses, and vote. When people feel the need to compete for scarce resources, including jobs, they are more prone to see outgroup relations in zero-sum terms (Blumer, Reference Blumer1958; LeVine and Campbell, Reference LeVine and Campbell1972). Automation, on the other hand, does not fit as neatly in the “us-versus-them” narrative, and no prominent politician has adopted such a frame so far.
Second, the lack of an “easy fix” makes automation an issue less ready for elites to politicize. It is, for example, relatively straightforward for politicians to convince citizens that a border wall and tighter visa restrictions will keep immigrants out, and imposing high tariffs will stop the influx of cheap imports. Nationalistic and ethnocentric sentiments also help justify the costs of such remedies. However, it requires much more effort to persuade citizens that increased efficiency and productivity due to computerization are undesirable and that impediments on innovation are suitable responses to the “problem.”
3.2 Predilection for technology and downplaying of technological threat
Technology also makes for a poor target for attack because of the convenience it brings. According to the World Values Survey, a majority of Americans believe that science and technology make our lives easier and more comfortable (Table 1, Question 2). Few would trade a personal computer for a shared typist, the ATM machine for visits to a teller, or automatic exchanges for manual telephone switchboards—not even if the sacrifice would save an occupation. It is because most recognize that the world moves forward because of advances in science and technology (Question 1), and to retard innovation while the rest of the world promotes it would be unwise. A case against technological improvement would be politically difficult to make as only 6.9 percent of the respondents agree that more emphasis on the development of technology is bad (Question 3). Similar pro-technology sentiments are observed in most parts of the world.
Table 1. Public attitudes toward technology (WVS, United States, 2011)

Note: 1=Strongly Disagree; 10=Strongly Agree.
The public's familiarity with technology helps explain the stark difference in attitudes toward machines during the Industrial Revolution and today. In recent history, workers have shown positive attitudes toward technology in the workplace. In an in-depth study of German workers, Thelen (Reference Thelen1991) shows that labor unions and workers were “fundamentally receptive to technological changes,” believing that firms’ failure to adapt new technology would make them lose their competitiveness and eventually force them out of business. Milkman (Reference Milkman1997) finds that technology vastly improved American autoworkers’ work environments and they considered technology a “necessary evil” to ensure their company's survival. An Office of Technology Assessment of the United States (1983) report states that “willing acceptance” of new technology was the most common response of unions and their opposition to technology tended to disappear once union leaders were convinced that their members would not be negatively affected or would receive appropriate compensation if they were.
Not only do individuals welcome the benefits of technological change, but they also have a tendency to downplay its risks. Automation is not on the minds of most. Of the 3650 responses to an open-ended question about the most important problem facing the United States in the nationally representative 2016 ANES, only three mentioned automation or technology. When directly prompted, two-thirds of American respondents expect machines “to do much of the work currently done by humans within fifty years” (Pew Research Center, Reference Pew Research Center2016). However, four-fifths of them think that their jobs will continue to exist in five decades. It is well documented in psychological research that people are more optimistic about their own prospects than those of others. In a study, college students believed that they were 50.2 percent more likely than their peers to land a job after graduation and 44.3 percent more likely to own a home (Weinstein, Reference Weinstein1980). Unrealistic optimism or over-confidence is considered to be a defensive strategy motivated by a need to reduce anxiety. It may also be a result of cognitive biases. Individuals have a bias in recall—they are more likely to bring to mind personal actions, experiences, plans, and attributes that make favorable outcomes more likely for them, but they do not give the stereotypical person the same consideration (Tversky and Kahneman, Reference Tversky and Kahneman1974; Weinstein, Reference Weinstein1980).
3.3 Intense media attention and overestimation of the extent of globalization
Although citizens downplay the risks of automation, journalists and the public tend to overstate the extent and downsides of globalization. The media sets the agenda and influences how issues are evaluated. Globalization is more heavily covered and scrutinized harder than automation. The often visible nature of offshoring—the image of a plant shutting down and relocating its operations—as opposed to automation which happens out of public view may have contributed to the discrepancy in reporting (Margalit, Reference Margalit2011).
News coverage of globalization also tends to emphasize its various problems and negative effects, such as employment concerns, factory closings, and safety and security risks. Analyzing over 40 years of trade news, Guisinger (Reference Guisinger2017) finds that “bad news” generate almost 40 percent more stories than “good news.” Like journalists, the public responds more strongly to negative than positive news, potentially leading responsive governments to take overly aggressive measures (Soroka, Reference Soroka2006). Technological change in manufacturing, in comparison with international competition, is a trend that receives little media (and thus public) attention.
3.4 Empirical expectations
Due to the much higher salience of globalization and the public's predilection for technology, I expect that workers at risk of automation are more likely to attribute their economic anxieties to globalization, instead of technology. Empirically, the theory of blame displacement suggests that increased automation threat should be associated with more hostile views toward immigration (hypothesis 1), trade (hypothesis 2), and offshoring (hypothesis 3).
4. Research design
I use the 2016 ANES to examine these hypotheses. The ANES does not have a ready-to-use measure of occupation that is detailed enough to allow the matching of meaningful estimates of automation risks. I thus leverage individual responses to an open-ended question about their occupations. Respondents are asked to describe “the kind of work [they] do” and “[their] most important activities or duties at work.” Based on these descriptions, I classified individuals into detailed occupation categories using the 2010 Standard Occupational Classification (SOC) system. In total, 2701 respondents provided descriptions of a current position and 2652 of them were assigned SOC codes.Footnote 8 For example, individuals who describe their jobs as “server, wait tables” are coded as “waiters and waitresses (35-3031.00).” People who “take care of the elderly” are classified as “personal care aides (39-9021.00).” In cases where respondents describe duties that could fit multiple related occupations, I assign them multiple SOC codes. For instance, individuals whose jobs are “special education teacher” are coded as “special education teachers, preschool (25-2051.00),” “special education teachers, kindergarten and elementary school (25-2052.00),” “special education teachers, middle school (25-2053.00),” and “special education teachers, secondary school (25-2054.00).” Their risk of job automation is the average of the estimate of each of these occupations.Footnote 9
There are two variables related to automation in the model. The first measures past levels of automation. The data comes from a survey administered by O*NET, which was sponsored by the Department of Labor. One of the questions asks a representative sample of job incumbents and occupation experts how automated their jobs are. Each SOC code has a corresponding subjectively assessed level of automation. It ranges from 0 to 1, from not at all automated to fully automated. In the past, researchers had typically used indicators that measure the prevalence of routine tasks in an occupation.Footnote 10 This self-reported data should provide a more accurate account of past levels of automation. Jobs sometimes remain manual because initial costs are prohibitive, not because it is technologically infeasible to automate. Job incumbents and experts are likely to have a better sense of the extent of automation on the ground. An individual holding a job with a high retrospective level of automation in our dataset means that he or she is working in an area where sweeping automation has occurred, but he or she continues to hold the job (e.g., to program machines). The important implication is that these individuals are likely to have witnessed automation in their workplace, but are the survivors, if not victors, of technological change.
The second and main independent variable is a prospective estimate of job automation risk. I adopt Frey and Osborne (Reference Frey and Osborne2017) measure. This proxy indicates the risk of automation for each occupation with the expectation that automation will become more sophisticated and cheaper over the next decades, from 0 (not computerizable) to 1 (totally computerizable). The authors, together with a group of machine learning researchers, determined whether 70 occupations are automatable or not based on the detailed descriptions of tasks of occupations on O*NET, an online service developed for the Department of Labor. A Gaussian process classifier was then used to estimate the probability of computerization for other detailed occupations based on a training set hand-labeled by the researchers. An occupation is deemed susceptible to computerization if the tasks of the job can be sufficiently specified and performed by state-of-the-art computer-controlled equipment. They further consider the extent to which the automation of those tasks would require overcoming engineering bottlenecks such as perception and manipulation, creative intelligence, and social intelligence. For example, the job of a fashion designer is less computerizable and under a much lower threat than a court clerk because creativity is needed to perform the tasks of the former.
The retrospective and prospective indicators measure different dimensions and facets of workplace automation. Figure 3 shows that the extent to which jobs had already computerized and the likelihood of future automation are only very weakly correlated. Unlike in the past, automation is no longer confined to routine, well-defined tasks. Computers can now perform many tasks that were, not long ago, considered impossible to automate. Levy and Murnane (Reference Levy and Murnane2005) wrote that drivers are immune from job automation because “it is hard to imagine discovering the set of rules that can replicate [a] driver's behavior.” Little did they know that autonomous driving would become an area of intense engineering and machine learning focus. On the other hand, there are occupations that had been heavily computer-assisted but are unlikely to be completely automated in the near future, such as air traffic controllers and medical services managers.

Figure 3. Degree of past automation and future automation risk.
Note: Retrospective and prospective measures of automation are only weakly correlated. The density plots show the distribution of the observations.
The outcomes of interest are directly drawn from the ANES.Footnote 11 The dependent variables include attitudes toward free trade agreements, immigration, and offshoring for individual i, in occupation j, in industry k, and living in congressional district l. I regress individuals’ attitudes on trade, immigration, and offshoring on these measures of automation. In addition, I control for the offshorability of individual i's job given their occupation j. I use Blinder and Krueger (Reference Blinder and Krueger2013)'s measure.Footnote 12 An occupation is deemed not offshorable if its tasks must be performed at specific location, and require personal contact with end users. The measure ranges from 1 (not offshorable) to 5 (offshorable with minor or no difficulty or quality loss). I also hold constant the level of immigration in i's congressional district l and import exposure in i's industry k. Level of immigration is defined as the size of the foreign-born population in a congressional district. Import penetration is operationalized as the total amount of imports over total sales in the United States in an industry (three-digit NAICS) (Owen and Johnston, Reference Owen and Johnston2017).
Furthermore, the model includes other variables known to affect i's policy attitudes and preferences—education, gender, age, family income, party identification, ethnocentrism, and nationalism. For ethnocentrism, I follow Kinder and Kam (Reference Kinder and Kam2009)'s method of estimation. It is defined as the average of the three out-group thermometers subtracted from the in-group thermometer. Each respondent is in one of these four groups: Asians, Blacks, Hispanics, or Whites. Ethnocentrism and nationalism are distinct concepts. Although ethnocentrism relates to how one evaluates their own ethnic group vis-a-vis other groups, nationalism relates to one's feeling toward their own country. Individuals who are in the labor force, meaning those who are either employed or unemployed but looking for work, are included in the main analysis. Those who are not are excluded because they are no longer directly exposed to the automation risks.Footnote 13
The data have a multilevel structure with four levels of analysis. To avoid biasing standard errors downward and producing spuriously “significant” effects, I adopt a multilevel ordered logistic model.Footnote 14 The fully specified model is as follows:

In this equation, Y ijkl are the outcomes of interest. X ijkl is a vector of individual-level covariates. Z jkl is a vector of occupational-level covariates. V kl is a vector of the industry-level covariate. Q l is a vector of the congressional district-level covariate. β1 is the fixed effect parameter for individual-level covariates. γ1 is the fixed effect parameter for occupational-level covariates. θ1 is the fixed effect parameter for the industry-level covariate. λ1 is the fixed effect parameter for the congressional district-level covariate. τj, ζk, and μl are random intercepts. εijkl is the error term.
5. Results
I report the main results in two ways. Table 2 presents results from multilevel ordered logistic regressions and Figure 4 features average marginal effects plots of the main variables of interest. In all, empirical analyses show that the threat of automation is linked to more intense hostility toward globalization, particularly immigration and trade. Table 2 shows that workers who are exposed to higher risks of automation are more likely to prefer tighter immigration restrictions (models 1 and 2) and oppose the negotiation of free trade agreements (models 3 and 4), providing support for hypotheses 1 and 2. These results hold in all model specifications, even with the addition of demographic and attitudinal controls. However, the relationship between automation risk and attitudes toward offshoring (models 5 and 6) is not statistically significant, but is in the expected direction. It may be due to the fact that there is little variation in the dependent variable. An overwhelming majority, 70 percent, of the respondents believe that the government should discourage companies from hiring workers abroad. Only 4 percent think that the government should encourage offshoring. This animus view toward offshoring is in line with findings in Mansfield and Mutz (Reference Mansfield and Mutz2013).
Table 2. Attitudes toward globalization (workers in labor force)

Note: Results from multilevel ordered logistic regressions of globalization attitudes on hypothesized determinants. Standard errors are in parentheses. *p < 0.05, **p < 0.01, ***p < 0.001.

Figure 4. Average marginal effects for key variables predicting attitudes.
Note: Average marginal effects based on ordered logistic regressions of attitudes toward globalization and technology on hypothesized determinants. This sample includes Americans in the labor force.
The confidence intervals of the average marginal effects of automation risk on immigration and trade attitudes do not overlap with zero (Figure 4). Especially, considering the real-world context, these effects are substantively important. Research thus far shows that the adverse impact of job automation is unevenly distributed (e.g., conditional on educational attainment) and will likely grow stronger in the years to come (Frey and Osborne, Reference Frey and Osborne2017; Kurer and Palier, Reference Kurer and Palier2019; Gallego et al., Reference Gallego, Kurer and SchöllForthcoming). Automation's impact is also expected to be geographically concentrated and will likely hit the industrialized Midwest and several low-wage metropolitan areas the hardest. There is evidence that robot exposure had swung the 2016 presidential election in favor of the more radical anti-status quo candidate (Frey et al., Reference Frey, Berger and Chen2018). Observing a similar phenomenon, Im et al. (Reference Im, Mayer, Palier and Rovny2019) find that automation threat increased support for radical right parties in 11 West European countries. A revival of radical politics fueled by outgroup scapegoating may cause long-lasting damages to the international system.
Overall, the effects of various control variables are consistent with our conventional understanding of their influences on globalization attitudes: education is negatively associated with protectionist sentiments, nationalism and ethnocentrism strongly correlates with immigrant hostility; and female respondents are more protectionist. There are two ostensible anomalies. First, strong Republicans are more likely to be anti-trade and anti-immigration than Democrats. In 2016, self-identified Republicans’ trade positions are inconsistent with those long held by the party itself (Karol, Reference Karol2000; Irwin, Reference Irwin2020), but they are consistent with those held by the presidential candidate of their party in the election, who controlled much of the narrative. Second, job offshorability and globalization attitudes do not share statistically significant relationships. Owen and Johnston (Reference Owen and Johnston2017) and Kaihovaara and Im (Reference Kaihovaara and Im2020), using European samples, find that offshorability conditions the effect of technological risks on globalization attitudes. However, in this American sample, I do not find such an effect using an interaction term of automation susceptibility and offshorability.Footnote 15
Additional robustness checks can be found in the online Appendix (A.1). Findings do not change meaningfully with a different estimation method and weights. These findings together offer evidence for the theory that workers facing higher risks of automation tend to displace their blame toward globalization. At-risk workers are more likely to oppose trade agreements and favor immigration restrictions, even after accounting for conventional explanations for these attitudes.Footnote 16
5.1 Further discussion and subgroup analyses
There are other findings and potential challenges to the paper that merit further discussion. At the first glance, it may seem counterintuitive that automation exposure in the past is not linked to immigration hostility (models 1 and 2, Table 2) and is even associated with more positive views toward free trade (models 3 and 4, Table 2). It is worth highlighting that workers in this analysis are still in the labor force and had most likely “survived” automation.Footnote 17 If computerization and mechanization are conducive to higher productivity and enhanced competitiveness, workers who are currently in more automated occupations may have an edge in the international market, making them not merely the survivors of technological change but also the winners of it. Among those who survived, animosity toward economic outgroups is driven not by past levels of computerization, but the prospective threat of automation.
Admittedly, the existing survey data limit our ability to trace and identify workers who did not survive technological change. Although the ANES is administered regularly, it is not a panel survey. Given these constraints, I leverage information on respondents’ past occupations for a preliminary analysis of the relationship between past automation and globalization attitudes among individuals who might have been displaced by technology. This analysis includes those who are no longer in the labor force or are currently unemployed but seeking work. These individuals must have had at least a job in the past to be included in the sample, as a job is the prerequisite for automation exposure. As we do not know when these workers might have been displaced, certain time-dependent covariates (e.g., import exposure at the time of displacement) have to be dropped. The variable measuring prospective risk of automation is also moot. Results in Table 3 show that past levels of automation have a statistically significant effect on protectionist trade and immigration policy preferences.Footnote 18 This suggests that probable losers of technological change are more opposed to globalization.
Table 3. Attitudes toward globalization and technology (potentially displaced individuals)

Note: Results from ordered logistic regressions of globalization attitudes on hypothesized determinants. Standard errors are in parentheses. *p < 0.05, **p < 0.01, ***p < 0.001. Respondents included in this analysis are those who are working in non-tradable sectors as defined by Blinder and Krueger (Reference Blinder and Krueger2013).
However, there may be reasons for us to believe that automation and globalization are related processes. If this is true, how do we know that losers of technological change adopt more protectionist policy preferences as a result of blame displacement, but not because of a rational response to material losses due to globalization? Although the structure of the ANES limits identification options, I reanalyze the data with only a subsample of Americans who worked in non-tradable sectors (as defined in Blinder and Krueger, Reference Blinder and Krueger2013). Examples of non-tradable occupations are teachers, firefighters, and restaurant servers. The intuition is that workers in non-tradable sectors are only minimally affected by import competition, and thus should have few economic reasons to be against trade. They may even favor trade in their capacities as consumers (Naoi and Kume, Reference Naoi and Kume2015). If we observe stronger hostility toward trade among workers exposed to higher threats of automation in this subsample, there are stronger reasons for us to believe that outgroup scapegoating may be at play.
Using the same ANES data, Table 4 shows that workers in non-tradable sectors facing higher threats of job automation are still more likely to support immigration restrictions, oppose free trade agreements, and prefer the government to discourage offshoring. These findings are robust and consistent across five out of six models, with and without controls. Figure 5 presents combined plots of average marginal effects for key variables predicting attitudes for both the main sample comprising all workers in the labor force and the non-tradable subsample. The magnitudes of effect of automation risk on attitudes are comparable or larger in the subsample than in the main sample. The consistent findings offer additional evidence that hostility toward globalization is related to anxiety about future job automation.
Table 4. Attitudes toward globalization and tech spending (workers in non-tradable sector)
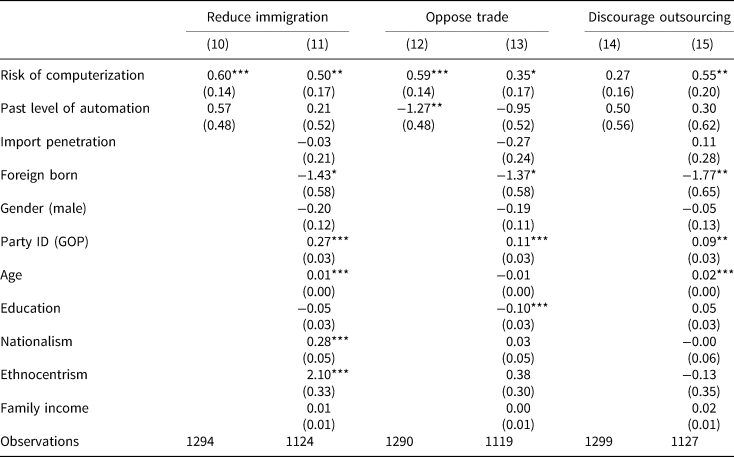
Note: Results from ordered logistic regressions of globalization attitudes on hypothesized determinants. Standard errors are in parentheses. *p < 0.05, **p < 0.01, ***p < 0.001. Respondents included in this analysis are those who are working in non-tradable sectors as defined by Blinder and Krueger (Reference Blinder and Krueger2013).

Figure 5. Average marginal effects for key variables predicting attitudes (full and non-tradable subsample).
Note: Average marginal effects based on ordered logistic regressions of attitudes toward globalization and technology on hypothesized determinants. This main sample includes Americans in the labor force, the non-tradable subsample includes workers in the non-tradable sector as defined by Blinder and Krueger (Reference Blinder and Krueger2013).
6. Conclusion
The existing scholarship on structural economic change emphasizes the impact of technology on wages and employment. Although immigration and trade only account for a small percentage of layoffs, globalization takes the brunt of the blame for labor market anxieties (Card, Reference Card1990; Peri and Sparber, Reference Peri and Sparber2009; Ottaviano et al., Reference Ottaviano, Peri and Wright2013; Helpman, Reference Helpman2018). Although decades of studies in economics have documented the distributional consequences of technology related to employment, income, inequality, and health, the political effects of automation are not well understood. This paper contributes to the nascent literature by examining the impact of automation on public opinion on globalization and technological change. Using a nationally representative survey, it shows that automation threat may have intensified globalization resistance. Workers who are threatened by automation are prone to displacing blame for labor market threats toward immigrants and foreign workers. This misattribution of blame is due to politically motivated framing by elites to reinforce ingroup and outgroup differences, the visibility of globalization-induced job losses relative to technological displacement, and the public's predilection for technology. Although this paper features a single-country study of the United States, these conditions for blame misattribution are present across much of the developed world.
To evaluate the theory empirically, I analyzed the American National Election Studies. I leveraged answers to an open-ended question about individuals’ occupations to assess their automation risks. I found that workers facing higher risks of automation are more likely to oppose free trade agreements and favor immigration restrictions, even after accounting for conventional explanations for these attitudes. These results are robust and consistent across different models and model specifications. The impact of automation threat on attitudes toward production offshoring, however, is not statistically significant. It might be attributable to the low variation in opinion toward offshoring, which is predominately adverse. A subsample analysis of individuals in non-tradable sectors provides additional evidence of blame misattribution. Even for those who are minimally affected by trade, automation threat is associated with protectionist policy preferences. Overall, the evidence suggests that automation anxiety increases attempts to resist globalization.
The theory of blame misattribution has important implications for debates over the determinants of globalization attitudes. The premise in open economy politics that economic self-interest shapes individual preferences was once regarded as conventional wisdom. Later research challenges the notion and suggests that non-economic factors, such as ethnocentrism and racism, rather than pocketbook concerns, determine preferences toward globalization. This paper shows that grievances against globalization have economic origins, but it calls into question the standard assumption that individuals understand and can correctly identify the sources of their economic anxieties. Anti-globalization attitudes are based on the beliefs, but not necessarily the reality, that foreign outgroups are the sources of their economic anxieties. Given these believes, demands for protectionist policies are motivated by self-interest regardless of whether these policies actually address their economic concerns.
Correct blame attribution is important for three main reasons. First, the misplacement of hostility toward globalization can lead to collective decisions that might be economically detrimental and difficult to be reversed, such as the fracturing of international political and economic unions (e.g., Brexit) and the election of political leaders who have protectionist agendas and the executive power to reverse trade liberalization. Second, the misattribution of blame may lead to poor policy outcomes. If free trade and immigration are linked to overall welfare gains, responding to technological change with protectionism may hurt constituencies that derive key benefits from globalization. Protection may even hurt those who demand these policies in the first place if they misidentified the source of their economic problems. Finally, it is only through the understanding of the real challenge to work that citizens can better prepare themselves for the advent of large-scale job automation. Knowledge of the threat of automation will also motivate more informed discussions about appropriate adjustment and compensation measures for displaced labor.
Supplementary material
The supplementary material for this article can be found at https://doi.org/10.1017/psrm.2021.43.
Acknowledgments
I thank Ted Brader, Mary Gallagher, Gary Herrigel, Anil Menon, James Morrow, Iain Osgood, Sergi Pardos-Prado, Emmanuel Teitelbaum, Clayton Webb, Stan Wong, Yujeong Yang, and participants at the 2017 Varieties of Backlash Workshop, the 2018 Pacific International Politics Conference, the 2018 APSA Annual Meeting, the 2018 Peace Science Society International Annual Meeting, the 2019 EPSA Annual Meeting, Michigan's Political Economy Workshop, and Princeton's International Relations Faculty Colloquium for their thoughtful suggestions.