Introduction
Protected areas are widely recognized for their critical role in conserving biodiversity in situ. They can benefit neighbouring communities, but living in close proximity to reserves and the wildlife they protect also incurs costs. The degree to which benefits outweigh costs has been debated extensively (Scherl et al., Reference Scherl, Wilson, Wild, Blockhus, Franks, McNeely and McShane2004; Wilkie et al., Reference Wilkie, Morelli, Demmer, Starkey, Telfer and Steil2006; de Sherbinin, Reference de Sherbinin2008; Naughton-Treves et al., Reference Naughton-Treves, Alix-Garcia and Chapman2011; Vedeld et al., Reference Vedeld, Jumane, Wapalila and Songorwa2012; Clements et al., Reference Clements, Suon, Wilkie and Millner-Gulland2014). Conservation conflicts develop when conservation objectives compete with other interests, or when people are affected by species of conservation priority (Redpath et al., Reference Redpath, Young, Evely, Adams, Sutherland and Whitehouse2013; Redpath et al., Reference Redpath, Bhatia and Young2015). The former considers the human–human dimension of conflicts and the underlying disagreement between conservation objectives and livelihoods, whereas the latter is concerned with developing solutions to human–wildlife impacts that arise when communities compete with wildlife for space and resources (Redpath et al., Reference Redpath, Bhatia and Young2015).
Technical approaches, although only one component in the long-term management of conservation conflicts, are urgently required to reduce rates of negative interactions between people and wildlife, and to lessen the associated effects upon livelihoods and conservation objectives (Woodroffe et al., Reference Woodroffe, Thirgood, Rabinowitz, Woodroffe, Thirgood and Rabinowitz2005; Dickman, Reference Dickman2010). Wildlife can have an impact on people by damaging assets (crops, livestock, structures), causing physical injury or death, and creating fear and restrictions to movement. People affected in this way are more likely to harbour negative attitudes towards conservation efforts, support the abolishment of protected areas and the use of lethal control, and use impacts as an excuse for poaching (Newmark et al., Reference Newmark, Leonard, Sariko and Gamassa1993; De Boer & Baquete, Reference De Boer and Baquete1998; Hill, Reference Hill2004; Madden, Reference Madden2004; Malima et al., Reference Malima, Hoare and Blank2005; Mbaiwa et al., Reference Mbaiwa, Ngwenya and Kgathi2008, Sebotho & Toteng, Reference Sebotho and Toteng2010).
The African elephant Loxodonta africana, categorized as Vulnerable on the IUCN Red List, is at risk from poaching for ivory, habitat loss, and retaliatory killings (Thouless et al., Reference Thouless, Dublin, Blanc, Skinner, Daniel and Taylor2016). Because of human activities, the species is estimated to occur at < 25% of its potential density (Robson et al., Reference Robson, Trimble, Purdon, Young-Overton, Pimm and Van Aarde2017). This is of concern because elephants play a critical role in savannah ecosystems by dispersing seeds, maintaining open wooded grasslands, increasing habitat structural complexity, and facilitating local increases in the abundance and diversity of small vertebrates (Western, Reference Western1989; Pringle, Reference Pringle2008).
Stable or increasing elephant populations are regarded as a priority objective amongst conservationists, but this can create conflict with competing interests such as agriculture. Communities often report elephants as the most significant contributors to crop damage among large animals (Newmark et al., Reference Newmark, Manyanza, Gamassa and Sariko1994; Nyhus & Tilson, Reference Nyhus and Tilson2000; Nelson et al., Reference Nelson, Bidwell and Sillero-Zubiri2003; Vedeld et al., Reference Vedeld, Jumane, Wapalila and Songorwa2012; Pittiglio et al., Reference Pittiglio, Skidmore, van Gils, McCall and Prins2014; Gross et al., Reference Gross, Lahkar, Subedi, Nyirenda, Lichtenfeld and Jakoby2018). Although damage caused by small mammals and birds may be most prevalent, elephants are of particular concern because they can cause widespread and economically catastrophic damage in a short period of time and typically do so during harvest periods, when crop loss is most detrimental from both a subsistence and an economic perspective (Naughton-Treves, Reference Naughton-Treves1998; Gillingham & Lee, Reference Gillingham and Lee2003; Madden, Reference Madden2004; Gadd, Reference Gadd2005; Thirgood et al., Reference Thirgood, Woodroffe, Rabinowitz, Woodroffe, Thirgood and Rabinowitz2005; Gross et al., Reference Gross, Lahkar, Subedi, Nyirenda, Lichtenfeld and Jakoby2018).
In areas where elephants damage crops, community members and protected area managers may utilize a variety of technical approaches to prevent damage and mitigate losses. Traditional strategies include the use of fire, loud noises, guarding, patrols, dogs, and the construction of barriers, such as stone walls, thorny or fast-growing plants (e.g. sisal), and trenches (Nelson et al., Reference Nelson, Bidwell and Sillero-Zubiri2003). Novel methods that exploit elephant behaviour include beehive fences, drones, and the use of chili pepper along fences and in briquettes (King et al., Reference King, Lawrence, Douglas-Hamilton and Vollrath2009, Reference King, Lala, Nzumu, Mwambingu and Douglas-Hamilton2017; Hedges & Gunaryadi, Reference Hedges and Gunaryadi2010; Chang'a et al., Reference Chang'a, de Souza, Muya, Keyyu, Mwakatobe and Malugu2016; Hahn et al., Reference Hahn, Mwakatobe, Konuche, de Souza, Keyyu and Goss2017; Pozo et al., Reference Pozo, Coulson, McCulloch, Stronza and Songhurst2019). Other technical approaches include electric fencing, the identification of individual problem animals for removal or translocation, compensation schemes, and land-use planning (Thouless & Sakwa, Reference Thouless and Sakwa1995; Kioko et al., Reference Kioko, Muruthi, Omondi and Chiyo2008; Davies et al., Reference Davies, Wilson, Hazarika, Chakrabarty, Das, Hodgson and Zimmermann2011; Hoare, Reference Hoare2012). All technical approaches have trade-offs in costs, efficacy, local suitability, and opportunity to deploy at scale (Distefano, Reference Distefano2005).
Conservation resources are limited and protected areas are often underfunded. This, and the fact that areas of extreme poverty often coincide with biodiversity hotspots, remains a limiting factor for biodiversity conservation (Barrett et al., Reference Barrett, Travis and Dasgupta2011; Juffe-Bignoli et al., Reference Juffe-Bignoli, Burgess, Belle, de Lima, Deguignet and Bertzky2014; Watson et al., Reference Watson, Dudley, Segan and Hockings2014). Efficient allocation of resources is therefore critical to achieve effective outcomes. A first step in this process is to understand the spatial and temporal extent of impacts, and to evaluate the factors contributing to increased risk of damage (Pozo et al., Reference Pozo, Coulson, McCulloch, Stronza and Songhurst2017). This information can then be used to identify priority areas for management and help inform the development of locally suitable strategies for mitigation and prevention.
Here, we use historical records to describe spatial and temporal trends in crop damage in the western Serengeti. We examine how spatially aggregated damage reports, a common source of primary data on the occurrence of human–wildlife impacts, may be interpreted and utilized, and discuss how the results of our analysis can be used to inform the allocation of prevention and mitigation efforts. We examine the influence of land use on the risk of crop damage at the village level, and comment on potential implications for land-use planning efforts that aim to reduce negative interactions between people and wildlife.
Study area
This work assesses crop damage patterns in Bunda and Serengeti Districts in the Mara Region, northern Tanzania (Fig. 1). The study area is bordered by a number of protected areas of varying designations that primarily prohibit extractive uses. The Ikorongo and Grumeti Game Reserves and the Ikona Wildlife Management Area provide an important buffer between permanent settlements and Serengeti National Park and are critical habitat zones during the famed seasonal herbivore migration.
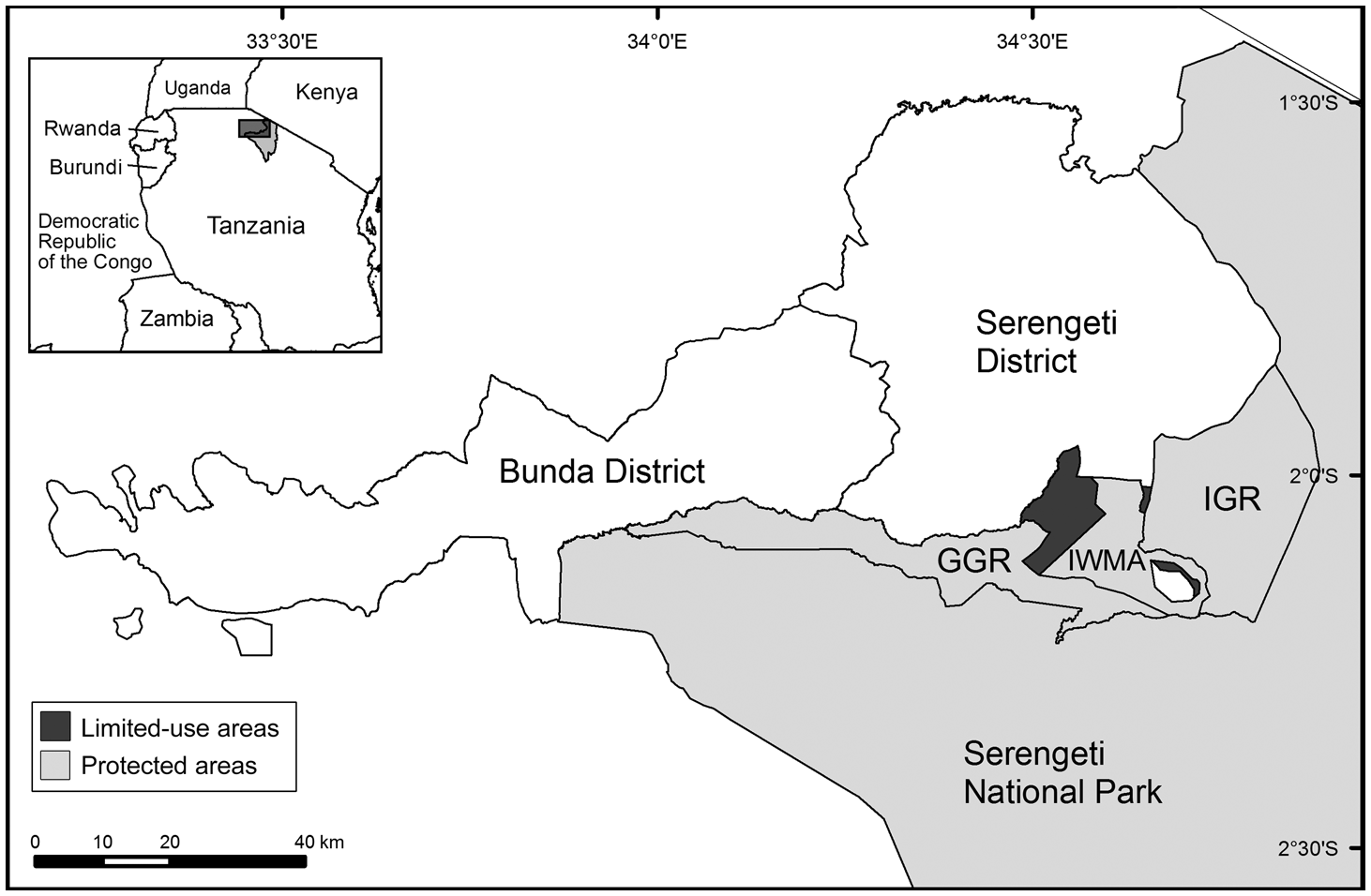
Fig. 1 The study area, which included villages in Bunda and Serengeti Districts in the Mara Region, Tanzania. Protected areas bordering the study villages include Serengeti National Park, Grumeti (GGR) and Ikorongo (IGR) Game Reserves, and the Ikona Wildlife Management Area (IWMA). One village (Robanda) is located within the IWMA. Limited-use areas are village-managed areas for livestock grazing and tree felling.
Effective conservation management and law enforcement have contributed to dramatic increases in animal populations, particularly of the African elephant, since 2003. Most notably, aerial surveys estimated a 50% increase in the total number of elephants during 2012–2014 (Goodman & Mbise, Reference Goodman and Mbise2016). The species currently uses more extensive areas of the Ikorongo and Grumeti Game Reserves than observed in the past. Crop damage has become a significant issue in villages that neighbour the reserves, and a source of increased tension between community members and park officials (Walpole et al., Reference Walpole, Ndoinyo, Kibasa, Masanja, Somba and Sungura2004; Kideghesho et al., Reference Kideghesho, Røskaft and Kaltenborn2007; Fridolin, Reference Fridolin2014). Reducing the occurrence and mitigating the effects of crop damage by elephants is a top priority among community members and protected area managers.
Agricultural activities are the most important source of income in both Bunda and Serengeti Districts; nearly all households are engaged in cropping (NBS, 2012; Grumeti Fund, 2016). Communities in the study area are vulnerable: access to electricity is limited, poverty is pervasive, and there are many socioeconomic barriers to educational attainment (Grumeti Fund, 2016). These districts are isolated from urban centres and large markets. Many community members associate few or no benefits with living in close proximity to a protected area, yet damage caused by wildlife is an additional burden (Walpole et al., Reference Walpole, Ndoinyo, Kibasa, Masanja, Somba and Sungura2004; Grumeti Fund, 2016). Permanent settlements are established directly along the reserve border in many areas, without a suitable buffer zone.
Methods
Record collection & standardization
Crop damage records for 2012–2014 were compiled in the summer of 2015 by staff at the Grumeti Fund, for monitoring purposes. Three measures of damage are included: reports, incidents and damaged area. Reports were filed at district offices for compensation purposes and represent damage at the household level. Area was recorded for each report and represents an estimate of damage extent. Reports were aggregated into incidents based on report date and village, to determine unique events where elephants left the reserves and damaged crops. Reports did not contain GPS locations and were aggregated by village.
Damage was unlikely to be over-reported. Reports are subject to verification by village and district agricultural officers, the compensation process requires extensive paperwork, claims take extended periods of time to process, and when consolation payments are distributed, their value accounts for only a fraction of what was lost. As a consequence, under-reporting was likely. To account for this and for uncertainty regarding how under-reporting varied between villages, within-village trends of conflict density were not assessed. We deemed incomplete reports less reliable or less likely to have been verified and excluded those that did not contain area, crop type, date, or location information.
Statistical analysis
We compared general trends in damage between villages bordering and not bordering a protected area. The Mann–Whitney U test was used to examine differences between border groups in report and incident density and damage intensity, measured as area damaged per report and incident. For comparisons of damage intensity, we eliminated outliers to exclude potential mistakes in reporting and to prevent rare, abnormal events from exerting too much influence. We determined extreme outliers by individual crop type and defined them as reports with a recorded area damaged more than three times the interquartile range (Tukey, Reference Tukey1977).
Using binomial logistic general additive models, we examined the contribution of various environmental factors to the probability of crop damage at the village level. Using a binary response seemed appropriate because of concerns of under-reporting; we determined that categorization of damage presence–absence across the 3-year period was the most reliable metric. General additive models use non-parametric smoothing functions to estimate response curves; both linear and nonlinear responses can be included within the same models (Hastie & Tibshirani, Reference Hastie and Tibshirani1986). This approach is particularly useful in cases where there are no a priori assumptions of a linear relationship between the response (in the case of binomial logistic regression, with the logit of the response) and the independent variables, or when the examination of relationships and potential thresholds are of particular interest.
We constructed models using the R package mgcv (Wood, Reference Wood2016). We selected predictor variables based on previous research that indicated their association with damage by elephants and represent easily measurable landscape features (e.g. distance of village to nearest waterway) and factors related to land-use planning and human disturbance (e.g. distance to nearest protected area, proportion of land converted for agriculture, distance to nearest road, built footprint; Table 1). We did not include estimates of population density because up-to-date village-level census data were not available. As the response is binomial and we were unable to directly control for variability in village geometry by adjusting the response variable to reflect incident rates per unit area, we included village attributes that are expected to increase elephant encounter rates (e.g. total area, length of shared boundary with a protected area) as additional predictor variables.
Table 1 The explanatory variables used as predictors for crop damage by elephants at the village level.

We first assessed independent variables in isolation, and then used them in combination to construct two models of the risk of crop damage: (1) an explanatory model of elephant crop damage as relating to human land use, and (2) a predictive model considering all variables, to identify the villages most at risk. Because of the small sample size of villages reporting crop damage and the requirements for logistic regression, we limited covariates to a maximum of three and examined them in all possible combinations. Highly correlated predictor variables (r > 0.7) were not examined in combination (distance to protected area and distance to river). We selected the models that best approximated crop damage risk based on Akaike Information Criterion (AIC) scores (Akaike, Reference Akaike1974) and determined variable inclusion by relative contributions to AIC and per cent deviance explained.
We examined the residuals of the best-performing model for spatial autocorrelation, using Monte Carlo simulations of Moran's I (Moran, Reference Moran1950). The ability of models to correctly distinguish between the presence and absence of crop damage was assessed using area under the curve by constructing receiver operating characteristic plots, which compare the distribution of true and false positives across probability thresholds (Fielding & Bell, Reference Fielding and Bell1997). We used the explanatory model of human land use to qualitatively describe the relationship between metrics of human disturbance and proximity to a reserve and the risk of crop damage. The predictive model was used to map the probability of crop damage in each village.
We used χ 2 tests to assess differences in damage by month, and calculated effect size using the contingency coefficient: values > 0.5 were considered evidence of a large effect, > 0.3 a medium effect, and > 0.1 a small effect. We examined adjusted residuals (called standardized residuals in output from the chisq.test package in R) to determine significance. We applied a Bonferroni adjustment to the z critical value, where z was determined by alpha divided by the number of cells in the contingency table (Sharpe, Reference Sharpe2015). We made comparisons using area, reports, and incidents. This prevented the reliance upon a single measure of damage; reported trends are based on agreement between the three, and specifics of each test can be found in the referenced tables. For each village we calculated the number of calendar months per year during which damage was reported, and compared this between villages bordering and not bordering a reserve.
Results
General characteristics of crop damage reports
The dataset includes 3,380 reports and 660 incidents, accounting for damage to c. 9,490 acres. Twenty-two crop types were damaged, primarily cassava, maize, millet, mixed crops and rice. Reports were submitted from 42 villages, 16 in Bunda District and 26 in Serengeti District. Half of the reporting villages, together comprising 46% of the total area of reporting villages, border a protected area. The majority of reports (80%), incidents (85%) and area damaged (85%) occurred in villages along a protected area border.
Crop damage by elephants increased significantly in both occurrence and extent during the study period: damage was far more common in 2014 (n = 2,404 reports, 458 incidents) than in 2012 (n = 405, 54). The spatial extent of villages reporting crop damage also increased, primarily within non-border villages. The number of villages bordering a protected area reporting crop damage remained consistent throughout the study period (n = 10, 14, 14 in 2012, 2013 and 2014, respectively), whereas many more non-border villages reported crop damage in 2014 compared to the previous 2 years (n = 1, 2, 19).
Spatial vulnerability
On an annual basis, 73–99% of crop damage reports and 81–99% of incidents were concentrated in villages bordering a protected area. Although the median area damaged per report was 2 acres in both villages bordering and not bordering protected areas, the distributions between the two groups differed significantly, but with a small effect (U = 953,650, P < 0.00001, Z = −5.8396, r = 0.1). The mean rank of area damaged per report was greater in villages bordering protected areas (1,658) than in non-border villages (1,423). Median area damaged per incident is higher in border villages (5 acres) than in non-border villages (4 acres), but the distributions between the two groups do not differ significantly (U = 28378, P = 0.1039).
The response curves for the single-variable generalized additive model indicate that crop damage is most likely in villages proximate to a reserve, and that beyond 30 km this risk declines sharply (P < 0.001). The relationship between the risk of crop damage and the length of the village border coinciding with a protected area is positive and linear (P < 0.001), and the relationship between damage risk and mean distance to river is markedly negative (P = 0.002). As total village area increases, risk increases in an exponential fashion (P < 0.001). Mean distance to road (P = 0.02), proportion of area converted for agriculture (P = 0.01) and proportion of built footprint (P = 0.26), all indicators of human presence, demonstrate an apparently quadratic relationship with damage peaks at intermediate levels of disturbance (where distance from roads is c. 5 km, proportion converted to agriculture is 0.6, and proportion of built footprint is 0.15; Fig. 2).

Fig. 2 Response curves for (a) all single variable models, (b) the best explanatory model of elephant crop damage as relating to human disturbance, and (c) the best predictive model considering all variables. Shaded areas represent the 95% CI.
We identified two top performing candidate models of human disturbance (Table 2). After further assessment to evaluate the influence of dropping variables on model performance, the best performing model explained 33.7% of total deviance and was able to reliably differentiate between villages where conflict was present and absent (area under curve = 0.87; Table 3). Mean distance to road (P < 0.0001) and distance to protected area (P < 0.0001) were significantly related to the probability of crop damage by elephants. The response curve for distance from a reserve is maintained from the single variable model, whereas damage risk increased in a linear fashion with mean distance from road (Fig. 2). Monte Carlo simulations of Moran's I indicated that model residuals were not spatially autocorrelated (P = 0.8396).
Table 2 Resulting output from all model combinations of crop damage. A maximum of three covariates were considered in combination because of statistical limitations. The best performing models of human disturbance (*) and predictive model (**), evaluated by deviance explained and Akaike information criterion (AIC), were selected for further assessment.

Table 3 Top performing candidate models of crop damage as relating to human disturbance and predictions of villages at highest risk. The influence of dropping variables on model performance was assessed (1–9). The final, best performing models evaluated by deviance explained and AIC are denoted with *.

The best-fit predictive model explained 51.4% of model deviance and evaluation metrics indicate good predictive performance (area under curve = 0.92; Table 2). Dropping variables did not improve model performance (Table 3). Village total area (P = 0.002) and length of shared boundary with a protected area (P = 0.003) were significantly related to whether a village experienced damage by elephants. Mean distance to river (P = 0.37) was not a significant factor but did improve model performance (10.4% improvement in deviance explained, 13.95 reduction in AIC; Table 3). The response curve for length of shared boundary with a protected area is consistent with that from the single variable model (Fig. 2). The relationship between total village area and risk remains positive, although the rate of increase in risk at larger areas is reduced in comparison to the single variable model. Risk has a negative relationship with mean distance to river but only beyond 25 km. Model residuals were not spatially autocorrelated (P = 0.7946). The mapped probabilities indicate that damage is most likely to occur in villages along a protected area boundary, but that even along the boundary the probability of damage is highly variable ($\bar{x}$ = 0.74, range = 0.013–1.0; Fig. 3).

Fig. 3 Predicted probability of crop damage by elephants at the village level. Highest-risk villages are adjacent to protected areas but moderate risk areas extend > 30 km from the reserves. High-risk villages far removed from protected areas tend to be proximate to rivers.
Several villages far removed from the protected areas (> 15 km) were predicted to be at high risk of crop damage. Visual assessments indicate that these high-risk villages tend to be located in closer proximity to riverine corridors and exhibit intermediate levels of human disturbance compared to low-risk villages that were positioned at similar distances from protected areas, but had lower or higher levels of human disturbance (Fig. 3).
Seasonal vulnerability
Elephants damage crops most frequently at the end of the rainy season and throughout the dry season. Damage during May–July accounts for the majority of reports (72%), area damaged (72%) and incidents (66%). In total, damage during these months is more than expected across all measures (Table 4). Variation between years is notable: concentration of damage during these three months ranged from 31 to 75% of reports and 36 to 79% of incidents during the study timeframe. Damage is most spatially extensive during these months; the greatest total number of villages experience damage during May–July (Table 4). A greater number of border villages experience damage than non-border villages during all months except October. Border villages are subject to damage throughout the year, whereas damage in non-border villages is primarily restricted to May–July and December. Villages bordering a protected area reported damage during 4 calendar months on average, whereas non-border villages reported damage during 1 calendar month on average.
Table 4 Assessments of the distribution of damage by month. The first three columns are results of χ 2 goodness-of-fit tests evaluating whether the observed frequencies of area damaged, reports, and incidents recorded each month depart significantly from the expectation that damage was distributed equally across months, weighted by the number of days in the month. Values are adjusted residuals; significant positive departures from expectation are marked with * and represent months where significantly more damage was observed than expected. Effect size was calculated using the contingency coefficient, C. The remaining columns are the number of villages reporting conflict during each month by those that border a reserve, do not border a reserve, and in total.

Discussion
A 60% reduction in Tanzania's elephant population during 2009–2015, driven by poaching, highlights the conservation significance of locally increasing populations in the western Serengeti (Kideghesho, Reference Kideghesho2016). At the same time, local conservation objectives compete with dominant forms of land use adjacent to the protected areas, and communities are burdened with the costs of living in close proximity to wildlife. Agricultural damage by elephants is the most common impact, increasing in severity and extent, and is a problem at the landscape level, rather than a localized issue. Nearly three-quarters of villages in Bunda and Serengeti districts that border a protected area reported damage, which occurred along the entire length of the boundary. Damage has also become increasingly common in villages farther removed from the protected areas.
Although technical approaches to prevent and mitigate damage could be applied at the landscape level, in most areas where crop damage by elephants is a serious issue, limited resource availability restricts large-scale implementation of interventions. The analysis presented here illustrates that risk is not equally distributed across time and space. An understanding of the dynamics of elephant crop damage can be used to optimize the geographical and temporal focus of selected preventative and mitigation efforts. Focusing prevention and mitigation efforts in villages directly adjacent to a protected area would capture 80–85% of crop losses. The concentration of damage within border villages is consistent across all years, and border-focused strategies are expected to support an efficient use of resources in the long term. Other studies also illustrate that crop damage by elephants primarily occurs near protected areas (Naughton-Treves, Reference Naughton-Treves1998; Monney et al., Reference Monney, Dakwa and Wiafe2010; Mackenzie & Ahabyona, Reference Mackenzie and Ahabyona2012; Vedeld et al., Reference Vedeld, Jumane, Wapalila and Songorwa2012).
In cases where implementing interventions along an entire reserve boundary is not feasible because of restricted resources, knowledge of variability in risk along a reserve edge can be used to direct resources towards the highest-risk villages, as indicated by the mapped prediction. Our predictive model indicates that at the village level, village total area and length of shared boundary with a reserve are two of the most important factors that determine risk. Factors related to key habitat features, such as proximity to rivers, were also important and were particularly notable in villages further removed from the protected areas. Understanding the relationship between village geometry and risk does not pinpoint the underlying drivers of crop damage by elephants. However, it does indicate that prioritizing interventions within the largest villages that share long stretches of their boundary with a protected area is a sensible starting point in cases where decision makers lack historical or environmental data to produce local risk predictions. This approach can then be further refined by local knowledge of important habitat features within villages.
Damage tends to be concentrated during particular months in the late rainy season and early dry season (May–July), coinciding with periods of peak crop production and harvest. Preventative efforts during these months would capture nearly three-quarters of reports. Other research has also demonstrated temporal vulnerability, particularly during times of crop ripening (Chiyo et al., Reference Chiyo, Cochrane, Naughton and Basuta2005; Jackson et al., Reference Jackson, Mosojane, Ferreira and van Aarde2008; King et al., Reference King, Lawrence, Douglas-Hamilton and Vollrath2009; Monney et al., Reference Monney, Dakwa and Wiafe2010). However, although the timing of peak crop damage is consistent from year to year, the degree to which it peaks in this period is highly variable, and probably driven by environmental variables not captured here (rainfall, crop and forage availability). If reducing the occurrence of crop damage and its economic impact is the sole priority, allocating preventative efforts within villages bordering a protected area during May–July is expected to capture 56–61% of incidents where elephants leave the protected areas, enter settlements and damage crops.
At the village level, prohibiting agricultural activities in villages that fall within a specified distance to reserves is unlikely to be practical, for two reasons. Firstly, elephants can move over long distances from protected areas and refuges (Pittiglio et al., Reference Pittiglio, Skidmore, van Gils, McCall and Prins2014); we found that villages as far as 25 km away from a reserve are still at risk of crop damage. Secondly, in areas such as the western Serengeti, where households primarily rely on subsistence agriculture, it is unrealistic to implement and enforce bans on agricultural activities within significant distances of protected areas.
However, understanding how patterns of human land use influence the risk of crop damage by elephants could inform future land-use planning efforts and facilitate predictions of how alternative land-use scenarios are likely to influence damage rates (Pozo et al., Reference Pozo, Coulson, McCulloch, Stronza and Songhurst2017). We found that patterns of human disturbance influence damage probability, and that risk was highest in villages characterized by moderate levels of human impact. This was observed across three separate, uncorrelated, measures of human disturbance: proportion of landscape converted, mean distance to road, and proportion of built footprint.
This finding is further corroborated by research from Kenya, which found that the risk of crop damage by elephants decreases beyond a threshold of 20 dwellings/km2 (Graham et al., Reference Graham, Notter, Adams, Lee and Ochieng2010). The reduced risk of crop damage in villages with relatively higher rates of human impact could be a result of elephants modifying their behaviour and movements to avoid people (Galanti et al., Reference Galanti, Preatoni, Martinoli, Wauters and Tosi2006; Graham et al., Reference Graham, Douglas-Hamilton, Adams and Lee2009; Wall et al., Reference Wall, Wittemyer, Klinkenberg, LeMay and Douglas-Hamilton2013). Similarly, outside protected areas elephants utilize patches of natural habitat as daytime refuges where they can avoid people (Galanti et al., Reference Galanti, Preatoni, Martinoli, Wauters and Tosi2006; Graham et al., Reference Graham, Notter, Adams, Lee and Ochieng2010); suitable refuges may be more prevalent in villages with lower levels of human disturbance.
In conclusion, understanding the geographical and seasonal extents of crop damage is a first step in developing strategies to mitigate or prevent losses. Visualizing the spatial extent of damage and detailing temporal windows of risk can help to determine management priorities. Based on the scale of the problem and available resources, stakeholders can determine what is feasible and how interventions will be implemented in space and time, and set reasonable expectations for resulting impacts. Our work presents evidence that crop damage risk is highest in areas with moderate levels of human impact. This suggests that risk can be reduced by concentrating areas of human disturbance. Additional work is needed to further substantiate this relationship and to develop clear land-use planning guidelines.
Acknowledgements
We thank the Tanzania Wildlife Research Institute and the Tanzania Commission for Science and Technology for research permissions, the University of California, Davis for funding this work via a Henry A Jastro Research award and a Provost's Dissertation Year Fellowship, and the Grumeti Fund for logistical support and for their willingness to share this dataset.
Author contributions
Research conception: KDS and NM; data collection and maintenance: PM and BB; data analysis and writing: KDS; revisions: all authors.
Conflicts of interest
None.
Ethical standards
All authors adhered to the Oryx Code of conduct. The original dataset contained personal identifiers; we obtained clearance from the Institutional Review Board of University of California, Davis to analyse these records for research purposes, and stored the data according to the Board's established guidelines (IRB ID: 900322-1, exempt).