1. Introduction
Research on the dynamics of love and conflict in the family studies how sentiment in the relationship between two family members affects sentiment in their relationships with other family members (Widmer, Reference Widmer2016; Erel & Burman, Reference Erel and Burman1995; Krishnakumar & Buehler, Reference Krishnakumar and Buehler2000). As such, this area of study invites network scientific analysis, even if it is not typically analyzed with an eye to testing network theories or using tools from network science. Studies in this field have produced robust evidence for “spillover effects,” whereby parental conflict negatively impacts the parent–child relationship (Amato, Reference Amato1986; Amato & Booth, Reference Amato and Booth1996; Frank, Reference Frank2007; Gerard et al., Reference Gerard, Krishnakumar and Buehler2006; Kaczynski et al., Reference Kaczynski, Lindahl, Malik and Laurenceau2006; Kouros et al., Reference Kouros, Papp, Goeke-Morey and Cummings2014; Orbuch et al., Reference Orbuch, Thornton and Cancio2000; Riggio, Reference Riggio2004; Sheehan et al., Reference Sheehan, Darlington, Noller and Feeney2004), and positive feelings of love and affection between parents positively influence the parent–child relationship (Brody et al., Reference Brody, Pellegrini and Sigel1986; Gerard et al., Reference Gerard, Krishnakumar and Buehler2006; Shek, Reference Shek1998; Stroud et al., Reference Stroud, Meyers, Wilson and Durbin2015). However, most research does not consider more than two dyadic relationships at once and has therefore been criticized for assuming an “unnecessary simplification of reality” (Kalmijn, Reference Kalmijn2012): Three family members have three dyadic relationships, so there is a missing link.
A few studies have analyzed the interplay of all three relationships in a family triad at once (Booth & Amato, Reference Booth and Amato1994; de Bel et al., Reference de Bel, Kalmijn and van Duijn2019; Kalmijn, Reference Kalmijn2012). Overall, the available evidence suggests that relationships between three family members tend to consist of either all-positive, harmonious relationships, or a pattern where two family members maintain a harmonious relationship with each other while both in conflict with the third. For example, Booth & Amato (Reference Booth and Amato1994) find that when marital quality is high, children who are close to one parent tend to be close to the other parent as well, while when marital quality is low, they tend to be close to one parent only. Similarly, Kalmijn (Reference Kalmijn2012) finds that divorce is associated with starker differences in the quality of relationships that children have with their mother and father. De Bel et al. (Reference de Bel, Kalmijn and van Duijn2019) find that relationships between siblings depend on whether they have similarly valanced relationships with their parents.
These relationship valence patterns are consistent with “structural balance” (de Bel et al., Reference de Bel, Kalmijn and van Duijn2019), a familiar equilibrium concept from social network analysis (Cartwright & Harary, Reference Cartwright and Harary1956; Heider, Reference Heider1946, Reference Heider1958). The theory of structural balance proposes that two individuals adjust their relationship in reaction to their relationships with a third such that exactly zero or two relationships are negative (i.e., following the proverb that “the enemy of my enemy is my friend” and “the friend of a friend is a friend”). These adjustments would be rooted in the tension, discomfort, and loyalty conflict stemming from situations with either one or three negative relationships (Heider, Reference Heider1946, Reference Heider1958). For example, in case of marital conflict, it would be difficult for children to maintain positive relations with both parents (one negative relationship in the triad), which would lead them to break with one in favor of the other (two negative relationships).
Although earlier studies have identified relationship patterns resembling structural balance between three family members, we argue that the evidence presented thus far also permits an alternative theoretical account. There are two types of competing dynamics that may produce the same relationship valence patterns found in these studies. First, individual family members may simply have negative characteristics that undermine all of their relationships, e.g., being untrustworthy, disagreeable, or having poor interpersonal skills. We refer to this as “individual-derived conflict.” Such negative individuals will tend to have relationships that are more negative compared to relationships other family members have with each other. Family triads with no negative individuals will then have three positive relationships and family triads with one negative individual, e.g., an uncommitted father, will have one positive and two negative relationships. In both cases, the end result would spuriously resemble structural balance.
Second, family members with shared characteristics such as similar personalities, shared hobbies, or matching demographics may cluster into homophilous groups with more positive relationships within than between groups. Such “group-derived conflict” will produce patterns wherein one relationship is positive, while the relationships to the third person are more negative by comparison. Again on the surface, the resulting relationship patterns would appear to correspond to structural balance.
The theory of structural balance, however, claims that balanced patterns are not induced by the characteristics of the individuals, but by triadic interdependency in the valences of their relationships (Abell, Reference Abell and Wright2015; Hummon & Doreian, Reference Hummon and Doreian2003; Newcomb, Reference Newcomb1961). A relationship turns sour because it resolves a social interactional problem of conflicting loyalties created by sentiment in two other relationships. Such a state of imbalance leads actors to adjust relationships in response to other relationships in the network until a balanced state is reached. The objective of this study is to empirically differentiate between structural balance and individual characteristics as sources of balanced patterns of family conflict. We examine whether family relationships are the result of a triadic balancing process rather than merely the spurious by-product of nodal characteristics.
As we will argue, by observing relationship change within families over time, we can assess the role of structural balance in isolation from the influence of individual attributes. Comparisons of the same family across multiple waves can identify tendencies toward structural balance net of time-constant individual- or group-derived conflict. Longitudinal approaches are common in the investigation of structural balance theory in other areas, such as urban communities (Rawlings & Friedkin, Reference Rawlings and Friedkin2017), children in classrooms (Rambaran et al., Reference Rambaran, Dijkstra, Munniksma and Cillessen2015), or governments and states (Doreian & Mrvar, Reference Doreian and Mrvar2015). Previous research on structural balance in families, however, has been limited to cross-sectional work, meaning that generative processes were confounded. This study’s longitudinal assessment of structural balance theory in the context of family relations thus provides a stronger test than has been possible thus far.
The analysis draws on the German large-scale multiactor longitudinal study pairfam—“Panel of Intimate Relationships in Families” (Huinink et al., Reference Huinink, Brüderl, Nauck, Walper, Castiglioni and Feldhaus2011), which is currently Europe’s most encompassing panel study with an emphasis on family relationships. Since 2008, it has been following a sample of 12,402 primary respondents and their family members on a yearly basis. Because of pairfam’s multiactor design and frequent yearly data collection, we can construct longitudinal records of family relationship networks with reports from all actors of interests. We focus on parents with young children living under one roof so that (a) relationships between all family members are strong and articulated and that (b) conflict-ridden relationships cannot be dealt with through avoidance. Both are scope conditions of structural balance theory (Hummon & Doreian, Reference Hummon and Doreian2003).
2. Theory
Structural balance theory (Cartwright & Harary, Reference Cartwright and Harary1956; Heider, Reference Heider1946, Reference Heider1958) is a general theory about the dynamics of relationship sentiment in a network (Abell, Reference Abell and Wright2015). It specifies how a set of relationships between three actors (hereafter a “triad”) is configured. Structural balance theory assumes that balanced triads, which are argued to be more stable and thus occur more frequently, may take on two forms: Either all relationships in a triad are harmonious (positive) or two relationships are conflictual (negative) with only the third relationship positive. These assumptions capture the intuitive propositions that (1) a friend of a friend is a friend, (2) an enemy of a friend is an enemy, (3) a friend of an enemy is an enemy, and (4) an enemy of an enemy is a friend. Triad A in Fig. 1 illustrates the first proposition, and Triad B captures propositions 2–4.

Figure 1. Balanced and unbalanced triads.
Unbalanced triads, on the other hand, violate propositions of structural balance and create cognitive tension and psychological dilemma for individuals involved in the triad (Newcomb, Reference Newcomb1961, Reference Newcomb1981). Since individuals try to avoid cognitive tension, unbalanced triads are less likely to be observed. For example, in triad C in Fig. 1, Actor 3 has two “friends” (Actor 1 and Actor 2) who are mutual enemies. For Actor 3, this violates the “an enemy of a friend is an enemy” proposition. This results in psychological dilemma in the form of a loyalty conflict, where Actor 3 tries to maintain a positive relationship with two others in enmity. Such a dilemma will eventually induce a change, e.g., pressure this actor into siding with either of the two conflicting others, which will return the triad to a balanced state.
Research on structural balance has generated mixed evidence. Relationships in urban communities (Rawlings & Friedkin, Reference Rawlings and Friedkin2017), school classes (Hallinan & Hutchins, Reference Hallinan and Hutchins1980; Rambaran et al., Reference Rambaran, Dijkstra, Munniksma and Cillessen2015), social media communities (Leskovec et al., Reference Leskovec, Huttenlocher and Kleinberg2010), or multiplayer gaming platforms (Szell et al., Reference Szell, Lambiotte and Thurner2010) have been found to behave mostly in accordance with structural balance. Evidence is weakest with regard to triad D, as there is often no clear tendency for it to resolve into triad B or C with time (Davis, Reference Davis1975; Rambaran et al., Reference Rambaran, Dijkstra, Munniksma and Cillessen2015; Leskovec et al., Reference Leskovec, Huttenlocher and Kleinberg2010; Szell et al., Reference Szell, Lambiotte and Thurner2010).Footnote 1 Doreian & Krackhardt (Reference Doreian and Krackhardt2001) find support for only some balance-theoretic tendencies in fraternity friendships. In the context of conflict in international relations balance theory has found some support (Antal et al., Reference Antal, Krapivsky and Redner2006; Healy & Stein, Reference Healy and Stein1973; Moore, Reference Moore1979), but Doreian & Mrvar (Reference Doreian and Mrvar2015) find no systematic temporal increase in structural balance in the most comprehensive analysis.
It is important to distinguish different forms of evidence. In general, static evidence for balance is confirmatory: Triads of types A and B tend to occur more frequently than random, and triads of type C and, also but less consistently so, D occur less than random (Davis, Reference Davis1967; Leskovec et al., Reference Leskovec, Huttenlocher and Kleinberg2010; Szell et al., Reference Szell, Lambiotte and Thurner2010). We problematize this static form of evidence in this paper in the context of family networks. Longitudinal evidence is less conclusive. There are two general forms of longitudinal evidence: Triad-level changes that resolve balance-theoretic tensions. Studies do not consistently find such predicted dynamic tendencies (Hallinan & Hutchins, Reference Hallinan and Hutchins1980; Leskovec et al., Reference Leskovec, Huttenlocher and Kleinberg2010; Szell et al., Reference Szell, Lambiotte and Thurner2010). Evidence is also conflicting on a second form of longitudinal evidence: System-level frequencies of violations of structural balance, which often do not go down with time (Doreian & Krackhardt, Reference Doreian and Krackhardt2001; Doreian & Mrvar, Reference Doreian and Mrvar2015; Szell et al., Reference Szell, Lambiotte and Thurner2010). However, the general failure for imbalances to gradually disappear may be reconciled with theory as a floor effect: Time-constant levels of balance violations would also obtain as a noisy equilibrium state of a system in which random tie valance changes happen at some rate combined with a tendency for any imbalances they generate to be resolved with time.
Perhaps of all contexts, structural balance is most strongly predicted to emerge in families, because relationships among cohabitating family members most clearly satisfy the scope conditions of the theory (Hummon & Doreian Reference Hummon and Doreian2003): Relationships between each of the individuals are strong and articulated and living under one roof means cognitive imbalances stemming from conflict-ridden relationships cannot simply be handled through avoidance. Early contributions from family therapy research have provided examples of how structural balance can be used in a therapeutic context (Cohen & Corwin, Reference Cohen and Corwin1975, Reference Cohen and Corwin1978; Levang, Reference Levang1989). While structural balance theory treats relationships as either positive or negative, the more recent empirical work discussed earlier adapts binary propositions of structural balance to a continuous framework and suggests that tendencies towards balanced triads in families exist. De Bel et al. (Reference de Bel, Kalmijn and van Duijn2019) conclude that better relationships of two adult siblings with a parent strengthen the sibling–sibling relationship. Similarly, more harmonious interparental relationships have been found to enhance the parent–child relationship for both parents (Booth & Amato, Reference Booth and Amato1994). Both findings speak for tendencies towards balanced all-positive triads (Fig. 1, panel A). Tendencies toward triads with one positive and two negative relationships (panel B) may be found in high-conflict marriages, where a child’s relationship with one parent improves based on how much the relationship with the other worsens (ibid.). In the same vein, divorce has been found to be associated with an increased difference in the relationship quality that adult children have with one parent versus the other (Kalmijn, Reference Kalmijn2012) again consistent with structural balance. And for adult siblings, the combination of a bad parental relationship of one sibling and a good parental relationship of the other sibling has been found associated with a more negative sibling–sibling bond (de Bel et al., Reference de Bel, Kalmijn and van Duijn2019).
2.1. Structural balance and personal characteristics
Structural balance theory argues that balancing tendencies are the consequence of a network effect: The nature of ties with a third actor has a direct influence on the nature of a tie between two members. Put differently, it is assumed that actors adapt relationships in accordance with those around them to achieve balance in the triads they are nested in (Hummon & Doreian, Reference Hummon and Doreian2003). However, not all triads tending toward balance are necessarily a product of such network effects. Personal characteristics, which can generally be expected to leave a great impact on the relationships individuals maintain with each other (Zheleva et al., Reference Zheleva, Getoor, Golbeck and Kuter2008), provide an alternative explanation as to why balanced patterns can be found. For the case of nuclear families, two mechanisms are highlighted here through which personal characteristics may produce sentiment patterns that are also compatible with structural balance theory.
The first mechanism pertains to a situation of “individual-derived conflict” in which family members differ in the quality of the relationships they are able to maintain. An example is a family in which one family member has a difficult personality, is disagreeable, or has a lasting mental or physical health problems (Henderson et al., Reference Henderson, Sayger and Horne2003; Kouros et al., Reference Kouros, Papp, Goeke-Morey and Cummings2014). If relationships tend to be positive when both individuals involved are of agreeable nature, then the condition of one disagreeable individual would result in two strained relationships and one comparatively harmonious relationship in any family triad. Namely, the difficult person maintains negative relationships with the two positive family members, who among them maintain a positive relationship. Hence, the triad resembles a balanced one positive, two negative state. Fig. 2 illustrates this distinction between structural balance and individual-derived conflict as a source of balanced relationship sentiment. With structural balance it is the influence of the two other relationships that induces a sentiment change, while with individual-derived conflict it is simply the negativity of the node that induces the negativity of the adjacent edges. In the absence of “negative” individuals, triads would be expected to be all-positive, again consistent with balance theory. Only with two or more negative individuals would an imbalanced, all-negative triad emerge.
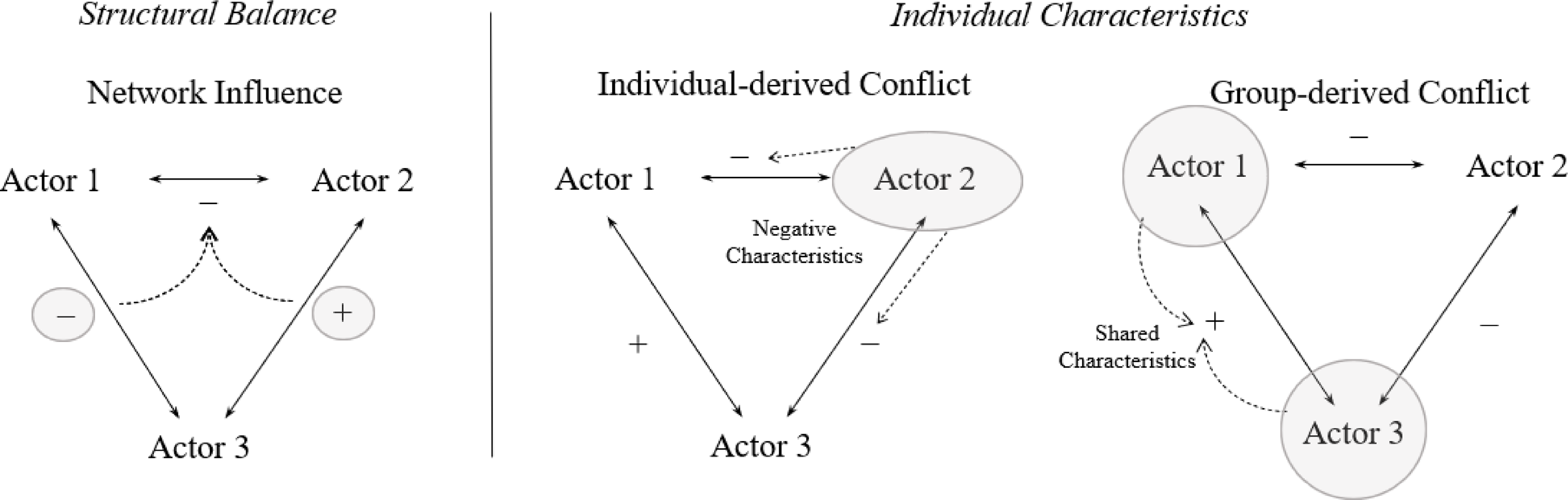
Figure 2. Structural balance, individual- and group-derived conflict.
The second mechanism involves two family members sharing some trait that they do not share with the third, which we refer to as “group-derived conflict”. Groups in families can arise on the basis of different preferences and interests between adult parents versus those of a teenage child (Collins & Laursen, Reference Collins and Laursen2006; Steinberg, Reference Steinberg2001). The general tendency for individuals with similar characteristics to form stronger and more positive bonds is referred to as “homophily” in the network-theoretical literature (McPherson et al., Reference McPherson, Smith-Lovin and Cook2001). For example, two parents may bond over preferences such as going to sleep early, while their teenage child prefers loud music at night. Groups may emerge over similar personality traits of two actors (Selfhout et al., Reference Selfhout, Burk, Branje, Denissen, Van Aken and Meeus2010), or shared demographic attributes such as gender (i.e., sons bonding with fathers or mothers with daughters; McHale, Reference McHale1995). In a triad, homophily on some characteristic always produces a spurious tendency toward balance, regardless of the distribution of that characteristic over the three individuals involved. Fig. 2 also illustrates this distinction between structural balance and group-derived conflict as a sources of balanced relationship sentiment.
Both “individual-derived conflict” and “group-derived conflict” produce family triads that resemble balanced patterns where either all relationships are equally positive or two relationships are more negative than the third. However, such patterns are then not a product of the network influence process assumed by balance theory, but instead spuriously produced by combinations of personal characteristics. Previous triadic studies (Booth & Amato, Reference Booth and Amato1994; de Bel et al., Reference de Bel, Kalmijn and van Duijn2019; Kalmijn, Reference Kalmijn2012) permit this alternative theoretical possibility, as the data used in these studies were cross-sectional. Sets of relationships were compared across families. This study suggests a new approach and examines three-way relationships in family over time, within families. To the extent that personal characteristics are time constant, their influence can be netted out through over-time comparisons. Hence, sentiment in family relationships evolving over time can disentangle network influence from time-constant personal attributes as generative processes. A within-family comparison of relationships over time can identify network changes consistent with structural balance that cannot be alternatively explained as resulting from fixed personal characteristics.
2.2. Congruence as a measure of balance
The structural balance principle implies that sentiment in a focal relationship is positively impacted by congruence in the relationship sentiments in the two other relationships. Congruence is defined as the product of two relationships with signed quantities. Structural balance theory is stated in terms of binary relationship sentiment, either positive or negative. We follow Booth & Amato (Reference Booth and Amato1994), Kalmijn (Reference Kalmijn2012), and de Bel et al. (Reference de Bel, Kalmijn and van Duijn2019) in conceptualizing relationship sentiment in family relations as continuous rather than binary. Down the road this prevents arbitrary dichotomization of nonbinary measures of relationship sentiment (see data section). A continuous approach accommodates nuanced changes in relationship sentiment that would not generate a sign change in a binary approach.Footnote 2 Identical to the works cited above, we can thus state structural balance hypotheses in terms of an interaction effect between two relationships on sentiment in the third. Balancing tendencies can be captured as a positive expectation on a single coefficient, the coefficient for the interaction effect. Congruence in the relationships with a third family member should be positively associated with sentiment in the relationship between two focal members.
H1: Dyadic relationship sentiment is positively associated with relationship sentiment congruence in the triad.
Fig. 3 depicts the hypothesized dependence of a focal dyad on the other two dyads in a triad, as illustrated by the dashed arrows pointing to the dyad between actors 1 and 2. In left and middle triad, we observe congruent relationships (both positive or both negative relationships), which is captured by a positive interaction (mathematically + * + = +, and – * – = +), hence a positive expectation. In the right triad, we observe incongruent relationships, which is captured by a negative interaction (+ * – = –), hence a negative expectation.

Figure 3. Sentiment in one relationship follows sentiment congruence in the two other relationships.
As we have argued, in addition to structural balance, individual- and group-derived conflict will also produce relationship sentiment patterns consistent with H1. However, to the extent that individual- and group-derived conflict are time constant and fixed, these alternative mechanisms will produce patterns associated with structural balance in (between-effects) comparisons between families, but less so in (fixed-effects) comparisons between the state-of-affairs in one family at one point with the state of affairs of that same family some time later.Footnote 3 In the next sections, we detail how we leverage longitudinal data to disentangle structural balance and confounding conflict-generating mechanisms.
H2: The association in H1 is confounded by fixed individual traits.
3. Data
We exploit data up until and including the 10th release of the German Panel of Intimate Relationships in Families (pairfam) (Huinink et al., Reference Huinink, Brüderl, Nauck, Walper, Castiglioni and Feldhaus2011; https://www.pairfam.de/). Pairfam is a large-scale multiactor longitudinal dataset consisting of 10 yearly waves. Since 2008, it has been following a sample of 12,402 primary respondents (hereafter “anchor respondents”). The initial response rate among anchor respondents was 37%. Anchor respondents were sampled from 343 randomly selected German municipalities and are recruited in similar numbers from three birth cohorts: 1971–1973, 1981–1983, and 1991–1993. Thus, respondents do not mirror the general German population but are representative along the birth cohorts that were sampled (Brüderl et al., Reference Brüderl, Schmiedeberg, Castiglioni, Becker, Buhr, Fuß and Schumann2019). In wave 10, 4102 of the initial anchor respondents participated and the average yearly attrition rate among anchors was 11.3%. For detailed information on sample demographics, attrition rates, and potential attrition bias see Müller & Castiglioni (Reference Müller and Castiglioni2015). From wave 2 and onwards, partner and children between 6 and 18 years of age living in one household with the anchor are sampled as well, providing detailed information about the relationship they have with each other. With the exception of child–child relationships, which were not included in the surveys, this allows for constructing otherwise complete family networks with reports from all actors of interest, cross-sectionally and longitudinally.
The analytical sample is derived as follows: First, at any given wave in pairfam, we selected families in which a heterosexual couple (i.e., anchor and their partner) and at least one child between 6 and 18 years had been living together in a household for at least one year (2195 distinct nuclear families with 30,071 observations). Families with fewer than three members were excluded since this study is interested in interdependent relationships between at least three individuals. Three families with same-sex parents were excluded from the analysis reported in the main text as they do not fit our models specifying effects specific to child–mother, father–child, and mother–father dyads, but main results are robust to their inclusion. Second, at any given wave, at least two members of each family needed to be interviewed in order to have information on all three relationships in a triad. 2194 families with 30,015 total observations meet this condition. Third, only those families are selected in which any two respondents were present for an interview in at least two waves (1718 families, 27,987 observations). This is done in order to enable assessment of relationship change across time while ensuring an identical sample for both cross-sectional and panel analyses. Participation in at least two waves did not have to be consecutive for families to be included. This is standard practice in panel (fixed effects) analyses because unlike lagged effects regressions, they do not assume a specific temporal ordering or interval. Fourth, in families where more than one child participated in the interviews, either across time or within one wave, a randomly selected child was chosen for the sample and the others excluded. This is the case for 38.5% of the selected families. It results in a loss of total observations (7851 or 28.1 % of all observations) but is necessary to ensure a single, independent triadic father–mother–child structure for all families in the data analysis. Our main results remain unaltered in additional analyses not shown here where rather than randomly selecting one child per family we include all children, naïvely treating the triads involving each child as evolving independently from the others. Lastly, families in which information is unavailable that is used for control variables in the regression analyses—household size, number of children in household; age, gender, kinship status of any of the selected family members—are excluded from the sample (8 families, 30 observations). The final analytical sample encompasses N = 1710 distinct family triads (covering 78 % of eligible families) with information from the anchor, their partner and one child with an average participation in ∼ 3.9 waves, totaling 6692 family-wave units and 20,076 observations. Table 1 summarizes descriptive statistics of the analytical sample.
Table 1. Sample information

4. Measurement
As said, we follow Booth & Amato (Reference Booth and Amato1994), Kalmijn (Reference Kalmijn2012), and de Bel et al. (Reference de Bel, Kalmijn and van Duijn2019) in operationalizing relationship sentiment in family relations as continuous rather than binary. Relationship sentiment is constructed using a latent factor score based on 5 items about recognition, trust and conflict from the two reporting family members each.Footnote 4 In other words, dyadic relationship sentiment at any timepoint (wave) t consists of 10 items in total, 5 items where family member i reports on their relationship with family member j, and vice versa. Note the third member of the triad, not part of the dyad, is referred to as k. We converted directed relationship indicators into a single undirected factor for three reasons. First, while there exist directional formulations of balance theory, our current and past theoretical treatments in family studies have been undirected. Second, since relationship reports may include individual-level measurement error, combining two-actor measurements presents an opportunity to reduce measurement error (Mandemakers & Dykstra, Reference Mandemakers and Dykstra2008). Third, some relationship items were formulated in an undirected manner (“you are annoyed with each other”) or asked about perceived behavior from the other person (“the other person tells you what he/she is thinking”), forcing us to treat the overall factor structure as undirected. Additional analyses reported in the Appendix, Section A.1. further show that substantial disagreement between two family members regarding their relationship was rare, supporting Mandemakers & Dykstra’s conjecture that discrepancies between reports of both actors are mostly due to noise. Aspects of different relationship dimensions are combined into a latent factor because they will better capture an encompassing underlying concept of relationship sentiment. Treating dimensions separately instead would imply that they exist separately from each other, which would be an unrealistic assumption to make. Considering the complex and multifaceted nature of family relationships, combining dimensions into a latent factor is a simplifying but necessary step to test structural balance theory, which operates with a universal concept of relationship valence. Items are chosen because they are identical for adult-adult and adult-child dyads, which was not the case for other relationship indicators in the data. For robustness, analyses were also conducted on a dichotomous sentiment measure using the grand mean of the latent factor as a threshold, obtaining comparable main results (Appendix A.4).
All items are measured with five-point likert scales referring to “How often do the following things happen?” (never, seldom, sometimes, often, very often). Recognition encompasses the item “You show recognition for the things your [family member] does.” Trust is captured by two items: “Your [family member] tells you what he/she is thinking” and “Your [family member] shares his/her intimate feelings and thoughts with you.”. Conflict is expressed in the items “You and your [family member] are annoyed or angry with each other” and “You and your [family member] disagree and quarrel.”
Data are structured in such a way that for any wave t in which a family participated, each dyad ij,t in the family forms one unit of observation. Summary statistics of the raw items are displayed in Appendix A.1. For the confirmatory factor analysis, data were pooled across dyads and waves. The factor structure of relationship sentiment measures of member i and member j fits well (Chi2 = 805.6 ; df = 21; p < 0.001; RMSEA = 0.043 ; CFI = 0.98 ; TLI = 0.96) and has good reliability (Cronbach’s alpha = 0.78). Alternative specifications in which the measurement component were allowed to vary across dyad types resulted in worse fit, leading us to choose the above CFA structure where no underlying grouping was assumed (see Appendix A.1). Additional analyses reported under Appendix A.1, Table A6 suggest that results are robust to alternative specifications with free intercepts and loadings across dyad types (configural invariance). Missing values in the relationship reports are imputed by using robust maximum likelihood estimation (MLR) in the factor analysis. 30.0% (6616 dyads) of the 20,076 dyads had missing values for either family member i or j because one member out of three did not participate for an interview in that wave. Another 355 dyads (1.8%) were missing at least one relationship item due to nonresponse or invalid answers. Detailed statistical information on the factor construction is provided under Appendix A.1.
4.1. Dependent variable
The dependent variable is the dyadic relationship sentiment between family members i and j at wave t (hereafter relationship sentiment ij,t ), see Table 2 for summary statistics.
Table 2. Relationship sentiment by type of dyadic relationship
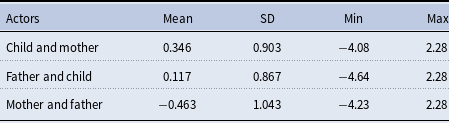
Note: Statistics are based on pooled waves.
4.2. Independent variables
Congruence in relationship sentiment of family members i and j with a third family member k at wave t (hereafter: “congruence t ”) is expressed as the product (interaction) of relationship sentiment ik,t and relationship sentiment jk,t . Again, relationship sentiment is centered around the mean so that sentiments have a value of either below or above zero. The interaction of two relationship sentiments thus has increasingly positive values when relationship sentiment ik,t and relationship sentiment jk,t both have increasingly negative, or both increasingly positive values.
4.3. Control variables
All analyses include main predictors for relationship sentiment ik,t and relationship sentiment jk,t . This is done because first, direct influences of dyads ik and jk on the dependent dyad ij are controlled for. Second, adding main effects to a model including an interaction term based on centered variables makes the interaction coefficient independent from the level at which the centering was undertaken (Aiken et al., Reference Aiken, West and Reno1991). Therefore, the effect of the independent variable is robust against the definition of the “neutral” zero point on the relationship sentiment continuum. This robustness is essential because all sentiments are based on factor scores where the scale is abstract. For summary statistics of dyadic relationship sentiment, see Table 2.Footnote 5
Additionally, variables with basic characteristics of family members i and j are added. An overview of this information by type of family member is presented in Table 1. We include terms for gender, age, age squared, and whether the family member is a biological parent to the child in the family triad subject to analysis. Demographic characteristics and parenthood status are expected to influence both dyads a family member is nested in, which in turn may produce spuriously balanced patterns. We control for a limited number of demographic traits that may produce spurious balance because first, it provides a starting point similar to previous cross-sectional studies—which also included demographic variables while still finding patterns suggesting balance. Second, by taking out some of the more obvious potential confounding traits a priori, we can assess effects of those traits that are more hidden and harder to measure.
At the family level, binary variables for whether additional adults or additional children are present in the household are included. This is done to account for the possibility that relationships in larger families are different than in single-child families. Notably, these variables cannot fully control for triadic influence resulting from additional family members in the household. For this reason, Appendix A.4 presents supplementary analyses in which influence from additional family members is absent because we restricted the sample to single-child, three-person families. Results are similar to those in the main text. Main respondent status is added to control for possible influence resulting from survey structure and design. Lastly, a categorical variable wave is included to control for time effects.
5. Analytical strategy
We use a random effects and a between-within regression model (also known as a hybrid model) to test our hypotheses. The random effects model combines both inter- and intradyadic comparisons, which has the advantage that it maximizes statistical efficiency for a test of H1 (Snijders & Bosker, Reference Snijders and Bosker2011). The disadvantage is that its estimators do not distinguish these components, which would be necessary if the interdyadic (cross-sectional) component additionally included influence from fixed individual traits, as this would then become a spurious component of the structural balance effect estimation in the random effects model.
In order to establish if and if so, to what extent fixed individual traits confound structural balance, a model that distinguishes estimators into an interdyadic and an intradyadic component is needed. This is done in the between-within model. For the interdyadic part, all information is averaged per family dyad over waves and is thus time invariant. Such an approach comes closest to the cross-sectional, cross-family comparisons made in prior research. As argued in the theory, this assessment may suffer from the effects of unobservable individual traits influencing the relationship of interest and potentially confounding structural balance. In contrast to the between part of the between-within model, the within component subtracts all time-invariant averages from the independent measures. By doing so, the within component only captures change within family dyads over time, producing a longitudinal analysis of intradyadic comparisons. This allows us to assess the effect of congruence t on relationship ij,t without any confounding influence of fixed personal characteristics. A simulation study reported in Appendix A.2 shows that only the within (intradyadic) component of a between-within approach (and not the between component) can correctly identify the true structural balance effect in synthetic data.
In both models, the unit of analysis is the dyadic relationship sentiment at wave t. We pool all three types of dependent dyads (i.e., mother–child dyads, father–child dyads, mother–father dyads) in a single model so that we can test our hypotheses for all dyad types simultaneously. In order to avoid assuming equally strong effects of independent variables for mother–child, father–child, and mother–father dyads, we estimate all parameters separately for each of the three types of dyads. Observations for different dyad types are not independent within family, which is corrected for by using standard errors clustered at the family level. In both models, relationship sentiment ij,t is regressed on the key variable of congruence t , which is operationalized as the interaction term of relationship sentiment ik,t * relationship sentiment jk,t .
We test the coefficients of congruence t for mother–child, father–child, and mother–father triads together and use a multiple-parameter Wald test for the hypothesis test: If the combined congruence t terms are positive in the random effects model, H1 is supported—that is, overall greater congruence t across types of dyads predicts a better relationship ij,t . We test H2 using a multiple-parameter Wald test comparing the combined congruence parameters from the between part of the between-within model with the combined congruence t parameters from the within part. We support H2 if the congruence parameters in the between part are larger than those of the within part, as this result would show that congruence in the between part is spuriously amplified (and hence, confounded) by fixed individual traits. Test statistics are derived from two-tailed tests.
6. Results
Results from the regression models are presented in Table 3. Model 1 (left column) represents the random effects model investigating the overall effect of congruence t on relationship ij,t . The middle column outlines the between part of the mixed model (Model 2), examining interdyadic effects. The right column presenting the within part of the mixed model addresses time-varying, intradyadic change. We discuss the hypotheses in order of appearance and close the section with a consideration of the direct effects of the additional covariates, relationship sentiment ik,t , and relationship sentiment jk,t .
Table 3. Random effects model (RE) M1 and between effects (BE)—within effects (WE) model M2 for sentiment ij,t (SE clustered at the family level)
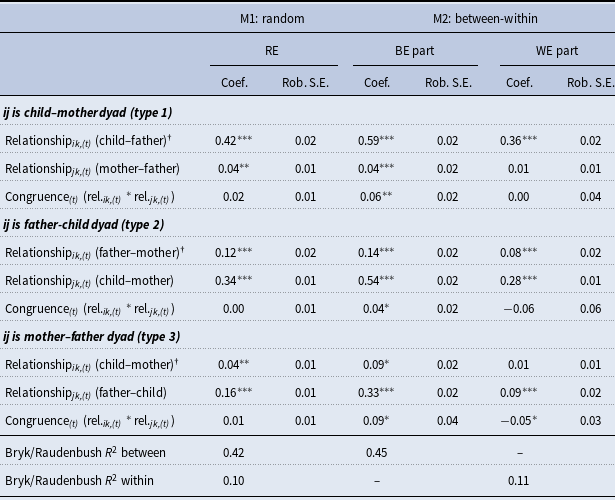
Tested two-sidedly: * p < 0.05, **<0.01, *** p < 0.001. N Observations = 20,076; N Dyads = 5,130; N Families = 1,710.
†Time-variant variables in the RE model and in the WE part of the b-w model, but not in the BE part of the b-w model (hence t in parentheses).
Note: Analyses control for the variables age, gender, biological parenthood status and anchor status of i and j; whether additional children live in the household, whether additional adults live in household, wave. See Appendix A.3.
The effect of congruence t on relationship sentiment ij,t is not significant for any of the three dyad types in the random-effects Model 1. A Wald test combining the three parameters is not significant (Chi2 = 1.67, df2020 = 1; p = 0.20), which leads us to reject H1. The effect of congruence on relationship sentiment ij is significantly positive when all three types of dyadic relationships in the between part of Model 2 are considered simultaneously (Wald χ2 = 7.78, df = 1; p = 0.01). However, the coefficient for congruence t is not significantly positive for any of the dyadic types in the within part of the mixed Model 2, and there is no significant effect when all three coefficients are considered simultaneously (Wald Chi2 = 0.97, df = 1; p = 0.33). Within a family and over time, the sentiment of no type of dyad improves with greater levels of congruence t . Instead, the sentiment of mother–father dyads worsens in greater levels of congruence t (β = - 0.05; s.e. = 0.03; p = 0.04). A Wald test finds a difference in the congruence coefficients comparing the between and within effects models for all three dyad types (χ2 = 6.26, df = 1; p = 0.01). This confirms that the effect of congruence is greater in interdyadic comparisons, which include confounding influences of fixed individual traits, than in intradyadic comparisons. H2 is supported. In order to test if this finding is robust, we conducted additional analyses in which dyadic relationship sentiment is not operationalized by a factor score but by the separate dimensions of the factor score, namely recognition, trust, and conflict (see Appendix A.4). This approach comes closest to that of previous works (de Bel et al., Reference de Bel, Kalmijn and van Duijn2019; Kalmijn, Reference Kalmijn2012). H2 is supported across all additional analyses conducted, underlining the robustness of the finding.
Fig. 4 visualizes the key contrast between the between and the within effects estimates from the between-within model. The three axes correspond to the three relationship sentiment variables in a triad. We chose to depict estimates where the child–mother dyad is the focal dyad, corresponding to the first panel (type 1) in Table 3. The ruled surfaces are projections of the three-way relationships. The left 3D figure depicts the between effects, the right figure the within effects. The plane in the left figure is tilted indicating a clear positive interaction effect (congruence) of structural balance for the between effects. The slope for the effect of mother–father (child–father) relationship sentiment increases with the relationship sentiment of the child–father (mother–child) relationship. In contrast, the plane in the right figure for the within effects is simple, with slopes mostly independent, in accordance with the absence of a congruence effect.
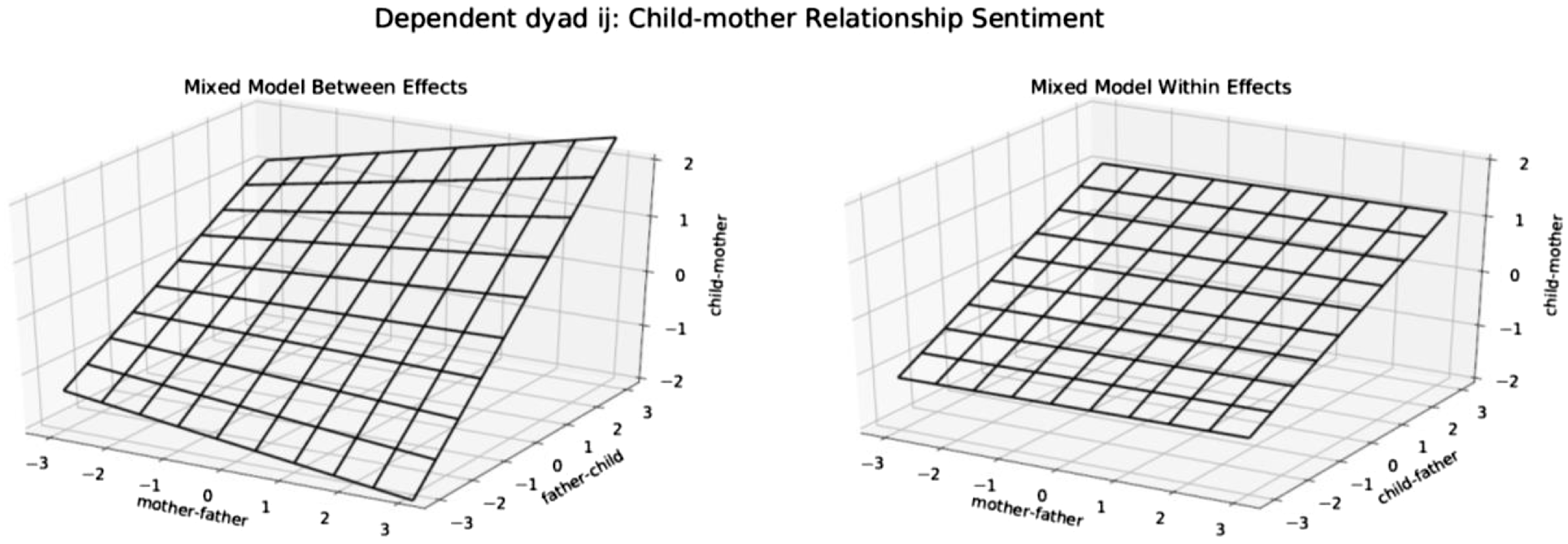
Figure 4. Sentiment of the child–mother relationship predicted by mother–father and child–father relationship sentiment.
All direct between effects of relationship sentiment (i.e., relationship ik,t and relationship jk,t ) on child–mother, father–child, and mother–father dyads are significant and positive, indicating that across dyads, relationship sentiments improve (deteriorate) when other relationship sentiments in the family triad are also getting better (worse). Interestingly, however, this finding is not universally true for all dyads when being examined on the intradyadic within level. While both dyads involving the father mutually influence one another (i.e., father–child on mother–father dyads and vice versa), as also both dyads involving the child (child–mother and father–child dyads), relationship sentiment in the mother–father dyad and mother–child dyad are independent from one another. This suggests that unlike fathers and children, mothers appear to maintain relationship sentiment independently. The relationship sentiment with their child is not impacted by their relationship sentiment with the father, nor is their relationship sentiment with the father impacted by the sentiment with their child: Conflict in the one dyad does not spill over onto the other.
7. Conclusion and discussion
The focus of this study was to evaluate the emergent claim that structural balance theory can account for triadic patterns of relationship sentiment in families. According to this theory, fault lines in family relationships would endogenously emerge through the same kinds of interactional processes thought to organize nations into axis and allies. Our main argument was that the enemy-of-a-friend-is-an-enemy patterns identified in cross-sectional data in previous studies in support of structural balance theory can emerge exogenously from fixed personal characteristics, such as when a mother and a child are close but share a strained relationship with a difficult father. Controlling for such alternative possibilities requires longitudinal data on all relationships in family triads, which we have leveraged here. In between dyadic comparisons we found static patterns suggestive of structural balance theory, replicating earlier studies. Comparing the same relationships over time, however, no balancing tendencies were found, supporting our argument of trait-induced conflicts and rejecting structural balance theory.
We did identify “spillover” (Erel & Burman, Reference Erel and Burman1995; Kalmijn, Reference Kalmijn2012; Krishnakumar & Buehler, Reference Krishnakumar and Buehler2000) or “enhancement” (de Bel et al., Reference de Bel, Kalmijn and van Duijn2019) effects found in earlier work, where a relationship between two family members is positively influenced by increasingly positive relationships with other family members. Specifically, when father–child relations improved from one survey wave to the next, father–mother relationships tended to also get better, and vice versa. When child–mother relations improved, child–father relations improved too, and vice versa. Similar to Kalmijn (Reference Kalmijn2012), mother–father sentiment was not found to spill over to mother–child sentiment, nor the other way round. This conclusion strengthens existing evidence from dyadic (Amato, Reference Amato1986; Amato & Booth, Reference Amato and Booth1996; Frank, Reference Frank2007; Riggio, Reference Riggio2004) and triadic studies (Booth & Amato, Reference Booth and Amato2001; de Bel et al., Reference de Bel, Kalmijn and van Duijn2019; Kalmijn, Reference Kalmijn2012), which found spillover effects but only when comparing relationships across families rather than over time.
Apart from leveraging the causal inference potential of longitudinal data, the empirical approach taken here distinguishes itself from previous studies in another important way. Previous studies have analyzed relationships of adult children with their parents as compared to this study, which examines relationships in families with underage children that live together in one household. The latter comes with the advantage of analyzing family networks where relationships tend to be strong and individuals cannot simply forego the kinds of psychological tensions presumed by structural balance theory through avoidance and distancing. As such, we studied a setting in which balance-theoretic pressures toward congruent relationships can in principle be expected to be stronger. Finding so little evidence supporting balance theory in this context is thus all the more striking, and problematic for the theory.
While the longitudinal nature of the study allowed us to rule out the confounding impact of time-constant traits that spuriously generate sentiment patterns consistent with structural balance theory, we cannot rule out potential effects from time-varying factors. Changes in health, career events or various other external circumstances could in principle lead to time-varying relationship dynamics that confound structural balance. Yet, no tendencies towards balance were found even in an analysis that did not control for the potentially confounding impact of time-varying factors, which strengthens our confidence that structural balance in nuclear families plays less of a role than previously thought. We cannot rule out that our result is a false negative caused by time-varying factors obscuring balance-theoretic tendencies by systematically working against balance. However, this would need to constitute a mechanism that produces a negative dependence of relationship sentiment on congruence in the other two relationships in a given triad. It would not be enough for a single relationship to turn sour with time, e.g., a general tendency for adolescent children to develop a negative relationship with precisely one parent. This would leave the interaction effect in our fixed-effects models unimpacted. Instead, a worsening relationship of the child with one parent would need to coincide with increased congruence in the relationship with the other parent and the relationship between the parents. A theoretical or intuitive underpinning for such an expectation is currently lacking.
Another important limitation of the present study is that it ignores the influence of relationships with others, most importantly siblings and grandparents, but also friends and peers. Pairfam lacks data on sentiment in sibling or grandparent relationships. Additional analyses not reported here show that results are similar when instead of selecting a random child, as we did in the main analysis, we included all child–mother–father triads in families with more than three members (violating assumptions of statistical independence between triads from the same family). It is theoretically possible that imbalance in the triads we analyzed is prevented from being resolved by the imbalance such resolution would cause in adjacent family or non-family triads. Such “jammed configurations” are consistent with structural balance at the global level even if not at the triadic level (Antal et al., Reference Antal, Krapivsky and Redner2005, Reference Antal, Krapivsky and Redner2006; Marvel et al., Reference Marvel, Strogatz and Kleinberg2009; Van de Rijt, Reference Van de Rijt2011). With the availability of more encompassing data, future research may explore structural balance logics in more extended family networks.
Acknowledgments.
We are grateful to have received helpful suggestions from two anonymous reviewers and would like to thank Ineke Maas, Jeroen Weesie, and the 2018 cohort of the Sociology and Social Science Research Master’s program at Utrecht University for constructive discussions and useful feedback. We are indebted to Florian Andersen, Ann Imke, Christiane Stein, Frida Stein, and Wolfgang Uhl for their invaluable support.
Competing interests.
None.
Funding information.
Not applicable.
Data availability.
This study uses release 10 of the Panel of Intimate Relationships in Families (Pairfam). Pairfam raw data is protected and distributed exclusively via pairfam.de upon request. Analyses were conducted using Stata16 and Mplus7.3. All code used to obtain the results for this study available at https://osf.io/b7tch/.
Appendix
Table A1. Raw items of dyadic relationship sentiment by type of Dyad
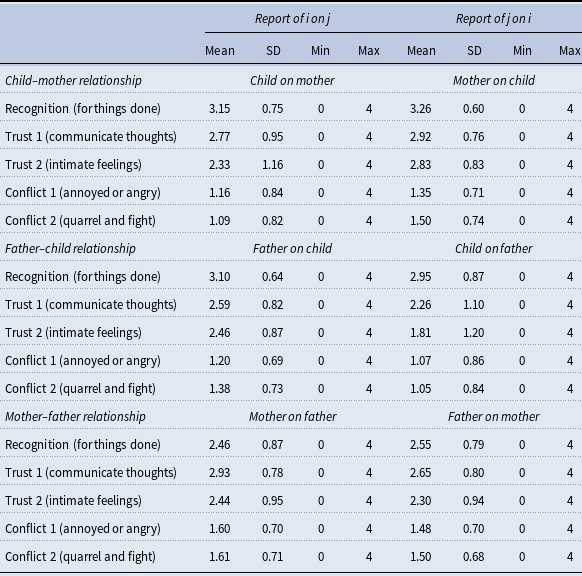
Note: Information is pooled across waves.
Table A2. Spearman’s rank correlations for raw items

Note: Highlighted cells represent correlations between items of actor i and actor j on the same dimension.
A.1. Factor construction
The confirmatory factor analysis for the latent construct “dyadic relationship sentiment” uses 5 items on recognition, trust and conflict from actor i and actor j each. Data are structured in such a way that for any wave in which a family participated, each dyad ij in the family forms one unit of observation. Table A1 outlines this data structure and presents summary statistics of the raw items by type of dyad. Information is pooled across waves and families. Table A2 shows correlations between items. Table A3 provides information on the frequency of disagreement between i and j for each relationship item, showing that substantial discrepancies in reports of i and j were rare. In the confirmatory factor analysis structure, some of the items are covaried to account for correlated values between items as outlined in Fig. A1. Missing data in the relationship sentiment items is handled by using robust maximum-likelihood estimation (MLR), which applies full information maximum likelihood (FIML). The measurement component of the model is summarized in Table A4. Descriptive statistics of the factor scores by type of dyad are outlined in Table A5. The confirmatory factor analysis was performed with MPlus7.3. After factor construction, scores were exported, standardized, and centered and used as variables in subsequent regression analyses executed with Stata16.
Table A3. Discrepancies in reports of actor i and actor j by raw item
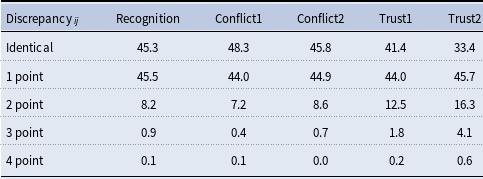
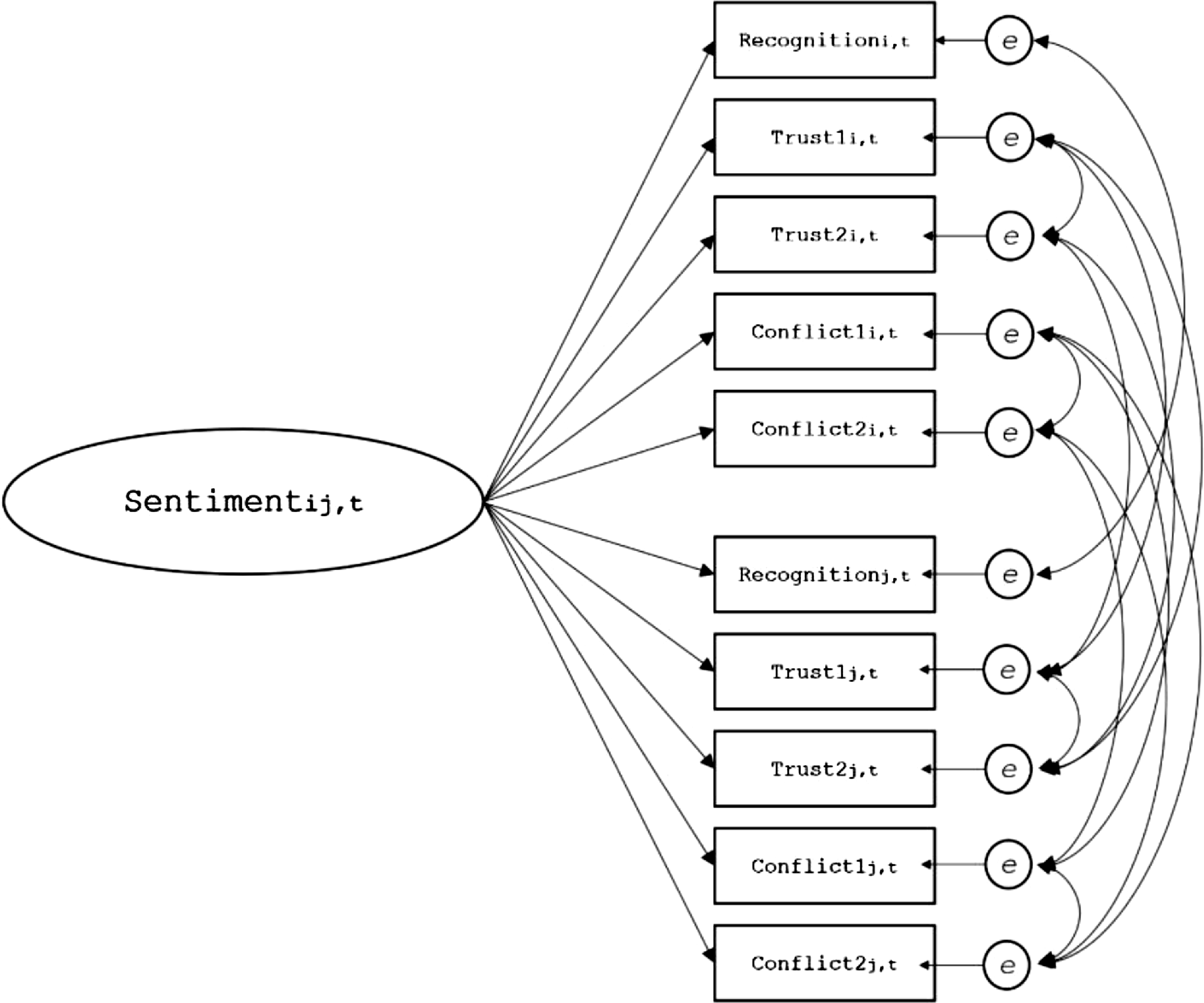
Figure A1. CFA structure for dyadic relationship sentiment.
Because relationship sentiment may have different meanings for the types of dyads studied here, we performed tests of measurement invariance and compared the fit of different CFA structures where measurement was allowed to vary across dyad types. A CFA structure assuming no underlying grouping by type of dyad at all resulted in the highest model fit (Chi2 = 806; df = 21) and was selected for all subsequent analyses. A structure with configural invariance resulted in a Chi2 Model Fit of 922 (df = 65), metric invariance produced Chi2 = 1280 with df = 83, and scalar invariance with Chi2 = 8,465 with df = 99. Likelihood-ratio comparisons of model fit between any of these models resulted in highly significant differences. Additional analyses confirm that main results are largely robust to how the factor is constructed. Table A6 shows that analyses based on factor scores with configural invariance (free loadings and intercepts across dyad types) reproduced the finding that comparisons across families produce patterns resembling structural balance, but these patterns disappear when within-family comparisons are applied.
Table A4. Measurement component of the factor structure

Table A5. Factor scores for dyadic relationship sentiment, by Dyad
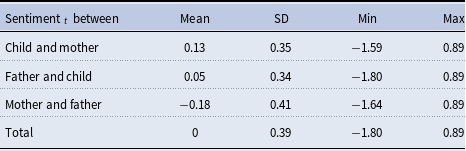
Note: Information is pooled across waves and families.
A.2. Simulation study
The aim of this simulation is to evaluate the claim that time-constant individual attributes can confound effects of structural balance in between effects regressions, but not in fixed-effects regressions. Three scenarios are created in synthetic data: (1) A “randomness” scenario, (2) a scenario with individual and group-derived conflict, and (3) a scenario with individual and group-derived conflict, and additionally imposed structural balance. Subsequently, we test for structural balance in each of these scenarios, comparing results from the between-part of a mixed effects regression with those of the respective within part.
Table A6. Main analyses using factor structure with configural invariance (free loadings and intercepts)

Tested two-sidedly: * p < 0.05, **<0.01, *** p < 0.001. N Observations = 20,076; N Dyads = 5,130; N Families = 1,710.
Note: Analyses control for the variables age, gender, biological parenthood status, and anchor status of i and j; whether additional children live in the household, whether additional adults live in household, wave.
Table A7. Between effects (BE)/within effects (WE) mixed regression analyses for relationship ij,t (simulated data)
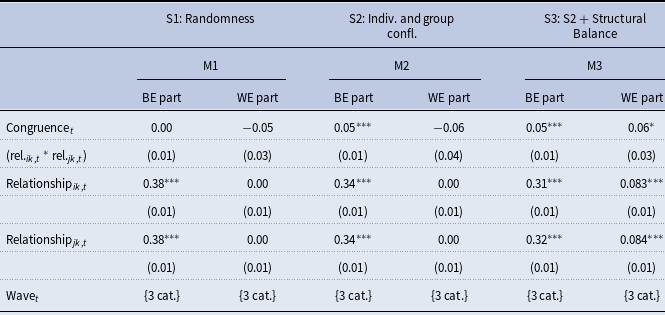
For the randomness scenario 1, we create a simplified world of 1,000 families in which three family members have relationship sentiments with other. Each relationship sentiment in a family constitutes one independent unit of observation, and each relationship sentiment is observed at three time points. A sentiment between any two family members i and j in family f at time point t is assumed to be influenced by four factors: (1) attributes at the family level, (2) attributes of member i, (3) attributes of member j, and (4) a time-varying component of their dyad. For the sake of simplicity, these components are weighted equally so that sentiment ij,f,t reflects the sum of these four components:
sentiment ij,f,t = family f + individual i,f + individual j,f + dyad ij,f,t
Values for the components are randomly assigned and follow normal distributions with a mean of zero and a standard deviation of one. Data are subsequently restructured in an identical manner to the empirical study. Each sentiment ij,f,t in a triad ijk is regressed as a function of the other two, sentiment ik,f,t and sentiment jk,f,t . Due to the family component, sentiments are similar (correlated) at the family level. Due to the individual components, two sentiments involving the same family member are also correlated.
For the individual- and group-derived conflict scenario 2, we repeat the procedure from the randomness scenario, but introduce systematic differences in means across individual and dyadic components. Individual-derived conflict is introduced by randomly choosing one member in 30% of the families and decreasing their individual component score by subtracting 1. Since an individual component affects both relationship sentiments this actor has with two others, it will produce patterns where two relationship sentiments are more negative than the third, hence a spuriously balanced triad. To introduce group-derived conflict, we randomly choose two of the three members in 30% of the families and increase the dyadic component for the two most similar members (in terms of individual attribute scores) by two. These two members will thus have better sentiment with each other due to their attribute similarity. Again, this leads to spuriously balanced patterns where one relationship sentiment is more positive than the two others.
For scenario 3, we introduce randomness and spurious balance identical to scenario 2, but also select 30% of families in the data on which we additionally impose structural balance. This is done by adding one to any sentiment ij,f,t score if (a) both other relationship sentiments in the family have a score above zero, or if (b) both other relationship sentiments in the family have a score below zero. If one of the other relationship sentiments in the family has a score above zero, and the other a score below zero, we subtract one from the sentiment score. Due to this manipulation, triads in selected families at any time point t either resemble patterns where all sentiments are positive, or two are negative while the other is positive. Hence, their sentiments change in accordance with structural balance.
For each of the three scenarios, we run a mixed effects regression model with a time constant between part and a time-varying within part. In an identical fashion to the empirical part of the study, sentiment ij,t is the dependent variable. We introduce a congruence t term to test for structural balance and main effects of sentiment ik, t and sentiment jk, t to control for direct influence. Table A7 presents results from the regressions for each of the three scenarios.
In the randomness scenario, no significant effect of congruence t is observed in either of the regression parts (Model 1). This was expected, since neither structural balance nor confounding attributes are present. In the individual and group-derived conflict scenario, the between effects part of Model 2 shows a positive and significant term for congruence t . Cross-sectionally, structural balance is (erroneously) supported, as individual- and group-derived conflict are entirely responsible for this effect. In the within part of Model 2, however, the effect is close to zero and not significant, showing that individual components do not affect the congruence t term. This is attributable to the fact these individual attributes are time constant, which means that their effects are absorbed by the over-time estimation of the within effects. Lastly, in the scenario with additionally imposed structural balance, the congruence t term is significant and positive across both parts of Model 3. Since in this scenario relationships vary in accordance with structural balance over time, their dynamic is also (correctly) captured by the within effects estimation.
Taken together, the analyses confirm that the congruence t term in between effects analyses may reflect structural balance and influence of individual attributes alike, while congruence t in within effects regressions only reflects structural balance. The simulation thereby shows that cross-sectional approaches are insufficient to disentangle individual and group-derived conflict from structural balance. Within effects analyses of panel data, on the other hand, show effects of structural balance in isolation from the influence of time-constant individual attributes.
A.3. Other covariates used in the main analyses (Table 3)
Table A8. Random effects model (RE) M1 and between effects (BE)/within effects (WE) mixed model M2 for relationship ij,t (SE clustered at the family level)
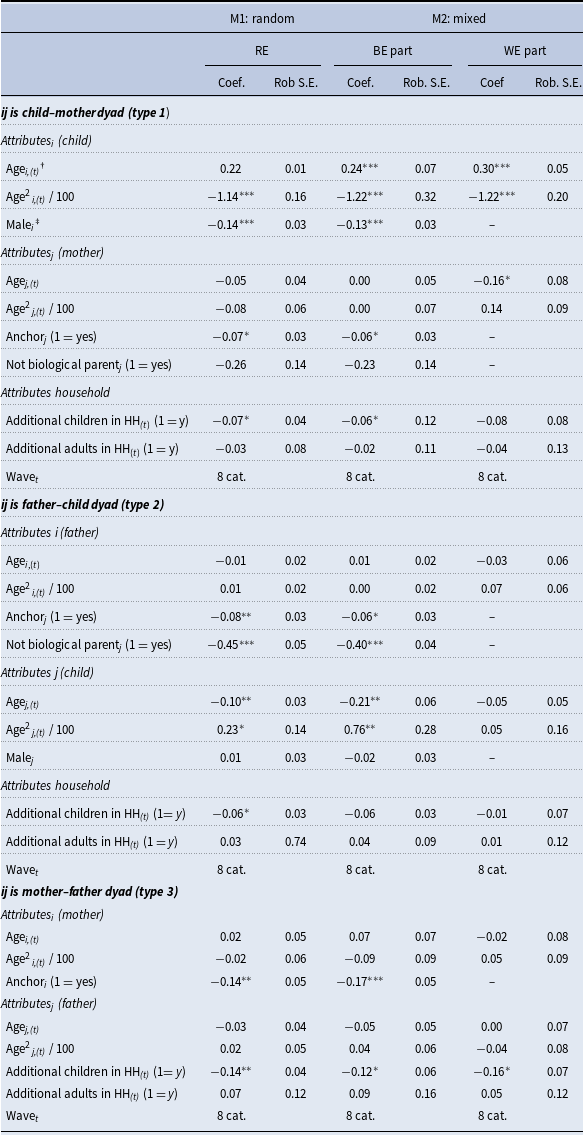
Tested two-sidedly: * p < 0.05, **<0.01, *** p < 0.001. N Observations = 20,076; N Dyads = 5,130; N Families = 1,710.
†Time-variant units in the RE model and in the WE part of the mixed model, but not in the BE part of the mixed model (for all variables with t in parentheses).
‡Time-invariant units are excluded in the within part of the mixed model (hence the empty cells).
Note: None of the coefficients of Wave t for any of the dyad types were significant.
Table A9. Multilevel between effects and fixed-effects analyses for sentimentij,t
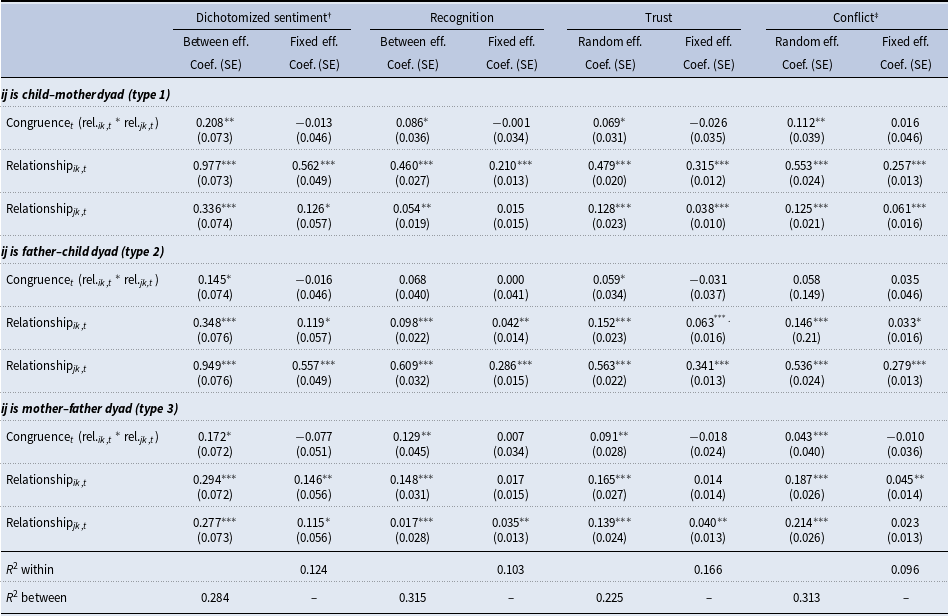
Tested two-sidedly: * p < 0.05, **< 0.01, *** p < 0.001. N Observations = 20,076; N Dyads = 5,130; N Families = 1,710.
†Logistic regression for dichotomized sentiment.
‡Items for conflict are reverse-coded.
A.4. Robustness of findings
We conduct robustness analyses in which dyadic relationship quality is operationalized by a dichotomized version of the relationship sentiment factor score, and by the different relationship dimensions that were used to construct the factor separately (i.e., recognition, trust and conflict). For the dichotomized measure, the mean score of the factor (zero) is taken as the cutoff point and values below zero are assigned a value of minus one. For the separate relationship dimensions models, we use the mean score of items belonging to a respective dimension (recognition: 2 items; trust: 4 items; conflict: 4 items). Items for conflict are reverse-coded to match the scale of the remaining items. For these models, dyadic relationship scores are centered around the grand mean so that low scores have a value below zero, and high scores have a value above zero. This operationalization is comparable to previous triadic studies (de Bel et al., Reference de Bel, Kalmijn and van Duijn2019; Kalmijn, Reference Kalmijn2012). Missing values in the relationship items are imputed using predictive mean matching with two closest neighbors over five runs.
We subsequently conduct mixed effects models including within-family and between-family comparisons in an identical fashion to the main analyses of the study. Similar to the main results, most dyads exhibit balanced patterns when they are compared across families. Only father–child dyads considering recognition or conflict, respectively, are not influenced by congruence in the other two dyads. When dyads are compared within families, the effect of congruence t is not significant in any of the models or dyads under consideration. We conclude that these additional results speak to the robustness of our main findings: namely, that although between-family comparisons may exhibit patterns resembling balance, these patterns may be spurious and explained by time-constant individual characteristics instead. Main analyses with three-person household sample.
To exclude the possibility that influence from additional family members leads to fundamentally different patterns than those observed in the main results, we conducted additional analyses in which we restricted our sample to three-person households with only one child. For comparison with original results see Table 3. As becomes evident from the comparison, results look very similar—with the main difference that between-family congruence terms are no longer significant, suggesting that no cross-sectional tendencies towards balance are found in this sample. We interpret this as evidence that cross-sectional (spurious) congruence may be driven by many-child families. However, the lack of significance may have also resulted from a 82% reduction in sample size that came along with selecting single-child families only, and the associated loss of statistical power.
Table A10. Main analyses for three-person households with one child at time of interview
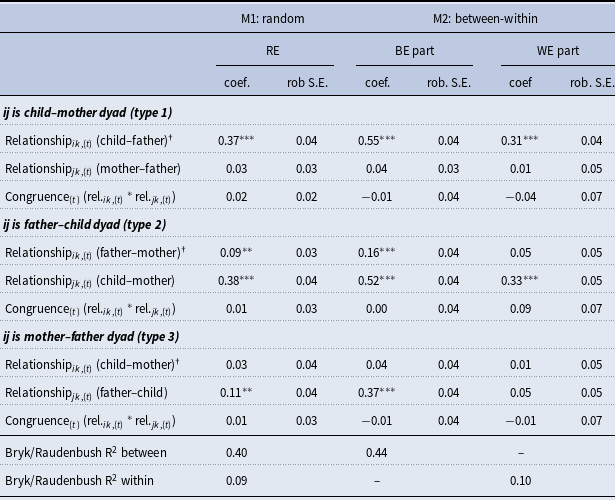
Tested two-sidedly: * p < 0.05, **<0.01, *** p < 0.001. N Observations = 3,702; N Dyads = 1,234; N Families = 318.
†Time-variant variables in the RE model and in the WE part of the b-w model, but not in the BE part of the b-w model (for all variables with t in parentheses).
Note: Analyses control for the variables age, gender, biological parenthood status, and anchor status of i and j; wave.